sap中泰国有预扣税设置吗
Hi! I am Tung, and this is my first stories for my weekend project. What inspired this project is that I have studied to become data scientist for almost two years now mostly from Youtube, coding sites and of course, Medium ,but my learning is just not enough, I need to show what I learnt, so I am here at Medium to post my Weekend project once a week.
嗨! 我是董先生,这是我周末计划的第一个故事。 激发这个项目的原因是,我已经学习成为数据科学家近两年了,主要是从Youtube,编码站点,当然还有中级,但是我的学习还不够,我需要展示我学到的东西,所以我在这里在Medium每周发布一次我的Weekend项目。
Disclaimer: The code is provided at the end of the story, I like to keep the story well-fit for both programmer and non-programmer, so I will not show code in this medium.
免责声明:代码是在故事的结尾提供的,我希望故事适合程序员和非程序员,因此我不会在这种媒介中显示代码。
背景 (Background)
I happen to learn the spatial data from utilize Foursquare API, I thought that it would actually be really useful if I can apply something out of it and, of course, it must be related to business. So I thought what actually be useful application with the location data, then tourism popped up, followed by accommodation, restaurant, pub &bar and else. The tourism location seem legit but it is pain to learn about tourism site, and anyplace is entertainment anyways how can I define a tourism and non-tourism place.( sure, I could, it was just too much for my sweet weekend.) So I want something more simple, something unique, that just gives away its distinction of itself with others. Pub & bar could be like Jazz, rock, modern or else, that sound mass-media stuff but I am not a fan of music, so passed. Accommodation, I don’t know anything about this business side which is surely hard pass. Then I left with restaurant, luckily, it just happen that I live in the country that is rich of culture of beautiful, famous and delicious cuisine, Thailand.
我碰巧是从利用Foursquare API学习空间数据的,我认为如果可以应用其中的某些东西,它实际上将非常有用,当然,它必须与业务有关。 因此,我认为使用位置数据实际上是有用的应用程序,然后出现旅游业,然后是住宿,餐厅,酒吧和酒吧等。 旅游地点似乎合法,但是要了解旅游地点是一件痛苦的事,无论如何在任何地方都是娱乐场所,如何定义旅游和非旅游地点。(当然,我的甜蜜周末实在太多了。)我想要一些更简单,独特的东西,而这仅仅是它与他人之间的区别。 Pub&bar可能像爵士,摇滚,现代音乐之类,听起来像是大众媒体,但我不是音乐迷,所以过去了。 住宿,我对这个业务方面一无所知,这肯定很难。 幸运的是,我离开了饭店,碰巧我住在这个有着美丽,著名和美味佳肴的泰国文化。
I really don’t know why my country’ cuisine happen to be famous in many places, Westerners just crazy about our cuisine, but darn sure it partially because of its deliciousness. Now that I pick my topic “Thai restaurant”, what left is where. It could be anywhere in the world except my country, but It should be some place that is totally far away from my country. But not so much far away in term of cultural difference. So It should be on the side of North American, so I just search for what country that is famous in cultural diversity and it appear that Canada ranks first in North america. And then I search for most diverse city in Canada. Ta Da! it is Toronto.
我真的不知道为什么我国的美食在许多地方都出名,西方人只是为我们的美食而疯狂,但由于其美味而不能肯定。 现在,我选择主题“泰国餐厅”,剩下的就是位置。 它可能在世界上除我的国家以外的任何地方,但应该在一个完全远离我的国家的地方。 但是就文化差异而言,相差不远。 因此,应该在北美这边,所以我只是寻找哪个在文化多样性方面著名的国家,而加拿大似乎在北美排名第一。 然后,我搜索加拿大最多样化的城市。 塔达! 是多伦多。

I still awed by google search every time I see how fast it search, I happen to create a search algorithm which will be the post for next week so just a head-up.
