knn 邻居数量k的选取
Classification is more-or-less just a matter of figuring out to what available group something belongs.
分类或多或少只是弄清楚某个事物所属的可用组的问题。
Is Old Town Road a rap song or a country song?
Old Town Road是说唱歌曲还是乡村歌曲?
Is the tomato a fruit or a vegetable?
番茄是水果还是蔬菜?
Machine learning (ML) can help us efficiently classify such data, even when we do not know (or have names for) the classes to which they belong. In cases where we do have labels for our groups, an easy-to-implement algorithm that may be used to classify new data is K Nearest Neighbors (KNN). This article will consider the following, with regards to KNN:
机器学习(ML)可以帮助我们有效地对此类数据进行分类,即使我们不知道(或为其命名)它们所属的类。 如果我们确实有组的标签,则可以用来对新数据进行分类的一种易于实现的算法是K最近邻居(KNN)。 本文将考虑以下有关KNN的问题:
- What is KNN 什么是KNN
- The KNN Algorithm KNN算法
- How to implement a simple KNN in Python, step by step 如何逐步在Python中实现简单的KNN
监督学习 (Supervised Learning)
In the image above, we have a collection of dyed squares, in variegated shades from light pink to dark blue. If we decide to separate the cards into two groups, where should we place the cards that are purple or violet?
在上图中,我们收集了一组染色的正方形,从浅粉红色到深蓝色的杂色阴影。 如果我们决定将卡片分成两组,那么应该将紫色或紫色的卡片放在哪里?
In supervised learning we are given labeled data, e.g., knowing that, “these 5 cards are red-tinted, and these five cards are blue-tinted.” A supervised learning algorithm analyzes the training data — in this case, the 10 identified cards — and produces an inferred function. This function may then be used for mapping new examples or determining to which or the two classes each of the other cards belongs.
在监督学习中,我们获得了带有标签的数据,例如,知道“这5张卡是红色的,而这5张卡是蓝色的”。 监督学习算法分析训练数据(在这种情况下为10张识别出的卡片),并产生推断功能。 然后,该功能可用于映射新示例或确定每个其他卡属于哪个类别或两个类别。
什么是分类? (What is Classification?)
Classification is an example of supervised learning. In ML, this involves identifying to which of a set of categories a new observation belongs, on the basis of a training dataset containing observations whose category membership is known (is labeled). Practical examples of classification include assigning an email as spam or not spam or predicting whether or not a client will default on a bank loan.
分类是监督学习的一个例子。 在ML中,这涉及基于训练数据集来识别新观测值属于一组类别中的哪一个,该训练数据集包含其类别成员身份已知(带有标签)的观测值。 分类的实际示例包括将电子邮件指定为垃圾邮件或不指定为垃圾邮件,或预测客户是否会拖欠银行贷款。
K最近的邻居 (K Nearest Neighbors)
The KNN algorithm is commonly used in many simpler ML tasks. KNN is a non-parametric algorithm which means that it doesn’t make any assumptions about the data. KNN makes its decision based on similarity measures, which may be thought of as the distance of one example from others. This distance can simply be Euclidean distance. Also, KNN is a lazy algorithm, which means that there is little to no training phase. Therefore, new data can be immediately classified.
