mfcc中的fft操作
What we should know about sound. Sound is produced when there’s an object that vibrates and those vibrations determine the oscillation of air molecules which creates an alternation of air pressure and this high pressure alternated with low pressure causes a wave.
w ^ 帽子,我们应该知道的声音。 当有物体振动时,就会产生声音,而这些振动决定了空气分子的振动,从而产生了气压的交替变化,而这种高压与低压交替产生的波动。
Some key terms in audio processing.
音频处理中的一些关键术语。
- Amplitude — Perceived as loudness 振幅-视为响度
- Frequency — Perceived as pitch 频率-视为音高
- Sample rate — It is how many times the sample is taken of a sound file if it says sample rate as 22000 Hz it means 22000 samples are taken in each second. 采样率—如果声音文件的采样率表示为22000 Hz,则它是对声音文件进行采样的次数,这表示每秒进行22000个采样。
- Bit depth — It represents the quality of sound recorded, It just likes pixels in an image. So 24 Bit sound is of better quality than 16 Bit. 位深度—它代表所记录声音的质量,就像图像中的像素一样。 因此,24位声音的质量比16位更好。
Here I have used the sound of a piano key from freesound.org
在这里,我使用了freesound.org上钢琴琴键的声音
signal, sample_rate = librosa.load(file, sr=22050)
plt.figure(figsize=FIG_SIZE)
librosa.display.waveplot(signal, sample_rate, alpha=0.4)
plt.xlabel(“Time (s)”)
plt.ylabel(“Amplitude”)
plt.title(“Waveform”)
plt.savefig(‘waveform.png’, dpi=100)
plt.show()

To move wave from a time domain to frequency domain we need to perform Fast Fourier Transform on data. Basically what we do with the Fourier transform is the process of decomposing a periodic sound into a sum of sine waves which all vibrate oscillate at different frequencies. It is quite incredible so we can describe a very complex sound as long as it’s periodic as a sum as the superimposition of a bunch of different sine waves at different frequencies.
为了将波从时域移动到频域,我们需要对数据执行快速傅立叶变换 。 基本上,我们对傅立叶变换所做的是将周期性声音分解为正弦波之和的过程,这些正弦波均以不同的频率振动。 这是非常不可思议的,因此我们可以描述一个非常复杂的声音,只要它是周期性的,与一堆不同频率的不同正弦波的叠加相加即可。
Below I have shown how two sine waves of different amplitude and frequency are combined into one.
下面我展示了如何将两个振幅和频率不同的正弦波组合为一个。

# perform Fourier transform
fft = np.fft.fft(signal)# calculate abs values on complex numbers to get magnitude
spectrum = np.abs(fft)# create frequency variable
f = np.linspace(0, sample_rate, len(spectrum))# take half of the spectrum and frequency
left_spectrum = spectrum[:int(len(spectrum)/2)]
left_f = f[:int(len(spectrum)/2)]# plot spectrum
plt.figure(figsize=FIG_SIZE)
plt.plot(left_f, left_spectrum, alpha=0.4)
plt.xlabel(“Frequency”)
plt.ylabel(“Magnitude”)
plt.title(“Power spectrum”)
plt.savefig(‘FFT.png’)
plt.show()

