数据治理 主数据 元数据
Data governance is top of mind for many of my customers, particularly in light of GDPR, CCPA, COVID-19, and any number of other acronyms that speak to the increasing importance of data management when it comes to protecting user data.
数据治理是我许多客户的首要考虑因素,尤其是考虑到GDPR,CCPA,COVID-19以及任何其他首字母缩写词,这些首字母缩写词表明了数据管理在保护用户数据方面的重要性日益提高。
Over the past several years, data catalogs have emerged as a powerful tool for data governance, and I couldn’t be happier. As companies digitize and their data operations democratize, it’s important for all elements of the data stack, from warehouses to business intelligence platforms, and now, catalogs, to participate in compliance best practices.
在过去的几年中, 数据目录已成为一种强大的数据治理工具 ,我对此感到高兴。 随着公司数字化及其数据运营的民主化,从仓库到商业智能平台,再到现在的目录,数据堆栈的所有元素都必须参与合规性最佳实践。
But are data catalogs all we need to build a robust data governance program?
但是,构建强大的数据治理程序所需的所有数据目录都是吗?
数据目录用于数据治理? (Data catalogs for data governance?)
Analogous to a physical library catalog, data catalogs serve as an inventory of metadata and give investors the information necessary to evaluate data accessibility, health, and location. Companies like Alation, Collibra, and Informatica tout solutions that not only keep tabs on your data, but also integrate with machine learning and automation to make data more discoverable, collaborative, and now, in compliance with organizational, industry-wide, or even government regulations.
类似于物理图书馆目录, 数据目录用作元数据清单,并向投资者提供评估数据可访问性,健康状况和位置所需的信息。 像Alation,Collibra和Informatica这样的公司都在宣传解决方案,这些解决方案不仅可以保留数据标签,还可以与机器学习和自动化集成,从而使数据更易于发现,协作,并且现在符合组织,整个行业甚至政府的要求。规定。
Since data catalogs provide a single source of truth about a company’s data sources, it’s very easy to leverage data catalogs to manage the data in your pipelines. Data catalogs can be used to store metadata that gives stakeholders a better understanding of a specific source’s lineage, thereby instilling greater trust in the data itself. Additionally, data catalogs make it easy to keep track of where personally identifiable information (PII) can both be housed and sprawl downstream, as well as who in the organization has the permission to access it across the pipeline.
由于数据目录提供有关公司数据源的唯一事实来源,因此利用数据目录来管理管道中的数据非常容易。 数据目录可用于存储元数据,从而使利益相关者更好地了解特定来源的血统,从而在数据本身上建立起更大的信任。 此外,数据目录使跟踪个人身份信息(PII)可以存放和向下游蔓延的位置以及组织中的谁有权通过管道访问变得容易。
什么适合我的组织? (What’s right for my organization?)
So, what type of data catalog makes the most sense for your organization? To make your life a little easier, I spoke with data teams in the field to learn about their data catalog solutions, breaking them down into three distinct categories: in-house, third-party, and open source.
那么,哪种类型的数据目录最适合您的组织? 为了使您的生活更轻松,我与该领域的数据团队进行了交谈,以了解他们的数据目录解决方案,并将它们分为三个不同的类别:内部,第三方和开源。
内部的 (In-house)
Some B2C companies — I’m talking the Airbnbs, Netflixs, and Ubers of the world — build their own data catalogs to ensure data compliance with state, country, and even economic union (I’m looking at you GDPR) level regulations. The biggest perk of in-house solutions is the ability to quickly spin up customizable dashboards, pulling out fields your team needs the most.
一些B2C公司(我正在谈论全球的Airbnbs , Netflix和Uber)建立自己的数据目录,以确保数据符合州,国家或经济联盟(我在看您的GDPR)级法规。 内部解决方案最大的好处是能够快速启动可定制的仪表板,从而拉出团队最需要的领域。
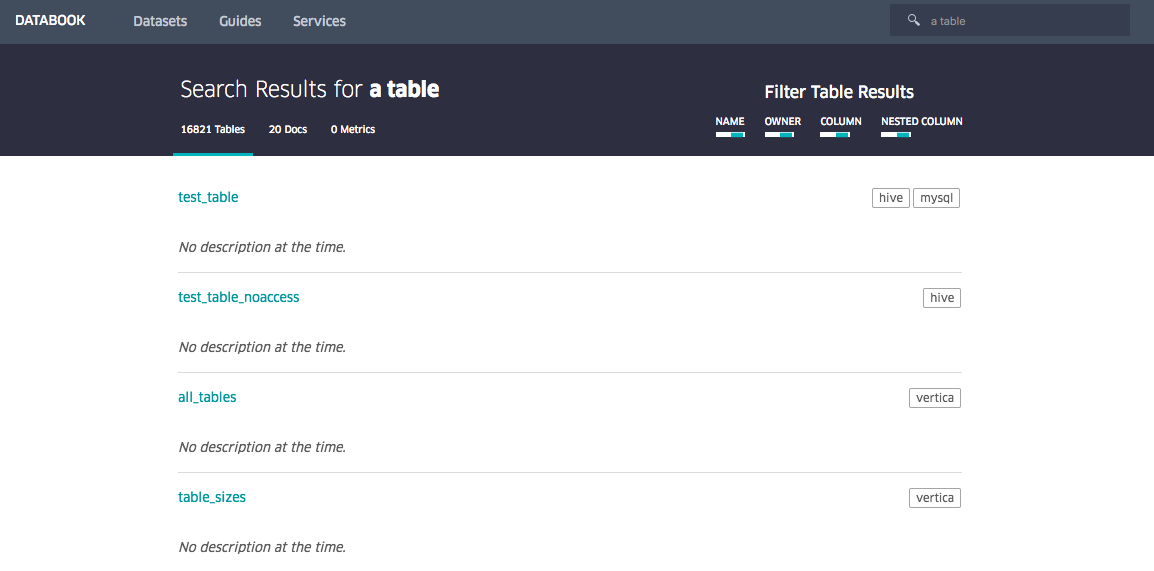
While in-house tools make for quick customization, over time, such hacks can lead to a lack of visibility and collaboration, particularly when it comes to understanding data lineage. In fact, one data leader I spoke with at a food delivery startup noted that what was clearly missing from her in-house data catalog was a “single pane of glass.” If she had a single source of truth that could provide insight into how her team’s tables were being leveraged by other parts of the business, ensuring compliance would be easy.