每当我看到搜索速度时,我仍然对Google搜索感到敬畏,我碰巧创建了一个搜索算法,该算法将在下周发布,因此请多加注意。
目的 (Objective)
Now that I have the full topic. I need business objective, what would be something interesting to be know for Thai restaurant in Toronto. I just got the Idea that Thai restaurant in foreigner must not be authentic as at my country this is quite the same for many of other countries’ cuisine such as Japanese, Chinese, Indian, Italian and else, that compensate their authenticity for foreigner taste bud. As I hypothesize the more diverse the society cuisine, the more authenticity it need to be compensated. Thai food is not that hard to make, such a famous dish as Som-Tum, Tom Yum Kung, Tom Kha Kai. It just happen that the combination of ingredient is rare in those country, and this make it an added-value to Western consumer. As a starting point, Thai cuisine is already established it market in Toronto, this might be that chance for entering the market with a unique Thai restaurant that serve real Thai dishes. Now it is clear that I want to open the restaurant, what can a data science tools help us getting closer to the goal.
现在,我有完整的主题。 我需要业务目标,这对于多伦多的泰国餐馆来说是一件有趣的事情。 我刚刚想到,外国人的泰国餐馆一定不能像我所在的国家那样真实,这与其他许多国家/地区的菜肴(例如日本,中国,印度,意大利和其他国家)完全一样,可以弥补他们对外国人味蕾的真实性。 我假设社会美食越多样化,就需要对它的真实性进行补偿。 泰国菜并不难做,像Som-Tum,Tom Yum Kung,Tom Kha Kai这样的著名菜。 碰巧的是,在这些国家,成分的组合很少见,这使它成为西方消费者的附加值。 首先,泰国美食已经在多伦多建立了市场,这可能是一个机会,可以使用一家独特的泰国餐厅来提供真正的泰国菜。 现在很明显,我想开餐厅,数据科学工具可以帮助我们更接近目标。
为什么要定位 (Why location)
If I have to give answer of what is the most important factors for any restaurant to be success, I would say that it is location. (Yes, yes, yes, for multivariate analysis, this is hard to just say it out loud. but I simply state the obvious.) Imagine that, if we open the restaurant as monopoly, we could gain a fortune from our uniqueness, but if we open Thai restaurant in high-Thai-restaurant density, for example Thailand, we lose the uniqueness to the crowd. I would not want that, anyone would not want that, but we happen to see a lot of people do that, so what is the benefit that is worth to lose our uniqueness to the crowd.
如果我必须回答任何一家餐厅取得成功的最重要因素是什么,我会说这是地理位置。 (是的,是的,是的,对于多变量分析,很难大声说出来。但是我只想简单地陈述一下。)想象一下,如果我们以垄断地位开张餐厅,就可以从我们的独特性中获利,但是如果我们以泰国等高泰国餐厅的密度开设泰国餐厅,我们就会失去人群的独特性。 我不想要那个,任何人都不想那个,但是我们碰巧看到很多人这样做,所以值得我们在人群中失去独特性的好处是什么。
It is the market, the existence of Thai restaurant is implied that their is customer for Thai dishes. And the reason that Thai restaurant might happen to open near to each other is because of the customer. There is more costly for a new restaurant to change people preference, it is much more efficient and less costly to simply open where the customer already exist. So where exactly is the best place to open the restaurant. To answer that we first need to know the density of Thai restaurant at Toronto. Now let the coding begin.
在市场上,泰国餐厅的存在暗示着他们是泰国菜的顾客。 泰国餐厅可能碰巧开门的原因是顾客。 一家新餐厅改变人们的喜好成本更高,仅在已有顾客的地方开店,效率更高,成本更低。 因此,确切的说是开餐厅的最佳地点。 要回答这个问题,我们首先需要知道多伦多泰国餐厅的密度。 现在开始编码。
数据 (Data)
The data that will be used here is the postal code for location in Toronto, which we can easily obtain through Wikipedia. The data will look like this.
此处使用的数据是多伦多的邮政编码,我们可以通过Wikipedia轻松获得。 数据将如下所示。

First, we need to clean the data, you can see it here that the Borough and Neighbourhood columns contain missing value which stated by the Postal code, the missing value here is not giving us any more of explanation power or segmentation benefit, so that all we can do is simply delete them. There are neighbourhoods that share the Postal code, so we just combine it together.
首先,我们需要清理数据,您可以在此处看到“自治市镇”和“邻域”列包含邮政编码所说明的缺失值,此处的缺失值并没有给我们更多的解释能力或细分优势,因此我们可以做的就是删除它们。 有些社区共享邮政编码,因此我们将其组合在一起。
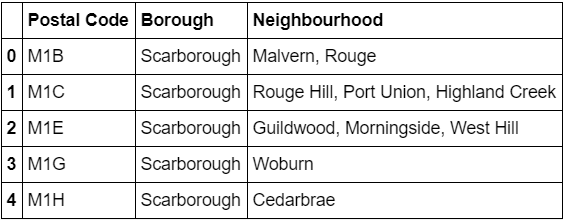
Now we assign the latitude and longitude for this neighbourhood, so that we can use it in Foursquare API to find the location of restaurant nearby.
