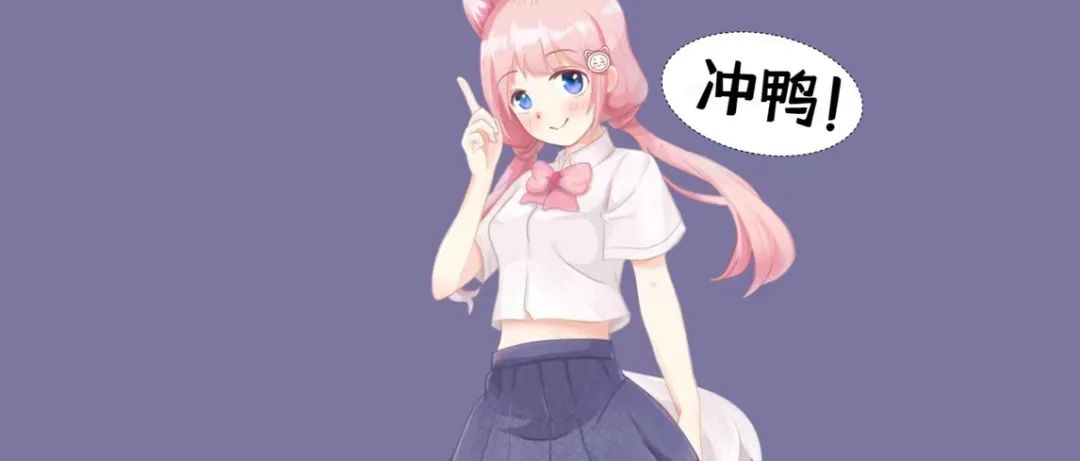
文 | 卖萌酱
大家好,我是卖萌酱。
昨天在知乎timeline上刷到一个问题:
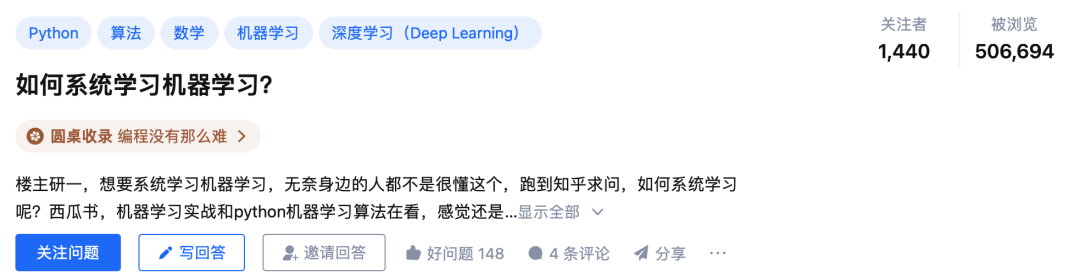
虽然卖萌酱已经不需要系统学习机器学习了,但无意间发现最高赞的id竟然叫“吴恩达”??
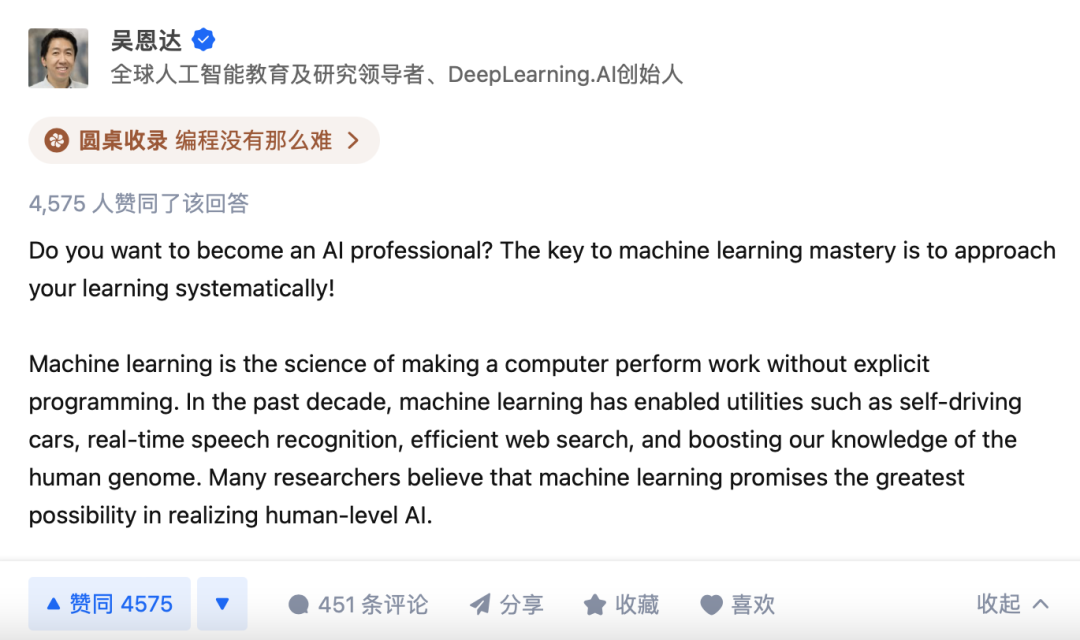
好家伙,看了看回答日期,是4月8号。
戳进去主页...