尽管内部工具可以快速进行自定义,但随着时间的流逝,此类黑客行为可能导致缺乏可见性和协作性,尤其是在了解数据沿袭时。 实际上,我在一家食品配送初创公司与之交谈的一位数据负责人指出,她内部数据目录中显然缺少的是“一块玻璃”。 如果她有一个真实的来源,可以洞察业务的其他部门如何利用她的团队的表,那么确保合规将很容易。
On top of these tactical considerations, spending engineering time and resources building a multi-million dollar data catalog just doesn’t make sense for the vast majority of companies.
除了这些战术上的考虑之外,花费大量的工程时间和资源来建立数百万美元的数据目录对于绝大多数公司来说都是没有意义的。
第三方 (Third-party)
Since their founding in 2012, Alation has largely paved the way for the rise of the automated data catalog. Now, there are a whole host of ML-powered data catalogs on the market, including Collibra, Informatica, and others, many with pay-for-play workflow and repository-oriented compliance management integrations. Some cloud providers, like Google, AWS, and Azure, also offer data governance tooling integration at an additional cost.
自2012年成立以来, Alation在很大程度上为自动化数据目录的兴起铺平了道路。 现在,市场上有大量基于ML的数据目录,包括Collibra , Informatica等,其中许多具有按需付费工作流程和面向存储库的合规性管理集成。 一些云提供商,例如Google,AWS和Azure,还提供了额外的数据治理工具集成。
In my conversations with data leaders, one downside of these solutions came up time and again: usability. While nearly all of these tools have strong collaboration features, one Data Engineering VP I spoke with specifically called out his third-party catalog’s unintuitive UI.
在与数据负责人的对话中,这些解决方案的一个缺点一次又一次出现:可用性。 尽管几乎所有这些工具都具有强大的协作功能,但与我交谈的一位数据工程副总裁特别提到了他的第三方目录的直观用户界面。
If data tools aren’t easy to use, how can we expect users to understand or even care whether they’re compliant?
如果数据工具不容易使用,我们如何期望用户理解甚至关心他们是否合规?
开源的 (Open source)
In 2017, Lyft became an industry leader by open sourcing their data discovery and metadata engine, Amundsen, named after the famed Antarctic explorer. Other open source tools, such as Apache Atlas, Magda and CKAN, provide similar functionalities, and all three make it easy for development-savvy teams to fork an instance of the software and get started.
