tukey检测
One of John Tukey’s landmark papers, “The Future of Data Analysis”, contains a set of analytical techniques that have gone largely unnoticed, as if they’re hiding in plain sight.
John Tukey的标志性论文之一,“ 数据分析的未来 ”,包含了一套几乎未被注意的分析技术,好像它们隐藏在视线中一样。
Multiple sources identify Tukey’s paper as a seminal moment in the history of data science. Both Forbes (“A Very Short History of Data Science”) and Stanford (“50 years of Data Science”) have published histories that use the paper as their starting point. I’ve quoted Tukey myself in articles about data science at Microsoft (“Using Azure to understand Azure”).
多个来源将Tukey的论文视为数据科学历史上的开创性时刻。 福布斯 (“ 数据科学史很短 ”)和斯坦福大学(“ 数据科学50年 ”)都发表了以该论文为起点的历史。 我在Microsoft的有关数据科学的文章(“ 使用Azure理解Azure ”中)中亲自引用了Tukey。
Independent of the paper, Tukey’s impact on data science has been immense: He was author of Exploratory Data Analysis. He developed the Fast Fourier Transform (FFT) algorithm, the box plot, and multiple statistical techniques that bear his name. He even coined the term “bit.”
独立于论文之外,Tukey对数据科学的影响是巨大的:他是《 探索性数据分析》的作者。 他开发了快速傅立叶变换(FFT)算法,箱形图以及多种以他的名字命名的统计技术。 他甚至创造了“位”一词。
But it wasn’t until I actually read “The Future of Data Analysis” that I discovered Tukey’s forgotten techniques. Of course, I already knew the paper was important. But I also knew that if I wanted to understand why — to understand the breakthrough in Tukey’s thinking — I had to read it myself.
但是直到我真正阅读了“数据分析的未来”之后,我才发现了Tukey被遗忘的技术。 当然,我已经知道该论文很重要。 但是我也知道,如果我想了解为什么 -要了解Tukey思维的突破-我必须自己阅读。
Tukey does not disappoint. He opens with a powerful declaration: “For a long time I have thought I was a statistician, interested in inferences from the particular to the general. But as I have watched mathematical statistics evolve, I have had cause to wonder and to doubt” (p 2). Like the opening of Beethoven’s Fifth, the statement is immediate and bold. “All in all,” he says, “I have come to feel that my central interest is in data analysis…” (p 2).
Tukey并不令人失望。 他以强有力的宣言开头:“很长一段时间以来,我一直以为我是统计学家,他对从个人到将军的推论很感兴趣。 但是当我看着数理统计的发展时,我就有理由怀疑和怀疑”(第2页)。 就像贝多芬第五届电影节的开幕一样,这份声明既直接又大胆。 他说:“总的来说,我已经感到我的主要兴趣是对数据分析…… ”(第2页)。
Despite Tukey’s use of first person, his opening statement is not about himself. He’s putting his personal and professional interests aside to make the much bolder assertion that statistics and data analysis are separate disciplines. He acknowledges that the two are related: “Statistics has contributed much to data analysis. In the future it can, and in my view should, contribute much more” (p 2).
尽管Tukey使用第一人称,但他的开场白与他本人无关 。 他将个人和职业兴趣放在一边,以大胆的断言认为统计和数据分析是独立的学科。 他承认这两者是相关的:“统计对数据分析做出了很大贡献。 在未来,它可以而且我认为应该做出更多贡献”(第2页)。
Moreover, Tukey states that statistics is “pure mathematics.” And, in his words, “…mathematics is not a science, since its ultimate standard of validity is an agreed-upon sort of logical consistency and provability” (p 6). Data analysis, however, is a science, distinguished by its “reliance upon the test of experience as the ultimate standard of validity” (p 5).
此外,Tukey指出统计是“ 纯粹的数学”。 用他的话来说,“……数学不是一门科学,因为其最终的有效性标准是人们商定的一种逻辑一致性和可证明性”(第6页)。 然而,数据分析是一门科学,其特点是“依靠经验检验作为有效性的最终标准”(第5页)。
CRAN上什么都没有 (Nothing on CRAN)
Not far into the paper, however, I stumbled. About a third of the way in (p 22), Tukey introduces FUNOP, a technique for automating the interpretation of plots. I paged ahead and spotted a number of equations. I worried that — before I could understand the equations — I might need an intuitive understanding of FUNOP. I paged further ahead and spotted a second technique, FUNOR-FUNOM. I soon realized that this pair of techniques, combined with a third that I didn’t yet realized was waiting for me, make up nearly half the paper.
然而,在论文不远处,我偶然发现。 在(p 22)中大约三分之一的方式中,Tukey引入了FUNOP,FUNOP是一种用于自动解释图的技术。 我向前翻页,发现了许多方程式。 我担心,在我无法理解方程式之前,我可能需要对FUNOP有直观的了解。 我向前翻页,发现了第二种技术,FUNOR-FUNOM。 我很快意识到,这两种技术加上我尚未意识到的三分之一正在等我,几乎占了论文的一半。
To understand “The Future of Data Analysis,” I would definitely need to learn more about FUNOP and FUNOR-FUNOM. I took that realization in stride, though, because I learned long ago that data science is — and will always be — full of terms and techniques that I don’t yet know. I’d do my research and come back to Tukey’s paper.
要理解“数据分析的未来”,我肯定需要了解有关FUNOP和FUNOR-FUNOM的更多信息。 但是,我大步迈进了这一认识,因为我很久以前就了解到,数据科学充满了并且将永远充满着我尚不知道的术语和技术。 我会做研究,然后回到Tukey的论文。
But when I searched online for FUNOP, I found almost nothing. More surprising, there was nothing in CRAN. Given the thousands of packages in CRAN and the widespread adoption of Tukey’s techniques, I expected there to be multiple implementations of the techniques from such an important paper. Instead, nothing.
但是,当我在网上搜索FUNOP时,却什么也没发现。 更令人惊讶的是,CRAN中什么也没有 。 鉴于CRAN中有成千上万的软件包以及Tukey技术的广泛采用,我希望从如此重要的论文中可以有多种技术实现。 相反,什么都没有。
FUNOP (FUNOP)
Fortunately, Tukey describes in detail how FUNOP and FUNOR-FUNOM work. And, fortunately, he provides examples of how they work. Unfortunately, he provides only written descriptions of these procedures and their effect on example data. So, to understand the procedures, I implemented each of them in R. (See my repository on GitHub.) And to further clarify what they do, I generated a series of charts that make it easier to visualize what’s going on.
幸运的是,Tukey详细描述了FUNOP和FUNOR-FUNOM的工作方式。 而且,幸运的是,他提供了它们如何工作的示例。 不幸的是,他仅提供了这些过程及其对示例数据的影响的书面说明。 因此,为了理解这些过程,我在R中实现了每个过程。(请参阅GitHub上的我的存储库 。)为了进一步阐明它们的作用,我生成了一系列图表,使可视化的过程变得更加容易。
Here’s Tukey’s definition of FUNOP (FUll NOrmal Plot):
这是Tukey对FUNOP(完整标称图)的定义:
(b1) Let aᵢ₍ₙ₎ be a typical value for the ith ordered observation in a sample of n from a unit normal distribution.
(b1)设aᵢ₍ₙ₎为单位正态分布的n个样本中第i次有序观察的典型值。
(b2) Let y₁ ≤ y₂ ≤ … ≤ yₙ be the ordered values to be examined. Let y̍ be their median (or let ӯ, read “y trimmed”, be the mean of the yᵢ with ⅓n < i ≤ ⅓(2n).
(B2)设y₁≤ÿ₂≤... ≤ÿₙ被有序进行检查值。 令y是其值(或让ӯ,读“Y修整”,是平均与⅓Ñ<I≤⅓(2 n中的yᵢ的)。
(b3) For i ≤ ⅓n or > ⅓(2n) only, let zᵢ = (yᵢ - y̍)/aᵢ₍ₙ₎ (or let
(B3),其中i≤⅓n或>⅓(2 n)的唯一的,让zᵢ=(yᵢ - Y)/aᵢ₍ₙ₎(或让
(b3) For i ≤ ⅓n or > ⅓(2n) only, let zᵢ = (yᵢ - y̍)/aᵢ₍ₙ₎ (or let zᵢ = (yᵢ - ӯ) /aᵢ₍ₙ₎).
(B3),其中i≤⅓n或>⅓(2 n)的唯一的,让zᵢ=(yᵢ - Y)/aᵢ₍ₙ₎(或让zᵢ=(yᵢ - ӯ)/aᵢ₍ₙ₎)。
(b4) Let z̍ be the median of the z’s thus obtained (about ⅓(2n) in number).
(b4)令z̍为由此获得的z的中值(数量约为⅓(2 n ))。
(b5) Give special attention to z’s for which both |yᵢ - y̍| ≥ A · z̍ and zᵢ ≥ B · z̍ where A and B are prechosen.
(b5)特别注意z的两个 | yᵢ - y̍ | ≥A·Z和zᵢ≥ 乙 ·Z,其中A和B是预先选定的。
(b5*) Particularly for small n, zⱼ’s with j more extreme than an i for which (b5) selects zᵢ also deserve special attention… (p23).
(b5 *)特别是对于较小的n , zⱼ的j值要比i (b5选择zᵢ的i ) 极端的情况还要特别注意…(p23)。
The basic idea is very similar to a Q-Q plot.
基本思想与QQ图非常相似。
Tukey gives us an example of 14 data points. On a normal Q-Q plot, if data are normally distributed, they form a straight line. But in the chart below, based upon the example data, we can clearly see that a couple of the points are relatively distant from the straight line. They’re outliers.
Tukey为我们提供了14个数据点的示例。 在正常的QQ图上,如果数据呈正态分布,则它们会形成一条直线。 但是在下面的图表中,基于示例数据,我们可以清楚地看到其中一些点与直线相对远离。 他们是离群值。