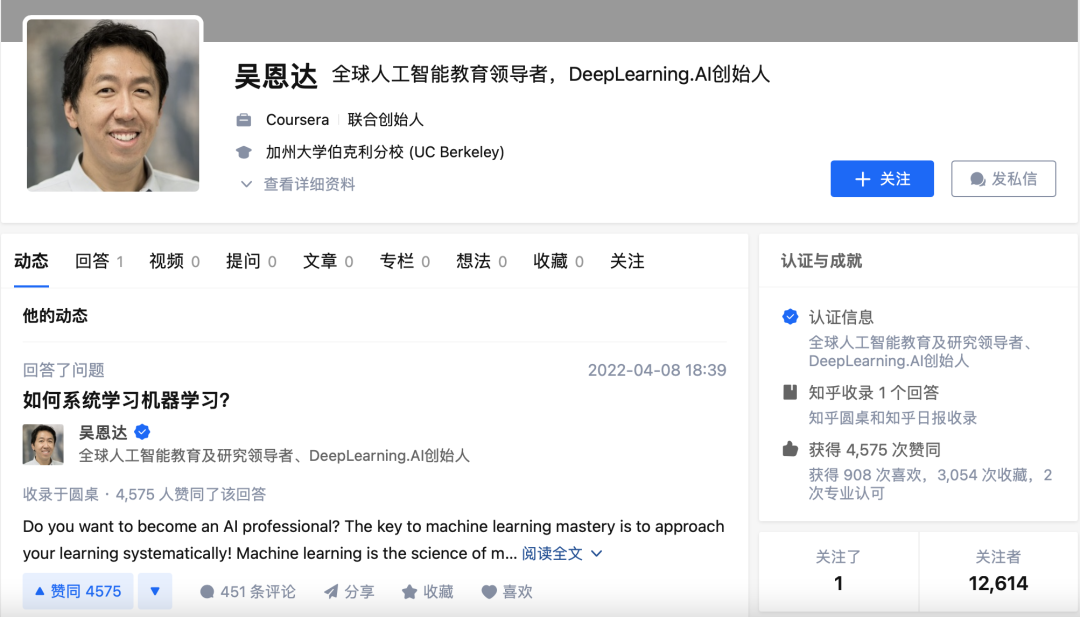
回答 1,关注者12614...