KNN算法通常用于许多更简单的ML任务中。 KNN是一种非参数算法,这意味着它不会对数据做任何假设。 KNN基于相似性度量进行决策,可以将其视为一个示例与其他示例之间的距离。 该距离可以简单地是欧几里得距离。 同样,KNN是一种惰性算法,这意味着几乎没有训练阶段。 因此,可以立即对新数据进行分类。
KNN的优缺点 (Advantages and Disadvantages of KNN)
Advantages
优点
- Makes no assumptions about the data 不对数据做任何假设
- Simple algorithm 简单算法
- Easily applied to classification problems 轻松应用于分类问题
Disadvantages
缺点
- High sensitivity to irrelevant features 对无关功能具有很高的敏感性
- Sensitive to the scale of data used to compute distance 对用于计算距离的数据规模敏感
- Can use a lot of memory 可以使用很多内存
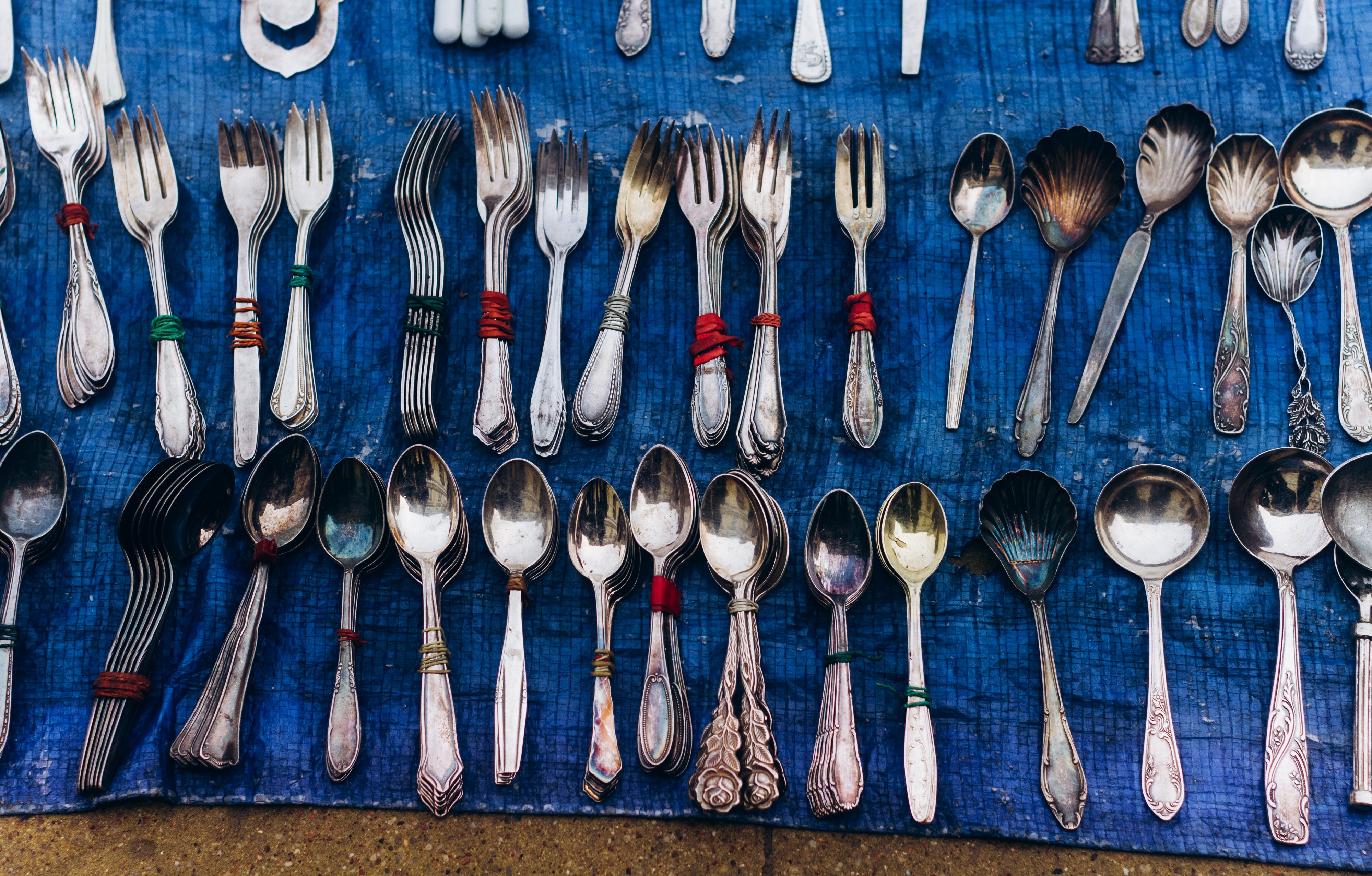
While KNN is considered a ‘lazy learner’, it can also be a bit of an over-achiever — searching the entire dataset to compute the distance between each new observation and each known observation.
虽然KNN被认为是“懒惰的学习者”,但它也可能有点过时-搜索整个数据集以计算每个新观测值与每个已知观测值之间的距离。
So, how do we use KNN?
那么,我们如何使用KNN?
