简介: # [scikit-opt](https://github.com/guofei9987/scikit-opt) [](https://pypi.org/project/scikit-opt/) [
安装
pip install scikit-opt
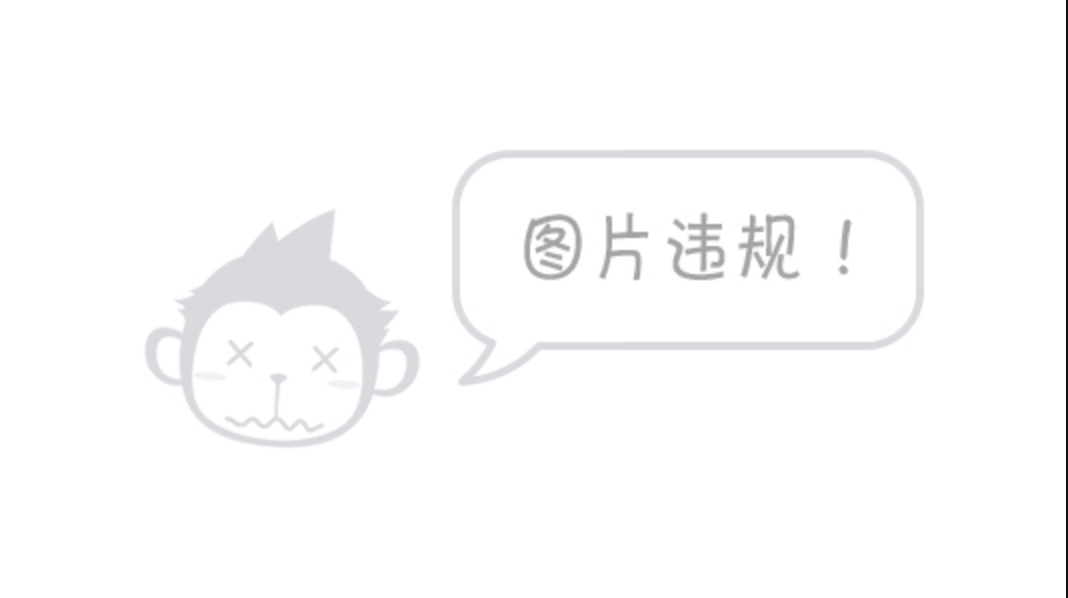
或者直接把源代码中的 sko
文件夹下载下来放本地也调用可以
特性
特性1:UDF(用户自定义算子)
举例来说,你想出一种新的“选择算子”,如下
-> Demo code: examples/demo_ga_udf.py#s1
# step1: define your own operator:
def selection_tournament(algorithm, tourn_size):FitV = algorithm.FitVsel_index = []for i in range(algorithm.size_pop):aspirants_index = np.random.choice(range(algorithm.size_pop), size=tourn_size)sel_index.append(max(aspirants_index, key=lambda i: FitV[i]))algorithm.Chrom = algorithm.Chrom[sel_index, :] # next generationreturn algorithm.Chrom
导入包,并且创建遗传算法实例
-> Demo code: examples/demo_ga_udf.py#s2
import numpy as np
from sko.GA import GA, GA_TSPdemo_func = lambda x: x[0] ** 2 + (x[1] - 0.05) ** 2 + (x[2] - 0.5) ** 2
ga = GA(func=demo_func, n_dim=3, size_pop=100, max_iter=500, lb=[-1, -10, -5], ub=[2, 10, 2],precision=[1e-7, 1e-7, 1])
把你的算子注册到你创建好的遗传算法实例上
-> Demo code: examples/demo_ga_udf.py#s3
ga.register(operator_name='selection', operator=selection_tournament, tourn_size=3)
scikit-opt 也提供了十几个算子供你调用
-> Demo code: examples/demo_ga_udf.py#s4
from sko.operators import ranking, selection, crossover, mutationga.register(operator_name='ranking', operator=ranking.ranking). \register(operator_name='crossover', operator=crossover.crossover_2point). \register(operator_name='mutation', operator=mutation.mutation)
做遗传算法运算
-> Demo code: examples/demo_ga_udf.py#s5
best_x, best_y = ga.run()
print('best_x:', best_x, '\n', 'best_y:', best_y)
现在 udf 支持遗传算法的这几个算子:
crossover
,mutation
,selection
,ranking
Scikit-opt 也提供了十来个算子,参考这里
提供一个面向对象风格的自定义算子的方法,供进阶用户使用:
-> Demo code: examples/demo_ga_udf.py#s6
class MyGA(GA):def selection(self, tourn_size=3):FitV = self.FitVsel_index = []for i in range(self.size_pop):aspirants_index = np.random.choice(range(self.size_pop), size=tourn_size)sel_index.append(max(aspirants_index, key=lambda i: FitV[i]))self.Chrom = self.Chrom[sel_index, :] # next generationreturn self.Chromranking = ranking.rankingdemo_func = lambda x: x[0] ** 2 + (x[1] - 0.05) ** 2 + (x[2] - 0.5) ** 2
my_ga = MyGA(func=demo_func, n_dim=3, size_pop=100, max_iter=500, lb=[-1, -10, -5], ub=[2, 10, 2],precision=[1e-7, 1e-7, 1])
best_x, best_y = my_ga.run()
print('best_x:', best_x, '\n', 'best_y:', best_y)
特性2: GPU 加速
GPU加速功能还比较简单,将会在 1.0.0 版本大大完善。
有个 demo 已经可以在现版本运行了: https://github.com/guofei9987/scikit-opt/blob/master/examples/demo_ga_gpu.py
特性3:断点继续运行
例如,先跑10代,然后在此基础上再跑20代,可以这么写:
from sko.GA import GAfunc = lambda x: x[0] ** 2
ga = GA(func=func, n_dim=1)
ga.run(10)
ga.run(20)
快速开始
1. 差分进化算法
Step1:定义你的问题,这个demo定义了有约束优化问题
-> Demo code: examples/demo_de.py#s1
'''
min f(x1, x2, x3) = x1^2 + x2^2 + x3^2
s.t.x1*x2 >= 1x1*x2 <= 5x2 + x3 = 10 <= x1, x2, x3 <= 5
'''def obj_func(p):x1, x2, x3 = preturn x1 ** 2 + x2 ** 2 + x3 ** 2constraint_eq = [lambda x: 1 - x[1] - x[2]