原来真的是本尊入驻了😂
翻了翻大佬的知乎首答评论区,发现把知乎CEO周源也炸出来了,大佬的能量果然很在线
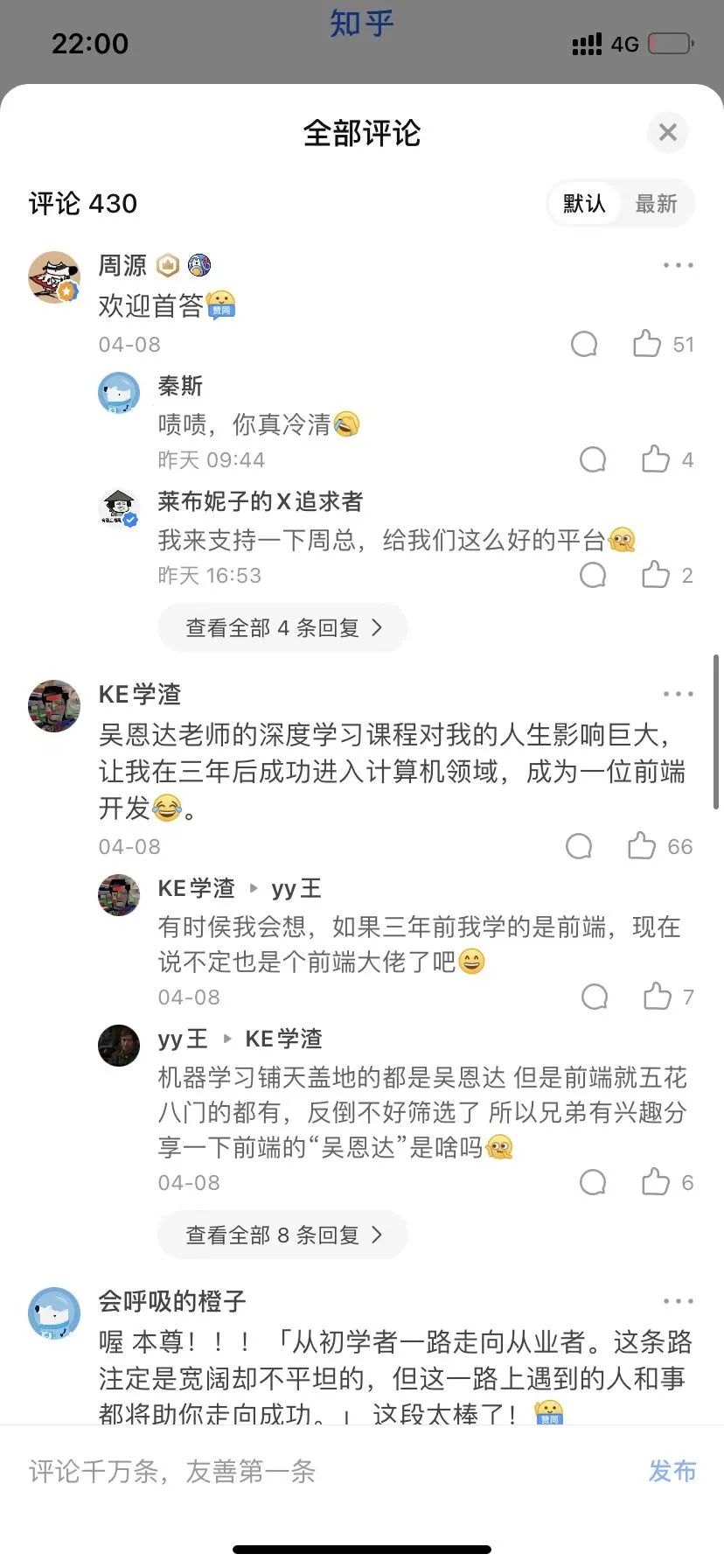
于是,本着回味青春的目的,卖萌酱开始认真看起了大佬的首答,竟然是中英双语的,这里转载一下:
Do you want to become an AI professional? The key to machine learning mastery is to approach your learning systematically!
Machine learning is the science of making a computer perform work without explicit programming. In the past decade, machine learning has enabled utilities such as self-driving cars, real-time speech recognition, efficient web search, and boosting our knowledge of the human genome. Many researchers believe that machine learning promises the greatest possibility in realizing human-level AI.
Here, I‘d like to share three steps to learn machine learning in a systematic way: First, you should learn coding basics. Second, you should study machine learning and deep learning. Third, you should focus on the role you would like to have. Fundamental programming skills are a prerequisite for building machine learning systems. You will need to be able to write a simple computer program (function calls, for loops, conditional statements, basic mathematical operations) before you can start implementing preliminary machine learning algorithms. Knowing more math can give you an edge, but it won’t be necessary to spend much time on specific mathematical issues such as linear algebra, probability and statistics.
Having gained some fundamental coding skills, you can officially begin your journey of machine learning. My Machine Learning course from Stanford University is a great choice. It provides a general introduction to machine learning, data mining, and the statistical approach of pattern recognition. The course will also help you to develop your practical understanding of how to use machine learning in the real world. For instance, when to use supervised learning, unsupervised learning, and machine learning. The machine learning course draws insights from numerous case studies and applications. It is suitable for learning how to apply algorithms to a wide-variety of tasks, such as intelligent robots building (perception, control), natural language understanding (web search, anti-spam emails), computer vision (identifying diseases in medical imagery, finding defects in manufacturing), and much more.
