对数据仓库进行数据建模
Some data sets are just not meant to have the geospatial representation that can be clustered. There is great variance in your features, and theoretically great features as well. But, it doesn’t mean is statistically separable.
某些数据集并不意味着具有可以聚类的地理空间表示。 您的功能差异很大,理论上也很棒。 但是,这并不意味着在统计上是可分离的。
那么,我什么时候停止? (So, WHEN DO I STOP?)
Always Visualize your data based on the class label you are trying to predict
始终根据您要预测的类标签可视化数据
columns_pairplot = x_train.select_dtypes(include=['int', 'float']).join(y_train)
sns.pairplot(columns_pairplot, hue = 'readmitted')
plt.show()
The distribution of different classes is almost exact. Of course, it is an imbalanced dataset. But, notice how the spread of the classes overlaps as well?
不同类别的分布几乎是准确的。 当然,它是一个不平衡的数据集。 但是,注意这些类的传播也是如何重叠的吗?
2. Apply the t-SNE visualization
2.应用t-SNE可视化
t-SNE is “t-distributed stochastic neighbor embedding”. It maps higher dimensional data to 2-D space. This approximately preserves the nearness of the samples.
t-SNE是“ t分布随机邻居嵌入”。 它将高维数据映射到二维空间。 这大致保持了样品的接近性。
You might need to apply different learning rates to find the best one for your dataset. Usually, try values between 50 and 200.
您可能需要应用不同的学习率才能为数据集找到最佳学习率 。 通常,请尝试输入介于50和200之间的值。
Hyper-parameter, perplexity balances the importance t-SNE gives to local and global variability of the data. It is a guess on the number of close neighbors each point has. Use values between 5–50. Higher, if there are more data points. Perplexity value should not be more than the number of data points.
超参数, 困惑度平衡了t-SNE对数据局部和全局可变性的重要性。 这是对每个点的近邻数量的猜测。 使用5-50之间的值。 如果有更多数据点,则更高 。 困惑度值不应大于数据点的数量。
NOTE: Axis to t-SNE plot are not interpretable. They will be different every time t-SNE is applied
注意:轴到t-SNE图是无法解释的。 每次应用t-SNE时它们都会不同
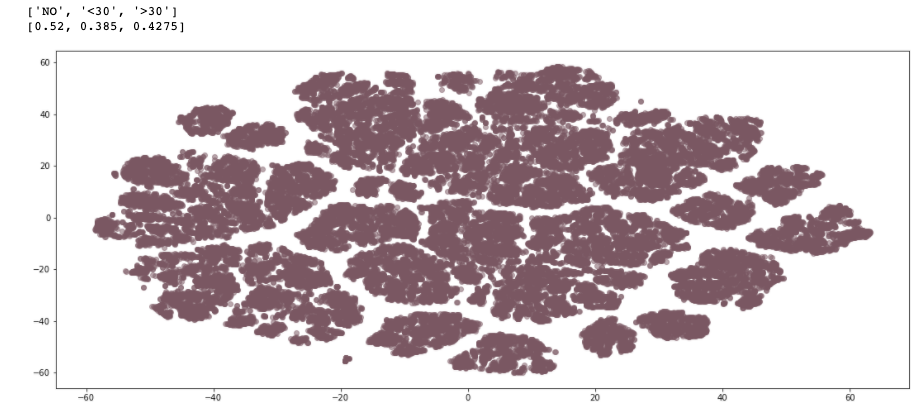
Hmm, let’s look a bit more- tweak some hyperparameters.
嗯,让我们再看一下-调整一些超参数。
# reduce dimensionality with t-sne
tsne = TSNE(n_components=2, verbose=1, perplexity=50, n_iter=1000, learning_rate=50)
tsne_results = tsne.fit_transform(x_train)
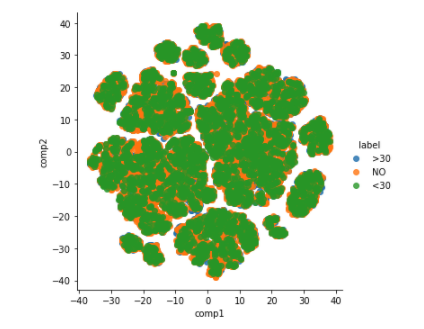
Do you see how the clusters can not be separated! I should have stopped here! But, I could not get myself out of the rabid hole. [YES WE ALL GO DOWN THAT SOMETIMES].
您是否看到群集无法分离! 我应该在这里停下来! 但是,我无法摆脱困境。 [是的,我们有时会倒下]。
3. Multi-Class Classification
3.多类别分类
We already know from above that the decision boundaries are non-linear. So, we can use an SVC (Support Vector Classifier with RBF Kernel)
从上面我们已经知道决策边界是非线性的。 因此,我们可以使用SVC (带有RBF内核的支持向量分类器)
from sklearn.svm import SVC
from sklearn.model_selection import GridSearchCVsvc_model = SVC() ## default kernel - RBF
parameters = {'C':[0.1, 1, 10], 'gamma':[0.00001, 0.0001, 0.001, 0.01, 0.1]}
searcher = GridSearchCV(svc_model, param_grid = parameters, n_jobs= 4, verbose = 2, return_train_score= True)
searcher.fit(x_train, y_train)
# Report the best parameters and the corresponding score
Train Score: 0.59Test Score: 0.53F1 Score: 0.23Precision Score: 0.24
火车得分:0.59测试得分:0.53F1得分:0.23精度得分:0.24
So, I should have stopped earlier…It is always good to have an understanding of your data before you try to over-tune and complicate the model in the hopes of better results. Good Luck!
因此,我应该早点停下来……在您尝试过度调整模型并使模型复杂化以期获得更好的结果之前,最好先了解您的数据。 祝好运!
翻译自: https://towardsdatascience.com/determine-if-your-data-can-be-modeled-e619d65c13c5
对数据仓库进行数据建模
本文来自互联网用户投稿,该文观点仅代表作者本人,不代表本站立场。本站仅提供信息存储空间服务,不拥有所有权,不承担相关法律责任。如若转载,请注明出处:http://www.mzph.cn/news/388332.shtml
如若内容造成侵权/违法违规/事实不符,请联系多彩编程网进行投诉反馈email:809451989@qq.com,一经查实,立即删除!