简述yolo1-yolo3
重点 (Top highlight)
目录: (Table Of Contents:)
- Introduction 介绍
- Why YOLO? 为什么选择YOLO?
- How does it work? 它是如何工作的?
- Intersection over Union (IoU) 联合路口(IoU)
- Non-max suppression 非最大抑制
- Network Architecture 网络架构
- Training 训练
- Limitation of YOLO YOLO的局限性
- Conclusion 结论
介绍: (Introduction:)
You Only Look Once (YOLO) is a new and faster approach to object detection. Traditional systems repurpose classifiers to perform detection. Basically, to detect any object, the system takes a classifier for that object and then classifies its presence at various locations in the image. Other systems generate potential bounding boxes in an image using region proposal methods and then run a classifier on these potential boxes. This results in a slightly efficient method. After classification, post-processing is used to refine the bounding boxes, eliminate duplicate detection, etc. Due to these complexities, the system becomes slow and hard to optimize because each component has to be trained separately.
“只看一次”(YOLO)是一种新的且更快的对象检测方法。 传统系统重新利用分类器来执行检测。 基本上,要检测任何物体,系统会对该物体进行分类,然后将其在图像中各个位置的存在进行分类。 其他系统使用区域提议方法在图像中生成潜在的边界框,然后在这些潜在的框上运行分类器。 这导致一种稍微有效的方法。 分类后,使用后处理来完善边界框,消除重复检测等。由于这些复杂性,系统变得缓慢且难以优化,因为每个组件都必须单独训练。

为什么选择YOLO? (Why YOLO?)
The base model can process images in real-time at 45 frames per second. A smaller version of the network, Fast YOLO can process images at 155 frames per second while achieving double the mAP of other real-time detectors. It outperforms other detection methods, including DPM (Deformable Parts Models) and R-CNN.
基本模型可以每秒45帧的速度实时处理图像。 Fast YOLO是网络的较小版本,可以每秒155帧的速度处理图像,同时使其他实时检测器的mAP达到两倍。 它优于其他检测方法,包括DPM(可变形零件模型)和R-CNN。
它是如何工作的? (How Does It Work?)
YOLO reframes object detection as a single regression problem instead of a classification problem. This system only looks at the image once to detect what objects are present and where they are, hence the name YOLO.
YOLO将对象检测重新构造为单个回归问题,而不是分类问题。 该系统仅查看图像一次即可检测出存在的物体及其位置,因此命名为YOLO。
The system divides the image into an S x S grid. Each of these grid cells predicts B bounding boxes and confidence scores for these boxes. The confidence score indicates how sure the model is that the box contains an object and also how accurate it thinks the box is that predicts. The confidence score can be calculated using the formula:
系统将图像划分为S x S网格。 这些网格单元中的每一个都预测B边界框和这些框的置信度得分。 置信度得分表明模型对盒子是否包含对象的确信程度,以及模型认为盒子预测的准确性。 置信度得分可以使用以下公式计算:
C = Pr(object) * IoU
C = Pr(对象)* IoU
IoU: Intersection over Union between the predicted box and the ground truth.
IoU:预测框与地面实况之间的并集交集。
If no object exists in a cell, its confidence score should be zero.
如果单元格中不存在任何对象,则其置信度得分应为零。
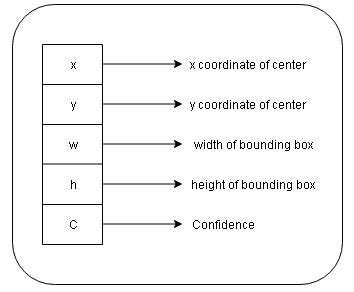
Each bounding box consists of five predictions: x, y, w, h, and confidence where,
每个边界框均包含五个预测: x,y,w,h和置信度,其中,
(x,y): Coordinates representing the center of the box. These coordinates are calculated with respect to the bounds of the grid cells.
(x,y):表示框中心的坐标。 这些坐标是相对于网格单元的边界计算的。
w: Width of the bounding box.
w:边框的宽度。
h: Height of the bounding box.
h:边框的高度。
Each grid cell also predicts C conditional class probabilities Pr(Classi|Object). It only predicts one set of class probabilities per grid cell, regardless of the number of boxes B. During testing, these conditional class probabilities are multiplied by individual box confidence predictions which give class-specific confidence scores for each box. These scores show both the probability of that class and how well the box fits the object.
每个网格单元还预测C个条件类别概率Pr(Classi | Object) 。 不管框B的数量如何,它仅预测每个网格单元的一组类别概率。在测试期间,这些条件类别概率乘以各个框的置信度预测,从而为每个框提供特定于类别的置信度得分。 这些分数既显示了该类别的可能性,也显示了盒子适合对象的程度。
Pr(Class i|Object)*Pr(Object)*IoU = Pr(Class i)*IoU.
Pr(类i |对象)* Pr(对象)* IoU = Pr(类i)* IoU。
The final predictions are encoded as an S x S x (B*5 + C) tensor.