KNN算法 (Algorithm of KNN)
We start by selecting some value of k, such as 3, 5 or 7.
我们首先选择k的某个值,例如3、5或7。
The value of k can be any number below the number of observations in the dataset. When the choice is between an even number of classes, setting this parameter to an odd number avoids the possibility of a tie between the two.
k的值可以是数据集中观测值以下的任何数字。 如果在偶数类之间进行选择,则将此参数设置为奇数可以避免两者之间产生联系。
One approach for selecting k is to use the integer nearest to the square root of the number of samples in the labeled classes (+/- 1 if the square root is an even number). Given 10 labeled points from our two classes, we would set k equal to 3, the integer nearest to √10.
选择k的一种方法是使用最接近标记类别中样本数量平方根的整数(如果平方根是偶数,则为+/- 1)。 给定两个类中的10个标记点,我们将k设置为3,即最接近√10的整数。
Next:
下一个:
- Choose k samples closest to the new data point according to their Euclidean distance from that point. 根据距该数据点的欧式距离选择k个最接近新数据点的样本。
- For each data point in test: Calculate the distance between test data and each row of training data with the help of Euclidean distance. 对于测试中的每个数据点:借助欧几里得距离来计算测试数据与训练数据的每一行之间的距离。
- Now, sort point distances in ascending order according to the distance computed. 现在,根据计算出的距离以升序对点距离进行排序。
- Choose top k from the distance array. 从距离数组中选择前k个。
- Now, assign a class to the test sample based on most frequent class of these rows. 现在,根据这些行中最常见的类别为测试样本分配一个类别。
If you comfortably read through those bullet points, you may already know enough about ML algorithms that you did not need to read this article (but please, continue).
如果您舒适地通读了这些要点,则可能已经对ML算法有足够的了解,而无需阅读本文(但请继续)。
Essentially, each of the k nearest neighbors is a vote for its own class. The new data point will be classified based on which class has the greater number of votes out of the test points k nearest neighbors.
本质上,k个最近的邻居中的每一个都是其所属阶级的投票。 新数据点将基于哪个类在k个最邻近邻居的测试点中具有更大的票数进行分类。
例 (Example)
Let’s see an example to understand better.
让我们看一个例子,以更好地理解。
Suppose we have some data which is plotted as follows:
假设我们有一些数据绘制如下:
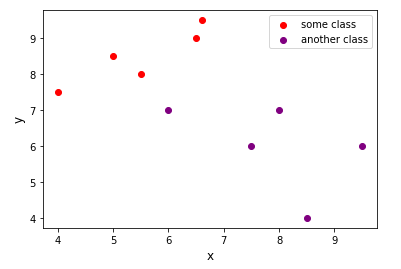
You can see that there are two classes of data, one red and the other purple.
您会看到有两类数据,一类是红色,另一类是紫色。
Now, consider that we have a test data point (indicated in black ) and we have to predict whether it belongs to the red class or the purple class. We will compute the Euclidean distance of the test point with k nearest neighbors. Here k = 3.
现在,考虑我们有一个测试数据点(用黑色表示),并且我们必须预测它是属于红色类别还是紫色类别。 我们将计算k个最近邻居的测试点的欧几里得距离。 这里k = 3。
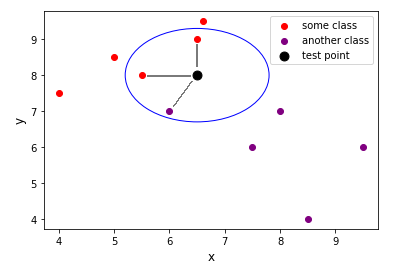
Now, we have computed the distance between the test point and its three nearest neighbors. Two of the neighboring points are from the red class, and one is from the purple class. Hence this data point will be classified as belonging to the red class.
现在,我们已经计算出测试点与其三个最近的邻居之间的距离。 相邻点中的两个来自红色类别,一个来自紫色类别。 因此,该数据点将被归类为属于红色类别。
使用Python实施 (Implementation using Python)
We will use the Numpy and Sklearn libraries to implement KNN. In addition, we will use Sklearn’s GridSearchCV function.