现在,我们为该邻域分配纬度和经度,以便可以在Foursquare API中使用它来查找附近餐厅的位置。

With this we can start plotting the Borough and Neighbourhood in the map as visualization. This map visualization is used by folium library.
有了这个,我们就可以开始在地图上绘制自治市镇和邻里关系了。 叶片库使用此地图可视化。

The color here does not have any meaning for suggestion opening the restaurant. It is the colors classified by the 4 different Boroughs. Now it is time to find Thai restaurant in these Boroughs. Using the Foursquare API we can obtain venue nearby this 4 Boroughs.
这里的颜色对建议开设餐厅没有任何意义。 它是按4个不同自治市镇分类的颜色。 现在是时候在这些自治市镇找到泰国餐馆了。 使用Foursquare API,我们可以在这四个自治市镇附近找到场地。

Here is the venue from Foursquare, but this is too much of uninterested stuff, what we need is the Thai restaurant.
这是Foursquare的场地,但这太多了无趣的东西,我们需要的是泰国餐厅。

The venue is categorized into 250 categories, which included Thai restaurant, the data is simply dummy variable that has value of 1 if it is that category and 0 if it is not. So it would be just a datasets of row contains 249 0s and single 1.
该场所被分为250个类别,其中包括泰国餐厅,数据只是该变量的虚拟变量,如果是该类别,则值为1;如果不是,则为0。 因此,这将仅仅是包含249个0和单个1的行的数据集。

Here we acquire the number of Thai restaurant in Toronto, which is 13. The number make me feel ambiguous, it does not imply that Thai cuisine is doing well or just getting start. I don’t know whether I can use Foursquare API for historical datasets on location, but that would be one hell of cool analysis.
在这里,我们获得了多伦多的泰国餐馆数量,即13。这个数字让我感到模棱两可,但这并不意味着泰国美食做得很好或刚刚起步。 我不知道是否可以对位置上的历史数据集使用Foursquare API,但这将是很酷的分析之一。
分割 (Segmentation)
Now we have all data we need, the last thing we need to segment the Thai restaurant. When doing the clustering, using human rule of thumb we tend to be biased because of high conditionality, so we just stick to what is easy. But it is different for machine learning algorithm, the algorithm follow the mathematics behind them, in this case we will be using K-mean clustering.
现在,我们有了所需的所有数据,这是分割泰国餐厅的最后一件事。 在进行聚类时,使用人类的经验法则,由于较高的条件性,我们倾向于产生偏见,因此我们只坚持简单易行。 但是机器学习算法有所不同,该算法遵循其背后的数学原理,在这种情况下,我们将使用K均值聚类。
K-mean clustering is the clustering technique used mostly for segmentation, its algorithm start from initialize K number of point as center of segmentation or centroids, for this sense I like to use K = 3 : High, low and zero density segmentation. Then the every point in the datasets will be calculate the distance between itself and this centroid, the data point will be assign to centroids that has least distance to itself and forming a cluster. When they form a cluster the centroid will change the point to the center using mean of its cluster. Then it repeat the whole process again and again until the centroid cannot move. Then that is its best cluster it can have because if the centroid cannot move that would mean that no data point can find the centroid that is nearer than the one it is connected with, which mean the all data points are assign to its closet centroid or best clusters. This is illustrated in the map below.
