为什么印度盛产码农
Agriculture is at the center of Indian economy and any major change in the sector leads to a multiplier effect on the entire economy. With around 17% contribution to the Gross Domestic Product (GDP), it provides employment to more than 50% of the workforce. The largest industries of the country like sugar,textiles, food processing etc. are dependent on agriculture and the allied services for their raw materials. The major crops grown in India include paddy, wheat, pulses , edible oil and horticulture. Along with this poultry, fishery and animal husbandry are other major allied services. But despite the robust demand, attractive opportunities and policy support, the sector suffers from high price volatility , low income among farmers, skewed MSP rates and lack of credit facilities. Hence there is a need for a long term strategy to assess demand and supply and to forecast prices for crops.
一个 griculture是在印度经济中心和行业导致对整个经济产生乘数效应的任何重大变化。 它对国内生产总值(GDP)的贡献约为17%,为超过50%的劳动力提供了就业机会。 该国最大的工业,如糖,纺织,食品加工等,都依赖于农业及其相关的原材料服务。 印度的主要农作物包括水稻,小麦,豆类,食用油和园艺。 除此家禽外,渔业和畜牧业也是其他主要的盟约服务。 但是,尽管需求旺盛,有吸引力的机会和政策支持,该部门仍遭受价格高波动,农民收入低,MSP利率偏高以及缺乏信贷便利的困扰。 因此,需要一种长期战略来评估供需并预测农作物的价格。
Being a cereal-centric agriculture, the prices of paddy, wheat and pulses are of the most importance. Prices of vegetables like potato, tomato, onion also need to be closely monitored as they impact the food inflation rates in the country. Moreover the prices of 24 commodities under the MSP regime including 8 oil seeds , raw cotton, raw jute and copra are vital in deciding minimum support prices as well as deciding procurement prices for the Public Distribution System(PDS). Thus agriculture prices directly impact the income of producers and the food security of the nation.
作为以谷物为中心的农业,稻谷,小麦和豆类的价格最为重要。 土豆,西红柿,洋葱等蔬菜的价格也需要受到密切监控,因为它们会影响该国的食品通货膨胀率。 此外,MSP体制下的24种商品的价格(包括8种油料,原棉,生黄麻和椰干)对于决定最低支持价格以及决定公共分配系统的采购价格至关重要。 因此,农业价格直接影响生产者的收入和国家的粮食安全。
Directorate of Economics & Statistics, Agriculture (DESAg), in the Ministry of Agriculture is the prime source for agricultural statistics. Other sources include National Sample Survey Organisation(NSSO),State’s Directorate of Economics and Statistics (DES) and Central Statistical Organisation. A major part of this data is time series data, that measures agriculture prices over changing time frequencies — yearly, monthly, quarterly or daily. These series of prices show trends , along with seasonality and cyclic nature of agricultural prices.
农业部农业统计经济局(DESAg)是农业统计的主要来源。 其他来源包括国家抽样调查组织(NSSO),州经济与统计局(DES)和中央统计组织。 此数据的主要部分是时间序列数据,该数据可以测量变化的频率频率上的农业价格-每年,每月,每季度或每天。 这些系列价格显示趋势,以及农产品价格的季节性和周期性。
These time series data associated with agricultural prices can be used for forecasting short term and long term price estimates. It can help to identify patterns and seasonal variations in prices especially with respect to production during kharif, rabi and zaid seasons. Forecasting of agricultural prices are also important for determining subsidy rates, minimum support prices, export prices etc.Thus monitoring and controlling agricultural price volatility and preplanning policies to mitigate any adverse situations. Time series of crop prices can also help to understand, interpret and explain instances of economic crisis in the agricultural sector. Intervention analysis of such time series can also provide us insights into understanding the impact of sudden price changes over other sectors and overall economy. Moreover agriculture price forecasts are essential in determining risks in agri-commodity futures and options trading markets.
这些与农产品价格相关的时间序列数据可用于预测短期和长期价格估计。 它可以帮助确定价格的模式和季节性变化,特别是在卡里夫,狂犬病和宰牲节季节的生产方面。 预测农产品价格对于确定补贴率,最低支持价格,出口价格等也很重要。因此,监测和控制农产品价格的波动性以及为减轻任何不利情况而制定的计划政策。 作物价格的时间序列还可以帮助理解,解释和解释农业部门发生经济危机的情况。 此类时间序列的干预分析还可以为我们提供洞察力,以了解突然的价格变化对其他行业和整体经济的影响。 此外,农业价格预测对于确定农产品期货和期权交易市场的风险至关重要。
An important component of time series data is trend and agricultural price data often show deterministic trends. Trend analysis can be performed by using Moving Average Method and Exponential Smoothing Method. In the Moving Average Method, the first average is the mean of the prices in the first m period. The second average is the mean of the prices of m data points starting from the second price data up to (m + 1) th period. Similarly, the third average is the mean of the prices of m data points from the third to (m + 2) th period and so on. This new set of data is then used to determine the trend.
时间序列数据的重要组成部分是趋势,而农产品价格数据通常显示确定性趋势。 趋势分析可以使用``移动平均法''和``指数平滑法''进行。 在移动平均法中,第一个平均值是前m个时期的价格平均值。 第二个平均值是从第二个价格数据到第(m + 1)个时期开始的m个数据点的价格平均值。 同样,第三次平均值是从第三到第(m + 2)期间的m个数据点的价格的平均值,依此类推。 然后使用这组新数据确定趋势。

