ai 中 统计
Today I plan to cover the following topics: Linear independence, special matrices, and matrix decomposition.
今天,我计划涵盖以下主题:线性独立性,特殊矩阵和矩阵分解。
线性独立 (Linear independence)
A set of vectors is linearly independent if none of these vectors can be written as a linear combination of other vectors.For example, V1=(1,0) and V2=(0,1). Here, V2 cannot be written in terms of V1. However, V3 (3,4) is linearly dependent as V3 can be expressed as 3V1+4V2.
如果这些向量中的任何一个都不能写成其他向量的线性组合,则它们是线性独立的,例如V1 =(1,0)和V2 =(0,1)。 在此,不能用V1来写V2。 但是,V3(3,4)与线性相关,因为V3可以表示为3V1 + 4V2。
Mathematically, s={V1, V2,…., Vn} is linearly independent if and only if the linear combination α1V1+α2V2+…..+αnVn=0 means that all αi=0.
在数学上,当且仅当线性组合α1V1+α2V2+ .... +αnVn= 0表示所有αi= 0时,s = {V1,V2,....,Vn}是线性独立的。
矩阵运算 (Matrix operations)
Matrices can transform one vector to another vector. For example, V is an Nx1 vector and w is also an Nx1 vector.
矩阵可以将一个向量转换为另一向量。 例如,V是Nx1向量,w也是Nx1向量。
矩阵的痕迹 (Trace of a matrix)
Trace of a matrix is given by its sum of diagonal elements. For matrix A, its trace will be the summation of all the elements with the same value of row and column.
矩阵的迹线由对角元素的总和给出。 对于矩阵A,其轨迹将是具有相同行和列值的所有元素的总和。
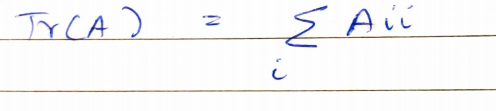
一些属性 (Some properties)
- Tr(A+B) = Tr(A)+Tr(B) Tr(A + B)= Tr(A)+ Tr(B)
- Tr(AB) = Tr(BA) Tr(AB)= Tr(BA)
- Tr(A) = Tr(A.T) (A.T means transpose of matrix A) Tr(A)= Tr(AT)(AT表示矩阵A的转置)
矩阵的行列式 (The determinant of a matrix)
Laplace expansion for an NxN matrix is given by the following formula:
NxN矩阵的拉普拉斯展开式由以下公式给出:
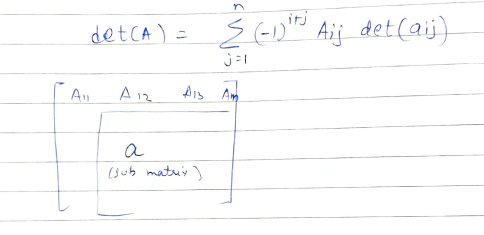
Determinant actually represents the volume formed by the column vectors. For a 2x2 vector, it represents the area.
行列式实际上表示由列向量形成的体积。 对于2x2向量,它表示面积。
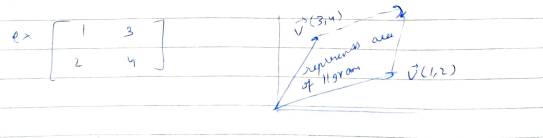
矩阵的可逆性 (Invertibility of a matrix)
The inverse of a matrix A is possible only if the det(A) is not 0. Note that this automatically means that the columns of A have to be linearly independent. Consider a matrix below.
仅当det(A)不为0时,矩阵A的逆才可能。请注意,这自动意味着A的列必须线性独立。 考虑下面的矩阵。
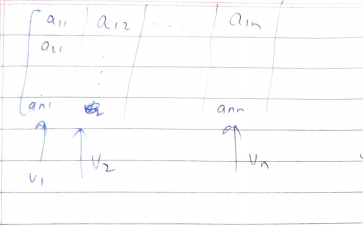
Note that V1, V2,…., Vn are vectors and if any vector, say, Vn can be written as linearly dependent vectors of the rest like Vn=α1V1+α2V2+…..+αn-1Vn-1 then, we can do a simple column operation i.e last column = the last column- (α1V1+α2V2+…..+αn-1Vn-1) and this would yield a column full of zeros. This will make the determinant of matrix 0. For a 2x2 matrix, we will have 2 vectors V1 and V2. If V1 and V2 are linearly dependent like V1=2V2, then the area formed by the two vectors is going to be zero. A smart way to put this would be that the two vectors are parallel to one another.