The goal of FUNOP is to eliminate the need for visual inspection by automating interpretation.
FUNOP的目标是通过自动解释来消除视觉检查的需要。
The first variable in the FUNOP procedure (aᵢ₍ₙ₎) simply gives us the theoretical distribution, where i is the ordinal value in the range 1..n and Gau⁻¹ is the quantile function of the normal distribution (i.e., the “Q” in Q-Q plot):
FUNOP过程中的第一个变量( aᵢ₍ₙ₎ )简单地提供了理论分布,其中i是范围1内的序数。n和Gau⁻¹是正态分布的分位数函数(即“ QQ图中的“ Q”):
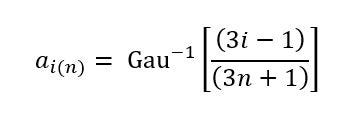
The key innovation of FUNOP is to calculate the slope of each point, relative to the median.
FUNOP的关键创新是计算每个点相对于中位数的斜率。

If y̍ is the median of the sample, and we presume that it’s located at the midpoint of the distribution (where a(y) = 0), then the slope of each point can be calculated as:
如果Y是样品的中值,并且我们假定它是位于所述分布(其中a(Y)= 0)的中点,那么每个点的斜率可以被计算为:

The chart above illustrates how slope of one point (1.2, 454) is calculated, relative to the median (0, 33.5).
上面的图表说明了如何计算相对于中位数(0,33.5)的一个点(1.2,454)的斜率。

