python3中朴素贝叶斯
你在这里 (You are here)
If you’re reading this, odds are: (1) you’re interested in bayesian statistics but (2) you have no idea how Markov Chain Monte Carlo (MCMC) sampling methods work, and (3) you realize that all but the simplest, toy problems require MCMC sampling so you’re a bit unsure of how to move forward.
如果您正在阅读此书,则可能是:(1)您对贝叶斯统计感兴趣,但(2)您不知道马尔可夫链蒙特卡洛(MCMC)采样方法的工作原理,以及(3)您意识到,除了最简单的玩具问题需要MCMC采样,因此您不确定如何前进。
Not to worry, we’ll explore this tricky concept using a 1-dimensional normal distribution, using nothing more than python, user-defined functions, and the random module (specifically, the uniform distribution.) We’ll discuss all this nonsense in terms of bars, beers, and a night out with your pals.
不用担心,我们将使用一维正态分布探索这个棘手的概念,仅使用python,用户定义函数和随机模块(特别是统一分布)。我们将在下面讨论所有这些废话。酒吧,啤酒和与朋友共度夜晚的条件
在酒吧想象自己 (Picture yourself at a bar)
It’s Friday night, you and your gang head out for burgers, beer and to catch a televised baseball game. Let’s say you arbitrarily pick a first bar (we’ll call it Larry’s Sports Bar), you sit down, grab a menu and consider your options. If the food and drinks are affordable, that’s a good reason to stay; but if there’s standing room only, you’ve got a reason to leave. If the bar’s filled with big screen TVs all dialed into the right game, another reason to stay. If the food or drinks aren’t appealing, you’ve got another reason to leave. Etc etc, you get the point.
这是星期五晚上,您和帮派前往汉堡,啤酒和观看电视转播的棒球比赛。 假设您随意选择第一个酒吧(我们称其为Larry's Sports Bar ),您坐下来,拿起一个菜单并考虑您的选择。 如果食物和饮料负担得起,那就是留下的充分理由。 但是如果只有客厅,那您就有理由离开。 如果酒吧的大屏幕电视都播放了正确的游戏,这是留下的另一个原因。 如果食物或饮料不受欢迎,您还有其他理由离开。 等等,您明白了。
Now, let’s say one (or several) of your friends have gripes with the current establishment — the food is cold, the beers are overpriced, whatever the reason. So he proposes the gang leave Larry’s Sports Bar in favor of Tony’s Pizzeria and Beer Garden because the food there is better, fresher, etc. So the gang deliberates, asking the questions (A) how favorable is Larry’s? (B) How favorable is Tony’s? And (C) how favorable is Tony’s relative to Larry’s?
现在,比方说,您的一个(或几个)朋友对当前的餐馆感到不快-不管是什么原因,食物都很冷,啤酒价格过高。 因此,他建议该团伙离开拉里的体育馆 ,转而使用托尼的比萨店和啤酒花园,因为那里的食物更好,更新鲜,等等。于是,该团伙在考虑以下问题:(A)拉里一家有多有利? (B)托尼的优惠程度如何? (C) 托尼相对于拉里有多有利?
This relative comparison is really the most important detail. If Tony’s is only marginally better than Larry’s (or far worse) there’s a good chance that it’s not worth the effort to relocate. But if Tony’s is unambiguously the better of the two, there’s only a slim chance that you might stay. This is the real juice that makes Metropolis-Hastings “work.”
相对比较确实是最重要的细节。 如果Tony的房屋仅比Larry的房屋略好(或更差),则很有可能不值得重新安置。 但是,如果托尼(Tony's)无疑是两者中的佼佼者,那么您留下的可能性很小。 这是使Metropolis-Hastings“工作”的真正汁液 。
算法 (The algorithm)
Metropolis-Hastings algorithm does:
Metropolis-Hastings算法可以:
Start with a random sample
从随机样本开始
Determine the probability density associated with the sample
确定与样本相关的概率密度
Propose a new, arbitrary sample (and determine its probability density)
提出一个新的任意样本(并确定其概率密度)
Compare densities (via division), quantifying the desire to move
比较密度(通过除法),量化移动的欲望
Generate a random number, compare with desire to move, and decide: move or stay
生成一个随机数,与移动的欲望进行比较,并决定:移动还是停留
- Repeat 重复
The real key is (as we’ve discussed) quantifying how desirable the move is as an action/inaction criteria, then (new stuff alert!) observe a random event, compare to said threshold, and make a decision.
真正的关键是(正如我们已经讨论过的那样)量化移动作为行动/不作为标准的期望程度,然后(新事物警报!) 观察随机事件 ,与所述阈值进行比较,然后做出决定。
随机事件 (The random event)
For our purposes, our threshold is the ratio of the proposed sample’s probability density to the current sample’s probability density. If this threshold is near (or above) 1, it means that the previous location was highly undesirable (a number close to 0, very improbable) and the proposed location is highly desirable (as close to 1 as is possible, near distribution’s expectation). Now, we need to generate a number in the range [0,1] from the uniform distribution. If the number produced is less than or equal to the threshold, we move. Otherwise, we stay. That’s it!
