地图 c-suite
We’ve all seen a great picture capture an audience of stakeholders.
我们所有人都看到了吸引利益相关者听众的美好画面。

It’s such a potent effect that Edward Tufte makes a living deconstructing it. When a great visualization is used in a presentation it has a magnetic pull on the group. It generates discussion and deep study (I’d count focus on a picture lasting more than 30 seconds or so as deep study in a typical meeting). In the right context some charts serve as complete, stand-alone executive summaries.
爱德华·塔夫特(Edward Tufte)靠这种强大的作用来谋求解构。 在演示中使用出色的可视化效果时,会在组上产生磁性吸引力。 它会引发讨论和深入研究(我希望将重点放在持续30秒钟以上的图片上,就像在典型会议中进行深入研究一样)。 在适当的情况下,某些图表可以作为完整的独立执行摘要。
Network models offer this kind of clear and compelling visualization, making them very well suited for the executive audience. But these models are far more than a pretty picture. They are generative models allowing for simulation and inference with efficient automated model discovery methods. Under generally accepted causality frameworks network models estimate causal effects. Any one of those attributes makes for an analytics tool worth using, but having all of them in one method is truly exceptional
网络模型提供了这种清晰而引人注目的可视化效果,非常适合高管受众。 但是这些模型远不止是一张漂亮的图画。 它们是生成模型,可以使用有效的自动模型发现方法进行仿真和推断。 在普遍接受的因果关系框架下,网络模型估计因果关系。 这些属性中的任何一个都值得使用一种分析工具,但是将所有这些属性合用一种方法确实是个例外
To demonstrate the power of network models, this article walks through a simple network model of Intel’s financials. We consider the link between net revenue and R&D and advertising expenses as our main focus.
为了演示网络模型的强大功能,本文将介绍英特尔财务的简单网络模型。 我们将净收入与研发和广告费用之间的联系作为我们的主要重点。
Let’s start with the simple network below.
让我们从下面的简单网络开始。

With no coaching at all, I’m willing to bet you immediately understand the key points of the picture to the left: each named dot (‘node’) is a quantity, the arrows (‘edges’) indicate relationships between those quantities, and you probably guessed that the arrows point from cause to effect.
完全没有指导,我敢打赌,您立即了解左侧图片的关键点:每个命名点('node')是一个数量,箭头('edge')表示这些数量之间的关系,您可能会猜到箭头指向的是因果关系。
These nodes are a minimal skeleton of Intel’s financial reporting plus the global retail sales (in units) for one of the company’s key markets. Most labels are self explanatory, but g&A is likely not. It is computed from the reported Advertising, General & Administrative expense line item, with advertising spend subtracted to keep ad spend separate from the other two. The Intel data here is sourced from the 28 annual10-K filings found on SEC.gov covering 1992 to 2019. The global PC sales for the same years were found on wikipedia and ars technica. All values are in millions, and dollar amounts have been adjusted for inflation to the value of the dollar in 2000.
这些节点是英特尔财务报告以及该公司主要市场之一的全球零售额(以单位为单位)的最小框架。 多数标签是不言自明的,但一般管理人员可能不会。 它是根据报告的广告,一般和行政费用行项目计算得出的,减去广告支出后,广告支出便会与其他两项分开。 英特尔数据在这里从28 annual10-K备案采购上找到SEC.gov涵盖1992年至2019年在被发现的全球PC销量为同里维基百科和Ars Technica的 。 所有值均以百万为单位,并且根据通货膨胀将美元金额调整为2000年的美元价值。
In the network, variables are tagged _0 to indicate that all of these represent the same time step; i.e. this network represents relationships between values in the same year.
在网络中,变量被标记为_0,以指示所有这些变量代表相同的时间步长; 即,该网络表示同一年中值之间的关系。
The network structure is learned from the data by a simple hill climbing algorithm with restarts and some trimming, using the AIC as an optimization metric. I’ve done this using R’s bnlearn package’s tabu() function. The method is fast (and applies well to larger networks) but does not guarantee a true global optimum is found. This ends up having some advantages can work to our advantage, leaving us room to apply business knowledge and still obtain a model no worse than the ‘optimal’ one.