Any point that has a slope significantly steeper than the rest of the population is necessarily farther from the straight line. To do this, FUNOP simply compares each slope (zᵢ) to the median of all calculated slopes (z̍).
斜率明显比其余人口陡峭的任何点都必须远离直线。 为此,FUNOP只需将每个斜率( zᵢ )与所有计算斜率的中值进行比较 ( z̍ )。
Note, however, that FUNOP calculates slopes for the top and bottom thirds of the sorted population only, in part because zᵢ won’t vary much over the inner third of that population, but also because the value of aᵢ₍ₙ₎ for the inner third will be close to 0 and dividing by ≈0 when calculating zᵢ might lead to instability.
但是请注意,这FUNOP计算山坡的顶部,只有有序人口的三分之二的底部,部分原因是zᵢ不会有太大了,人口的内第三次有所不同,但也因为aᵢ₍ₙ₎对内部价值第三个将接近0,并且在计算zᵢ时除以≈0可能会导致不稳定。
Significance — what Tukey calls “special attention” — is partially determined by B, one of two predetermined values (or hyperparameters). For his example, Tukey recommends a value between 1.5 and 2.0, which means that FUNOP simply checks whether the slope of any point, relative to the midpoint, is 1.5 or 2.0 times larger than the median.
重要性(Tukey称为“特别注意”)部分由B (两个预定值(或超参数)之一)确定。 对于他的示例,Tukey建议使用介于1.5和2.0之间的值,这意味着FUNOP只是检查相对于中点的任何点的斜率是否比中值大1.5或2.0倍。
The other predetermined value is A, which is roughly equivalent to the number of standard deviations of yᵢ from y̍ and serves as a second criterion for significance.
另一个预定值是A ,它大致等于y 1与y 1的标准偏差的数量,并用作重要性的第二标准。
The following chart shows how FUNOP works.
下图显示了FUNOP的工作方式。
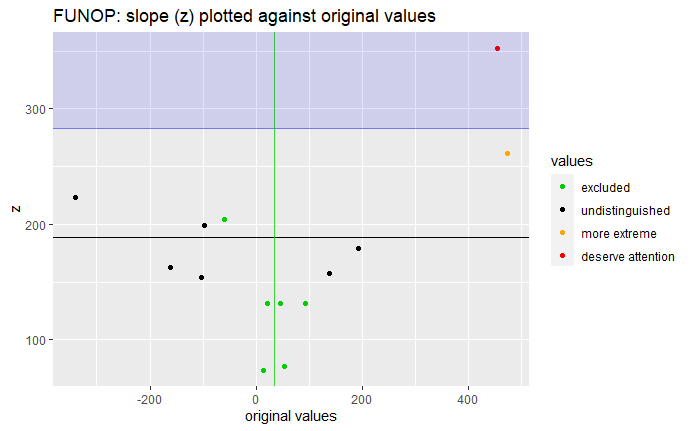
Our original values are plotted along the x-axis. The points in the green make up the inner third of our sample, and we use them to calculate y̍, the median of just those points, indicated by the green vertical line.
我们的原始值沿x轴绘制。 绿色的点构成了样本的内部三分之一,我们用它们来计算y̍ ,即这些点的中值,由绿色的垂直线表示。
The points not in green make up the outer thirds (i.e., the top and bottom thirds) of our sample, and we use them to calculate z̍, the median slope of just those points, indicated by the black horizontal line.
非绿色的点构成样本的外部三分之二(即顶部和底部的三分之二),我们使用它们来计算z̍ ,即这些点的中间斜率,用黑色水平线表示。
Our first selection criterion is zᵢ ≥ B · z̍. In his example, Tukey sets B = 1.5, so our threshold of interest is 1.5z̍, indicated by the blue horizontal line. We’ll consider any point above that line (the shaded blue region) as deserving “special attention”. We have only one such point, colored red.
我们的第一选择标准是zᵢ≥·B·Z ^。 在他的示例中,Tukey设置B = 1.5,因此我们的关注阈值为1.5z̍ ,由蓝色水平线表示。 我们将认为该线上方的任何点(蓝色阴影区域)都值得“特别注意”。 我们只有一个这样的点,红色。
Our second criterion is |yᵢ - y̍| ≥ A · z̍. In his example, Tukey sets A = 0, so our threshold of interest is |yᵢ - y̍| ≥ 0 or (more simply) yᵢ ≠ y̍. Basically, any point not on the green line. Our one point in the shaded blue region isn’t on the green line, so we still have our one point.
我们的第二个标准是| yᵢ- y̍ | ≥ 甲 ·Z。 在他的示例中,Tukey设置A = 0,因此我们的兴趣阈值为|。 yᵢ- y̍ | ≥0或(或更简单地) yᵢ ≠ y̍ 。 基本上,任何一点都不在绿线上。 我们在阴影蓝色区域中的一点不在绿线上,因此我们仍然有一点。
Our final criterion is any zⱼ’s with j more extreme than any i selected so far. Basically, that’s any value more extreme than the ones already identified. In this case, we have one value that’s larger (further to the right on the x-axis) than our red dot. That point is colored orange, and we add it to our list.
我们的最终标准是任何jⱼ的z都比我迄今为止选择的任何z都极端。 基本上,这是比已确定的值更极端的任何值。 在这种情况下,我们有一个比红点更大的值(在x轴上更靠右)。 该点显示为橙色,我们将其添加到列表中。
The two points identified by FUNOP are the same ones that we identified visually in Chart 1.
FUNOP标识的两点与我们在图1中直观标识的相同。
技术 (Technology)
FUNOP represents a turning point in the paper.
FUNOP代表了本文的一个转折点。
In the first section, Tukey explores a variety of topics from a much more philosophical perspective: The role of judgment in data analysis, problems in teaching analysis, the importance of practicing the discipline, facing uncertainty, and more.
在第一部分中,Tukey从更哲学的角度探讨了多个主题:判断在数据分析中的作用,教学分析中的问题,实践该学科的重要性,面临不确定性等。
In the second section, Turkey turns his attention to “spotty data” and its challenges. The subsections get increasingly more technical, and the first of many equations appears. Just before he introduces FUNOP, Tukey explores “automated examination”, where he discusses the role of technology.
在第二部分中,土耳其将注意力转移到“斑点数据”及其挑战上。 这些小节的技术水平越来越高,出现了许多方程式中的第一个。 在介绍FUNOP之前,Tukey探索了“自动检查”,并在其中讨论了技术的作用。
Even though Tukey wrote his paper nearly 60 years ago, he anticipates the dual role that technology continues to play to this day: It will democratize analysis, making it more accessible for casual users, but it will also enable the field’s advances:
尽管Tukey在将近60年前写了论文,但他预计技术将在今天继续发挥双重作用:它将使分析民主化,使休闲用户更易于使用,但也将推动该领域的发展:
“(1) Most data analysis is going to be done by people who are not sophisticated data analysts…. Properly automated tools are the easiest to use for [someone] with a computer.
“(1)大多数数据分析将由不是经验丰富的数据分析师的人来完成。 适当的自动化工具最适合[某人]与计算机一起使用。
“(2) …[Sophisticated data analysts] must have both the time and the stimulation to try out new procedures of analysis; hence the known procedures must be made easy for them to apply as possible. Again automation is called for.
“(2)……[复杂的数据分析人员]必须有时间和动力去尝试新的分析程序; 因此,必须使已知程序易于使用。 再次需要自动化。
“(3) If we are to study and intercompare procedures, it will be much easier if the procedures have been fully specified, as must happen [in] the process of being made routine and automatizable” (p 22).