Deep learning is a subset of machine learning that is growing more important, and is worth your attention as well. It uses neural networks to make powerful predictions, and is the driving force behind many of today’s most exciting technologies. For example, self-driving cars, advanced web search, and face recognition all use deep learning. The Deep Learning Specialization, developed by DeepLearning.AI, covers the knowledge you need to build deep learning applications in fields such as computer vision, natural language processing, and speech recognition. You will conduct case studies in healthcare, autonomous driving, sign language reading, music creation, and natural language processing, so you can familiarize yourself with the practical application of deep learning in various industries while mastering theoretical knowledge at the same time.
Once you have learned the foundations of machine learning and deep learning, the next move depends on the role you have in mind. For example, do you want to be a data scientist, engineer, or machine learning researcher? Or, do you consider developing AI skills to complement your existing expertise? If so, you can learn AI as a way to better apply your expertise to real-world problems.
After deciding the role, it's time to move on to real practice. You’ll want to get experience working on projects and as a part of a team. Identifying viable and valuable projects is an important skill, and it’s one that you’ll continue to develop throughout your career. The best way to start is to volunteer to help with other peoples’ projects. Eventually you will develop the confidence and experience to lead your own. For completing a project, teamwork is more likely to succeed than solo effort. It is critical to have the ability to collaborate with others, give and take advice, as this helps you build connections. Teamwork also helps you build out your network of professional connections. You can call on people who you have worked with in the past to provide advice and support as you move through your career.
The ultimate goal, of course, is to find a job in machine learning. This will come after you have acquired both theoretical knowledge as well as practical experience. When looking for a job, don’t be shy about reaching out to people you have met while taking courses or working on projects. You can also connect directly with professionals who are already working in the field. Many of them are happy to act as your mentor. Finding your first job, however, is a small step in a long-term career. It is important to cultivate self-discipline and commit to constant learning. People around you may not be able to tell whether you spend your weekends studying or on your smartphone, but day by day, and year over year, it will make a difference. Discipline ensures that you move forward while staying healthy.
I hope these suggestions could open the door to machine learning and help get you job-ready. The journey ahead will surely be a bumpy one, but rest assured that what you encounter along the way will help you succeed.
By the way, courses from DeepLearning.AI will be available on Zhihu soon. Stay tuned and see you next time!
Keep Learning!
Andrew
想要成为一名人工智能从业者?系统学习机器学习是重点!
机器学习是一门不需要进行明确编程就能使计算机发挥作用的科学。在过去的十年里,机器学习已经为我们提供了自动驾驶汽车、实时语音识别、高效网络搜索等实用工具,并帮助我们极大地提升了对人类基因组的认知。许多研究人员都认为发展机器学习是向人类水平的人工智能迈进的最好方式。
这里向大家提供三个系统学习机器学习的步骤:学习基础编码知识、学习机器学习及深度学习、专注于一个角色。
想要成功构建机器学习系统,基本的编程技能是先决条件。在开始实践简单的机器学习算法之前,你需要具备编写一个简单的计算机程序(函数调用,for loops,条件语句,基本的数学操作)的能力。虽然掌握更多数学知识能让你更具优势,但也不必将精力过多投入到诸如线性代数、概率和统计这样的数学基础上。
在学习了基础编码知识后,就可以正式开始你的机器学习之旅了。由斯坦福大学推出的“机器学习课程”是你不错的选择。该课程提供了对机器学习、数据挖掘和统计模式识别的广泛介绍,能帮助大家有效构建对机器学习的认知和理解。主要内容包括:监督学习、无监督学习和机器学习的最佳实践。
该课程从大量的案例研究和应用中汲取经验,便于大家学习如何将学习算法应用于构建智能机器人(感知、控制)、文本理解(网络搜索、反垃圾邮件)、计算机视觉等任务。
此外,深度学习也是你需要涉猎的领域。由DeepLearning.AI开发的“深度学习专业课程”涵盖了你在计算机视觉、自然语言处理和语音识别等领域构建应用程序所需的知识。你将从医疗保健、自动驾驶、手语阅读、音乐生成和自然语言处理等方面开展案例研究,以便于在掌握理论知识的基础上了解深度学习在各行业中的实际应用。
当你对机器学习和深度学习都有了较为深入的学习后,下一步行动将取决于你心中想要成为的角色,例如成为数据科学家、机器学习工程师或机器学习研究员等,亦或是将所学的AI技能与你目前从事的工作相结合,将人工智能更好地应用于现实世界问题。
确定角色之后就要迈入真正的实践环节了。对此,项目选择和团队合作至关重要。确定可行和有价值的项目是一个重要的步骤,必须在你的职业生涯中反复实践。在完成项目的过程中,团队合作比单打独斗更容易取得成功。与他人合作、提供及听取建议的能力至关重要,这能帮助你在协作过程中建立广泛的关系网。当你需要帮助或建议的时候,拥有一个强大的职业关系网能够助你前行。
在积累了一定的机器学习理论知识和实践经验后,找到一份相关的工作看似是每个人的最终目标,但它只是漫长职业生涯中的一小步。你需要保持自律,不断学习。身边的人并不清楚你把周末的时间是用在学习还是刷手机上了,但随着时间的推移,他们终将注意到差异。自律的生活可以帮助你在保持健康的同时继续进步。
希望上述建议能为你打开机器学习的大门,从初学者一路走向从业者。这条路注定是宽阔却不平坦的,但这一路上遇到的人和事都将助你走向成功。
我的深度学习相关课程也将在近期登录知乎,敬请关注,我们下次再见!
