mongdb 群集
This is a part 2 of the series analyzing healthcare chart notes using Natural Language Processing (NLP)
这是使用自然语言处理(NLP)分析医疗保健图表笔记的系列文章的第2部分。
In the first part, we talked about cleaning the text and extracting sections of the chart notes which might be useful for further annotation by analysts. Hence, reducing their time in manually going through the entire chart note if they are only looking for “allergies” or “social history”.
在第一部分中 ,我们讨论了清理文本和提取图表注释的各个部分,这可能有助于分析师进一步注释。 因此,如果他们只是在寻找“过敏”或“社会病史”,则可以减少他们手动查看整个图表笔记的时间。
NLP任务: (NLP Tasks:)
Pre-processing and Cleaning
预处理和清洁
Text Summarization — We are here
文字摘要-我们在这里
Topic Modeling using Latent Dirichlet allocation (LDA)
使用潜在Dirichlet分配(LDA)进行主题建模
Clustering
聚类
If you want to try the entire code yourself or follow along, go to my published jupyter notebook on GitHub: https://github.com/gaurikatyagi/Natural-Language-Processing/blob/master/Introdution%20to%20NLP-Clustering%20Text.ipynb
如果您想亲自尝试或遵循整个代码,请转至我在GitHub上发布的jupyter笔记本: https : //github.com/gaurikatyagi/Natural-Language-Processing/blob/master/Introdution%20to%20NLP-Clustering% 20Text.ipynb
数据: (DATA:)
Source: https://mimic.physionet.org/about/mimic/
资料来源: https : //mimic.physionet.org/about/mimic/
Doctors take notes on their computer and 80% of what they capture is not structured. That makes the processing of information even more difficult. Let’s not forget, interpretation of healthcare jargon is not an easy task either. It requires a lot of context for interpretation. Let’s see what we have:
医生会在计算机上做笔记,而所捕获的内容中有80%都是没有结构的。 这使得信息处理更加困难。 别忘了,对医疗术语的解释也不是一件容易的事。 它需要很多上下文来进行解释。 让我们看看我们有什么:
文字摘要 (Text Summarization)
Spacy isn’t great at identifying the “Named Entity Recognition” of healthcare documents. See below:
Spacy不能很好地识别医疗文档的“命名实体识别”。 见下文:
doc = nlp(notes_data["TEXT"][178])
text_label_df = pd.DataFrame({"label":[ent.label_ for ent in doc.ents],
