多重线性回归 多元线性回归
Video Link
影片连结
We have taken a look at Simple Linear Regression in Episode 4.1 where we had one variable x to predict y, but what if now we have multiple variables, not just x, but x1,x2, x3 … to predict y — how would we approach this problem? I hope to explain in this article.
我们看了第4.1集中的简单线性回归,其中我们有一个变量x来预测y ,但是如果现在我们有多个变量,不仅是x,而且还有x1,x2,x3 …来预测y ,我们将如何处理?这个问题? 我希望在本文中进行解释。
简单线性回归回顾 (Simple Linear Regression Recap)
From Episode 4.1 we had our data of temperature and humidity:
从第4.1集开始,我们获得了温度和湿度数据:
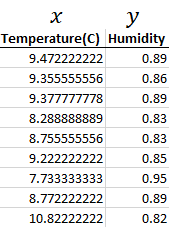
We plotted our Data, found and found a linear relationship — making linear regression suitable:
我们绘制了数据,发现并找到了线性关系,从而使线性回归适用:
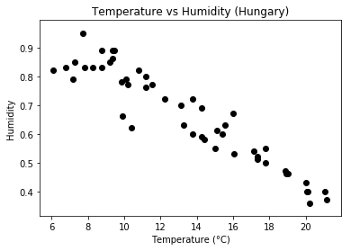
We then calculated our regression line:
然后,我们计算了回归线:
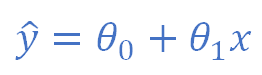
using gradient descent to find our parameters θ₀ and θ₁.
使用梯度下降找到我们的参数 θ₀和θ₁。

We then used the regression line calculated to make predictions for Humidity given any Temperature value.
然后,我们使用计算得出的回归线对给定任何温度值的湿度进行预测。
什么是多元线性回归? (What is Multiple Linear Regression?)
Multiple linear regression takes the exact same concept as simple linear regression but applies it to multiple variables. So instead of just looking at temperature to predict humidity, we can look at other factors such as wind speed or pressure.
多元线性回归采用与简单线性回归完全相同的概念,但将其应用于多个变量。 因此,我们不仅可以查看温度来预测湿度,还可以查看其他因素,例如风速或压力 。
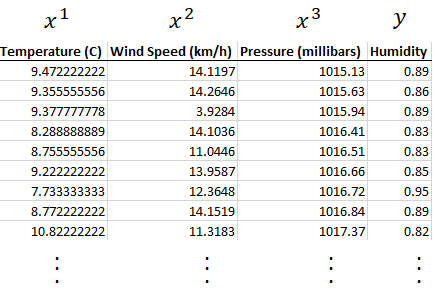
We are still trying to predict Humidity so this remains as y.
我们仍在尝试预测湿度,因此仍为y。
We rename Temperature, Wind Speed and Pressure to 𝑥¹,𝑥² and 𝑥³.
我们将温度,风速和压力重命名为𝑥¹ , 𝑥²和𝑥³。
Just as with Simple Linear Regression we must ensure that our variables 𝑥₁,𝑥₂ and 𝑥₃ form a linear relationship with y, if not we will be producing a very inaccurate model.
就像简单线性回归一样,我们必须确保变量𝑥₁,𝑥_2和𝑥₃ 与y形成线性关系 ,否则,我们将生成一个非常不准确的模型。
Lets plot each of our variables against Humidity:
让我们针对湿度绘制每个变量:
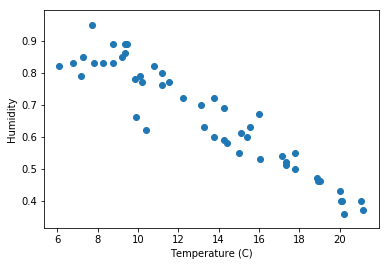
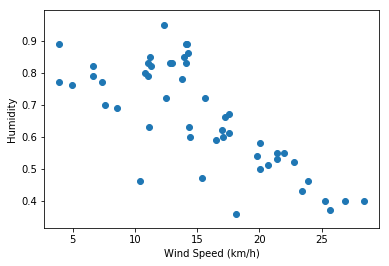