请注意,V1,V2,...,Vn是向量,如果有任何向量,例如,Vn可以写为其余部分的线性相关向量,例如Vn =α1V1+α2V2+ ..... +αn-1Vn-1,那么我们可以一个简单的列运算,即最后一列=最后一列-(α1V1+α2V2+ ..... +αn-1Vn-1),这将产生一列充满零的列。 这将决定矩阵0的行列式。对于2x2矩阵,我们将有2个向量V1和V2。 如果V1和V2是线性相关的,例如V1 = 2V2,则由两个向量形成的面积将为零。 一种明智的解释是,两个向量彼此平行。
特殊矩阵和向量 (Special matrices and vectors)
- Diagonal matrix: Only diagonal entries are non zero and rest all elements are zero. D(i,j) = 0 if i is not equal to j. 对角矩阵:仅对角线条目为非零,其余所有元素为零。 如果i不等于j,则D(i,j)= 0。
- Symmetric matrix: A matrix is said to be symmetric if the matrix and its transpose are equal. 对称矩阵:如果矩阵及其转置相等,则称该矩阵是对称的。
- Unit vector: vector with unit length. 2-Norm of the vector is 1. 单位向量:单位长度的向量。 向量的2范数为1。
- Orthogonal vectors: Two vectors X and Y are orthogonal if (X.T)Y = 0 正交向量:如果(XT)Y = 0,则两个向量X和Y是正交的
- Orthogonal matrix: If a transpose of a matrix is equal o its inverse, then we can say that the matrix is orthogonal. Also, all columns are orthonormal. The orthogonal matrix can be used to rotate vectors which preserve volume. 正交矩阵:如果矩阵的转置等于其逆,则可以说矩阵是正交的。 同样,所有列都是正交的。 正交矩阵可用于旋转保留体积的向量。
- Orthonormal matrix: If the inverse of a matrix is equal to its transpose with unit determinant, the matrix is said to be orthonormal. 正交矩阵:如果矩阵的逆等于其行列式的转置,则称该矩阵为正交的。
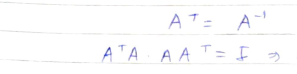
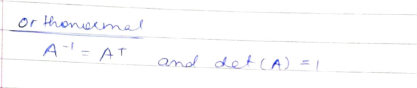
本征分解 (Eigen decomposition)
Eigen decomposition is extremely useful for a square symmetric matrix. Let's look at the physical meaning of the term.
本征分解对于平方对称矩阵非常有用。 让我们看一下该术语的物理含义。
Every real matrix can be thought of as a combination of rotation and stretching.
每个实数矩阵都可以视为旋转和拉伸的组合。
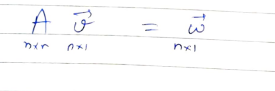
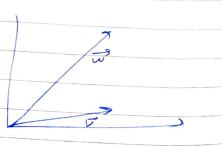
Here, A can be thought of as an operator tat stretches and rotates a vector v to obtain a new vector w. Eigenvectors for a matrix are those special vectors that only stretch under the action of a matrix. Eigenvalues are the factor by which the eigenvectors stretch. In the equation below, the vector v is stretched by a value of lambda when operated with an eigenvector A.
