分布分析和分组分析
数据分析 (DATA ANALYSIS)
Being a regular at a restaurant is great.
乙 eing定期在餐厅是伟大的。
When I started university, my dad told me I should find a restaurant I really liked and eat there every month with some friends. Becoming a regular was supposed to be great because you got to build personal connections with the staff and create a ton of good memories over time.
当我上大学时,父亲告诉我应该找到我真正喜欢的餐厅,并每月和一些朋友在那儿吃饭。 成为普通人应该很棒,因为随着时间的推移,您必须与员工建立个人联系并创造大量美好的回忆。
But how much do regulars actually help businesses?
但是常客实际上对企业有多大帮助?
You might think that because the same people keep coming back to order, they would be the “customer group” that makes up a majority of the restaurant’s sales. However, this intuitive answer isn’t always the case. If you took a fast-food chain near a popular tourist attraction, it could be that the customer group of those who only ordered once contribute far more to sales than the locals who eat there every week.
您可能会认为,由于相同的人不断恢复订购,因此他们将成为构成餐厅销售额绝大部分的“客户群”。 但是,这种直观答案并非总是如此。 如果您在一个受欢迎的旅游景点附近搭了一条快餐连锁店,那可能是那些只订购一次的顾客群对销售的贡献远大于每周在那儿用餐的当地人。
To answer questions like these, we can turn to cohort analysis. This type of analytics focuses on breaking up the users or customers of a business (or service) into different groups. Members of a cohort will exhibit the same behavior in a specified time range. For example, customers who ordered again within a week after their first purchase could be the first cohort, then customers who ordered within two weeks would be the second cohort, and so on.
要回答此类问题,我们可以转向队列分析。 这种类型的分析重点在于将业务(或服务)的用户或客户分为不同的组。 同类群组成员在指定的时间范围内将表现出相同的行为。 例如,在第一次购买后一周内再次订购的客户可能是第一批,然后在两周内订购的客户将成为第二批,依此类推。
Cohort analysis can help you identify trends across a customer’s “life cycle” (the time over which they purchase your goods or use your service), so you can better understand how to serve each cohort. It’s one thing to know that you had 500 total users of your app in one month. It’s another to know that of those 500, only 12 were users of your app since its first launch. With that knowledge, then you could try to understand why those 12 kept using the app after its launch and try to improve your service based on those findings.
同类群组分析可以帮助您确定客户“生命周期”(他们购买商品或使用服务的时间)内的趋势,因此您可以更好地了解如何为每个同类群组提供服务。 知道您在一个月内拥有500个应用程序用户是一回事。 另一个要知道的是,自您的应用首次发布以来,在这500个应用中,只有12个是该应用的用户。 有了这些知识,您就可以尝试了解为什么这12个应用在启动后仍继续使用该应用,并根据这些发现尝试改善您的服务。
Now, let’s take a look at how you can perform this analysis with Tableau/
现在,让我们看一下如何使用Tableau /
Tableau中同类群组分析的四个步骤 (Four steps to cohort analysis in Tableau)
There is no one-click “cohort analysis” option in Tableau, but you can create such a visualization with just a few quick steps.
Tableau中没有一键式的“队列分析”选项,但是您只需几个简单的步骤就可以创建这种可视化。
If you want to follow along, we’re going to be working with the “Sample — Superstore” data set that comes with Tableau. The main question we’re going to answer is as follows:
如果您想继续学习,我们将使用Tableau随附的“样本-超级商店”数据集。 我们要回答的主要问题如下:
How much do longer-tenured customers impact total and annual profit of the business?
长期客户会在多大程度上影响企业的总利润和年利润?
Here, we’ll be creating cohorts of customers grouped by the first year they placed an order with the business. We’ll look at how much each cohort has contributed to total profit, as well as how much each cohort contributed to the profit in each year.