K均值聚类是主要用于分割的聚类技术,其算法从初始化以分割点或质心的K个点开始,为此,我喜欢使用K = 3:高,低和零密度分割。 然后,将计算数据集中每个点与该质心之间的距离,该数据点将分配给与自身具有最小距离并形成聚类的质心。 当它们形成簇时,质心将使用其簇的均值将点更改为中心。 然后,它一次又一次地重复整个过程,直到质心无法移动为止。 这就是它可能具有的最佳群集,因为如果质心无法移动,则意味着没有数据点可以找到比与其连接的质心更近的质心,这意味着所有数据点都分配给了其壁橱质心或最好的集群。 如下图所示。
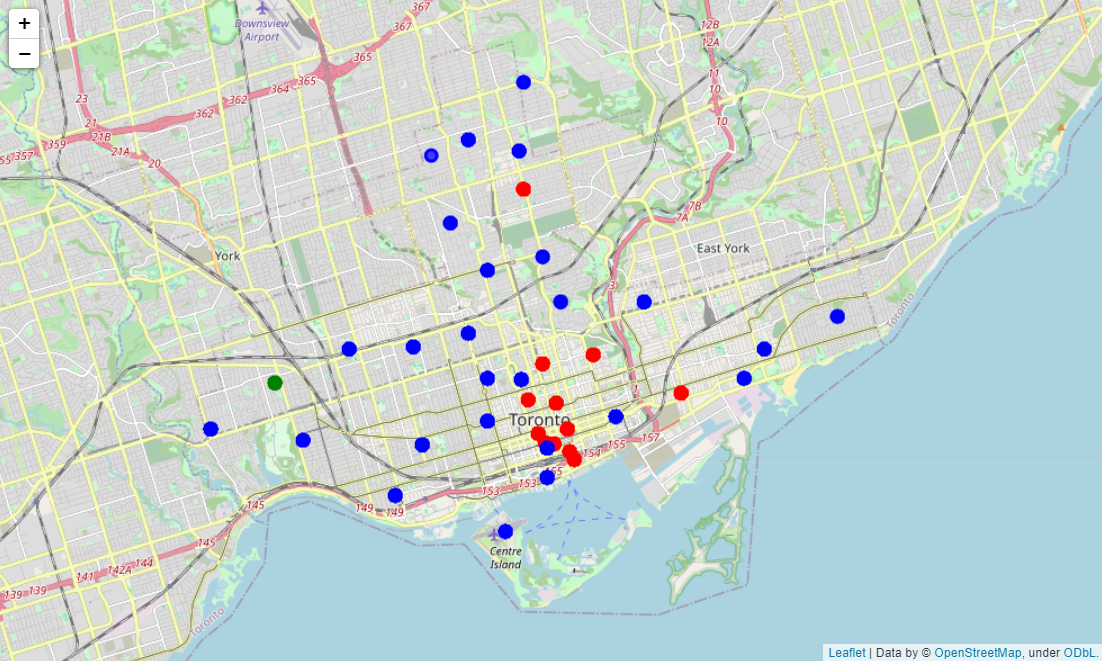
Here we have three clusters, cluster green, blue and red. Taking a look inside this three clusters, by first going through the red one.
在这里,我们有三个聚类,聚类为绿色,蓝色和红色。 首先看一下红色的三个集群,以了解这三个集群。
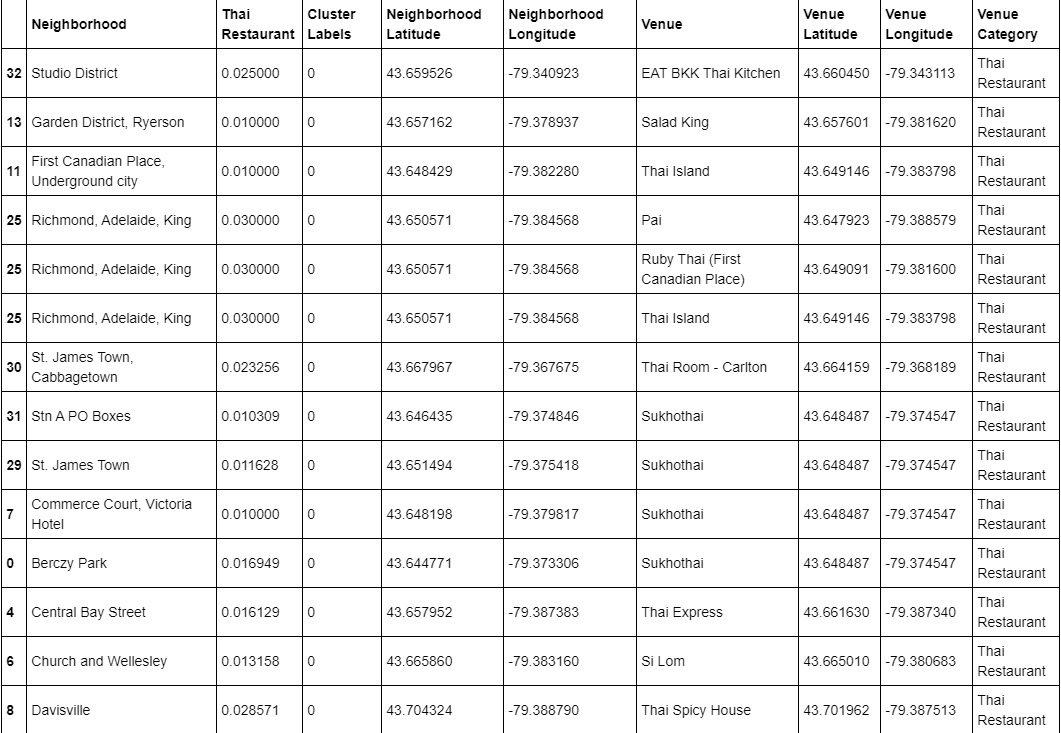
There is 11 Thai restaurant in red cluster out of 13. Which is surely a high density area. As we mentioned before, it is clearly a dead flag area that have too much competition. Now let’s look at green one.