最终预测被编码为S x S x(B * 5 + C)张量。
联合路口(IoU): (Intersection Over Union (IoU):)
IoU is used to evaluate the object detection algorithm. It is the overlap between the ground truth and the predicted bounding box, i.e it calculates how similar the predicted box is with respect to the ground truth.
IoU用于评估对象检测算法。 它是基本事实和预测边界框之间的重叠,即,它计算了预测框相对于基本事实的相似程度。




Usually, the threshold for IoU is kept as greater than 0.5. Although many researchers apply a much more stringent threshold like 0.6 or 0.7. If a bounding box has an IoU less than the specified threshold, that bounding box is not taken into consideration.
通常,IoU的阈值保持大于0.5。 尽管许多研究人员采用了更为严格的阈值,例如0.6或0.7。 如果边界框的IoU小于指定的阈值,则不考虑该边界框。
非最大抑制: (Non-Max Suppression:)
The algorithm may find multiple detections of the same object. Non-max suppression is a technique by which the algorithm detects the object only once. Consider an example where the algorithm detected three bounding boxes for the same object. The boxes with respective probabilities are shown in the image below.
该算法可以找到同一物体的多个检测。 非最大抑制是一种算法,算法仅将对象检测一次。 考虑一个示例,该算法检测到同一对象的三个边界框。 下图显示了具有相应概率的框。

The probabilities of the boxes are 0.7, 0.9, and 0.6 respectively. To remove the duplicates, we are first going to select the box with the highest probability and output that as a prediction. Then eliminate any bounding box with IoU > 0.5 (or any threshold value) with the predicted output. The result will be:
框的概率分别为0.7、0.9和0.6。 要删除重复项,我们首先选择具有最高概率的框,然后将其输出作为预测。 然后,用预测输出消除IoU> 0.5(或任何阈值)的任何边界框。 结果将是:
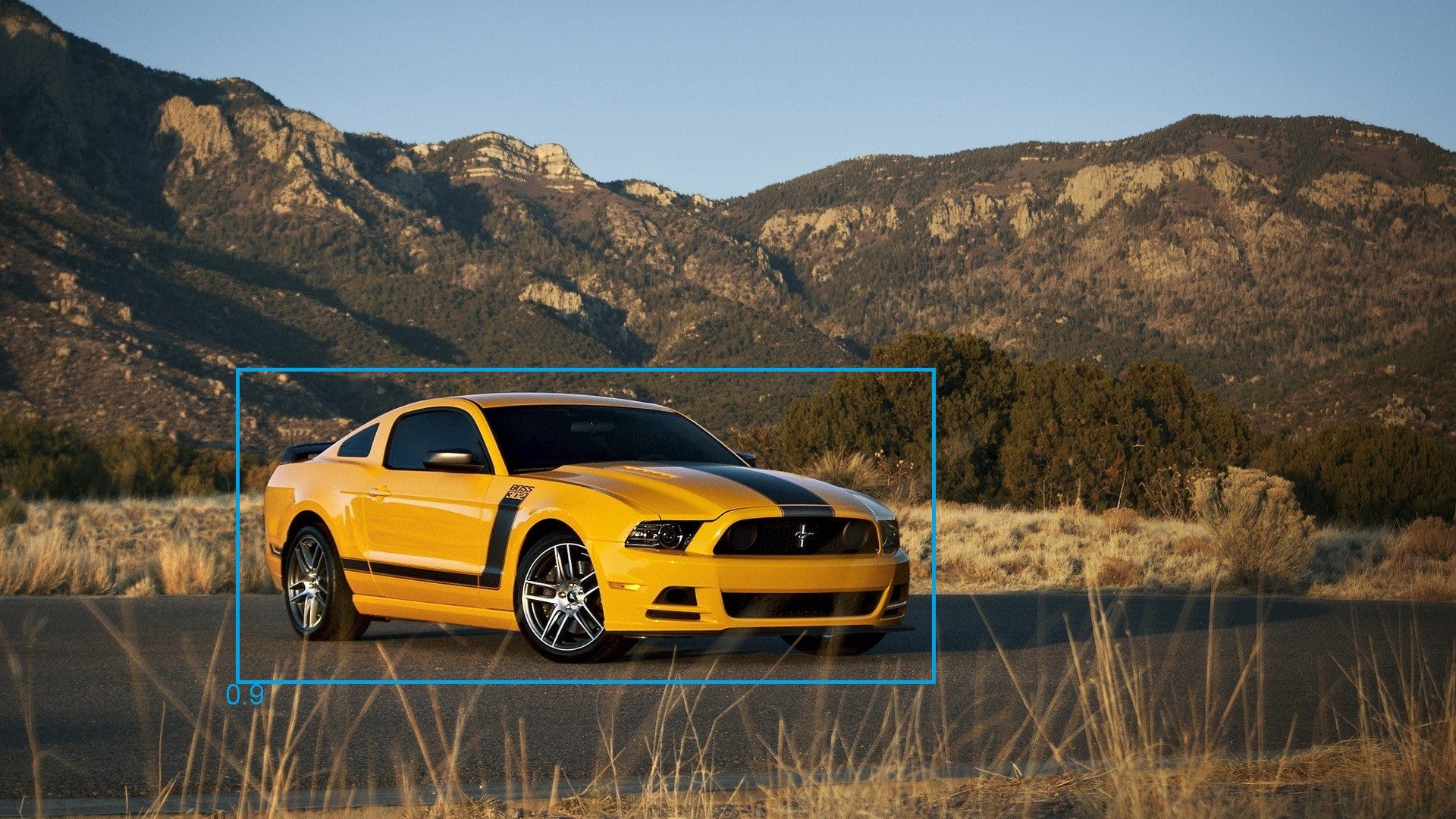
网络架构: (Network Architecture:)
The base model has 24 convolutional layers followed by 2 fully connected layers. It uses 1 x 1 reduction layers followed by a 3 x 3 convolutional layer. Fast YOLO uses a neural network with 9 convolutional layers and fewer filters in those layers. The complete network is shown in the figure.