在这里,我们将创建按客户下订单的第一年分组的客户群。 我们将查看每个同类群组对总利润的贡献,以及每个同类群组每年对利润的贡献。
To do so, we’ll need to calculate the minimum order date for every customer. This might sound easy enough, as each order has a corresponding “Order Date”. However, to group customers by this calculated field, we’ll need to use a Level of Detail (LOD)
expression in Tableau. We’ll specifically be using a FIXED LOD
calculation so that we can give each customer its own “First Order Date” value.
为此,我们需要计算每个客户的最短订购日期。 这听起来很容易,因为每个订单都有一个对应的“订单日期”。 但是,要按此计算字段对客户进行分组,我们需要在Tableau中使用Level of Detail (LOD)
表达式。 我们将特别使用FIXED LOD
计算,以便为每个客户提供自己的“首次订购日期”值。
Here’s how we would get the “First Order Date” field:
这是我们如何获取“第一订单日期”字段的方法:
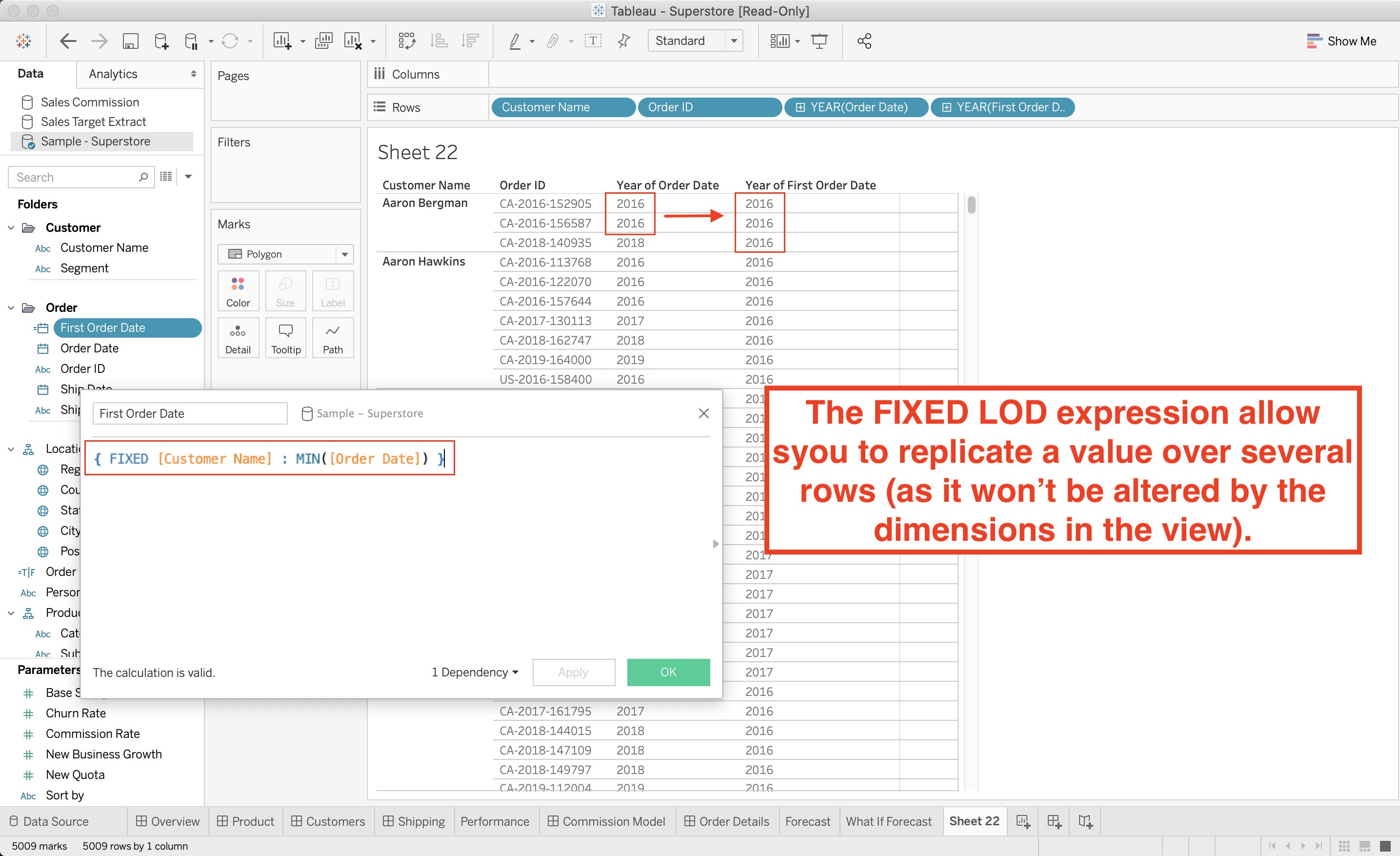
The calculated field is pretty simple:
计算字段非常简单:
{FIXED [Customer Name] : MIN([Order Date]) }
{FIXED [Customer Name] : MIN([Order Date]) }
Here, we’re looking for the minimum order date for every customer and “fixing” it. If we were to only write MIN([Order Date])
, we wouldn’t get the correct result when we create a graph without “Customer Name” in the field, as Tableau would then attempt to calculate the minimum order date for whatever other dimensions are in the field.