请继续学习!
吴恩达
最后用一张图结尾:
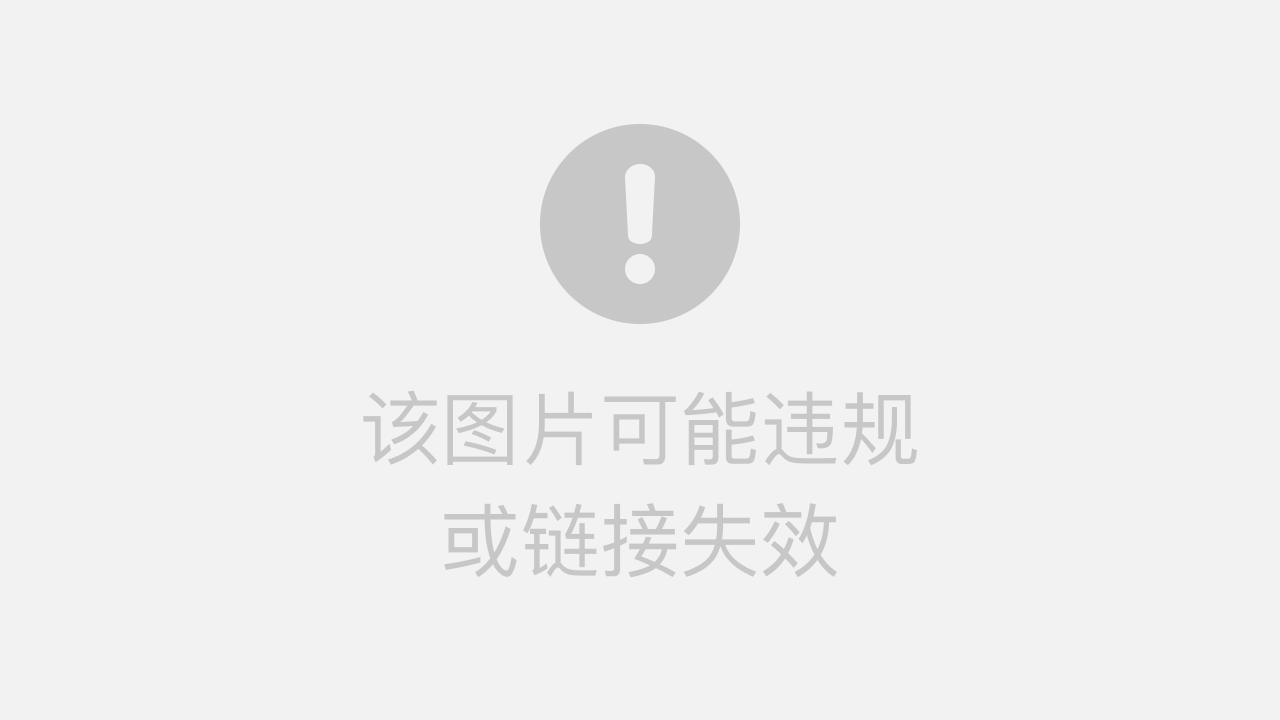
后台回复关键词【入群】
加入卖萌屋NLP、CV、搜推广与求职讨论群
后台回复关键词【顶会】
获取ACL、CIKM等各大顶会论文集!