2017年,Lyft通过开源其数据发现和元数据引擎Amundsen成为行业领导者, Amundsen以著名的南极探险家的名字命名。 其他开放源代码工具(例如Apache Atlas , Magda和CKAN )提供了相似的功能,而这三者使精通开发的团队可以轻松地派生该软件的实例并开始使用。
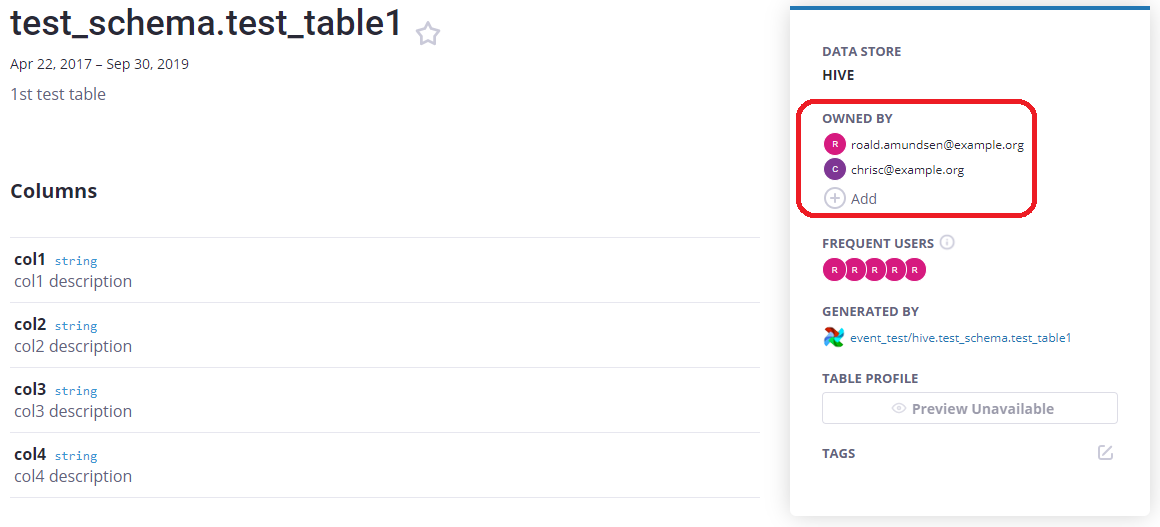
While some of these tools allow teams to tag metadata within to control user access, this is an intensive and often manual process that most teams just don’t have the time to tackle. In fact, a product manager at a leading transportation company shared that his team specifically chose not to use an open source data catalog because they didn’t have off-the-shelf support for all the data sources and data management tooling in their stack, making data governance extra challenging. In short, open source solutions just weren’t comprehensive enough.
尽管其中一些工具允许团队在其中标记元数据来控制用户访问,但这是一个密集且通常是手动的过程,大多数团队只是没有时间解决。 实际上,一家领先的运输公司的产品经理分享说,他的团队特别选择不使用开源数据目录,因为他们没有对堆栈中所有数据源和数据管理工具的现成支持,使数据治理更具挑战性。 简而言之,开源解决方案还不够全面。
Still, there’s something critical to compliance that even the most advanced catalog can’t account for: data downtime.
尽管如此,即使对于最高级的目录,也无法解决合规性方面的关键问题: 数据停机 。
缺少的链接:数据停机 (The missing link: data downtime)
Recently, I developed a simple metric for a customer that helps measure data downtime, in other words, periods of time when your data is partial, erroneous, missing, or otherwise inaccurate. When applied to data governance, data downtime gives you a holistic picture of your organization’s data reliability. Without data reliability to power full discoverability, it’s impossible to know whether or not your data is fully compliant and usable.
最近,我为客户开发了一个简单的指标 ,该指标可以帮助您衡量数据停机时间 ,换句话说,就是您的数据不完整,错误,丢失或不准确时的时间段。 当应用于数据治理时,数据停机时间可以使您全面了解组织的数据可靠性。 没有数据可靠性来增强完全可发现性,就无法知道您的数据是否完全合规和可用。
Data catalogs solve some, but not all, of your data governance problems. To start, mitigating governance gaps is a monumental undertaking, and it’s impossible to prioritize these without a full understanding of which data assets are actually being accessed by your company. Data reliability fills this gap and allows you to unlock your data ecosystem’s full potential.
数据目录解决了部分但不是全部的数据治理问题。 首先,减轻治理差距是一项艰巨的任务,如果无法完全了解贵公司实际上正在访问哪些数据资产,就不可能对这些差距进行优先排序。 数据可靠性填补了这一空白,并允许您释放数据生态系统的全部潜力。
Additionally, without real-time lineage, it’s impossible to know how PII or other regulated data sprawls. Think about it for a second: even if you’re using the fanciest data catalog on the market, your governance is only as good as your knowledge about where that data goes. If your pipelines aren’t reliable, neither is your data catalog.
此外,如果没有实时沿袭,就不可能知道PII或其他受监管的数据是如何蔓延的。 仔细考虑一下:即使您使用的是市场上最高级的数据目录,您的治理也仅取决于您对数据去向的了解。 如果管道不可靠,那么数据目录也不可靠。
Owing to their complementary features, data catalogs and data reliability solutions work hand-in-hand to provide an engineering approach to data governance, no matter the acronyms you need to meet.
由于具有互补功能,因此数据目录和数据可靠性解决方案可以协同工作,从而为数据治理提供一种工程方法,无论您需要使用首字母缩写词如何。
Personally, I’m excited for what the next wave of data catalogs have in store. And trust me: it’s more than just data.
就个人而言,我对下一波数据目录的存储感到兴奋。 相信我:这不仅仅是数据。
If you want to learn more, reach out to Barr Moses.
如果您想了解更多信息,请联系 Barr Moses 。
翻译自: https://towardsdatascience.com/what-we-got-wrong-about-data-governance-365555993048
数据治理 主数据 元数据
本文来自互联网用户投稿,该文观点仅代表作者本人,不代表本站立场。本站仅提供信息存储空间服务,不拥有所有权,不承担相关法律责任。如若转载,请注明出处:http://www.mzph.cn/news/388796.shtml
如若内容造成侵权/违法违规/事实不符,请联系多彩编程网进行投诉反馈email:809451989@qq.com,一经查实,立即删除!