我们将使用Numpy和Sklearn库来实现KNN。 另外,我们将使用Sklearn的GridSearchCV函数。
网格搜索简历 (Grid Search CV)
Grid search is the process of performing hyperparameter tuning in order to determine the optimal values of the hyperparameters for a given model. This is significant as the performance of the entire model is based on the values specified.
网格搜索是执行超参数调整以确定给定模型的超参数的最佳值的过程。 这很重要,因为整个模型的性能基于指定的值。
为什么要使用它? (Why use it?)
Models can involve more than a dozen parameters. Each of these parameters can take on specific characteristics, based on their hyperparameter settings; and hyperparameters can present as ranges or conditions, some of which may be programmatically changed during modeling.
模型可以包含十几个参数。 这些参数中的每一个都可以基于其超参数设置而具有特定的特性; 超参数可以表示为范围或条件,其中某些可以在建模过程中以编程方式更改。
Manually selecting best hyperparameters in the ML process can feel like a nightmare for practitioners. Sklearn’s GridSearchCV instance helps to automate this process, programatically determining the best settings for specified parameters.
在ML流程中手动选择最佳超参数对于从业者来说就像一场噩梦。 Sklearn的GridSearchCV实例有助于自动执行此过程,以编程方式确定指定参数的最佳设置。
So, what does this look like in (pseudocode) practice? We start be importing required libraries.
那么,这在(伪代码)实践中是什么样的呢? 我们开始导入所需的库。
import pandas as pd
import numpy as npfrom sklearn.model_selection import train_test_split
from sklearn.preprocessing import StandardScaler
from sklearn.neighbors import KNeighborsClassifier
from sklearn.model_selection import GridSearchCV
from sklearn.metrics import accuracy_score
KNN功能 (KNN function)
We will create a custom KNN method with 5 parameters: training examples, training labels, test examples, test label and a list of possible values of k to train on.
我们将创建一个具有5个参数的自定义KNN方法:训练示例,训练标签,测试示例,测试标签和要训练的k可能值列表。
First, we create a KNeighborsClassifier() object, imported from Sklearn. Then we create a dictionary named “parameters” and store the list k in it. Our third step is to pass the classifier, i.e. KNN, and the parameters to GridSearchCV and fit this model on the training data. GridSearchCV will optimize hyperparameters for training and we will make predictions on test data using the tuned hyperparameters. To predict the labels on test data, we call model.predict(). We can check the accuracy of our model and its predictions with the accuracy_score() function we import from Sklearn.
首先,我们创建一个从Sklearn导入的KNeighborsClassifier()对象。 然后,我们创建一个名为“ parameters”的字典,并将列表k存储在其中。 我们的第三步是将分类器(即KNN)和参数传递给GridSearchCV,并将此模型拟合到训练数据上。 GridSearchCV将优化用于训练的超参数,我们将使用调整后的超参数对测试数据进行预测。 为了预测测试数据上的标签,我们调用model.predict()。 我们可以使用从Sklearn导入的precision_score()函数检查模型的准确性及其预测。
def KNN(x_tr, y_tr, x_te, y_te, k):
print('\nTraining Started for values of k', [each for each in k],'.......')
# Create an knn object using imported KNeighborsClassifier() from sklearn
knn = KNeighborsClassifier()# parameters i.e. k neighbors list
parameters = {'n_neighbors':k}
# Training the model
model = GridSearchCV(knn, param_grid = parameters, cv=3)
model.fit(x_tr, y_tr)
print('Best value of k is ',model.best_params_)
# Making Predictions on test data
print('\nPredicting on Test data.......')
pred = model.predict(x_te)
print('\nAccuracy of model on test is', accuracy_score(y_te, pred)*100,'%')
return accuracy_score(y_te, pred)
This custom method is just some pre-processing done on the Google Playstore dataset. Note: a version of the dataset may be obtained from Kaggle. Data filenames and required pre-processing steps may vary.