使用AIC作为优化指标,通过简单的爬山算法重新启动并进行一些修整,从数据中了解网络结构。 我已经使用R的bnlearn包的tabu()函数完成了此操作。 该方法快速(并且适用于较大的网络),但不能保证找到真正的全局最优值。 最终,有些优势可以发挥我们的优势,给我们留出了应用业务知识的空间,并且仍然获得了不比“最佳”模型更糟糕的模型。
The heart of a network model is showing relationships between variables — even very complex relationships. If a variable is a controllable quantity (e.g. ad spend) then the network shows what quantities will be impacted by changing the controllable quantity. If no path exists between two nodes in the network (not the case for any pair of nodes in our network) then the two quantities are independent of each other, both practically (i.e. we cannot change one to influence the other) and probabilistically (i.e. the distribution of one conditioned on the other is the same as the distribution first quantities distribution). If two nodes do have a path between them there is evidence that information about one node has implications for the other.
网络模型的核心是显示变量之间的关系,甚至是非常复杂的关系。 如果变量是可控制的数量(例如广告支出),则网络会显示更改可控制的数量会影响哪些数量。 如果网络中的两个节点之间不存在路径(我们网络中的任何一对节点都不存在),则这两个数量实际上是相互独立的(即,我们不能改变一个以影响另一个),并且概率是相互独立的(即,一个以另一个为条件的分布与分布第一数量分布相同)。 如果两个节点之间确实有路径,则有证据表明有关一个节点的信息对另一节点有影响。
In our example network, we can see that R&D expenses drive all the other values except advertising and that advertising drives both net revenue and total PC sales. While that’s interesting in and of itself, I think we would all agree that this is expected. Maybe a harder question is are there lasting (i.e. multi-year) effects of ad and research expenses?
在我们的示例网络中,我们可以看到R&D支出驱动着除广告之外的所有其他价值,而广告推动了净收入和PC总销量。 尽管这本身很有趣,但我认为我们都同意这是预期的。 也许更难的问题是广告和研究费用是否具有持久(即多年)的影响?
The model is easily extended to address this; we simply add nodes to cover previous years’ values. I’ll add two more years to our model and see what results. In the visualization below a suffix of “_-1” implies a variable value 1 year before the “_0” value.
该模型很容易扩展以解决此问题。 我们只需添加节点即可覆盖前几年的价值。 我将在模型中再加上两年,看看结果如何。 在下面的图表中,后缀“ _-1”表示“ _0”值之前1年的变量值。

As you explore the multi-year relationships (the link in the caption will get you to an interactive plot that might help make relationships more viewable) you’ll see that are persistent effects from last year’s R&D and indirectly R&D from two years ago via the previous years’ net revenue.
当您探索多年的关系时(标题中的链接将带您到一个互动的情节,这可能有助于使关系更加可见),您将看到这是去年研发的持续影响,以及两年前通过R&D间接产生的影响。前几年的净收入。
We also see that the relationships within a year aren’t the same in each layer. This means that if the current year is 2018, the current ad spend influences net revenue but once the current year is 2019, the 2018 spend no longer influenced 2018 net revenue.
我们还看到,一年之内的关系在每个层中都不相同。 这意味着如果当前年份为2018年,当前广告支出会影响净收入,但是当当前年份为2019年时,2018年支出将不再影响2018年净收入。
Frankly, that doesn’t make a lot of sense. And because I promised a model that immediately made sense, we need to help the structure learning algorithm with some expert advice. I propose to keep the within-year relationship in all years the same as the initial model and let the between year relationships be identified algorithmically. This is simple to do by passing the edges of the single year network as a whitelist dataframe to bnlearn’s structure finding functions.
