高斯模糊为什么叫高斯滤波
高斯分布及其主要特征: (Gaussian Distribution and its key characteristics:)
- Gaussian distribution is a continuous probability distribution with symmetrical sides around its center. 高斯分布是连续概率分布,其中心周围具有对称边。
- Its mean, median and mode are equal. 其均值,中位数和众数相等。
- Its shape looks like below with most of the data points clustered around the mean with asymptotic tails. 它的形状如下图所示,大多数数据点均以渐近尾部聚类在均值周围。
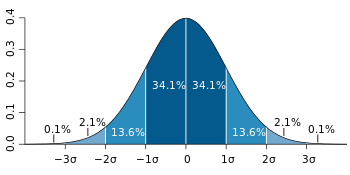
Interpretation:
解释:
- ~68% of the values drawn from normal distribution lie within 1𝜎 从正态分布得出的值的约68%位于1𝜎之内
- ~95% of the values drawn from normal distribution lie within 2𝜎 从正态分布得出的值的约95%位于2𝜎之内
- ~99.7% of the values drawn from normal distribution lie within 3𝜎 从正态分布得出的值的约99.7%位于3𝜎之内
我们在哪里找到高斯分布的存在? (Where do we find the existence of Gaussian distribution?)
ML practitioners or not, almost all of us have heard of this most popular form of distribution somewhere or the other. Everywhere we look around us, majority of the processes follow approximate Gaussian form, for e.g. age, height, IQ, memory, etc.
不管是否有ML从业者,我们几乎所有人都听说过这种最流行的发行形式。 我们环顾四周的任何地方,大多数过程都遵循近似的高斯形式,例如年龄,身高,智商,记忆力等。
On a lighter note, there is one well-known example of Gaussian lurking around all of us i.e. ‘bell curve’ during appraisal time 😊
轻松地说,有一个众所周知的例子,高斯潜伏在我们所有人周围,即在评估期间出现“钟形曲线”😊
Yes, Gaussian distribution resonates with bell curve quite often and its probability density function is represented by the following mathematical formula:
是的,高斯分布经常与钟形曲线产生共振,其概率密度函数由以下数学公式表示:
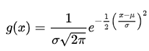
Notation:
符号:
A random variable X with mean 𝜇 and variance 𝜎² is denoted as:
具有均值𝜇和方差𝜎²的随机变量X表示为:

高斯分布有何特别之处? 为什么我们几乎到处都可以找到高斯? (What is so special about the Gaussian distribution? Why do we find Gaussian almost everywhere?)
Whenever we need to represent real valued random variables whose distribution is not known, we assume the Gaussian form.
每当我们需要表示其分布未知的实值随机变量时,我们都采用高斯形式。
This behavior is largely owed to Central Limit Theorem (CLT) which involves the study of sum of multiple random variables.
这种行为很大程度上归因于中央极限定理(CLT) ,该定理涉及多个随机变量之和的研究。
As per CLT: normalized sum of a number of random variables, regardless of which distribution they belong to originally, converges to Gaussian distribution as the number of terms in the summation increases.
根据CLT:许多随机变量的归一化总和,无论它们最初属于哪个分布,都随着总和中项数的增加而收敛到高斯分布 。
An important point to note is that CLT is valid at a sample size of 30 observations i.e. sampling distribution can be safely assumed to follow Gaussian form, if we have a minimum sample size of 30 observations.
需要注意的重要一点是,CLT在30个观测值的样本量下有效,即,如果我们的最小样本量为30个观测值,则可以安全地假定样本分布遵循高斯形式。
Therefore, any physical quantity that is sum of many independent processes is assumed to follow Gaussian. For e.g., “in a typical machine learning framework, there are multiple sources of errors possible — data entry error, data measurement error, classification error etc”. The cumulative effect of all such forms of error is likely to follow normal distribution”
因此,假定许多独立过程之和的任何物理量都遵循高斯。 例如,“在典型的机器学习框架中,可能有多种错误来源—数据输入错误,数据测量错误,分类错误等”。 所有这些形式的错误的累积影响很可能遵循正态分布。”
Let’s check this using python:
让我们使用python检查一下:
Steps:
脚步:
- Draw n samples from exponential distribution 从指数分布中抽取n个样本
- Normalize the sum of n samples 归一化n个样本的总和
- Repeat above steps N times 重复上述步骤N次
- Keep storing the normalized sum in sum_list 继续将归一化的和存储在sum_list中
- In the end, plot the histogram of the normalized sum_list 最后,绘制归一化的sum_list的直方图
- The output closely follows Gaussian distribution, as shown below: 输出紧密遵循高斯分布,如下所示:
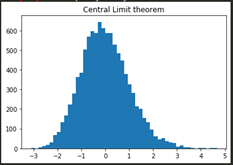
Similarly, there are several other distributions like Student t distribution, chi-squared distribution, F distribution etc which have strong dependence on the Gaussian distribution. For e.g. t-distribution is a result of infinite mixture of Gaussians leading to longer tails as compared to a Gaussian one.