“(3)如果我们要研究和相互比较程序,那么,如果程序被完全指定,就会变得容易得多,因为这必定会在[使其成为例行程序和自动化过程中发生](p 22)。
The juxtaposition of “automated examination” and “FUNOP” made me wonder about Tukey’s reason for including the technique in his paper. Did he develop FUNOP simply to prove his point about technology? It effectively identifies outliers, but it’s complicated enough to benefit from automation.
“自动检查”和“ FUNOP”的并置使我想知道Tukey将这项技术包括在他的论文中的原因。 他开发FUNOP只是为了证明他对技术的观点吗? 它可以有效地识别异常值,但是它很复杂,可以从自动化中受益。
Feel free to skip ahead if you’re not interested in the code:
如果您对代码不感兴趣,请随时跳过:
# a helper function for FUNOP and FUNOR_FUNOM, which use the output of
# a_qnorm as the denominator for their slope calculations.
a_qnorm <- function(i, n) {qnorm((3 * i - 1) / (3 * n + 1))
}funop <- function(x, A = 0, B = 1.5) {# (b1)# Let a_{i|n} be a typical value for the ith ordered observation in# a sample of n from a unit normal distribution.n <- length(x)# initialze dataframe to hold resultsresult <- data.frame(y = x,orig_order = 1:n,a = NA,z = NA,middle = FALSE,special = FALSE)# put array in orderresult <- result %>%dplyr::arrange(x) %>%dplyr::mutate(i = dplyr::row_number())# calculate a_{i|n}result$a <- a_qnorm(result$i, n)# (b2)# Let y_1 ≤ y_2 ≤ … ≤ y_n be the ordered values to be examined.# Let y_split be their median (or let y_trimmed be the mean of the y_i# with (1/3)n < i ≤ (2/3)n).middle_third <- (floor(n / 3) + 1):ceiling(2 * n / 3)outer_thirds <- (1:n)[-middle_third]result$middle[middle_third] <- TRUEy_split <- median(result$y)y_trimmed <- mean(result$y[middle_third])# (b3)# For i ≤ (1/3)n or > (2/3)n only,# let z_i = (y_i – y_split) / a_{i|n}# (or let z_i = (y_i – y_trimmed) / a_{i|n}).result$z[outer_thirds] <-(result$y[outer_thirds] - y_split) / result$a[outer_thirds]# (b4)# Let z_split be the median of the z’s thus obtained.z_split <- median(result$z[outer_thirds])# (b5)# Give special attention to z’s for which both# |y_i – y_split| ≥ A · z_split and z_i ≥ B · z_split# where A and B are prechosen.result$special <-ifelse(result$z >= (B * z_split) &abs(result$y - y_split) >= (A * z_split), TRUE, FALSE)# (b5*)# Particularly for small n, z_j’s with j more extreme than an i# for which (b5) selects z_i also deserve special attention.# in the top third, look for values larger than ones already foundtop_third <- outer_thirds[outer_thirds > max(middle_third)]# take advantage of the fact that we've already indexed our result set# and simply look for values of i larger than the smallest i in the# top third (further to the right of our x-axis)if (any(result$special[top_third])) {min_i <- result %>%dplyr::filter(special == TRUE) %>%{min(.$i)}result$special[which(result$i > min_i)] <- TRUE}# in the top third, look for values smaller than ones already foundbottom_third <- outer_thirds[outer_thirds < min(middle_third)]# look for values of i smaller than the largest i in the bottom third# (further to the left of our x-axis)if (any(result$special[bottom_third])) {max_i <- result %>%dplyr::filter(special == TRUE) %>%{.$max_i}result$special[which(result$i < max_i)] <- TRUE}result <- result %>%dplyr::arrange(orig_order) %>%dplyr::select(y, i, middle, a, z, special)attr(result, 'y_split') <- y_splitattr(result, 'y_trimmed') <- y_trimmedattr(result, 'z_split') <- z_splitresult
}
火葬场 (FUNOR-FUNOM)
One common reason for identifying outliers is to do something about them, often by trimming or Winsorizing the dataset. The former simply removes an equal number of values from upper and lower ends of a sorted dataset. Winsorizing is similar but doesn’t remove values. Instead, it replaces them with the closest original value not affected by the process.
识别离群值的一个常见原因是通常通过修剪或Winsorize数据集来对它们进行处理。 前者只是从排序后的数据集的上端和下端删除相等数量的值。 Winsorizing与之类似,但不会删除值。 相反,它替换它们不受进程最接近原始值。
Tukey’s FUNOR-FUNOM (FUll NOrmal Rejection-FUll NOrmal Modification) offers an alternate approach. The procedure’s name reflects its purpose: FUNOR-FUNOM uses FUNOP to identify outliers, and then uses separate rejection and modification procedures to treat them.
Tukey的FUNOR-FUNOM(完全范式拒绝-完全范式修改)提供了另一种方法。 该过程的名称反映了其目的:FUNOR-FUNOM使用FUNOP识别异常值,然后使用单独的拒绝和修改过程对其进行处理。
The technique offers a number of innovations. First, unlike trimming and Winsorizing, which affect all the values at the top and bottom ends of a sorted dataset, FUNOR-FUNOM uses FUNOP to identify individual outliers to treat. Second, FUNOR-FUNOM leverages statistical properties of the dataset to determine individual modifications for those outliers.
该技术提供了许多创新。 首先,与修剪和Winsorizing不同,修剪和Winsorizing会影响排序数据集的顶端和底端的所有值,而FUNOR-FUNOM使用FUNOP来识别要处理的单个异常值。 其次,FUNOR-FUNOM利用数据集的统计属性来确定这些异常值的单独修改。
FUNOR-FUNOM is specifically designed to operate on two-way (or contingency) tables. Similar to other techniques that operate on contingency tables, it uses the table’s grand mean (x..) and the row and column means (xⱼ. and x.ₖ, respectively) to calculate expected values for entries in the table.
FUNOR-FUNOM是专门为在双向(或偶发性)表上运行而设计的。 与对列联表进行操作的其他技术类似,它使用表的均值( x .. )以及行和列均值(分别为xⱼ。和x.ₖ )计算表中条目的期望值。
The equation below shows how these effects are combined. Because it’s unlikely for expected values to match the table’s actual values exactly, the equation includes a residual term (yⱼₖ) to account for any deviation.
以下等式显示了这些效应如何组合。 由于期望值不太可能与表的实际值完全匹配,因此该方程式包含一个残差项( yⱼₖ )以解决任何偏差。