The Exponential Smoothing Method gives larger weights to recent price observations compared to past observations. Such that the weights decrease exponentially.
与过去的观察相比,指数平滑法对最近的价格观察给予更大的权重。 这样权重呈指数下降。

Another component of time series, that is often present in agricultural price data is seasonality. This is evident from the fact that the harvesting of crops takes place over a certain period of time in a year and the prices fluctuate accordingly. It is thus necessary to identify the seasonality and obtain thedeseasonalized series for further model development. The methods used are the Dummy Variable method and Ratio to Moving Average methods.
农业价格数据中经常出现的时间序列的另一个组成部分是季节性。 从事实来看,农作物的收获是在一年的一定时期内发生的,价格也随之波动。 因此,有必要确定季节并获得反季节序列以用于进一步的模型开发。 所使用的方法是``虚拟变量''方法和``移动平均比率''方法。
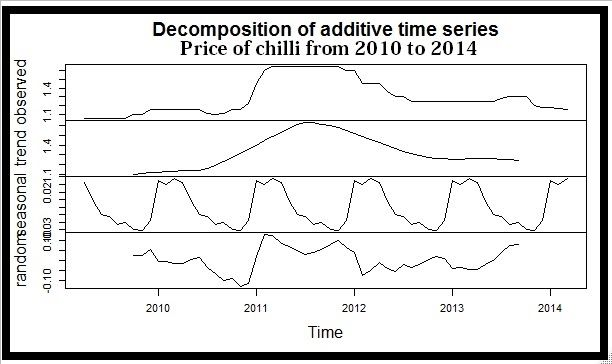
Before forecasting the price of agriculture produce, it is essential to identify the presence of non-stationarity. This is necessary to avoid spurious and misleading model estimates. The most common test used for the purpose is Augmented Dickey Fuller(ADF) unit root test. The framework of ADF methods is based on analysis of the following model.
在预测农产品价格之前,确定非平稳状态的存在至关重要。 这对于避免虚假和误导的模型估计是必要的。 为此目的最常用的测试是增强Dickey Fuller(ADF)单元根测试。 ADF方法的框架基于以下模型的分析。

If the time series data is non-stationary then we make it stationary by differencing and again performing the ADF test. This step is repeated until we get a stationary time series. After getting a deseasonalized stationary series without having a deterministic trend, we can proceed to identification of the model. The model can be either AR(p) / MA(q) / ARMA(p,q). To determine the model and its order we calculate the sample ACF ,sample PACF and use the L Jung Box Test to conclude the final model. The parameter estimation can be done based on the MLE/ Yule Walker equation/OLS approach. ARIMA models are fitted and accuracy of the model is tested on the basis of residual diagnostics. The L Jung Box test is applied on the residuals to determine whether they are white noise. The final time series model is thus ready for forecasting and prediction.
如果时间序列数据不稳定,则可以通过微分并再次执行ADF测试使其稳定。 重复此步骤,直到获得稳定的时间序列。 在获得没有确定性趋势的反季节平稳序列后,我们可以继续进行模型识别。 该模型可以是AR(p)/ MA(q)/ ARMA(p,q)。 为了确定模型及其顺序,我们计算了样本ACF,样本PACF并使用L Jung Box Test得出了最终模型。 可以基于MLE / Yule Walker方程/ OLS方法进行参数估计。 拟合ARIMA模型,并根据残留诊断对模型的准确性进行测试。 将L Jung Box测试应用于残差以确定它们是否为白噪声。 因此,最终的时间序列模型已准备好进行预测和预测。