出于我们的目的,我们的阈值是建议样本的概率密度与当前样本的概率密度之比。 如果此阈值接近(或高于)1,则意味着先前的位置非常不理想(数字接近0,非常不可能),建议的位置也非常理想(尽可能接近1,接近分布的期望值) 。 现在,我们需要根据均匀分布生成一个在[0,1]范围内的数字。 如果产生的数字小于或等于阈值,则移动。 否则,我们留下。 而已!
那硬东西呢? (What about the hard stuff?)
This is starting to sound a little too good to be true, right? We haven’t discussed Markov Chains or Monte Carlo simulations yet but fret not. Both are huge topics in their own right and we only need the most basic familiarity with each to make use of MCMC magic.
这听起来似乎太好了,难以置信,对吧? 我们尚未讨论马尔可夫链或蒙特卡洛模拟,但不用担心。 两者本身都是很重要的话题,我们只需要对它们有最基本的了解就可以使用MCMC魔术。
A Markov Chain is is a chain of discrete events where the probability of the next event is conditioned only upon the current event. (ex: I just finished studying, do I go to sleep or go to the bar? These are my only choices of a finite set.) In this system of discrete choices, there exists a transition matrix, which quantifies the probability of transitioning from any given state to any given state. A Monte Carlo method is really just a fancy name for a simulation/experiment that relies on usage of random numbers.
马尔可夫链是离散事件的链,其中下一个事件的概率仅取决于当前事件。 (例如:我刚刚完成学习,我要去睡觉还是去酒吧? 这是我对有限集合的唯一选择。 )在这个离散选择的系统中,存在一个转换矩阵,该矩阵量化从任何给定状态到任何给定状态。 蒙特卡洛方法实际上只是依赖于随机数使用的模拟/实验的奇特名称。
As discussed, we’ll be sampling from the normal distribution — a continuous, not discrete, distribution. So how can there be a transition matrix? Surprise!— there’s no transition matrix at all. (It’s actually called a Markov kernel, and it’s just the comparison of probability densities, at least for our purposes.)
正如讨论的那样,我们将从正态分布中进行采样-连续而不是离散的分布。 那么如何有一个过渡矩阵呢? 惊喜! —根本没有过渡矩阵。 (实际上,它被称为Markov核 ,它只是概率密度的比较,至少出于我们的目的。)
代码 (The code)
below, we define three functions: (1) Normal, which evaluates the probability density of any observation given the parameters mu and sigma. (2) Random_coin, which references a fellow TDS writer’s post (lined below). And (3) Gaussian_mcmc, which samples executes the algorithm as described above.
下面,我们定义三个函数:(1)正态,在给定参数mu和sigma的情况下评估任何观测值的概率密度。 (2)Random_coin,它引用了TDS同行作家的帖子(如下所示)。 以及(3)采样的高斯_mcmc执行上述算法。
As promised, we’re not calling any Gaussian or normal function from numpy, scipy, etc. In the third function, we initialize a current sample as an instance of the uniform distribution (where the lower and upper boundaries are +/- 5 standard deviations from the mean.) Likewise, movement is defined in the same way. Lastly, we move (or stay) based on the random event’s observed value in relation to acceptance, which is the probability density comparison discussed at length elsewhere.
如所承诺的,我们不会从numpy,scipy等中调用任何高斯函数或正态函数。在第三个函数中,我们将当前样本初始化为均匀分布的实例(上下边界为+/- 5标准同样,以相同的方式定义运动。 最后,我们根据与接受相关的随机事件的观察值来移动(或停留),这是在其他地方详细讨论的概率密度比较。
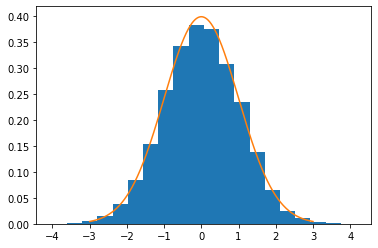
Lastly, one must always give credit where credit is due: Rahul Agarwal’s post defining a Beta distribution MH sampler was instrumental to my development of the above Gaussian distribution MH sampler. I encourage you to read his post as well for a more detailed exploration of the foundational concepts, namely Markov Chains and Monte Carlo simulations.
最后,必须始终在应归功的地方给予信誉: Rahul Agarwal的职位定义了Beta分布MH采样器,这对我开发上述高斯分布MH采样器至关重要。 我鼓励您也阅读他的文章,以更详细地探索基本概念,即马尔可夫链和蒙特卡洛模拟。
Thank you for reading — If you think my content is alright, please subscribe! :)
感谢您的阅读-如果您认为我的内容还可以,请订阅! :)
翻译自: https://towardsdatascience.com/bayesian-statistics-metropolis-hastings-from-scratch-in-python-c3b10cc4382d
python3中朴素贝叶斯
本文来自互联网用户投稿,该文观点仅代表作者本人,不代表本站立场。本站仅提供信息存储空间服务,不拥有所有权,不承担相关法律责任。如若转载,请注明出处:http://www.mzph.cn/news/388801.shtml
如若内容造成侵权/违法违规/事实不符,请联系多彩编程网进行投诉反馈email:809451989@qq.com,一经查实,立即删除!