坦白说,这没有什么意义。 而且因为我承诺了一个可以立即生效的模型,所以我们需要在专家建议下帮助结构学习算法。 我建议将所有年份的年内关系保持与初始模型相同,并通过算法确定年间关系。 通过将单年网络的边缘作为白名单数据帧传递到bnlearn的结构查找功能,可以很容易地做到这一点。

Now we see the original relationships conserved. We also see more connections between the years (each layer here is one year). More arrows mean more parameters, so it is important to note that the AIC of this modified model is very close to auto-magically learned model’s AIC.
现在,我们看到原始关系得以保留。 我们还可以看到年份之间的联系更多(这里的每一层都是一年)。 箭头越多意味着参数越多,因此需要注意的是,此修改模型的AIC非常接近自动学习模型的AIC。
So this ‘proves’ that R&D and advertising expenditures have lasting business impact. Which arms executives of those functions to argue that cutting budgets this year will leave the business hampered for years to come.
因此,这“证明”研发和广告支出具有持久的业务影响。 这些职能部门的哪位高管争论说,今年削减预算将使该业务在未来几年受到阻碍。
Presumably, the follow-up question will be ‘how much will the business be hampered if we make the cuts anyway?’ Our network model allows for inference and simulation by simply setting the values of the decision nodes, our advertising and R&D budgets, and sampling the resulting distribution of the outcome of interest, net revenue.
据推测,后续问题将是“如果我们继续裁员,业务将受到多少阻碍?” 我们的网络模型可以通过简单地设置决策节点的值,我们的广告和R&D预算,以及对利息结果(净收入)的结果分布进行采样来进行推理和模拟。
If all relevant variables are accounted for in the model, the difference in the expected value of the outcome as the value of the decision node varies is the causal impact of varying the decision. We can easily find the historical causal impact of R&D and ad spends by comparing the expected value of net revenue in 2019 with actual spend levels in the last 3 years to the expected value of net revenue with 0 spend for all 3 of those years.
如果在模型中考虑了所有相关变量,那么随着决策节点的值变化,结果的预期值的差异就是决策变化的因果关系。 通过将2019年净收入的预期值与过去3年的实际支出水平进行比较,将这3年中支出为0的净收入的预期值进行比较,可以轻松找到R&D和广告支出的历史因果关系。

Well, this is certainly an unexpected result. A quick investigation shows that the within-year advertising coefficients are positive (as expected) but the between-year advertising coefficients are always negative. I suppose we could rationalize this by saying that ads driving sales this year are stealing from sales in the next year. . .but we can also note that the ad spend in year 0 is highly correlated to the spends in year -1 and year -2. This indicates the estimates for present and past ad spends onto a given net revenue node are subject to the ill effects of collinearity.
好吧,这肯定是出乎意料的结果。 快速调查显示,年内广告系数为正(如预期),但年间广告系数始终为负。 我想我们可以通过说今年推动销售的广告从明年的销售中窃取来合理化。 。 。,但我们还可以注意到,第0年的广告支出与第-1年和-2年的支出高度相关。 这表明对给定净收入节点上的当前和过去广告支出的估算会受到共线性的不良影响。

Given the high correlation, and the unreasonable estimates, we can simply ban previous years’ advertising from having a direct impact on current net revenue. This still leaves room for an indirect influence through, e.g., previous years net revenue. The final network is charted below.
鉴于相关性高且估算值不合理,我们可以简单地禁止前几年的广告直接影响当前的净收入。 这仍然通过例如前几年的净收入为间接影响留下了空间。 最终网络如下图所示。

The AIC is lower than our last model, so we haven’t destroyed our model by removing the offending edges!
AIC比我们的上一个模型低,因此我们还没有通过移除有害的边来破坏我们的模型!
Now we can apply the same ‘turn it off’ approach as before to get the causal impact of R&D and advertising effect on net revenue. This model shows positive impacts all around, aligning with common sense and giving the CMO some relief. Interestingly, R&D is now showing a much stronger positive impact on revenue than before. So everyone is going to prefer this model!