在这里,我们正在寻找每个客户的最小订购日期并“定下来”。 如果我们只写MIN([Order Date])
,则在我们创建的字段中没有“ Customer Name”的图形时,我们将无法获得正确的结果,因为Tableau随后将尝试计算其他任何商品的最小订购日期尺寸在字段中。
Now, to get our first graph with the total contribution of each customer cohort, there are just three steps:
现在,要获得包含每个客户群组的总贡献的第一张图,只需执行以下三个步骤:
Drag
Profit
andFirst Order Date
into the view (or just double-click them). These should change toSUM([Profit])
andYEAR(First Order Date)
.将
Profit
和First Order Date
拖到视图中(或双击它们)。 这些应更改为SUM([Profit])
和YEAR(First Order Date)
。- Click show me (or CTRL+Click/CMD+Click) and select the pie chart option. 单击“向我显示”(或CTRL +单击/ CMD +单击),然后选择饼图选项。
(Optional) resize the visualization to “Entire View”, add a
Percent of Total
calculation, and edit the “Label” to make the graph look prettier.(可选)将可视化文件的大小调整为“整个视图”,添加“
Percent of Total
计算,然后编辑“标签”以使图形看起来更漂亮。
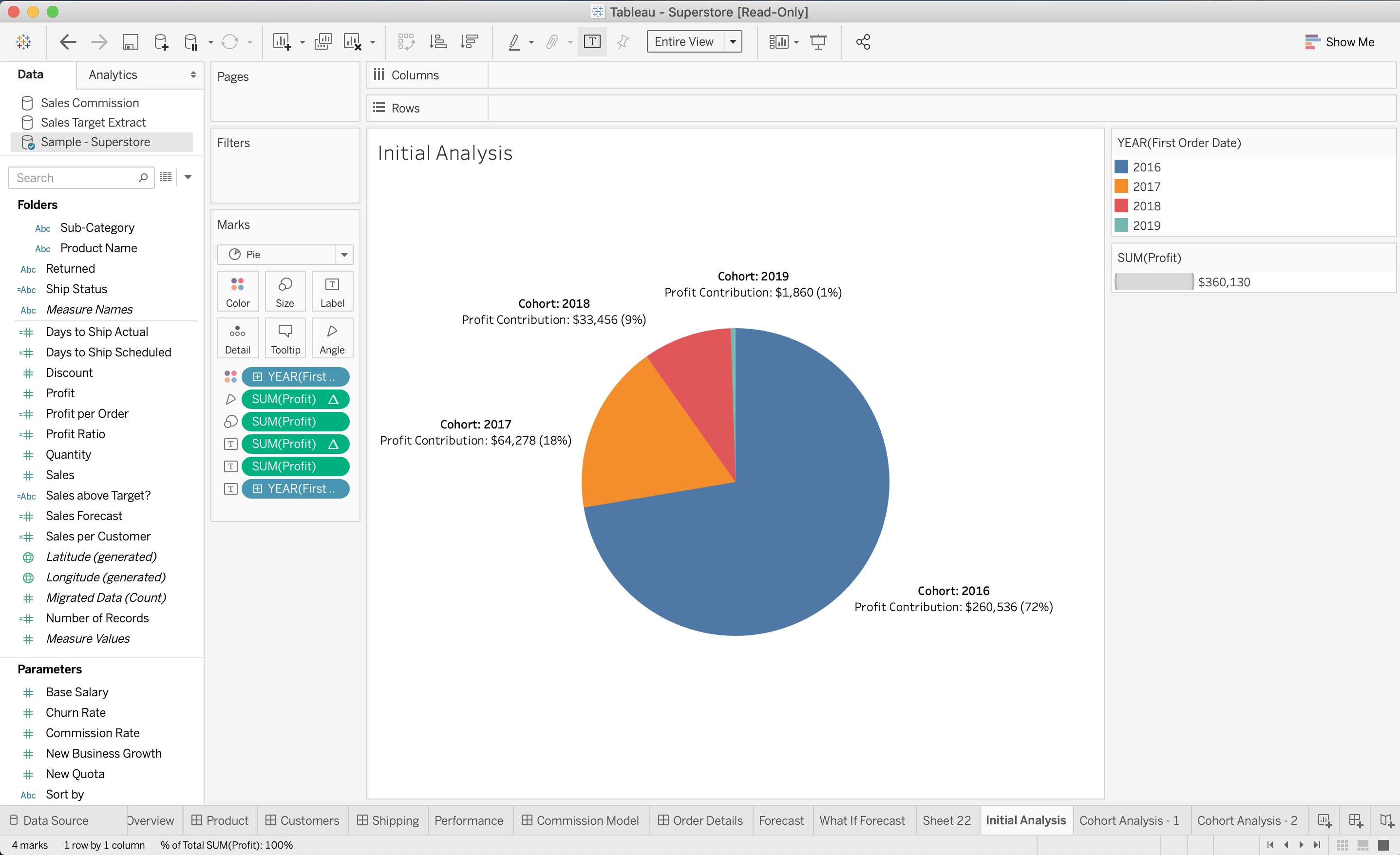
Ta-da!
-
Here, the sales are summed across all years the store has been in business. You can see that the majority of the profit came from the 2016 cohort, which is the first year the store was in business. It would be more interesting to see a chart with this data plotted across several years, so we could see if the 2016 cohort kept contributing a large percentage of yearly profit over time.
