php amazon-s3
Item-based collaborative and User-based collaborative approach for recommendation system with simple coding.
推荐系统的基于项目的协作和基于用户的协作方法,编码简单。
推荐系统概述 (Overview of Recommendation System)
There are many methods of recommendation system where each of them serve for different purposes. My previous article is talking about the simple and content-based recommendation. These recommendations are non-personalised recommenders, but that doesn’t mean they are less useful when compare to the other. These method are very popular for recommending top music of the week and recommending music of similar genre.
推荐系统的方法很多,每种方法都有不同的用途。 我的上一篇文章讨论的是基于内容的简单推荐。 这些推荐是非个性化的推荐者,但这并不意味着它们与其他推荐相比没有太大用处。 这些方法在推荐本周热门音乐和推荐类似流派的音乐时非常流行。
In this article, it will focus on collaborative filtering method. This method considers your taste in comparison to people/items that are in similar. Then, it recommends a list of items based on consumption similarity and suggest what you probably interested. These method only focus on calculating the rating.
在本文中,它将重点介绍协作过滤方法。 与相似的人/物品相比,此方法考虑了您的口味。 然后,它根据消费相似性推荐商品清单,并建议您可能感兴趣的商品。 这些方法仅专注于计算等级 。
There are two main filtering for this method: item-based filtering and user-based filtering. Item-based filtering will suggest items that are similar to what you have already liked. User-based filtering will suggest items that people similar to you have liked but you have not yet consumed.
此方法主要有两种过滤:基于项目的过滤和基于用户的过滤。 基于项目的过滤将建议与您喜欢的项目相似的项目。 基于用户的过滤将建议与您相似的人喜欢但尚未消耗的物品。
With the Amazon movie data, we will apply item-based filtering and user-based filtering recommendation methods to analyze similar items to be recommend and identify users that have similar taste.
借助Amazon电影数据 ,我们将应用基于项目的过滤和基于用户的过滤推荐方法来分析要推荐的相似项目并识别具有相似品味的用户。
分析概述 (Analysis Overview)
For both item-based filtering and user-based filtering recommendation, we need to clean data and prepare them into matrix so that it can be used for analysis. All ratings need to be in numbers and normalized and cosine similarity will be used to calculate items/users similarity.
对于基于项目的过滤和基于用户的过滤建议,我们都需要清理数据并将它们准备成矩阵,以便可以将其用于分析。 所有等级都必须以数字表示并进行归一化,余弦相似度将用于计算项目/用户相似度。
资料总览 (Data Overview)
There are 4,848 users with a total of 206 movies in the dataset.
数据集中有4848位用户,总共206部电影。
实作 (Implementation)
Now, lets import all tools that we are going to use for the analysis, put data into DataFrame, and clean them.
现在,让我们导入我们将用于分析的所有工具,将数据放入DataFrame并清理它们。
import pandas as pd
import numpy as np
import scipy as sp
from scipy import sparse
from sklearn.metrics.pairwise import cosine_similarityamazon = pd.read_csv('.../Amazon.csv')
amazon.info()
amazon.head()


Then, we need to rearrange data into matrix format where we will set index for the rows as user_id and index for the column as name.
然后,我们需要将数据重新排列为矩阵格式,在该格式中,将行的索引设置为user_id,将列的索引设置为name。
amazon = amazon.melt(id_vars=['user_id'], var_name='name', value_name='rating')
amazon_pivot = amazon.pivot_table(index=['user_id'], columns=['name'], values='rating')
amazon_pivot.head()

From here, we need to normalized the rating values so that value range are closer to one and another. Then, turn the NaN values into 0 and select only those users who at least rate one movie.
从这里开始,我们需要对评级值进行归一化,以使值范围彼此接近。 然后,将NaN值设置为0,然后仅选择至少对一部电影评分的用户。
amazon_normalized = amazon_pivot.apply(lambda x: (x-np.min(x))/(np.max(x)-np.min(x)), axis=1)amazon_normalized.fillna(0, inplace=True)
amazon_normalized = amazon_normalized.T
amazon_normalized = amazon_normalized.loc[:, (amazon_normalized !=0).any(axis=0)]

We nearly there. Now we need to put them into sparse matrix.
我们快到了。 现在我们需要将它们放入稀疏矩阵。
amazon_sparse = sp.sparse.csr_matrix(amazon_normalized.values)
Lets look at item-based filtering recommendation.
让我们看一下基于项目的过滤建议 。
item_similarity = cosine_similarity(amazon_sparse)
item_sim_df = pd.DataFrame(item_similarity, index=amazon_normalized.index, columns=amazon_normalized.index)
item_sim_df.head()

All the columns and rows are now become each of the movie and it is ready for the recommendation calculation.
现在,所有的列和行都成为电影的每一个,并且可以进行推荐计算了。
def top_movie(movie_name):
for item in item_sim_df.sort_values(by = movie_name, ascending = False).index[1:11]:
print('Similar movie:{}'.format(item))top_movie("Movie102")

These are the movies that are similar to Movie102.
这些是与Movie102类似的电影。
Lets look at user-based filtering recommendation. Who has similar taste to me?
让我们看一下基于用户的过滤推荐 。 谁有和我相似的品味?
user_similarity = cosine_similarity(amazon_sparse.T)
user_sim_df = pd.DataFrame(user_similarity, index = amazon_normalized.columns, columns = amazon_normalized.columns)
user_sim_df.head()

def top_users(user):
sim_values = user_sim_df.sort_values(by=user, ascending=False).loc[:,user].tolist()[1:11]
sim_users = user_sim_df.sort_values(by=user, ascending=False).index[1:11]
zipped = zip(sim_users, sim_values)
for user, sim in zipped:
print('User #{0}, Similarity value: {1:.2f}'.format(user, sim))top_users('A140XH16IKR4B0')
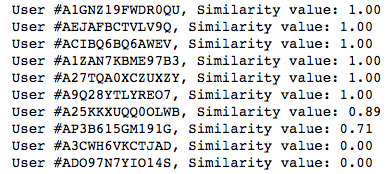
These are the examples on how to implement the item-based and user-based filtering recommendation system. Some of the code are from https://www.kaggle.com/ajmichelutti/collaborative-filtering-on-anime-data
这些是有关如何实施基于项目和基于用户的过滤推荐系统的示例。 一些代码来自https://www.kaggle.com/ajmichelutti/collaborative-filtering-on-anime-data
Hope that you enjoy!
希望你喜欢!
翻译自: https://medium.com/analytics-vidhya/recommend-amazon-movie-a-collaborative-approach-9b3db8f48ad6
php amazon-s3
本文来自互联网用户投稿,该文观点仅代表作者本人,不代表本站立场。本站仅提供信息存储空间服务,不拥有所有权,不承担相关法律责任。如若转载,请注明出处:http://www.mzph.cn/news/391226.shtml
如若内容造成侵权/违法违规/事实不符,请联系多彩编程网进行投诉反馈email:809451989@qq.com,一经查实,立即删除!