]constraint_ueq = [lambda x: 1 - x[0] * x[1],lambda x: x[0] * x[1] - 5
]
Step2: 做差分进化算法
-> Demo code: examples/demo_de.py#s2
from sko.DE import DEde = DE(func=obj_func, n_dim=3, size_pop=50, max_iter=800, lb=[0, 0, 0], ub=[5, 5, 5],constraint_eq=constraint_eq, constraint_ueq=constraint_ueq)best_x, best_y = de.run()
print('best_x:', best_x, '\n', 'best_y:', best_y)
2. 遗传算法
第一步:定义你的问题
-> Demo code: examples/demo_ga.py#s1
import numpy as npdef schaffer(p):'''This function has plenty of local minimum, with strong shocksglobal minimum at (0,0) with value 0'''x1, x2 = px = np.square(x1) + np.square(x2)return 0.5 + (np.sin(x) - 0.5) / np.square(1 + 0.001 * x)
第二步:运行遗传算法
-> Demo code: examples/demo_ga.py#s2
from sko.GA import GAga = GA(func=schaffer, n_dim=2, size_pop=50, max_iter=800, lb=[-1, -1], ub=[1, 1], precision=1e-7)
best_x, best_y = ga.run()
print('best_x:', best_x, '\n', 'best_y:', best_y)
第三步:用 matplotlib 画出结果
-> Demo code: examples/demo_ga.py#s3
import pandas as pd
import matplotlib.pyplot as pltY_history = pd.DataFrame(ga.all_history_Y)
fig, ax = plt.subplots(2, 1)
ax[0].plot(Y_history.index, Y_history.values, '.', color='red')
Y_history.min(axis=1).cummin().plot(kind='line')
plt.show()
2.2 遗传算法用于旅行商问题
GA_TSP
针对TSP问题重载了 交叉(crossover)
、变异(mutation)
两个算子
第一步,定义问题。
这里作为demo,随机生成距离矩阵. 实战中从真实数据源中读取。
-> Demo code: examples/demo_ga_tsp.py#s1
import numpy as np
from scipy import spatial
import matplotlib.pyplot as pltnum_points = 50points_coordinate = np.random.rand(num_points, 2) # generate coordinate of points
distance_matrix = spatial.distance.cdist(points_coordinate, points_coordinate, metric='euclidean')def cal_total_distance(routine):'''The objective function. input routine, return total distance.cal_total_distance(np.arange(num_points))'''num_points, = routine.shapereturn sum([distance_matrix[routine[i % num_points], routine[(i + 1) % num_points]] for i in range(num_points)])
第二步,调用遗传算法进行求解
-> Demo code: examples/demo_ga_tsp.py#s2
from sko.GA import GA_TSPga_tsp = GA_TSP(func=cal_total_distance, n_dim=num_points, size_pop=50, max_iter=500, prob_mut=1)
best_points, best_distance = ga_tsp.run()
第三步,画出结果:
-> Demo code: examples/demo_ga_tsp.py#s3
fig, ax = plt.subplots(1, 2)
best_points_ = np.concatenate([best_points, [best_points[0]]])
best_points_coordinate = points_coordinate[best_points_, :]
ax[0].plot(best_points_coordinate[:, 0], best_points_coordinate[:, 1], 'o-r')
ax[1].plot(ga_tsp.generation_best_Y)
plt.show()
3. 粒子群算法
(PSO, Particle swarm optimization)
3.1 带约束的粒子群算法
第一步,定义问题
-> Demo code: examples/demo_pso.py#s1
def demo_func(x):x1, x2, x3 = xreturn x1 ** 2 + (x2 - 0.05) ** 2 + x3 ** 2
第二步,做粒子群算法
-> Demo code: examples/demo_pso.py#s2
from sko.PSO import PSOpso = PSO(func=demo_func, dim=3, pop=40, max_iter=150, lb=[0, -1, 0.5], ub=[1, 1, 1], w=0.8, c1=0.5, c2=0.5)
pso.run()
print('best_x is ', pso.gbest_x, 'best_y is', pso.gbest_y)
第三步,画出结果