Temperature and Humidity form a strong linear relationship
温度和湿度形成很强的线性关系
Wind Speed and Humidity form a linear relationship
风速和湿度形成线性关系
Pressure and Humidity do not form a linear relationship
压力和湿度不是线性关系
We therefore can not use Pressure (𝑥³) in our multiple linear regression model.
因此,我们不能在多元线性回归模型中使用压力 (𝑥³)。
绘制数据 (Plotting our Data)
Let’s now plot both Temperature (𝑥¹) and Wind Speed (𝑥²) against Humidity.
现在让我们绘制两个温度(𝑥¹) 以及相对于湿度的风速(𝑥²)。

We can see that our data follows a roughly linear relationship, that is we can fit a plane on our data that captures the relationship between Temperature, Wind-speed(𝑥₁, 𝑥₂) and Humidity (y).
我们可以看到我们的数据遵循大致线性关系,也就是说,我们可以在数据上拟合一个平面 ,以捕获温度,风速(𝑥₁,𝑥²)和湿度(y)之间的关系。

计算回归模型 (Calculating the Regression Model)
Because we are dealing with more than one 𝑥 variable our linear regression model takes the form:
因为我们要处理多个𝑥变量,所以线性回归模型采用以下形式:

Just as with simple linear regression in order to find our parameters θ₀, θ₁ and θ₂ we need to minimise our cost function:
与简单的线性回归一样,为了找到我们的参数θ₀,θ₁和θ2,我们需要最小化成本函数:

We do this using the gradient descent algorithm:
我们使用梯度下降算法执行此操作:

This algorithm is explained in more detail here
此算法在这里更详细地说明
After running our gradient descent algorithm we find our optimal parameters to be θ₀ = 1.14 , θ₁ = -0.031 and θ₂ =-0.004
运行梯度下降算法后,我们发现最优参数为θ₀= 1.14,θ₁= -0.031和θ2= -0.004
Giving our final regression model:
给出我们的最终回归模型:

We can then use this regression model to make predictions for Humidity (ŷ) given any Temperature (𝑥¹) or Wind speed value(𝑥²).
然后,我们可以使用该回归模型对给定温度(𝑥¹)或风速值(𝑥²)的湿度(ŷ)进行预测。
In general models that contain more variables tend to be more accurate since we are incorporating more factors that have an effect on Humidity.
通常,包含更多变量的模型往往更准确,因为我们纳入了更多会影响湿度的因素。
_________________________________________
_________________________________________
潜在问题 (Potential Problems)
When including more and more variables in our model we run into a few problems:
当在模型中包含越来越多的变量时 ,我们会遇到一些问题:
- For example certain variables may become redundant. E.g look at our regression line above, θ₂ =0.004, multiplying our wind speed (𝑥²) by 0.004 barely changes our predicted value for humidity ŷ, which makes wind speed less useful to use in our model. 例如,某些变量可能变得多余。 例如,看一下上面的回归线θ2 = 0.004,将我们的风速()²)乘以0.004几乎不会改变我们对湿度predicted的预测值,这使得风速在模型中的用处不大。
- Another example is the scale of our data, i.e we can expect temperature to have a range of say -10 to 100, but pressure may have a range of 1000 to 1100. Using different scales of data can heavily affect the accuracy of our model. 另一个例子是我们的数据规模,即我们可以预期温度范围在-10到100之间,但是压力可能在1000到1100之间。使用不同的数据规模会严重影响我们模型的准确性。
How we solve these issues will be covered in future episodes.
我们如何解决这些问题将在以后的章节中介绍。
上一集 - 下一集 (Prev Episode — Next Episode)
如有任何疑问,请留在下面! (If you have any questions please leave them below!)

翻译自: https://medium.com/ai-in-plain-english/understanding-multiple-linear-regression-2672c955ec1c
多重线性回归 多元线性回归
本文来自互联网用户投稿,该文观点仅代表作者本人,不代表本站立场。本站仅提供信息存储空间服务,不拥有所有权,不承担相关法律责任。如若转载,请注明出处:http://www.mzph.cn/news/391920.shtml
如若内容造成侵权/违法违规/事实不符,请联系多彩编程网进行投诉反馈email:809451989@qq.com,一经查实,立即删除!