在这里,汇总了该商店营业的所有年份的销售额。 您可以看到,大部分利润来自2016年同期,这是该商店开业的第一年。 看到一个图表,用多年的数据绘制图表会更有趣,因此我们可以看到2016年同期是否随着时间的推移继续贡献了很大一部分年度利润。
To make our second graph with the yearly cohort profit contribution, we do the following steps:
为了使第二张图表具有年度同类群组利润贡献,我们执行以下步骤:
Drag
[Order Date]
to “Columns” and[Profit]
to “Rows”. These should change toYEAR(Order Date)
andSUM([Profit])
.将
[Order Date]
拖到“列”,将[Profit]
拖到“行”。 这些应更改为YEAR(Order Date)
和SUM([Profit])
。Drag
[First Ordet Date]
to the “Color” mark.将
[First Ordet Date]
“颜色”标记。- Click show me (or CTRL+Click/CMD+Click) and select the stacked bar chart option. 单击“向我显示”(或CTRL +单击/ CMD +单击),然后选择堆积的条形图选项。
(Optional) resize the visualization to “Entire View” and drag
[Profit]
to the “Label” mark.(可选)将可视化文件的大小调整为“整个视图”,然后将
[Profit]
拖动到“标签”标记。

Ta-da part 2!
Ta-da Part 2!
With this graph, we can see that over time a large amount of the yearly profit still comes from the 2016 cohort. This means that the customers who were with the business at the very beginning continue to purchase goods that give the business high profit, and we can even see that their absolute profit contribution increases every year (from $51,984 in 2016 to $91,600).
