文章信息
标题:CNN feature based graph convolutional network for weed and crop recognition in smart farming
期刊:《 Computers and Electronics in Agriculture》
第一单位:山东农业大学
在线日期:2020-05-13
Highlights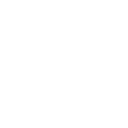
1.提出了一种基于图像的半监督学习方法用于杂草和作物识别;
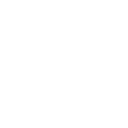
2.在四个不同的杂草数据集上进行了性能评估,准确率高达98.93%,优于传统的CNN方法;
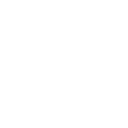
3.该方法可用于类似的识别任务。
摘要除草是提高作物产量的有效方法。准确可靠的杂草识别是精准农业实现高精度定点除草的前提。为了提高杂草和农作物识别的准确率,提出了一种基于CNN特征的图像卷积网络(GCN)识别方法。基于提取的杂草CNN特征及其欧氏距离,构建了GCN图。在半监督学习的基础上,GCN图通过利用已标记和未标记的图像特征来丰富模型,测试样本通过在图上进行传播来从已标记的杂草数据中获取标签信息。GCN-ResNet-101方法在4个不同的杂草数据集上的识别率分别达到97.80%、99.37%、98.93%和96.51%,优于目前最先进的方法(AlexNet、VGG16和ResNet-101)。此外,该方法的运行时间也满足了田间杂草控制的实时性要求。本文提出的基于CNN特征的GCN方法有利于在有限的标签数据下进行多类农作物和杂草的识别,在处理类似的农业识别任务中具有应用潜力。此外,所使用的数据集和源代码是公开的,以便于在田间杂草识别方面的研究。
图3. 基于CNN特征的GCN用于杂草和作物识别流程
AbstractWeeding is an effective way to increase crop yields. Reliable and accurate weed recognition is a prerequisite for achieving high-precision site-specific weed control in precision agriculture. To improve weed and crop recognition accuracy, a CNN feature based graph convolutional network (GCN) based approach is proposed. A GCN graph was constructed based on extracted weed CNN features and their Euclidean distances. Based on the semi-supervised learning, the GCN graph enriched the model by exploiting labeled and unlabeled image features, and testing samples obtain label information from labeled weed data by performing propagation over the graph. The proposed GCN-ResNet-101 approach achieved 97.80%, 99.37%, 98.93% and 96.51% recognition accuracies on four different weed datasets respectively, which outperformed the state-of-the-art methods (AlexNet, VGG16 and ResNet-101). Additionally, the runtime of the proposed approach also satisfies the real-time requirement of field weed control. The proposed CNN feature based GCN approach is favorable for multi-class crops and weeds recognition with limited labeled data, which is a promising approach in dealing with similar agricultural recognition tasks. Furthermore, the used datasets and source code are publicly available to facilitate the research in the recognition of field weeds.