-> Demo code: examples/demo_pso.py#s3
import matplotlib.pyplot as pltplt.plot(pso.gbest_y_hist)
plt.show()
↑see examples/demo_pso.py
3.2 不带约束的粒子群算法
-> Demo code: examples/demo_pso.py#s4
pso = PSO(func=demo_func, dim=3)
fitness = pso.run()
print('best_x is ', pso.gbest_x, 'best_y is', pso.gbest_y)
4. 模拟退火算法
(SA, Simulated Annealing)
4.1 模拟退火算法用于多元函数优化
第一步:定义问题
-> Demo code: examples/demo_sa.py#s1
demo_func = lambda x: x[0] ** 2 + (x[1] - 0.05) ** 2 + x[2] ** 2
第二步,运行模拟退火算法
-> Demo code: examples/demo_sa.py#s2
from sko.SA import SAsa = SA(func=demo_func, x0=[1, 1, 1], T_max=1, T_min=1e-9, L=300, max_stay_counter=150)
best_x, best_y = sa.run()
print('best_x:', best_x, 'best_y', best_y)
第三步,画出结果
-> Demo code: examples/demo_sa.py#s3
import matplotlib.pyplot as plt
import pandas as pdplt.plot(pd.DataFrame(sa.best_y_history).cummin(axis=0))
plt.show()
另外,scikit-opt 还提供了三种模拟退火流派: Fast, Boltzmann, Cauchy. 更多参见 more sa
4.2 模拟退火算法解决TSP问题(旅行商问题)
第一步,定义问题。(我猜你已经无聊了,所以不黏贴这一步了)
第二步,调用模拟退火算法
-> Demo code: examples/demo_sa_tsp.py#s2
from sko.SA import SA_TSPsa_tsp = SA_TSP(func=cal_total_distance, x0=range(num_points), T_max=100, T_min=1, L=10 * num_points)best_points, best_distance = sa_tsp.run()
print(best_points, best_distance, cal_total_distance(best_points))
第三步,画出结果
-> Demo code: examples/demo_sa_tsp.py#s3
from matplotlib.ticker import FormatStrFormatterfig, ax = plt.subplots(1, 2)best_points_ = np.concatenate([best_points, [best_points[0]]])
best_points_coordinate = points_coordinate[best_points_, :]
ax[0].plot(sa_tsp.best_y_history)
ax[0].set_xlabel("Iteration")
ax[0].set_ylabel("Distance")
ax[1].plot(best_points_coordinate[:, 0], best_points_coordinate[:, 1],marker='o', markerfacecolor='b', color='c', linestyle='-')
ax[1].xaxis.set_major_formatter(FormatStrFormatter('%.3f'))
ax[1].yaxis.set_major_formatter(FormatStrFormatter('%.3f'))
ax[1].set_xlabel("Longitude")
ax[1].set_ylabel("Latitude")
plt.show()
咱还有个动画
↑参考代码 examples/demo_sa_tsp.py
5. 蚁群算法
蚁群算法(ACA, Ant Colony Algorithm)解决TSP问题
-> Demo code: examples/demo_aca_tsp.py#s2
from sko.ACA import ACA_TSPaca = ACA_TSP(func=cal_total_distance, n_dim=num_points,size_pop=50, max_iter=200,distance_matrix=distance_matrix)best_x, best_y = aca.run()
6. 免疫优化算法
(immune algorithm, IA)
-> Demo code: examples/demo_ia.py#s2
from sko.IA import IA_TSPia_tsp = IA_TSP(func=cal_total_distance, n_dim=num_points, size_pop=500, max_iter=800, prob_mut=0.2,T=0.7, alpha=0.95)
best_points, best_distance = ia_tsp.run()
print('best routine:', best_points, 'best_distance:', best_distance)
7. 人工鱼群算法
人工鱼群算法(artificial fish swarm algorithm, AFSA)
-> Demo code: examples/demo_afsa.py#s1
def func(x):x1, x2 = xreturn 1 / x1 ** 2 + x1 ** 2 + 1 / x2 ** 2 + x2 ** 2from sko.AFSA import AFSAafsa = AFSA(func, n_dim=2, size_pop=50, max_iter=300,max_try_num=100, step=0.5, visual=0.3,q=0.98, delta=0.5)
best_x, best_y = afsa.run()
print(best_x, best_y)
原文链接
本文为阿里云原创内容,未经允许不得转载。