最佳子集aic选择
As there is a lot of buzz about AutoML, I decided to write about the original AutoML; step-wise regression and best subset selection. Then I decided to ignore step-wise regression because it is bad and should probably stop being taught. That leaves best subset selection to discuss.
由于有关AutoML的话题很多,因此我决定写有关原始AutoML的文章。 逐步回归和最佳子集选择。 然后我决定忽略逐步回归,因为它很糟糕 ,应该停止讲授。 剩下的最佳子集选择需要讨论。
The idea behind best subset selection is choose the “best” subset of variables to include in a model, looking at groups of variables together as opposed to step-wise regression which compares them one at a time. We determine which set of variables are “best” by assessing which sub-model fits the data best while penalizing for the number of independent variables in the model to avoid over-fitting. There are multiple metrics for assessing how well a model fits: adjusted 𝑅-squared, the Akaike Information Criterion (AIC), the Bayesian Information Criterion (BIC), and Mallow’s 𝐶𝑝 are probably the best known.
最佳子集选择的思想是选择要包括在模型中的变量的“最佳”子集,将变量组放在一起查看,而不是逐步比较一次将它们进行比较的逐步回归。 我们通过评估哪个子模型最适合数据,同时对模型中自变量的数量进行惩罚以避免过度拟合,从而确定哪个变量组“最佳”。 有多种评估模型拟合程度的指标:调整后的𝑅平方,Akaike信息准则(AIC),贝叶斯信息准则(BIC)和Mallow's𝐶𝑝 是最有名的。
The formulas for each are below.
每个公式如下。
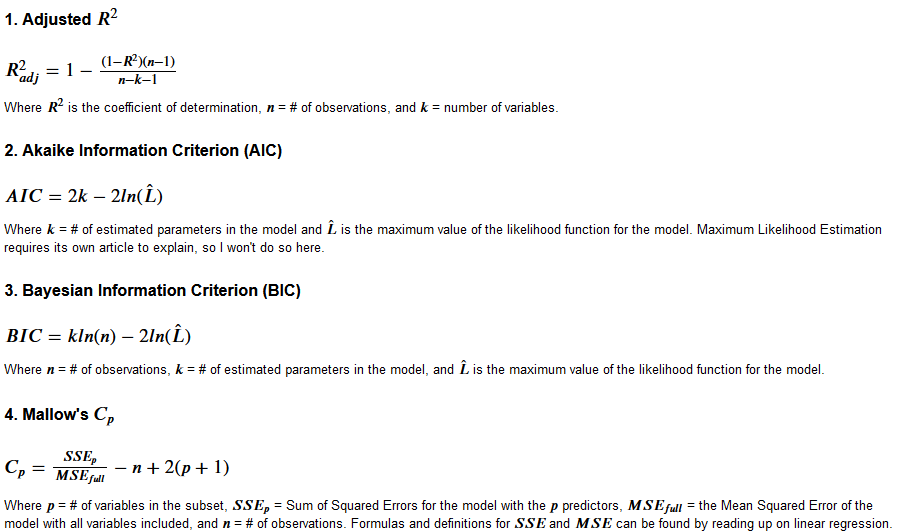
With Adjusted R-squared, you want to find the model with the largest Adjusted R-squared because it explains the most variance in the dependent variable, penalized for model complexity. For the others, you want to find the model with the smallest Information Criterion because it is the model with the least unexplained variance in the dependent variable, penalizing for model complexity. They’re the same idea, i.e. maximizing something good versus minimizing something bad.
使用“已调整R平方”时,您要查找“已调整R平方”最大的模型,因为它解释了因变量中最大的方差,并因模型复杂性而受到惩罚。 对于其他模型,您希望找到信息准则最小的模型,因为它是因变量中无法解释的方差最小的模型,因此对模型的复杂性不利。 他们是同一主意,即最大化好事物与最小化坏事物。
Both the AIC and Mallow’s 𝐶𝑝 tend to give better predictive models, while BIC tends to give models with fewer independent variables because it penalizes complex models more than the other two.
