nlp自然语言处理
自然语言处理 (Natural Language Processing)
到底是怎么回事? (What is going on?)
NLP is the new Computer Vision
NLP是新的计算机视觉
With enormous amount go textual datasets available; giants like Google, Microsoft, Facebook etc have diverted their focus towards NLP.
大量可用的文本数据集; 谷歌,微软,Facebook等巨头已经将注意力转向了自然语言处理。
Models using thousands of super-costly TPUs/GPUs, making them infeasible for most.
使用成千上万的价格昂贵的TPU / GPU进行建模,这对于大多数人来说是不可行的。
This gave me anxiety! (we’ll come back to that)
这让我感到焦虑! (我们会回到那个)
Let’s these Tweets put things into perspective:
让我们通过以下Tweet透视事物:
Tweet 1:
鸣叫1:
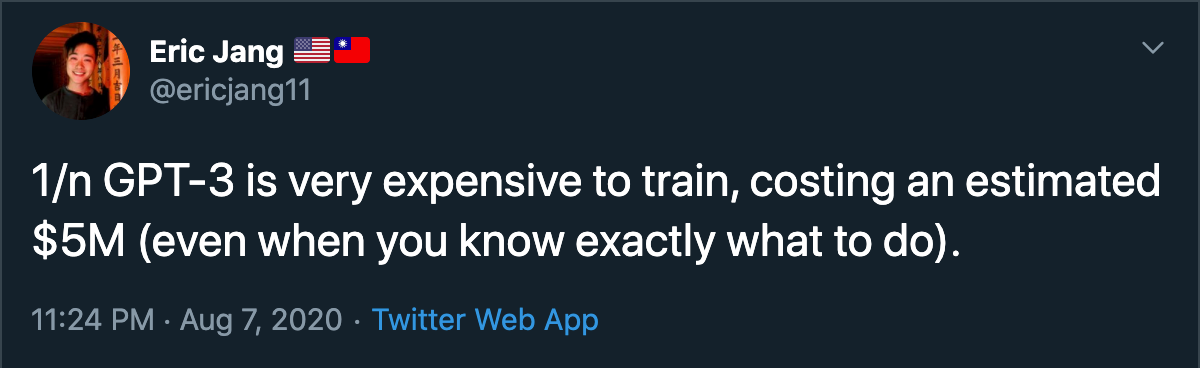
Tweet 2: (read the trailing tweet)
鸣叫2 :(请 阅读尾随的鸣叫)
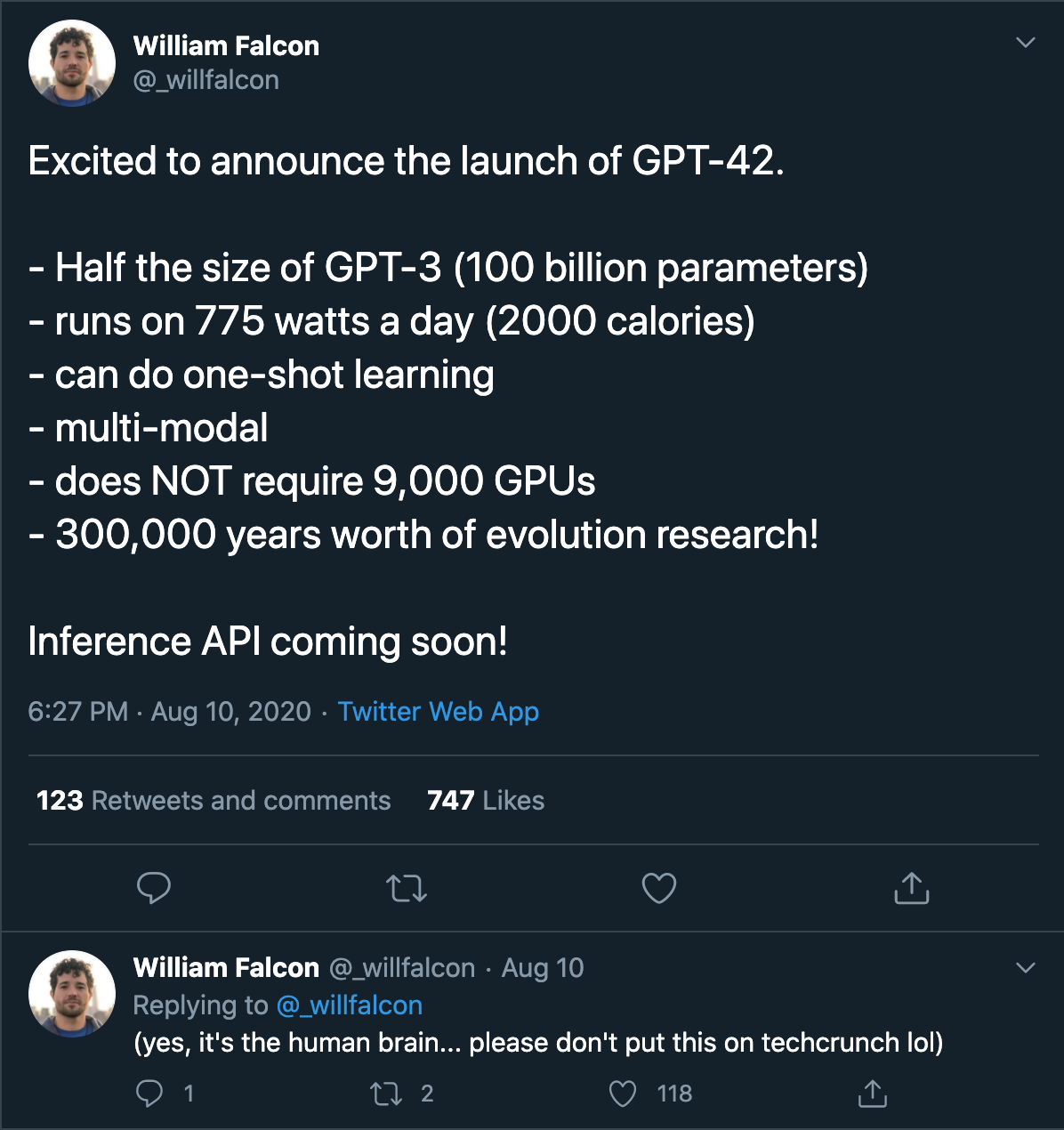
结果呢? (Consequences?)
In about last one-year following knowledge became mainstream:
在大约一年的时间里,以下知识已成为主流:
- Transformers was followed by Reformer, Longformer, GTrXL, Linformer, and others. 紧随其后的是Transformers,Reformer,Longformer,GTrXL,Linformer等。
- BERT was followed by XLNet, RoBERTa, AlBERT, Electra, BART, T5, Big Bird, and others. BERT之后是XLNet,RoBERTa,AlBERT,Electra,BART,T5,Big Bird等。
- Model Compression was extended by DistilBERT, TinyBERT, BERT-of-Theseus, Huffman Coding, Movement Pruning, PrunBERT, MobileBERT, and others. 模型压缩由DistilBERT,TinyBERT,Theseus BERT,Huffman编码,Motion Pruning,PrunBERT,MobileBERT等扩展。