"text": [ent.text for ent in doc.ents]
})
display(HTML(text_label_df.head(10).to_html()))
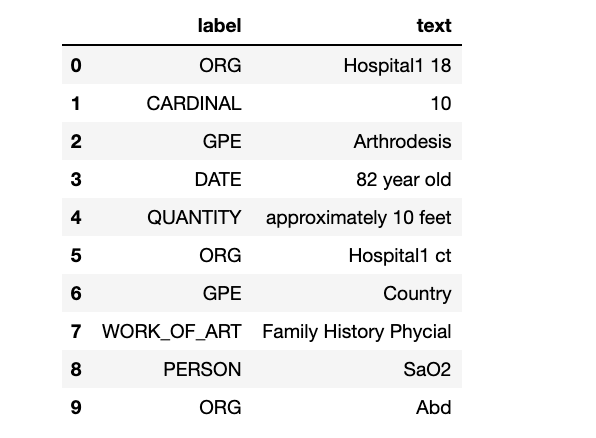
But, that does not mean it can not be used to summarize our text. It is still great at identifying the dependency in the texts using “Parts of Speech tagging”. Let’s see:
但是,这并不意味着它不能用来总结我们的文字。 在使用“词性标签”来识别文本中的依存关系方面仍然很棒。 让我们来看看:
# Process the text
doc = nlp(notes_data["TEXT"][174][:100])
print(notes_data["TEXT"][174][:100], "\n")
# Iterate over the tokens in the doc
for token in doc:
if not (token.pos_ == 'DET' or token.pos_ == 'PUNCT' or token.pos_ == 'SPACE' or 'CONJ' in token.pos_):
print(token.text, token.pos_)
print("lemma:", token.lemma_)
print("dependency:", token.dep_, "- ", token.head.orth_)
print("prefix:", token.prefix_)
print("suffix:", token.suffix_)
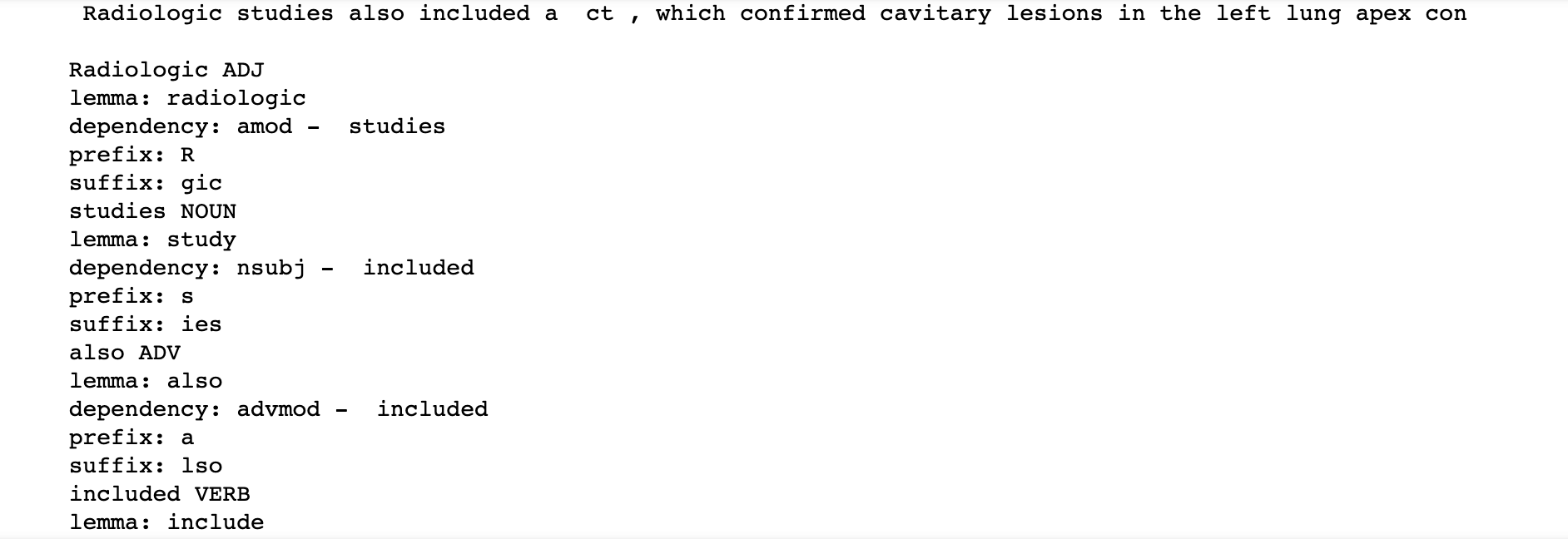
So, we can summarize the text; based on the dependency tracking. YAYYYYY!!!
因此,我们可以总结文本; 基于依赖项跟踪。 耶!
Here are the results for the summary! (btw, I tried zooming out my jupyter notebook to show you the text difference, but still failed to capture the chart notes in its entirety. I’ll paste these separately as well or you can check my output on the Github page(mentioned at the top).
这是摘要的结果! (顺便说一句,我尝试将jupyter笔记本放大以显示文本差异,但仍然无法完整捕获图表注释。我也将它们分别粘贴,或者您可以在Github页面上检查我的输出(顶端)。

Isn’t it great how we could get the gist of the entire document into concise and crisp phrases? These summaries will be used in topic modeling (in section 3) and the clustering of documents in section 4.
我们怎样才能使整个文档的要旨简明扼要,这不是很好吗? 这些摘要将用于主题建模(第3节)和第4节中的文档聚类。
翻译自: https://towardsdatascience.com/text-summarization-for-clustering-documents-2e074da6437a
mongdb 群集
本文来自互联网用户投稿,该文观点仅代表作者本人,不代表本站立场。本站仅提供信息存储空间服务,不拥有所有权,不承担相关法律责任。如若转载,请注明出处:http://www.mzph.cn/news/389499.shtml
如若内容造成侵权/违法违规/事实不符,请联系多彩编程网进行投诉反馈email:809451989@qq.com,一经查实,立即删除!