基本模型具有24个卷积层,然后是2个完全连接的层。 它使用1 x 1缩小层,然后是3 x 3卷积层。 Fast YOLO使用具有9个卷积层和较少层过滤器的神经网络。 完整的网络如图所示。

Note:
注意:
- The architecture was designed for use in the Pascal VOC dataset, where S = 7, B = 2, and C = 20. This is the reason why final feature maps are 7 x 7, and also the output tensor is of the shape (7 x 7 x (2*5 + 20)). To use this network with a different number of classes or different grid size you might have to tune the layer dimensions. 该体系结构设计用于Pascal VOC数据集,其中S = 7,B = 2和C =20。这就是为什么最终特征图为7 x 7以及输出张量为(7 x 7 x(2 * 5 + 20)。 若要将此网络用于不同数量的类或不同的网格尺寸,则可能必须调整图层尺寸。
- The final layer uses a linear activation function. The rest uses a leaky ReLU. 最后一层使用线性激活函数。 其余使用泄漏的ReLU。
训练: (Training:)
- Pre train the first 20 convolutional layers on the ImageNet 1000-class competition dataset followed by average — pooling layer and a fully connected layer. 在ImageNet 1000类竞赛数据集上训练前20个卷积层,然后进行平均-池化层和完全连接的层。
- Since detection requires better visual information, increase the input resolution from 224 x 224 to 448 x 448. 由于检测需要更好的视觉信息,因此将输入分辨率从224 x 224增加到448 x 448。
- Train the network for 135 epochs. Throughout the training, use a batch size of 64, a momentum of 0.9, and a decay of 0.0005. 训练网络135个纪元。 在整个训练过程中,请使用64的批量大小,0.9的动量和0.0005的衰减。
- Learning Rate: For first epochs raise the learning rate from 10–3 to 10–2, else the model diverges due to unstable gradients. Continue training with 10–2 for 75 epochs, then 10–3 for 30 epochs, and then 10–4 for 30 epochs. 学习率:首先,将学习率从10–3提高到10–2,否则模型由于不稳定的梯度而发散。 继续训练10–2代表75个时期,然后10–3代表30个时期,然后10–4代表30个时期。
- To avoid overfitting, use dropout and data augmentation. 为避免过度拟合,请使用辍学和数据扩充。
YOLO的局限性: (Limitations Of YOLO:)
- Spatial constraints on bounding box predictions as each grid cell only predicts two boxes and can have only one class. 边界框预测的空间约束,因为每个网格单元仅预测两个框,并且只能具有一个类别。
- It is difficult to detect small objects that appear in groups. 很难检测出现在组中的小物体。
- It struggles to generalize objects in new or unusual aspect ratios as the model learns to predict bounding boxes from data itself. 当模型学习从数据本身预测边界框时,它很难以新的或不寻常的宽高比来概括对象。
结论: (Conclusion:)
This was a brief explanation of the research paper as well as details obtained from various other sources. I hope I made this concept easier for you to understand.
这是对研究论文的简要说明,以及从其他各种来源获得的详细信息。 希望我使这个概念更容易理解。
Although if you really want to check your understanding, the best way is to implement the algorithm. In the next section, we will do exactly that. Many details cannot be explained via text and can only be understood while implementing it.
尽管如果您真的想检查自己的理解,最好的方法是实现算法。 在下一节中,我们将完全做到这一点。 许多细节无法通过文本解释,只能在实施过程中理解。
Thank you for reading. Click here to go to the next part.
感谢您的阅读。 单击此处转到下一部分。
翻译自: https://towardsdatascience.com/object-detection-part1-4dbe5147ad0a
简述yolo1-yolo3
本文来自互联网用户投稿,该文观点仅代表作者本人,不代表本站立场。本站仅提供信息存储空间服务,不拥有所有权,不承担相关法律责任。如若转载,请注明出处:http://www.mzph.cn/news/391220.shtml
如若内容造成侵权/违法违规/事实不符,请联系多彩编程网进行投诉反馈email:809451989@qq.com,一经查实,立即删除!