FUNOR-FUNOM is primarily interested in the values that deviate most from their expected values, the ones with the largest residuals. So, to calculate residuals, simply swap the above equation around:
FUNOR-FUNOM主要对与期望值有最大差异的值感兴趣,这些期望值具有最大的残差。 因此,要计算残差,只需将上面的等式交换为:

FUNOR-FUNOM starts by repeatedly applying FUNOP, looking for outlier residuals. When it finds them, it modifies the outlier with the greatest deviation by applying the following modification:
FUNOR-FUNOM首先重复应用FUNOP,以寻找异常值残差。 找到它们后,通过应用以下修改,以最大的偏差修改离群值:

where
哪里

Recalling the definition of slope (from FUNOP)
回顾坡度的定义(来自FUNOP)
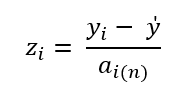
the first portion of the Δxⱼₖ equation reduces to just yⱼₖ - y̍, the difference of the residual from the median. The second portion of the equation is a factor, based solely upon table size, meant to compensate for the effect of an outlier on the table’s grand, row, and column means.
Δxⱼₖ方程的第一部分减少到yⱼₖ- y̍ ,即残差与中值之差。 等式的第二部分是一个仅基于表大小的因数,旨在补偿异常值对表的盛大,行和列均值的影响。
When Δxⱼₖ is applied to the original value, the yⱼₖ terms cancel out, effectively setting the outlier to its expected value (based upon the combined effects of the contingency table) plus a factor of the median residual (~ xⱼ. + x.ₖ + x.. + y̍).
当将Δx to应用于原始值时, yⱼₖ项会被抵消,从而有效地将离群值设置为其预期值(基于列联表的综合影响)加上中位数残差因子( 〜xⱼ。 + x.ₖ)。 + x .. + y̍ )。
FUNOR-FUNOM repeats this same process until it no longer finds values that “deserve special attention.”
FUNOR-FUNOM重复相同的过程,直到不再找到“值得特别注意”的值为止。
In the final phase, the FUNOM phase, the procedure uses a lower threshold of interest — FUNOP with a lower A — to identify a final set of outliers for treatment. The adjustment becomes
在最后阶段,即FUNOM阶段,该过程使用较低的关注阈值(FUNOP的A较低)来识别最终的离群值进行治疗。 调整变为

There are a couple of changes here. First, the inclusion of (–Bₘ · z̍) effectively sets the residual of outliers to FUNOP’s threshold of interest, much like the way that Winsorizing sets affected values to the same cut-off threshold. FUNOM, though, sets only the residual of affected values to that threshold: The greater part of the value is determined by the combined effects of the grand, row, and column means.
这里有几个更改。 首先,列入( - Bₘ·Z)有效地将剩余离群感兴趣FUNOP的门槛,很像设置极值调整受影响的值相同的截止阈值的方式。 但是,FUNOM 仅将受影响的值的残差设置为该阈值:值的较大部分由grand,row和column平均值的组合效果确定。
Second, because we’ve already taken care of the largest outliers (whose adjustment would have a more significant effect on the table’s means), we no longer need the compensating factor.
其次,因为我们已经处理了最大的离群值(其调整将对表格的均值产生更大的影响),所以我们不再需要补偿因子。
The chart below shows the result of applying FUNOR-FUNOM to the data in Table 2 of Tukey’s paper.
下图显示了将FUNOR-FUNOM应用于Tukey论文表2中的数据的结果。