AIC和Mallow的𝐶𝑝 BIC倾向于提供更好的预测模型,而BIC倾向于提供具有更少自变量的模型,因为它比其他两个模型对复杂模型的惩罚更大。
Like most things in life, automating model selection comes at a cost. If you use your data to select a linear model, the coefficients of the selected variables will be biased away from zero! The null hypotheses of both the individual t-tests for each coefficient and the F-test for overall model significance are based on the assumption that each coefficient is normally distributed with mean 0. Since we have introduced bias into our coefficients, the Type I error level increases for these tests! This may not be an issue if you just need a predictive model, but it completely invalidates any statistical inferences made with the selected model. AutoML may be able to generate decent predictive models, but inference still requires a person to think carefully about the problem and follow the scientific method.
就像生活中的大多数事情一样,自动选择模型是有代价的。 如果您使用的数据来选择线性模型,所选择的变量的系数将从0 偏向了! 每个系数的单独t检验和整体模型显着性的F检验的零假设均基于以下假设:每个系数的正态分布均值为0。由于我们在系数中引入了偏差,因此I型错误这些测试的水平提高了! 如果您只需要预测模型,这可能就不成问题了,但是这完全会使使用所选模型做出的任何统计推断无效。 AutoML可能能够生成不错的预测模型,但是推理仍然需要人们仔细考虑问题并遵循科学方法。
展示最佳子集选择的偏见 (Demonstrating the Bias of Best Subset Selection)
I performed a simulation study to demonstrate the bias caused by best subset selection. Instead of looking at the bias in the coefficients, we will look at the bias in the estimated standard deviation of the error term in the model
我进行了仿真研究,以证明最佳子集选择所引起的偏差。 除了查看系数中的偏差之外,我们还将查看模型中误差项的估计标准偏差中的偏差。

where the error terms are identically and independently distributed 𝑁(0,𝜎) random variables.
其中误差项是相同且独立分布的𝑁(0,𝜎)随机变量。
At each round of the simulation, a sample of 100 observations are generated from the same distribution. The true model, which contains only the truly significant variables, as well as the best subset models selected by AIC and BIC are also estimated. From each model, I estimate the 𝜎 of the error term using the formula
在模拟的每一轮中,从同一分布生成100个观测值的样本。 还估计仅包含真正重要变量的真实模型,以及由AIC和BIC选择的最佳子集模型。 从每一个模型,我估计σ 使用公式计算误差项

This is performed 500 times.
这执行了500次。
The particular parameters of my simulation are as follows: 𝑛 = 100, # of independent variables = 6, 𝜎 = 1, and the number of significant independent variables is 2. The intercept is significant as well, so 3 coefficients are non-zero. The non-zero coefficients are selected using 𝑁(5,1) random numbers because I am too lazy to define fixed numbers, but they remain fixed for all rounds of the simulation.
我的模拟的特定参数如下: 𝑛 = 100,自变量= 6,σ= 1,和显著独立变量的数目的#是2。截距是显著为好,这样的3个系数为非零。 非零系数是使用𝑁(5,1)随机数选择的,因为我懒于定义固定数,但是在所有模拟回合中它们都保持固定。
I first defined my own function to perform best subset selection using AIC or BIC using a naive approach by looking at every combination of variables. It only works for a small number of variables because the number of models it has to consider blows up as the number of variables increases. The number of models considered is
我首先定义了自己的函数,以天真的方法通过查看变量的每种组合来使用AIC或BIC来执行最佳子集选择。 它仅适用于少量变量,因为随着变量数量的增加,必须考虑的模型数量会激增。 考虑的模型数量是

but smarter implementations of best subset selection use a tree search to reduce the number of models considered.