同样,还有其他一些分布,例如学生t分布,卡方分布,F分布等,它们对高斯分布有很强的依赖性。 例如,t分布是高斯混合的无限结果,与高斯相比,它导致更长的尾巴。
Properties of Gaussian Distribution:
高斯分布的性质:
1) Affine transformation:
1) 仿射变换:
It is a simple transformation of multiplying the random variable with a scalar ‘a’ and adding another scalar ‘b’ to it.
这是将随机变量与标量“ a”相乘并向其添加另一个标量“ b”的简单转换。
The resulting distribution is Gaussian with mean:
结果分布为高斯,均值:
If X ~ N(𝜇, 𝜎²), then for any a,b ∈ ℝ,
如果X〜N(𝜇,𝜎²),那么对于任何a,b∈ℝ,
a.X+b ~ N(a. 𝜇+b, a².𝜎²)
a.X + b〜N(a。𝜇 + b,a².𝜎²)
Note that not all transformations result into Gaussian, for e.g. square of a Gaussian will not lead to Gaussian.
请注意,并非所有的变换都会产生高斯,例如,高斯的平方不会导致高斯。
2) Standardization:
2) 标准化:
If we have 2 sets of observations, each drawn from a normal distribution with different mean and sigma, then how do we compare the two observations to calculate the probabilities with respect to their population?
如果我们有两组观测值,每组观测值均来自具有不同均值和sigma的正态分布,那么我们如何比较这两个观测值以计算其总体的概率?
Hence, we need to convert the observations mentioned above into Z score. This process is called as Standardization which adjusts the raw observation with respect to its mean and sigma of the population it is generated from and brings it onto a common scale
因此,我们需要将上述观察值转换为Z分数。 此过程称为“标准化”,它根据原始观测值的平均值和总和来调整原始观测值,并将其放到一个通用范围内

3) Conditional distribution: An important property of multivariate Gaussian is that if two sets of variables are jointly Gaussian, then the conditional distribution of one set conditioned on the other set is again Gaussian
3) 条件分布:多元高斯的一个重要属性是,如果两组变量联合为高斯,则以另一组为条件的一组的条件分布又是高斯
4) Marginal distribution of the set is also a Gaussian
4)集合的边际分布也是高斯分布
5) Gaussian distributions are self-conjugate i.e. given the Gaussian likelihood function, choosing the Gaussian prior will result in Gaussian posterior.
5)高斯分布是自共轭的,即给定高斯似然函数,选择高斯先验将导致高斯后验。
6) Sum and difference of two independent Gaussian random variables is a Gaussian
6)两个独立的高斯随机变量的和与差是一个高斯
Limitations of Gaussian Distributions:
高斯分布的局限性:
- Simple Gaussian distribution fails to capture the below structure: 简单的高斯分布无法捕获以下结构:
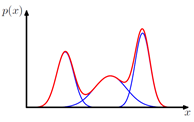
Such structure is better characterized by the linear combination of two Gaussians (also known as mixture of Gaussians). However, it's complex to estimate the parameters of such mixture of Gaussians.
通过两个高斯线性组合(也称为高斯混合)可以更好地描述这种结构。 但是,估计这种高斯混合参数很复杂。
2) Gaussian distribution is uni-modal, i.e. it fails to provide a good approximation to multi-modal distributions thereby restricting the range of distributions that it can represent adequately.
2)高斯分布是单峰分布,即它不能很好地近似多峰分布,从而限制了它可以充分表示的分布范围。
3) Degrees of freedom grow quadratically with an increase in the number of dimensions. This results in high computational complexity in inverting such large covariance matrix.
3)自由度随着尺寸数量的增加而平方增长。 在反转这样大的协方差矩阵时,这导致很高的计算复杂度。
Hope the post gives you a sneak peek into the world of Gaussian distributions.
希望这篇文章能使您对高斯分布的世界有所了解。
Happy Reading!!!
阅读愉快!
翻译自: https://towardsdatascience.com/why-is-gaussian-the-king-of-all-distributions-c45e0fe8a6e5
高斯模糊为什么叫高斯滤波
本文来自互联网用户投稿,该文观点仅代表作者本人,不代表本站立场。本站仅提供信息存储空间服务,不拥有所有权,不承担相关法律责任。如若转载,请注明出处:http://www.mzph.cn/news/388881.shtml
如若内容造成侵权/违法违规/事实不符,请联系多彩编程网进行投诉反馈email:809451989@qq.com,一经查实,立即删除!