The black dots represent the original values affected by the procedure. The color of their treated values is based upon whether they were determined by the FUNOR or FUNOM portion of the procedure. The grey dots represent values unaffected by the procedure.
黑点表示受该过程影响的原始值。 其处理值的颜色取决于它们是由程序的FUNOR还是FUNOM部分确定的。 灰色点表示不受影响的值 通过程序。
FUNOR handles the largest adjustments, which Tukey accomplishes by setting Aᵣ = 10 and Bᵣ = 1.5 for that portion of the process, and FUNOM handles the finer adjustments by setting Aₘ = 0 and Bₘ = 1.5.
FUNOR处理最大的调整,Tukey通过在该过程的那部分设置Aᵣ = 10和Bᵣ = 1.5来完成,而FUNOM通过设置Aₘ = 0和Bₘ = 1.5处理更细的调整。
Again, because the procedure leverages the statistical properties of the data, each of the resulting adjustments is unique.
同样,由于该过程利用了数据的统计属性,因此每个调整结果都是唯一的。
Here is the code:
这是代码:
funor_funom <- function(x, A_r = 10, B_r = 1.5, A_m = 0, B_m = 1.5) {x <- as.matrix(x)# Initializer <- nrow(x)c <- ncol(x)n <- r * c# this will be used in step a3, but only need to calc oncechange_factor <- r * c / ((r - 1) * (c - 1))# this data frame makes it easy to track all values# j and k are the rows and columns of the tabledat <- data.frame(x = as.vector(x),j = ifelse(1:n %% r == 0, r, 1:n %% r),k = ceiling(1:n / r),change_type = 0)############# FUNOR #############repeat {dat <- dat %>%dplyr::select(x, j, k, change_type) # clean up dat from last loop# (a1)# Fit row and column means to the original observations# and form the residuals# calculate the row meansdat <- dat %>%dplyr::group_by(j) %>%dplyr::summarise(j_mean = mean(x)) %>%dplyr::ungroup() %>%dplyr::select(j, j_mean) %>%dplyr::inner_join(dat, by = 'j')# calculate the column meansdat <- dat %>%dplyr::group_by(k) %>%dplyr::summarise(k_mean = mean(x)) %>%dplyr::ungroup() %>%dplyr::select(k, k_mean) %>%dplyr::inner_join(dat, by = 'k')grand_mean <- mean(dat$x)# calculate the residualsdat$y <- dat$x - dat$j_mean - dat$k_mean + grand_mean# put dat in order based upon y (which will match i in FUNOP)dat <- dat %>%dplyr::arrange(y) %>%dplyr::mutate(i = dplyr::row_number())# (a2)# apply FUNOP to the residualsfunop_residuals <- funop(dat$y, A_r, B_r)# (a4)# repeat until no y_{jk} deserves special attentionif (!any(funop_residuals$special)) {break}# (a3) modify x_{jk} for largest y_{jk} that deserves special attentionbig_y <- funop_residuals %>%dplyr::filter(special == TRUE) %>%dplyr::top_n(1, (abs(y)))# change x by an amount that's proportional to its# position in the distribution # here's why it's useful to have z be on same scale as the raw valuedelta_x <- big_y$z * big_y$a * change_factordat$x[which(dat$i == big_y$i)] <- big_y$y - delta_xdat$change_type[which(dat$i == big_y$i)] <- 1}# Done with FUNOR. To apply subsequent modifications we need # the following from the most recent FUNOPdat <- funop_residuals %>%dplyr::select(i, middle, a, z) %>%dplyr::inner_join(dat, by = 'i')z_split <- attr(funop_residuals, 'z_split')y_split <- attr(funop_residuals, 'y_split')############# FUNOM ############## (a5)# identify new interesting values based upon new A & B# start with threshold for extreme Bextreme_B <- B_m * z_splitdat <- dat %>%dplyr::mutate(interesting_values = ((middle == FALSE) &(z >= extreme_B)))# logical AND with threshold for extreme Aextreme_A <- A_m * z_splitdat$interesting_values <-ifelse(dat$interesting_values &(abs(dat$y - y_split) >= extreme_A), TRUE, FALSE)# (a6)# adjust just the interesting valuesdelta_x <- dat %>%dplyr::filter(interesting_values == TRUE) %>%dplyr::mutate(change_type = 2) %>%dplyr::mutate(delta_x = (z - extreme_B) * a) %>%dplyr::mutate(new_x = x - delta_x) %>%dplyr::select(-x, -delta_x) %>%dplyr::rename(x = new_x)# select undistinguied values from dat and recombine with# adjusted versions of the interesting valuesdat <- dat %>%dplyr::filter(interesting_values == FALSE) %>%dplyr::bind_rows(delta_x)# return data to original shapedat <- dat %>%dplyr::select(j, k, x, change_type) %>%dplyr::arrange(j, k)# reshape result into a table of the original shapematrix(dat$x, nrow = r, byrow = TRUE)
}
“傻瓜不要使用它!” (“Foolish not to use it!”)
After describing FUNOR-FUNOM, Tukey asserts that it serves a real need — one not previously addressed — and he invites people to begin using it, to explore its properties, even to develop competitors. In the meantime, he says, people would “…be foolish not to use it” (p 32).
在描述FUNOR-FUNOM之后,Tukey断言它满足了一项真正的需求-以前没有解决过-并邀请人们开始使用它,探索其特性,甚至发展竞争对手。 他说,与此同时,人们“…… 不使用它会很愚蠢 ”(第32页)。
Throughout his paper, Tukey uses italics to emphasize important points. Here he’s echoing an earlier point about arguments against the adoption of new techniques. He’d had colleagues suggest that no new technique should be published — much less used — before its power function was given. Tukey recognized the irony, because much of applied statistics depended upon Student’s t. In his paper, he points out,
在整个论文中,Tukey使用斜体字强调重点。 在这里,他回应了关于反对采用新技术的争论的较早观点。 他曾让同事们建议,在给出幂函数之前,不应发布任何新技术,而应使用更少的新技术。 Tukey意识到了讽刺意味,因为许多应用统计数据都取决于Student的t 。 他在论文中指出,
“Surely the suggested amount of knowledge is not enough for anyone to guarantee either
“当然,建议的知识量不足以使任何人都能保证
“(c1) that the chance of error, when the procedure is applied to real data, corresponds precisely to the nominal levels of significance or confidence, or
“(c1)当将该程序应用于真实数据时,错误机会恰好与名义上的显着性或置信度水平相对应,或者
“(c2) that the procedure, when applied to real data, will be optimal in any one specific sense.
“(c2)该程序应用于真实数据时,在任何一种特定意义上都是最佳的。
“BUT WE HAVE NEVER BEEN ABLE TO MAKE EITHER OF THESE STATEMENTS ABOUT Student’s t” (p 20).
“但是我们永远无法做出关于学生t的所有这些陈述” (第20页)。
This is Tukey’s only sentence in all caps. Clearly, he wanted to land the point.
这是图基在所有大写字母中唯一的一句话。 显然,他想提出这一点。
And, clearly, FUNOR-FUNOM was not meant as an example of theoretically possible techniques. Tukey intended for it to be used.
而且,很明显,FUNOR-FUNOM并不是作为理论上可行的技术的例子。 Tukey打算用于它。
吸尘器 (Vacuum cleaner)
FUNOR-FUNOM treats the outliers of a contingency table by identifying and minimizing outsized residuals, based upon the grand, row, and column means.
FUNOR-FUNOM通过基于大数,行数和列数均值来识别和最小化超大残差,从而处理列联表的异常值。
Tukey takes these concepts further with his vacuum cleaner, whose output is a set of residuals, which can be used to better understand sources of variance in the data and enable more informed analysis.
Tukey的真空吸尘器进一步完善了这些概念,该真空吸尘器的输出是一组残差,这些残差可以用来更好地理解数据中的差异来源并进行更明智的分析。
To isolate residuals, Tukey’s vacuum cleaner uses regression to break down the values from the contingency table into their constituent components (p 51):
为了隔离残差,Tukey的真空吸尘器使用回归将列联表中的值分解为它们的组成部分(第51页):