By applying the Fourier transform we move in the frequency domain because here we have on the x-axis the frequency and the magnitude is a function of the frequency itself but by this we lose information about time so it’s as if this a special power spectrum here was a snapshot of all the elements which concur to form this sound, so basically what this spectrum is telling us is that these different frequencies have different powers but throughout all of them all of the sound here so it’s a snapshot it’s a static which could be seen as a problem because obviously audio data alike is a time series right so things change in time and so we want to know about how things change in time and it seems that with the Fourier transform we we can’t really do that so we are missing on a lot of information right but obviously there’s a solution to that and the solution it’s called the Short Time Fourier Transform or STFT and so what the short time Fourier transform does it computes several Fourier transforms at different intervals and in doing so it preserves information about time and the way sound evolved it’s over time right and so the different intervals at which we perform the Fourier transform is given by the frame size and so a frame is a bunch of samples and so we fix the number of samples and we say let’s consider only for example 200 samples and do the Fourier transform there and then let’s move on to let’s shift and move on to to the rest lack of the waveform and what happens here is that we get a spectogram which gives us information of (time + frequency + magnitude)
通过应用傅立叶变换,我们在频域中移动,因为在这里我们在x轴上具有频率,并且幅度是频率本身的函数,但是由于此,我们会丢失有关时间的信息,因此好像这是一个特殊的功率谱是构成声音的所有元素的快照,因此,基本上,该频谱告诉我们的是,这些不同的频率具有不同的功率,但是在所有这些声音中,所有这些声音都是静态的,因此它是静态的,可能是之所以认为这是一个问题,是因为显然音频数据都是一个时间序列,所以事情会随时间变化,因此我们想知道事情是如何随时间变化的,似乎通过傅立叶变换我们无法真正做到这一点,所以缺少很多信息,但是很显然,有一个解决方案,该解决方案称为短时傅立叶变换或STFT ,因此短时傅立叶变换所执行的操作将计算出几个傅立叶tra ns以不同的时间间隔进行变换,这样就保留了有关时间以及声音随时间变化的信息,因此执行傅立叶变换的不同时间间隔由帧大小给出,因此一帧是一堆样本,因此我们确定了样本数量,我们说让我们仅考虑例如200个样本,然后在其中进行傅立叶变换,然后继续进行下去,移至剩下的没有波形的地方,这就是我们得到的一张频谱图,它为我们提供了(时间+频率+幅度)信息
# STFT -> spectrogram
hop_length = 512 # in num. of samples
n_fft = 2048 # window in num. of samples
# calculate duration hop length and window in seconds
hop_length_duration = float(hop_length)/sample_rate
n_fft_duration = float(n_fft)/sample_rate
print(“STFT hop length duration is:{}s”.format(hop_length_duration))
print(“STFT window duration is: {}s”.format(n_fft_duration))
# perform stft
stft = librosa.stft(signal, n_fft=n_fft, hop_length=hop_length)
# calculate abs values on complex numbers to get magnitude
spectrogram = np.abs(stft)
# display spectrogram
plt.figure(figsize=FIG_SIZE)
librosa.display.specshow(spectrogram, sr=sample_rate, hop_length=hop_length)
plt.xlabel(“Time”)
plt.ylabel(“Frequency”)
plt.colorbar()
plt.title(“Spectrogram”)
plt.savefig(‘spectogram.png’)
plt.show()
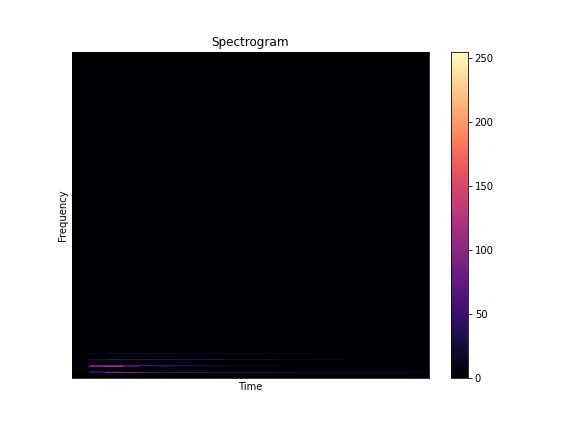
# apply logarithm to cast amplitude to Decibels
log_spectrogram = librosa.amplitude_to_db(spectrogram)
plt.figure(figsize=FIG_SIZE)
librosa.display.specshow(log_spectrogram, sr=sample_rate,
hop_length=hop_length)
plt.xlabel(“Time”)
plt.ylabel(“Frequency”)
plt.colorbar(format=”%+2.0f dB”)
plt.title(“Spectrogram (dB)”)
plt.savefig(‘spectogram_log.png’)
plt.show()