但是最佳子集选择的更智能实现使用树搜索来减少所考虑模型的数量。
The graphs of interest are below these chunks of code for the best subset selection function and for the simulation.
感兴趣的图在这些代码块的下方,用于最佳子集选择功能和仿真。
The red line is the line where the y-axis equals the x-axis, which is the unbiased estimate of 𝜎 from the true model. As you can see in the plots below, the estimates of 𝜎 are biased from the models selected by best AIC and BIC. In fact they will always be less than or equal to the unbiased estimate of 𝜎 from the true model. This demonstrates why models selected via best subset selection are invalid for inference.
红线是y轴等于x轴的线,这是真实模型中𝜎的无偏估计。 如下图所示, 𝜎的估计值是从最佳AIC和BIC选择的模型中得出的。 实际上,它们将始终小于或等于真实模型中𝜎的无偏估计。 这说明了为什么通过最佳子集选择所选择的模型对于推理无效。
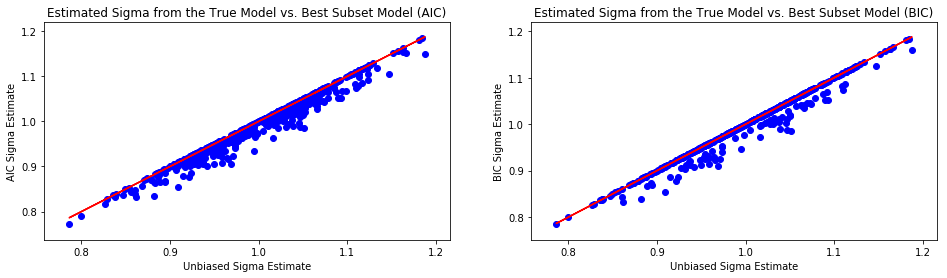
奖励部分:调查LASSO和岭回归中误差项的估计标准偏差的偏差 (Bonus Section: Investigating Bias in Estimated Standard Deviation of the Error term in LASSO and Ridge Regression)
While working on the simulation study above, I became interested in the potential bias of regularization methods on estimates of the standard deviation of the error term in a linear model, although one wouldn’t use a regularized model to estimate a parameter for the purposes of inference. As you most likely know, LASSO and Ridge regression intentionally bias estimated coefficients towards zero to reduce the amount of variance in the model (how much estimated coefficients change from sample to sample from the same population). The LASSO can set coefficients equal to zero, performing variable selection. Ridge regression biases coefficients towards zero, but will not set them equal to zero, so it isn’t a variable selection tool like best subset selection or the LASSO.
在进行上述仿真研究时,我对正则化方法对线性模型中误差项的标准偏差的估计的潜在偏差感兴趣,尽管出于以下目的,人们不会使用正则化模型来估计参数:推理。 如您最可能知道的那样,LASSO和Ridge回归有意将估计系数偏向零,以减少模型中的方差量(在同一总体中,样本之间的估计系数变化了多少)。 LASSO可以将系数设置为零,从而执行变量选择。 岭回归将系数偏向零,但不会将其设置为零,因此它不是像最佳子集选择或LASSO这样的变量选择工具。
I used the same set up as before, but upped the sample size from 100 to 200, the number of independent variables from 6 to 100, and the number of significant independent variables from 2 to 50. The shrinkage parameter in both the LASSO and Ridge models were chosen among 0.01, 0.1, 1.0, and 10.0 using 3-fold cross validation. I counted the number of non-zero coefficients in the LASSO model for purposes of calculating 𝜎̂ and used all 100, plus 1 for the intercept, for the Ridge model, since it biases coefficients to zero but doesn’t set them to zero.