The idea is very similar to the one based upon the grand, row, and column means. In fact, the first stage of the vacuum cleaner produces the same result as subtracting the combined effect of the means from the original values.
这个想法与基于大写,行和列方式的想法非常相似。 实际上,真空吸尘器的第一阶段产生的结果与从原始值中减去均值的组合效果相同。
To do this, the vacuum cleaner needs to calculate regression coefficients for each row and column based upon the values in our table (yᵣₖ) and a carrier — or regressor — for both rows (aᵣ) and columns (bₖ). [Apologies for using “k” for columns, but Medium has its limitations.]
为此,真空吸尘器需要基于表( yᵣₖ )中的值以及行( aᵣ )和列( bₖ )的载体(或回归器)来计算每一行和每一列的回归系数。 [为列使用“ k”表示歉意,但“中等”有其局限性。]
Below is the equation used to calculate regression coefficients for columns.
以下是用于计算列的回归系数的方程式。

Conveniently, the equation will give us the mean of a column when we set aᵣ ≡ 1:
方便地,当我们设置aᵣ≡1时,该方程式将给我们列的平均值:

where nᵣ is the number of rows. Effectively, the equation iterates through every row (Σᵣ), summing up the individual values in the same column (c) and dividing by the number of rows, the same as calculating the mean (y.ₖ).
其中nᵣ是行数。 有效地,该方程式遍历每一行( Σᵣ ),将同一列( c )中的各个值相加,然后除以行数,这与计算平均值( y.ₖ )相同。
Note, however, that aᵣ is a vector. So to set aᵣ ≡ 1, we need our vector to satisfy this equation:
但是请注意, aᵣ是向量。 因此,要设置aᵣ≡1 ,我们需要向量来满足以下等式:

For a vector of length nᵣ we can simply assign every member the same value:
对于长度为nᵣ的向量,我们可以简单地为每个成员分配相同的值:

Our initial regressors end up being two sets of vectors, one for rows and one for columns, containing either √(1/nₖ) for rows or √(1/nᵣ) for columns.
我们的初始回归器最终是两组向量,一组用于行,一组用于列,其中包含√(1 / n1 /)用于行或√(1 / nᵣ )用于列。
Finally, in the same way that the mean of all row means or the mean of all column means can be used to calculate the grand mean, either the row coefficients or column coefficients can be used to calculate a dual-regression (or “grand”) coefficient:
最后,以所有行均值或所有列均值的均值可用于计算总体均值的方式,行系数或列系数均可以用于计算双回归(或“均值” )系数:

The reason for calculating all of these coefficients, rather than simply subtracting the grand, row, and column means from our table’s original values, is that Tukey’s vacuum cleaner reuses the coefficients from this stage in the procedure as regressors in the next. (To ensure aᵣ ≡ 1 and aₖ ≡ 1 for the next stage, we normalize both sets of new regressors.)
之所以要计算所有这些系数,而不是简单地从表的原始值中减去大,中,平均值,是因为Tukey的真空吸尘器在此过程中将这一阶段的系数作为下一个回归变量重复使用 。 (确保a≡1 并在下一阶段使用aₖ≡1 ,我们将两组新的回归器标准化。)
The second phase is the real innovation here. It’s take an earlier idea of Tukey’s, one degree of freedom for non-additivity, and applies it separately to each row and column. This, Tukey tells us, “…extracts row-by-row regression upon ‘column mean minus grand mean’ and column-by-column regression on ‘row mean minus grand mean’” (p 53).
第二阶段是这里的真正创新。 它采用了Tukey的早期思想,即非可加性的一种自由度,并将其分别应用于每一行和每一列。 Tukey告诉我们,“……提取'列均值减去总均值”的逐行回归,并提取'行均值减去总均值'的逐列回归”(第53页)。
The result is a set of residuals, vacuum cleaned of systemic effects.
结果是一组残留物,真空清除了系统影响。
Here’s the code for the entire procedure:
这是整个过程的代码:
vacuum <- function(x) {input_table <- xr <- nrow(input_table)c <- ncol(input_table)# number of rows and columns must be at least 3 (p 53)if (r < 3 | c < 3)print('Insuffienct size')######################## Initial carriers ######################### sqrt(1/n)carrier_r <- rep(sqrt(1 / r), r)carrier_c <- rep(sqrt(1 / c), c)##################### Start of loop ###################### Tukey passes through the loop twice.# Suggests further passes possible in the "attachments" section (p 53)for (pass in 1:2) {#################### Coefficients ##################### Calculate the column coefficientscoef_c <- rep(NA, c)for (i in 1:c) {# loop through every column, summing every row# denominator is based upon the number of rows in the columncoef_c[i] <-sum(carrier_r * input_table[, i]) / sum(carrier_r ^ 2)}# Calculate the row coefficientscoef_r <- rep(NA, r)for (i in 1:r) {# loop through every row, summing every column# denominator is based upon the number of columns in the rowcoef_r[i] <-sum(carrier_c * input_table[i,]) / sum(carrier_c ^ 2)}############ y_ab ############# either one of thesey_ab <- sum(coef_c * carrier_c) / sum(carrier_c ^ 2)y_ab <- sum(carrier_r * coef_r) / sum(carrier_r ^ 2)########################## Apply subprocedure ########################### create a destination for the outputoutput_table <- input_tablefor (i in 1:r) {for (j in 1:c) {output_table[i, j] <- input_table[i, j] -carrier_r[i] * (coef_c[j] - carrier_c[j] * y_ab) -carrier_c[j] * (coef_r[i] - carrier_r[i] * y_ab) -y_ab * carrier_c[j] * carrier_r[i]}}# These are the coefficients that will get carried forwardcoef_c <- coef_c - carrier_c * y_abcoef_r <- coef_r - carrier_r * y_ab########################## Prep for next pass ########################### normalize coefficients because we want sqrt(sum(a^2)) == 1carrier_r <- coef_r / l2_norm(coef_r)carrier_c <- coef_c / l2_norm(coef_c)input_table <- output_table################### End of loop ###################}output_table}
外卖 (Takeaways)
When I started this exercise, I honestly expected it to be something of an archaeological endeavor: I thought that I’d be digging through an artifact from 1962. Instead, I discovered some surprisingly innovative techniques.
当我开始这个练习时,老实说,我希望这是一项考古工作:我认为我会从1962年的一件文物中挖掘出来。相反,我发现了一些令人惊讶的创新技术。
However, none of the three procedures has survived in its original form. Not even Tukey mentions them in Exploratory Data Analysis, which he published 15 years later. That said, the book’s chapters on two-way tables contain the obvious successors to both FUNOR_FUNOM and the vacuum cleaner.
但是,这三个过程都没有以其原始形式保留下来。 15年后,他在《 探索性数据分析》中甚至没有提到图基。 就是说,该书在双向表上的章节包含FUNOR_FUNOM和真空吸尘器的明显后继。
Perhaps one reason they’ve faded from use is that the base procedure, FUNOP, requires the definition of two parameters, A and B. Tukey himself recognized “the choice of Bₐ is going to be a matter of judgement” (p 47). When I tried applying FUNOR_FUNOM on other data sets, it was clear that use of the technique requires tuning.
他们退出使用的原因之一可能是基本过程FUNOP需要定义两个参数A和B。 图基亲自认识到“选择Bₐ将是一个判断问题”(第47页)。 当我尝试在其他数据集上应用FUNOR_FUNOM时,很明显,使用该技术需要调整。
Another possibility is that these procedures have a blind spot, which the paper itself demonstrates. One of Tukey’s goals was to avoid “…errors as serious as ascribing the wrong sign to the resulting correlation or regression coefficients” (p 58). So it’s perhaps ironic that one of the values in Tukey’s example table of coefficients (Table 8, p 54) has an inverted sign.
另一种可能是这些程序有一个盲点,本文本身就证明了这一点。 Tukey的目标之一是避免出现“……错误,将错误的符号归因于相关系数或回归系数”(第58页)。 因此,具有讽刺意味的是,Tukey的示例系数表(表8,第54页)中的值之一具有反号。
I tested each of Tukey’s procedures, and none of them would have caught the typo: Both the error (-0.100) and the corrected value (0.100) are too close to the relevant medians and means to be noticeable. I found it only because the printed row and column means did not add up to ones that I calculated.
我测试了Tukey的每个过程,但都没有错字:误差(-0.100)和校正值(0.100)都太接近相关的中位数和平均值,因此不明显。 我发现它仅是因为打印的行和列均值不等于我计算出的值。
The flaw isn’t fatal. And, ultimately, the utility of these procedures is beside the point. My real goal with this article is simply to encourage people to read Tukey’s paper and to make that task a little easier by providing the intuitive explanations that I myself had wanted.
该缺陷不是致命的。 最终,这些程序的实用性已不重要。 我写这篇文章的真正目的仅仅是鼓励人们阅读Tukey的论文,并通过提供我自己想要的直观解释使这项任务变得更容易。
To be clear, no one should mistake my explanations nor my implementations of Tukey’s techniques as a substitute for reading his paper. “The Future of Data Analysis” contains much more than I’ve covered here, and many of Tukey’s ideas remain just as fresh — and just as relevant — today, including his famous maxim: “Far better an approximate answer to the right question, which is often vague, than an exact answer to the wrong question” (pp 14–15).
明确地说,没有人会误解我的解释或我对Tukey的技术的实现,而不是代替他阅读论文。 “数据分析的未来”包含的内容远远超出了我在这里所讨论的范围,并且Tukey的许多思想在今天仍然一样新鲜和相关,包括他著名的格言:“更好地为正确的问题提供近似答案,这通常比对错误问题的确切答案还模糊”(第14-15页)。
翻译自: https://towardsdatascience.com/back-to-the-future-of-data-analysis-a-tidy-implementation-of-tukeys-vacuum-87c561cdee18
tukey检测
本文来自互联网用户投稿,该文观点仅代表作者本人,不代表本站立场。本站仅提供信息存储空间服务,不拥有所有权,不承担相关法律责任。如若转载,请注明出处:http://www.mzph.cn/news/391896.shtml
如若内容造成侵权/违法违规/事实不符,请联系多彩编程网进行投诉反馈email:809451989@qq.com,一经查实,立即删除!