we have time here on the x-axis but we also have frequency on the y-axis and we have a third axis which is given by the colour and the colour is telling us how much a given frequency is present in the sound at a given time so for example here we see that low-frequency sound is more in the most of the audio.
我们在x轴上有时间,但在y轴上也有频率,还有第三个轴,该第三轴由颜色给定,颜色告诉我们在给定的声音中给定的频率有多少时间,例如,在这里我们看到低频声音在大多数音频中更多。
Mel Frequncy Cepstral Spectogram in short MFCC’s capture many aspects of sound so if you have for example a guitar or flute playing the same melody you would have potentially same frequency and same rhythm more or less there depending on the performance but what would change is the quality of sound and the MFCC’s are capable of capturing that information and for extracting them MFCC’s we perform a Fourier transform and we move from the time domain in so you the frequency domain so MFCC’s are basically frequency domain feature but the great advantage of MFCC’s over spectrograms is that they approximate the human auditory system they try to model the way we perceive frequency right and so that’s very important if you then want to do deep learning stuff to have some data that represent the way we kind of process audio now the results of extracting MFCC’s is a bunch of coefficients it’s an MFCC vector and so you can specify a number of different coefficients usually in all your music applications you want to use between 13 to 39 coefficients and then again you are going to calculate all of these coefficients at each frame so that you have an idea of how the M FCC’ are evolving over time right.
简短的MFCC的 “ Mel频率倒谱 ”可以捕获声音的许多方面,因此,例如,如果吉他或长笛演奏相同的旋律,则根据性能可能会或多或少地具有相同的频率和相同的节奏,但是改变的是声音的质量和MFCC能够捕获该信息并提取它们,我们执行傅立叶变换,并且从时域移到频域,因此MFCC基本上是频域功能,但MFCC的巨大优势在于频谱图是他们近似于人类听觉系统,他们试图对我们正确感知频率的方式进行建模,因此如果您想进行深度学习以获取一些数据来表示我们现在处理音频的方式,那么这非常重要。提取MFCC是一堆系数,它是MFCC向量,因此您通常可以在所有音乐应用程序中指定许多不同的系数 您希望使用13到39个系数,然后再次计算每个帧上的所有这些系数,这样您就可以正确了解M FCC'随时间的变化情况。
# MFCCs
# extract 13 MFCCs
MFCCs = librosa.feature.mfcc(signal, sample_rate, n_fft=n_fft,
hop_length=hop_length, n_mfcc=13)
# display MFCCs
plt.figure(figsize=FIG_SIZE)
librosa.display.specshow(MFCCs, sr=sample_rate,
hop_length=hop_length)
plt.xlabel(“Time”)
plt.ylabel(“MFCC coefficients”)
plt.colorbar()
plt.title(“MFCCs”)
plt.savefig(‘mfcc.png’)
plt.show()

so here I have 13 MFCC’s coefficient represented in the y-axis, time in the x-axis and more the red, more is the value of that coefficient in that time frame.
因此,在这里,我有13个MFCC系数,以y轴表示,时间以x轴表示,红色越多,则该系数在该时间范围内的值越大。
MFCC’s are used for a number of the audio application. Originally they have been introduced for speech recognition, but it also has uses in music recognition, music instrument classification, music genre classification.
MFCC用于许多音频应用程序。 最初将它们引入语音识别,但它也用于音乐识别,乐器分类,音乐体裁分类。
Link to code:
链接到代码:
Code for sine waves
正弦波代码
Code for FFT, STFT and MFCC’s
FFT,STFT和MFCC的代码
翻译自: https://medium.com/analytics-vidhya/simplifying-audio-data-fft-stft-mfcc-for-machine-learning-and-deep-learning-443a2f962e0e
mfcc中的fft操作
本文来自互联网用户投稿,该文观点仅代表作者本人,不代表本站立场。本站仅提供信息存储空间服务,不拥有所有权,不承担相关法律责任。如若转载,请注明出处:http://www.mzph.cn/news/391832.shtml
如若内容造成侵权/违法违规/事实不符,请联系多彩编程网进行投诉反馈email:809451989@qq.com,一经查实,立即删除!