通过此图,我们可以看到,随着时间的流逝,大量的年度利润仍然来自2016年同期。 这意味着从一开始就与公司打交道的客户继续购买可为公司带来高利润的商品,我们甚至可以看到他们的绝对利润贡献每年都在增加(从2016年的51,984美元增加到91,600美元)。
It’s also interesting that the 2017 cohort’s profit contribution actually decreased from 2017 to 2018. Perhaps the business spent a lot of money to acquire new customers, which then decreased the profit gained from sales to the 2017 cohort.
有趣的是,2017年队列的利润贡献实际上从2017年减少到2018年。也许企业花了很多钱获得新客户,然后这减少了从2017年队列的销售获得的利润。
Another interesting visualization would be the same one as above, but with the yearly profit percentage contribution. To do so, follow these quick steps:
另一个有趣的可视化将与上面相同,但是具有年度利润百分比贡献。 为此,请按照以下快速步骤操作:
- Duplicate the previous graph 复制上一张图
Right-click on
SUM(Profit)
and you’ll see the option to add aQuick Table Calculation
. SelectPercent of Total
.右键单击
SUM(Profit)
,您将看到添加Quick Table Calculation
的选项。 选择Percent of Total
。Right-click on
SUM(Profit)
again, select “Compute Using” and set it to “Table (down)”.再次右键单击
SUM(Profit)
,选择“ Compute Using”,然后将其设置为“ Table(down)”。
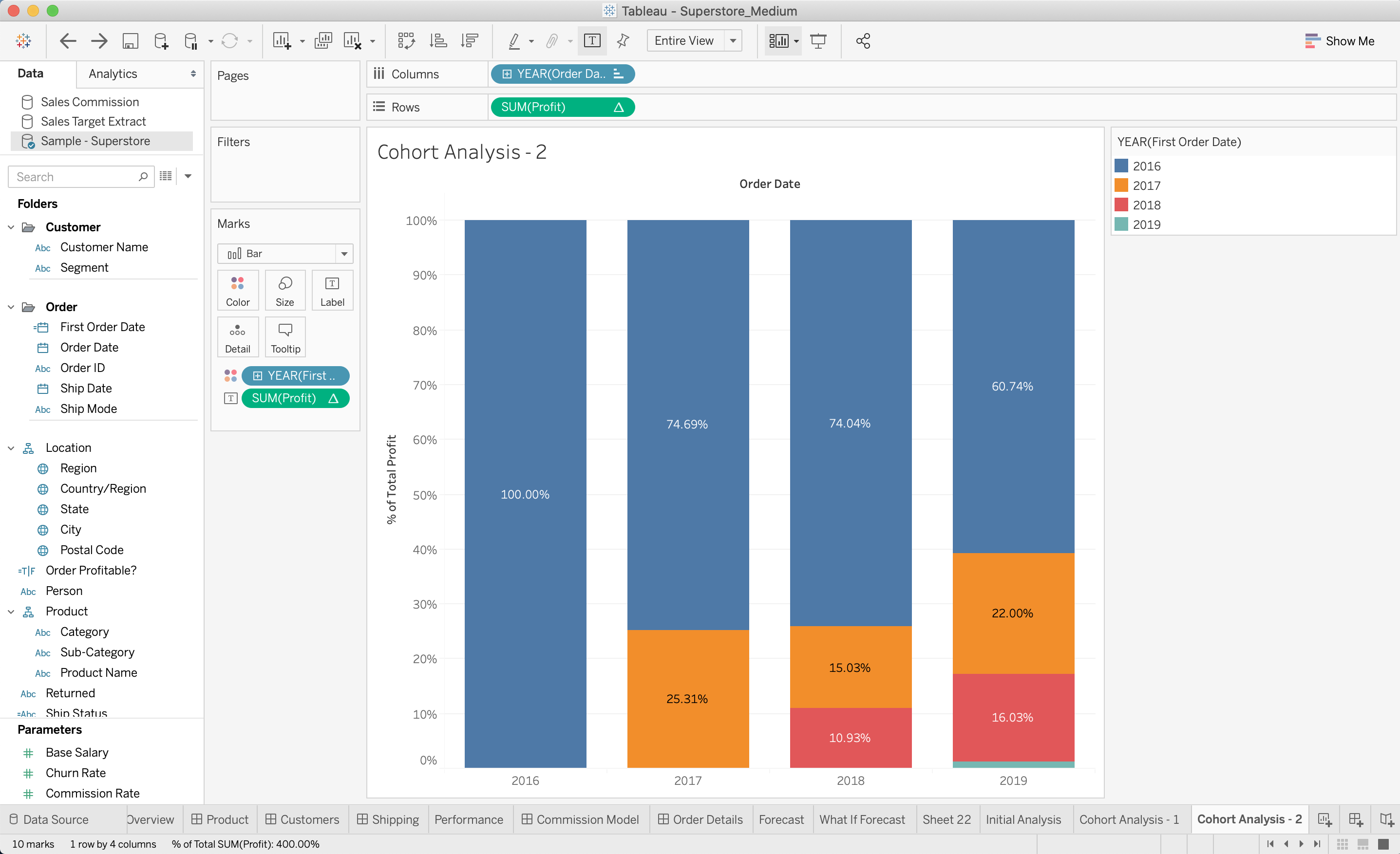
Ta-da part 3!
Ta-da第3部分!
With this graph, we same the same numbers as before, but this time with the Y-axis set to Percentage. We can clearly see that over time, the majority of the profit comes from the 2016 cohort. It would be helpful to investigate why this is happening by analyzing the 2016 cohort for features that distinguish them from the other three. Doing so could help the business either better tailor its offerings to other customer cohorts, or it could choose to simply focus only on the 2016 cohort and maximize its profit that way.