此自定义方法只是对Google Playstore数据集进行的一些预处理。 注意:数据集的版本可以从Kaggle获得。 数据文件名和所需的预处理步骤可能会有所不同。
def data_preprocess():
# processing Apps.csv
data = pd.read_csv('apps.csv')
columns = ['App', 'Category', 'Rating', 'Size', 'Type', 'Price', 'Genres']
data[columns]
new_data = data[columns].copy()
new_data = new_data.fillna(0)
for each in range(0, len(new_data['Rating'])):
if new_data['Rating'][each] == 0:
new_data.at[each, 'Rating'] = new_data['Rating'].mean()
price_list = [float(each.replace("$","")) for each in new_data.Price]
new_data.Price = price_list
# processing User_reviews.csv
data2 = pd.read_csv('user_reviews.csv')
column = ['App', 'Sentiment_Polarity', 'Sentiment_Subjectivity', 'Sentiment']
data2[column]
new_data2 = data2[column].copy()
# merging the two datasets into one final dataset
df = new_data.merge(new_data2, on='App')
df.Sentiment = df['Sentiment'].replace(to_replace='Positive', value=1).replace(to_replace='Negative', value=-1).replace(to_replace='Neutral', value=0)
df.Sentiment_Polarity = df.Sentiment_Polarity.fillna(df.Sentiment_Polarity.mean())
df.Sentiment_Subjectivity = df.Sentiment_Subjectivity.fillna(df.Sentiment_Subjectivity.mean())
df = df[df['Sentiment'].notna()]
df.Type = df['Type'].replace(to_replace='Free', value=1).replace(to_replace='Paid', value=0)
df = df.drop(['Size'], axis=1)
# separating dataset into samples and labels
X = df.iloc[:, 0:7]
y = df.iloc[:, 8:9]
# encoding the dataset
X = pd.get_dummies(X)
print('\nFinished pre-processing data....')
return X, y
We create a main function and all the processing is done in this function. We will call the above created methods in this main function. Also, we are applying some data normalization techniques in this function and calling the custom function on our data.
我们创建一个主要功能,所有处理都在该功能中完成。 我们将在此主函数中调用上面创建的方法。 另外,我们在此函数中应用了一些数据标准化技术,并在数据上调用了自定义函数。
Normalization may not be required, depending on the data you use.
根据您使用的数据,可能不需要规范化。
Finished pre-processing data....Training Started for values of k [3, 5, 7] .......
Best value of k is {'n_neighbors': 7}Predicting on Test data.......Accuracy of model on test is 86.07469428225184 %
Running our function results in a respectable accuracy score of 86 %.
运行我们的功能可获得可观的86%准确度。
In this article, we took a look at the K Nearest Neighbors machine learning algorithm. We discussed how KNN uses Euclidean distance to compare the similarity of test data features to those of labeled training data. We also explored a simple solution for determining a value for k. In our custom code example, we demonstrated the use of Sklearn’s GridSearchCV for optimizing our model’s hyperperameters (and for sparing ourselves the intense manual effort that might be otherwise required to exhaustively tune those hyperparameters).
在本文中,我们研究了K最近邻居机器学习算法。 我们讨论了KNN如何使用欧氏距离将测试数据特征与标记训练数据的相似性进行比较。 我们还探索了确定k值的简单解决方案。 在我们的自定义代码示例中,我们演示了使用Sklearn的GridSearchCV来优化模型的超级参数(并为自己节省了可能需要详尽调整这些超级参数的大量手工工作)。
We can dive much deeper into KNN theory and leverage it over a broad range of applications. KNN has many uses, from data mining to recommender systems and competitor analysis. For those seeking to further explore KNN in Python, a good course of action is to try it for yourself.
我们可以更深入地研究KNN理论,并在广泛的应用中利用它。 从数据挖掘到推荐系统和竞争对手分析,KNN有许多用途。 对于那些寻求用Python进一步探索KNN的人来说,一个好的做法是自己尝试一下。
If you would like some suggestions, let me know in the comments or feel free to connect with me on Linkedin.
如果您想提出建议,请在评论中让我知道,或随时通过Linkedin与我联系。
翻译自: https://medium.com/swlh/choosing-k-nearest-neighbors-6f711449170d
knn 邻居数量k的选取
本文来自互联网用户投稿,该文观点仅代表作者本人,不代表本站立场。本站仅提供信息存储空间服务,不拥有所有权,不承担相关法律责任。如若转载,请注明出处:http://www.mzph.cn/news/388403.shtml
如若内容造成侵权/违法违规/事实不符,请联系多彩编程网进行投诉反馈email:809451989@qq.com,一经查实,立即删除!