我使用了与以前相同的设置,但是样本量从100增加到200,自变量从6增加到100,有效自变量从2增加到50。LASSO和Ridge中的收缩参数使用3倍交叉验证从0.01、0.1、1.0和10.0中选择模型。 我数的非零系数的数目在LASSO模型用于计算σ的目的,所使用的所有100,再加上1截距,对于岭模型,因为它偏置系数为零,但不将它们设置为零。
Obviously, regularized linear models are not valid for the purposes of inference because they bias estimates of coefficients. I still thought investigating any bias in the estimated standard deviation of the error term was worth writing a little code.
显然,正则化线性模型出于推论的目的是无效的,因为它们会使系数的估计产生偏差。 我仍然认为调查误差项的估计标准偏差中的任何偏差都值得编写一些代码。
The plots are below this code chunk for the simulations.
用于仿真的图在此代码块下方。
By visual inspection, 𝜎̂ appears biased downwards in the LASSO models, but the unbiased estimate doesn’t form an upper bound as it does with the best AIC and BIC models. The Ridge models do not show obvious bias in estimating this parameter. Let’s investigate with a paired t-test, since the estimates are derived from the same sample at each iteration. I’m using the standard p-value cutoff of 0.05, because I’m too lazy to decide my desired power of the test.
通过目视检查,在LASSO模型中𝜎̂出现向下偏差,但与最佳AIC和BIC模型一样,无偏差估计值不会形成上限。 Ridge模型在估计此参数时没有显示明显的偏差。 让我们用配对t检验进行研究,因为估计是在每次迭代时从相同的样本得出的。 我使用的标准p值截止值为0.05,因为我太懒了,无法确定所需的测试功效。
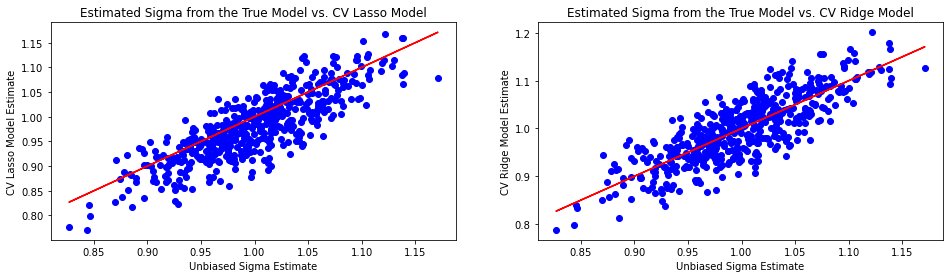

As guessed by the visual inspection, there is insufficient evidence for a difference in means between the estimates of 𝜎̂ , from the true and Ridge models. However, there is sufficient evidence at the 0.05 significance level to conclude that the LASSO models tended to make downwardly biased estimates of 𝜎̂. Whether or not this is a generalizable fact is unknown. It would require a formal proof to make a conclusion.
由于猜测由目视检查,对在σ的估计方法之间的差异,从真与岭车型证据不足。 然而,在0.05的显着性水平足够的证据得出的结论是套索模型倾向于使σ向下偏估计。 这是否是一个普遍的事实还不得而知。 得出结论需要正式证明。
Thanks for making it to the end. Although using the data to select a model invalidates classical inference assumptions, post-selection inference is a hot area of statistical research. Perhaps we’ll be talking about AutoInference in a few years.
感谢您的努力。 尽管使用数据选择模型会使经典推论假设无效,但是选择后推论是统计研究的热门领域。 也许几年后我们会谈论自动推理。
All of my code for this project can be found here.
我在这个项目中的所有代码都可以在这里找到。
翻译自: https://towardsdatascience.com/origins-of-automl-best-subset-selection-1c40144d86df
最佳子集aic选择
本文来自互联网用户投稿,该文观点仅代表作者本人,不代表本站立场。本站仅提供信息存储空间服务,不拥有所有权,不承担相关法律责任。如若转载,请注明出处:http://www.mzph.cn/news/388451.shtml
如若内容造成侵权/违法违规/事实不符,请联系多彩编程网进行投诉反馈email:809451989@qq.com,一经查实,立即删除!