现在,我们可以使用与以前相同的“关闭”方法来获得研发和广告效应对净收入的因果关系。 该模型显示了积极的影响,符合常识,并给CMO带来了一些缓解。 有趣的是,现在研发对收入的积极影响比以前大得多。 所以每个人都会喜欢这种模型!

To wrap up, the models presented here are intentionally simple, and somewhat limited by the publicly available data used in the example.
总结起来,这里介绍的模型是故意简单的,并且受到示例中使用的公开可用数据的限制。
For convenience, we have used bnlearn’s inbuilt fitting functions which limits continuous variales to gaussian distributions. This is not an inherent assumption to network models. More complex networks could ahve different distributions by node (bnlearn can handle this by discretizing continous variables and other software can directly model different distributions). Additionally (but not-additively), the relationships between the nodes could be non-linear whereas in these examples each nodes mean is a linear combination of its parents’ means.
为了方便起见,我们使用了bnlearn的内置拟合函数,该函数将连续变量限制为高斯分布。 这不是网络模型的固有假设。 更复杂的网络可能按节点具有不同的分布(bnlearn可以通过离散化连续变量来处理此问题,其他软件可以直接对不同的分布进行建模)。 另外(但不是累加地),节点之间的关系可以是非线性的,而在这些示例中,每个节点的均值是其父代均值的线性组合。
From the analytics side, it is hard to swallow looking for a global impact of aggregate R&D spend (maybe capex vs working spend should be split out? Perhaps large initiatives should be modeled separately with different possible durations of effect?) or advertising spend (surely B2B and direct to consumer campaigns have potentially different effects over time?). Perhaps we need more years in the network to account for very long term effects? And we almost certainly should add more external factors (Competitor ad spend? Server sales? Merge and Acquisition activity in computer manufacturers?) to the model before claiming we had included all relevant variables influencing net revenue.
从分析的角度来看,很难吞噬研发总支出的全球影响(也许应该将资本支出与工作支出分开?也许应该对大型计划进行建模,并采用不同的有效期限?)或广告支出(一定要确定) B2B和直接面向消费者的运动随着时间的推移可能会产生不同的影响?)。 也许我们需要在网络中花费更多的时间来解决长期影响? 而且,在声称我们已包括影响净收入的所有相关变量之前,我们几乎肯定应该在模型中添加更多外部因素(竞争对手的广告支出,服务器销售,计算机制造商的并购活动)。
But our simplified example serves to demonstrate the flexbility and utility of graph models. And it highlights how fundamental data issues like collinearity are fundamental because they matter no matter the model being built.
但是我们的简化示例用来说明图模型的灵活性和实用性。 它强调了共线性之类的基本数据问题是多么基本,因为无论所构建的模型如何,它们都至关重要。
I hope I have also convinced you of the value of the network diagram itself. It is impossible to over-emphasize how potent it is for gaining an audience’s alignment with a model. Because the audience can really see the model, they almost immediately feel comfortable reasoning with it.
我希望我也已经使您相信网络图本身的价值。 不可能过分强调它对于使观众与模型保持一致的效力。 因为观众可以真正看到模型,所以他们几乎立刻就可以轻松地进行推理了。
Imagine a final presentation where most of the conversation is about your recommendations and not what your model is — wouldn’t that be a nice change of pace?
想象一个最后的演讲,其中大部分对话都是关于您的建议而不是您的模型的,这不是很好的步调吗?