在这里,可以将A视为操作员tat拉伸并旋转向量v以获得新的向量w。 矩阵的特征向量是那些仅在矩阵作用下才拉伸的特殊向量。 特征值是特征向量伸展的因子。 在下面的公式中,向量v在使用特征向量A时被拉伸了一个lambda值。
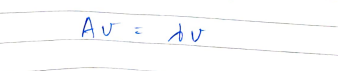
Say, A has n linearly independent eigenvectors {V1, V2,….., Vn}. On concatenating all the vectors as a column, we get a single eigenvector matric V where V=[V1, V2,….., Vn]. If we concatenate the corresponding eigenvalues into a diagonal matrix i.e Λ=diag(λ1, λ2,…, λn), we get the eigendecomposition (factorization) of A as:
假设A有n个线性独立的特征向量{V1,V2,…..,Vn}。 将所有向量连接为一列后,我们得到单个特征向量矩阵V,其中V = [V1,V2,.....,Vn]。 如果将对应的特征值连接到对角矩阵即Λ = diag(λ1,λ2,...,λn),则得到A的特征分解(因式分解)为:
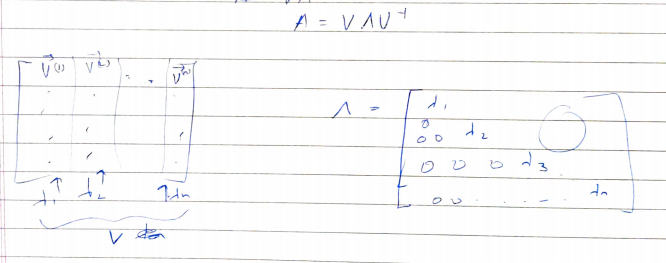
Real symmetric matrices have real eigenvectors and real eigenvalues.
实对称矩阵具有实特征向量和实特征值。

二次形式和正定矩阵 (Quadratic form and positive definite matrix)
The quadratic form can be interpreted as a ‘weighted’ length.
二次形式可以解释为“加权”长度。


The positive definite (PD) matrix has all eigenvalues greater than zero. The semi-definite positive(PSD) matrix has eigenvalues greater than equal to zero. A PD matrix has a property that for all X, (X.T)AX is greater than 0. For example, if A=I or identity matrix then, (X.T)I(X)=(X.T)(X) which is greater than 0. A PSD matrix has a property that for all X, (X.T)AX is greater than equal to 0. Similarly, a negative definite (ND)matrix has all eigenvalues less than zero. And semi-negative definite (PD)matrix has all eigenvalues less than equal to zero.
正定(PD)矩阵的所有特征值均大于零。 半定正(PSD)矩阵的特征值大于零。 PD矩阵的属性是,对于所有X,(XT)AX都大于0。例如,如果A = I或单位矩阵,则(XT)I(X)=(XT)(X)大于0. PSD矩阵具有以下特性:对于所有X,(XT)AX都等于0。类似地,负定(ND)矩阵的所有特征值均小于零。 半负定(PD)矩阵的所有特征值均小于零。
奇异值分解 (Singular value decomposition)
If A is an MxN matrix, then
如果A是MxN矩阵,则
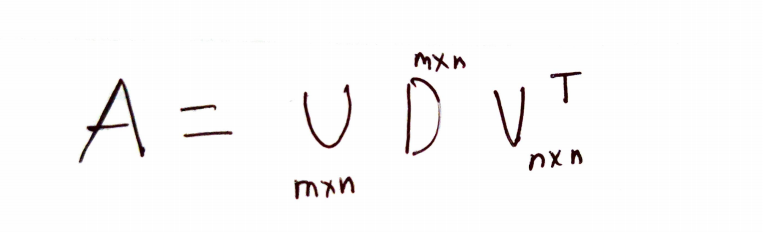
- U is an MxM matrix and orthogonal U是MxM矩阵并且正交
- V is an NxN matrix and orthogonal V是NxN矩阵并且正交
- D is an MxN matrix and diagonal D是MxN矩阵和对角线
- Elements of U are the eigenvectors of A(A.T), called left singular vectors U的元素是A(AT)的特征向量,称为左奇异向量
- Elements of Vare the eigenvectors of (A.T)A, called right singular vectors Vare的元素(AT)A的特征向量,称为右奇异向量
- Non zero elements of D are the square-root( λ((A.T)(A))) which means square-root of eigenvalues of (A.T)(A), called as singular values D的非零元素是平方根(λ((AT)(A))),它表示(AT)(A)特征值的平方根,称为奇异值
结束 (End)
Thank you and stay tuned for more blogs on AI.
谢谢,请继续关注更多有关AI的博客。
翻译自: https://towardsdatascience.com/statistics-for-ai-part-2-43d81986c87c
ai 中 统计
本文来自互联网用户投稿,该文观点仅代表作者本人,不代表本站立场。本站仅提供信息存储空间服务,不拥有所有权,不承担相关法律责任。如若转载,请注明出处:http://www.mzph.cn/news/388300.shtml
如若内容造成侵权/违法违规/事实不符,请联系多彩编程网进行投诉反馈email:809451989@qq.com,一经查实,立即删除!