ILLUSTRATIONS1. Price forecast of paddy based on ARMA(1,1) model
插图 1.基于ARMA(1,1)模型的稻谷价格预测


Based on the ACF and PACF correlogram, a time series ARIMA(1,1,1) model has been built to forecast paddy prices for the future.
基于ACF和PACF相关图,建立了时间序列ARIMA(1,1,1)模型来预测未来的稻米价格。

2. Price forecast of maize based on MA(1) model
2.基于MA(1)模型的玉米价格预测
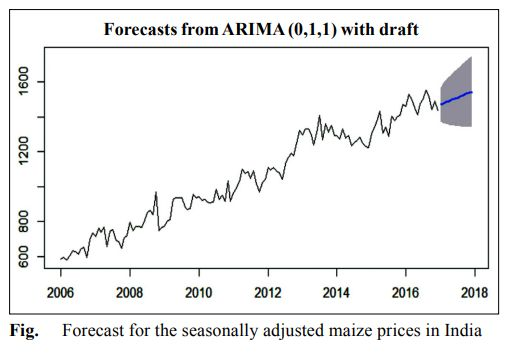
3. Price forecast of pigeon pea based on AR(1) model
3.基于AR(1)模型的木豆价格预测

CONCLUSION
结论
The time series AR/MA/ARMA models serve as a good methodology for predicting the magnitude of any price variable. Its strength lies in the fact that the method is effective in any time series on agricultural prices with a pattern of change. Fluctuation in prices have increased significantly with a lot of uncertainty in profitability of growing agricultural crops. Under this scenario, forecasting future prices and disseminating the same to farmers to facilitate informed decisions by farmers before sowing is important. Forecasting of prices can help the farmers to decide the area allocation for a particular crop and marketing of agricultural produce. The forecasted values are almost similar to actual prices but like any other method, this technique also does not guarantee perfect forecasts. Nevertheless time series analysis is handy and can be successfully implemented using R and Python programming to get desired results.
时间序列AR / MA / ARMA模型可作为预测任何价格变量幅度的良好方法。 其优势在于,该方法在任何时间序列上都可以有效地改变农产品价格。 价格的波动已经大大增加,而农业作物的获利能力存在很多不确定性。 在这种情况下,预测未来的价格并将其分发给农民以促进农民在播种前做出明智的决定非常重要。 价格预测可以帮助农民确定特定作物的区域分配和农产品销售。 预测值几乎与实际价格相似,但是像其他任何方法一样,此技术也不能保证完美的预测。 尽管如此,时间序列分析还是很方便的,并且可以使用R和Python编程成功实现,以获得期望的结果。
REFERENCES1. V. Jadhav , B. V. Chinnappa Reddy , and G. M. Gaddi(2017), Application of ARIMA Model for Forecasting Agricultural Prices ,J. Agr. Sci. Tech. Vol. 19: 981–9922. Ashwini Darekar · A. Amarender Reddy(2017),Price forecasting of maize in major states, Maize Journal 6(1&2): 1–53. Ashwini Darekar · A. Amarender Reddy(2017), Price forecasting of pulses: case of pigeonpea,Journal of Food Legumes 30(3): 42–46
参考文献 1. V. Jadhav,BV Chinnappa Reddy和GM Gaddi(2017),ARIMA模型在预测农产品价格中的应用,J。 农业 科学 科技 卷 19:981–9922。 Ashwini Darekar·A.Amarender Reddy(2017),主要州玉米价格预测,《玉米杂志》 6(1&2):1-53。 Ashwini Darekar·A.Amarender Reddy(2017),豆类价格预测:木豆案,《食品豆类杂志》 30(3):42–46
翻译自: https://medium.com/analytics-vidhya/time-series-analysis-of-agricultural-prices-in-india-47e7ebebd8b4
为什么印度盛产码农
本文来自互联网用户投稿,该文观点仅代表作者本人,不代表本站立场。本站仅提供信息存储空间服务,不拥有所有权,不承担相关法律责任。如若转载,请注明出处:http://www.mzph.cn/news/391903.shtml
如若内容造成侵权/违法违规/事实不符,请联系多彩编程网进行投诉反馈email:809451989@qq.com,一经查实,立即删除!