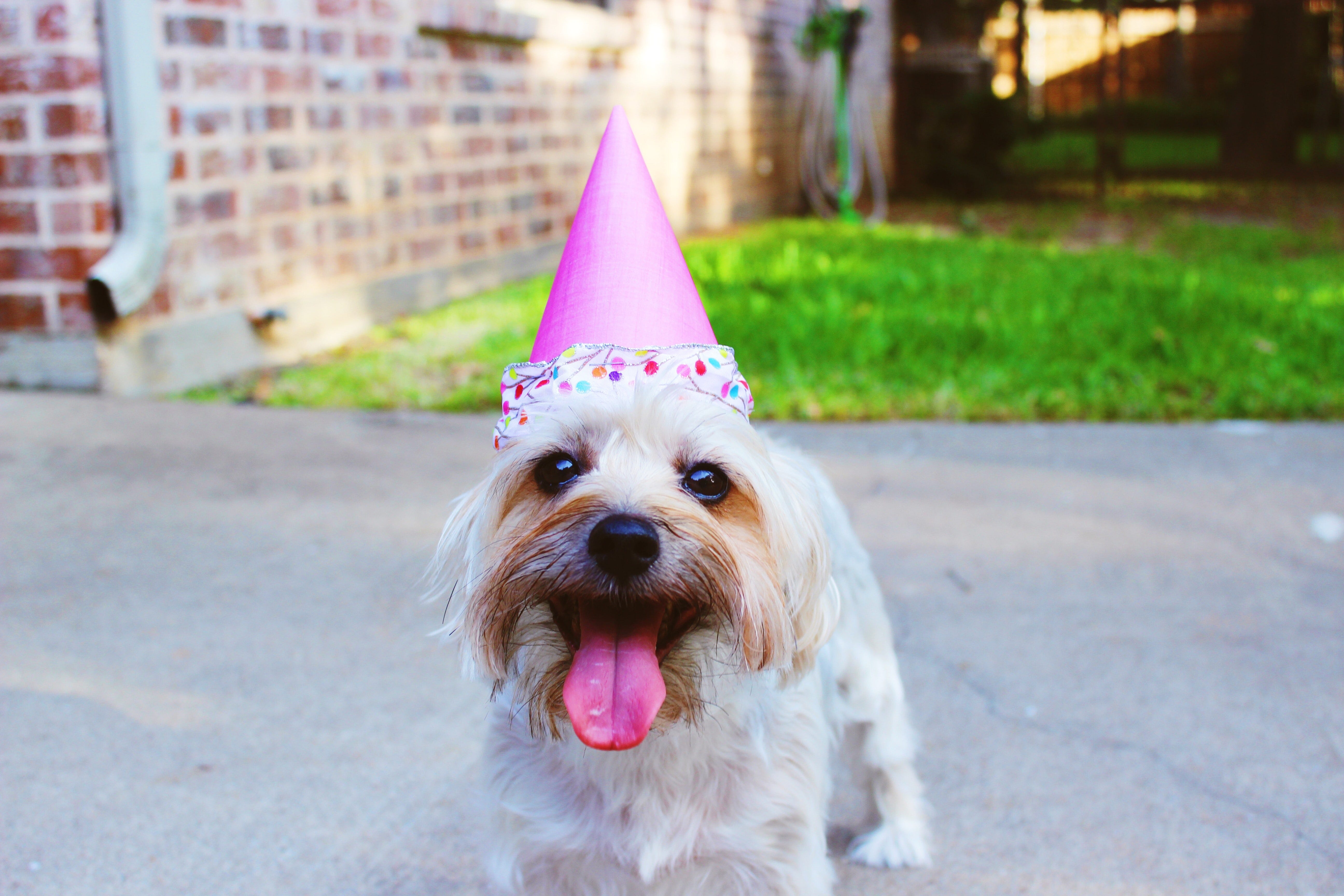
I’ll write a how-to-code-it companion article to this one and future articles on other methods; follow me here on medium for updates.
我将在这篇文章以及其他方法的后续文章中写一篇“如何编码”的配套文章。 在媒体上关注我,以获取更新。
翻译自: https://towardsdatascience.com/a-model-for-the-c-suite-2031a0cecc6d
地图 c-suite
本文来自互联网用户投稿,该文观点仅代表作者本人,不代表本站立场。本站仅提供信息存储空间服务,不拥有所有权,不承担相关法律责任。如若转载,请注明出处:http://www.mzph.cn/news/392048.shtml
如若内容造成侵权/违法违规/事实不符,请联系多彩编程网进行投诉反馈email:809451989@qq.com,一经查实,立即删除!