红色的群集中有11处泰国餐厅,其中13处肯定是高密度区域。 正如我们之前提到的,这显然是一个竞争激烈的死角地区。 现在让我们看看绿色的。

The rest of the restaurants are here, this mean the green cluster is low density cluster and the blue is zero density cluster.
其余的餐厅都在这里,这意味着绿色的群集是低密度群集,蓝色的是零密度群集。
我应该在哪里开餐厅? (Where should I open restaurant?)
From the three clusters, it still needs a little more data based on the customer preference instead of location data to verify the best location for opening a restaurant. However, with the given data it would seem to be enough to some extent to suggest opening restaurants either in green or blue clusters.
在这三个集群中,它仍然需要基于客户偏好的更多数据而不是位置数据,以验证开设餐厅的最佳位置。 但是,根据给定的数据,在某种程度上似乎足以建议以绿色或蓝色集群形式开设餐厅。
The reason that it still needs more data is that the revenue generated from the restaurant is not purely based on the location of the restaurant itself, but based on the location of the potential customers. In this sense, the red area has high-density would imply that there are a lot of customers who like Thai cuisine, however opening up there might result in an intense competition that will only drive the cost instead of profit. The superior place should be the subarea (The area near the red cluster, but is not red) which is the blue area surrounding the red area.
它仍然需要更多数据的原因是,从餐馆产生的收入不完全基于餐馆本身的位置,而是基于潜在客户的位置。 从这个意义上说,红色区域具有高密度,这意味着会有很多喜欢泰国菜的顾客,但是在泰国开设这种餐馆可能会导致激烈的竞争,这只会拉高成本而不是利润。 上级位置应该是分区(红色簇附近的区域,但不是红色的区域),也就是围绕红色区域的蓝色区域。
However, we still not know the exact location where should be open in blue area because it is too big, but for the green area which is surprisingly small but contain a guarantee potential customer, this might be a gold mine or just gold nuggets that a small number of restaurant is taking all the profit and by entering this market might result in low or no profit at all. For this perspective, I believe that blue cluster that is close to red cluster is that best location to open restaurant.
但是,我们仍然不知道应该在蓝色区域中打开的确切位置,因为它太大了,但是对于绿色区域而言,该区域很小,但却包含有潜在保证的客户,这可能是金矿,或者仅仅是金块。少数餐馆会利用所有利润,而进入这个市场可能会导致低利润或根本没有利润。 从这个角度来看,我认为靠近红色群集的蓝色群集是开设餐厅的最佳位置。
Here is the code for the story.
这是故事的代码 。
翻译自: https://medium.com/analytics-vidhya/thai-restaurant-density-segmentation-python-with-k-means-clustering-45d299cb3dca
sap中泰国有预扣税设置吗
本文来自互联网用户投稿,该文观点仅代表作者本人,不代表本站立场。本站仅提供信息存储空间服务,不拥有所有权,不承担相关法律责任。如若转载,请注明出处:http://www.mzph.cn/news/392042.shtml
如若内容造成侵权/违法违规/事实不符,请联系多彩编程网进行投诉反馈email:809451989@qq.com,一经查实,立即删除!