- Even new tokenizations were introduced: Byte-Pair encoding (BPE), Word-Piece Encoding (WPE), Sentence-Piece Encoding (SPE), and others. 甚至引入了新的标记化:字节对编码(BPE),字片编码(WPE),句子片编码(SPE)等。
This is barely the tip of the iceberg.
这仅仅是冰山一角。
So while you were trying to understand and implement a model, a bunch of new lighter and faster models were already available.
因此,当您尝试理解和实现模型时,已经有很多新的更轻,更快的模型。
如何应付呢? (How to Cope with it?)
The answer is short:
答案很简短:
you don’t need to know it all, know only what is necessary and use what is available
您不需要一无所知,只知道什么是必要的,并使用可用的
原因 (Reason)
I read them all to realize most of the research is re-iteration of similar concepts.
我阅读了所有内容,以了解大部分研究是对类似概念的重复 。
At the end of the day (vaguely speaking):
在一天结束时(含糊地说):
- the reformer is hashed version of the transformers and longfomer is a convolution-based counterpart of the transformers 重整器是变压器的哈希版本,而longfomer是变压器的基于卷积的对应形式
- all compression techniques are trying to consolidate information 所有压缩技术都在尝试整合信息
- everything from BERT to GPT3 is just a language model 从BERT到GPT3的一切都只是一种语言模型
优先级->准确性管道 (Priorities -> Pipeline over Accuracy)
Learn to use what’s available, efficiently, before jumping on to what else can be used
在跳到其他可用功能之前,学会有效地使用可用的功能
In practice, these models are a small part of a much bigger pipeline.