在此图表中,我们使用与以前相同的数字,但是这次Y轴设置为“百分比”。 我们可以清楚地看到,随着时间的流逝,大部分利润来自2016年同期。 通过分析2016年同类群组中有区别于其他三个类别的功能,有助于调查为什么会发生这种情况。 这样做可以帮助企业更好地为其他客户群体量身定制其产品,或者可以选择仅专注于2016年客户群体并以此最大化其利润。
I hope you found this quick introduction to conducting a cohort analysis with Tableau useful! You can do a lot more with Tableau than just create the basic graphs from “Show Me”, but that means understanding some of its more advanced features like LOD Expressions
. These and other skills can help you make sense of the data your business collects and drive well-informed decision making.
我希望您发现使用Tableau进行同类群组分析的快速入门对您有所帮助! 使用Tableau可以做更多的事情,而不仅仅是从“ Show Me”中创建基本图形,但这意味着了解其一些更高级的功能,例如LOD Expressions
。 这些技能和其他技能可以帮助您理解企业收集的数据并推动明智的决策。
If you’re looking to acquire a deeper understanding of Tableau, you may want to check out this comprehensive introduction to the Tableau Desktop Certifications.
如果您希望对Tableau有了更深入的了解,则可能需要查看有关Tableau Desktop认证的全面介绍 。
For a look at another type of analysis, you can do with Tableau, review this quick guide to forecasting.
要查看另一种类型的分析,可以使用Tableau,请查看此预测快速指南 。
Happy graphing.
快乐绘图。
翻译自: https://medium.com/swlh/how-to-group-your-users-and-get-actionable-insights-with-a-cohort-analysis-b2b281f82f33
分布分析和分组分析
本文来自互联网用户投稿,该文观点仅代表作者本人,不代表本站立场。本站仅提供信息存储空间服务,不拥有所有权,不承担相关法律责任。如若转载,请注明出处:http://www.mzph.cn/news/389224.shtml
如若内容造成侵权/违法违规/事实不符,请联系多彩编程网进行投诉反馈email:809451989@qq.com,一经查实,立即删除!