实际上,这些模型只是规模更大的产品线的一小部分 。
Your first instinct should not be of competeing with Tech Giants’ in-terms of training a better model.
您的第一个本能不应该是与Tech Giants在训练更好模型方面的竞争。
Instead, Your first instinct should be to use the availbale models to build an end-to-end application which solves a practical problem.
相反,您的第一个本能应该是使用availbale模型来构建解决实际问题的端到端应用程序。
Now if you feel that the model is the performance bottleneck of your application; re-train that model or switch to another model.
现在,如果您认为模型是应用程序的性能瓶颈,那就可以了。 重新训练该模型或切换到另一个模型。
Consider the following:
考虑以下:
- Huge deep learning models usually take thousands for GPU hours just to train. 庞大的深度学习模型通常需要数千个小时才能进行GPU训练。
- This increases 10x when you consider hyper-parameter tuning (HP Tuning). 当您考虑进行超参数调整(HP调整)时,这将增加10倍。
- HP Tuning something as efficient as an Electra model can also take a week or two. HP调整与Electra型号一样高效的东西也可能需要一两个星期。
实际方案->实际加速 (Practical Scenario -> The Real Speedup)
Take an example of Q&A Systems. Given millions of documents, for this task, something like ElasticSearch is way more essential to the pipeline than a new Q&A model (comparatively).
以问答系统为例。 给定数百万个文档,对于此任务,相对于新的问答模型,ElasticSearch之类的东西对于管道更重要。
In production success of your pipeline will not (only) be determined by how awesome are your Deep Learning models but also by:
在生产中,成功的流水线(不仅)取决于深度学习模型的出色程度,还取决于:
the latency of the inference time
推理时间的延迟
predictability of the results and boundary cases
结果和边界案例的可预测性
- the ease of fine-tuning 易于调整
- the ease of reproducing the model on a similar dataset 在相似的数据集上再现模型的难易程度
Something like DistilBERT can be scaled to handle Billion queries as beautifully mentioned in this blog by Robolox.
正如Robolox在本博客中提到的那样,可以扩展DistilBERT之类的功能来处理十亿个查询。
While new models can decrease the inference time by 2x-5x.
新模型可以将推理时间减少2x-5x 。
Techniques like quantization, pruning and using Onnx can decrease the inference time by 10x-40x!
量化 ,修剪和使用Onnx等技术可以将推理时间减少10x-40x !
个人经验 (Personal Experience)
I was working on an Event Extraction pipeline, which used:
我正在研究事件提取管道,该管道使用:
- 4 different transformer-based models 4种基于变压器的不同模型
- 1 RNN-based model 1基于RNN的模型
But. At the heart of the entire pipeline were:
但。 整个流程的核心是:
- WordNet 词网
- FrameNet 框架网
- Word2Vec Word2Vec
- Regular-Expressions 常用表达
And. Most of my team’s focus was on:
和。 我团队的大部分精力都放在:
Extraction of text from PPTs, images & tables
从PPT,图像和表格中提取文本
Cleaning & preprocessing text
清洗和预处理文本
Visualization of results
结果可视化
- Optimization of ElasticSearch ElasticSearch的优化
- Format of info for Neo4J Neo4J的信息格式
结论 (Conclusion)
It is more essential to have an average performing pipeline than to have a non-functional pipeline with a few brilliant modules.
具有平均性能的管道比具有一些出色模块的非功能性管道更为重要。
Neither Christopher Manning nor Andrew NG knows it all. They just know what is required and when it is required; well enough.
Christopher Manning和Andrew Andrew都不知道这一切。 他们只知道需要什么,什么时候需要。 足够好。
So, have realistic expectations of yourself.
因此,对自己有现实的期望。
Thank you!
谢谢!
翻译自: https://medium.com/towards-artificial-intelligence/dont-be-overwhelmed-by-nlp-c174a8b673cb
nlp自然语言处理
本文来自互联网用户投稿,该文观点仅代表作者本人,不代表本站立场。本站仅提供信息存储空间服务,不拥有所有权,不承担相关法律责任。如若转载,请注明出处:http://www.mzph.cn/news/391059.shtml
如若内容造成侵权/违法违规/事实不符,请联系多彩编程网进行投诉反馈email:809451989@qq.com,一经查实,立即删除!