安卓代码还是xml绘制页面
Plotting the actual and predicted data is frequently used for visualizing and analyzing how the actual data correlate with those predicted by the model. Ideally, this should correspond to a slope of 1 and an intercept of 0. However, for many people, it can sometimes be confusing to exactly know which data should be plotted on the x-axis and which one should be on the y-axis. Indeed, by a quick search on Google, one finds many results with predicted on the x-axis or on the y-axis. However, a paper by Piñeiro et al., shows that there is only one correct answer.
P印迹的实际和预测数据被频繁用于可视化和分析实际数据是如何与模型预测相关。 理想情况下,它应该对应于斜率1和截距0。但是,对于许多人来说,有时可能很难准确地知道哪些数据应绘制在x轴上,哪些数据应绘制在y轴上。 。 实际上,通过在Google上进行快速搜索,可以找到许多在x轴或y轴上具有预测结果的结果。 但是,Piñeiro 等人的一篇论文表明,只有一个正确的答案。
As an example, let’s generate a randomly noisy set of data.
例如,让我们生成一个随机嘈杂的数据集。
n <- 200
k <- .5 # k will be equal to .1, .5 and 1
noise <- rnorm(n, 0, 20)
x1 <- runif(n, 1, 100)
x2 <- runif(n, 1, 100)
y <- x1 + x2 + k*noisedf <- data.frame(actual = y, var1 = x1, var2 = x2)
Fitting a linear regression, which should have a slope and an intercept of 1 and of 0, respectively.
拟合线性回归,其斜率和截距应分别为1和0。
lm_fit <- df %>%
lm(actual ~ var1 + var2, .) %>%
broom::augment()
Plotting the actual vs. predicted plot (left panel) and the predicted vs. actual plot (right panel).
绘制实际与预测图(左图)和预测与实际图(右图)。
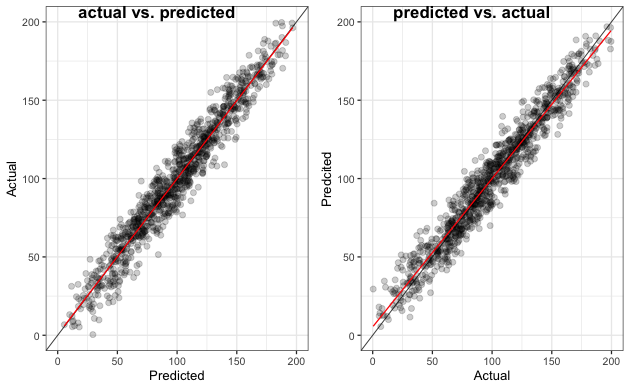
In the following, the noise level (k) was increased from 0.1, 0.5 to 1, and in each case, the linear regression was run 100 times. The intercept (model bias), slope (model consistency), and R-squared (explained variance) are compared when the predicted data are plotted on the x- and y-axes.
在下文中,噪声水平(k)从0.1、0.5增加到1,并且在每种情况下,线性回归均进行了100次。 当将预测数据绘制在x和y轴上时,将比较截距( 模型偏差 ),斜率( 模型 一致性 )和R平方( 解释的方差 )。
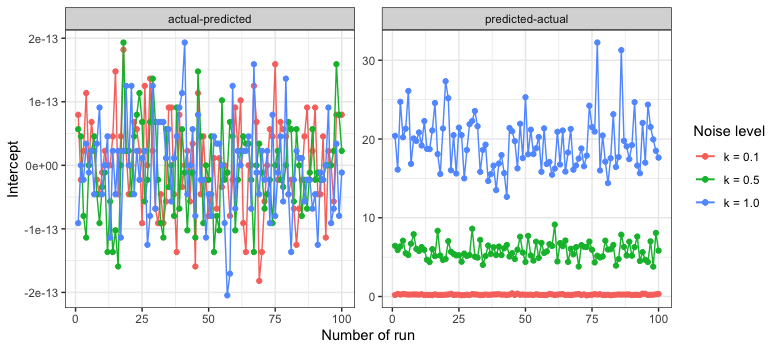
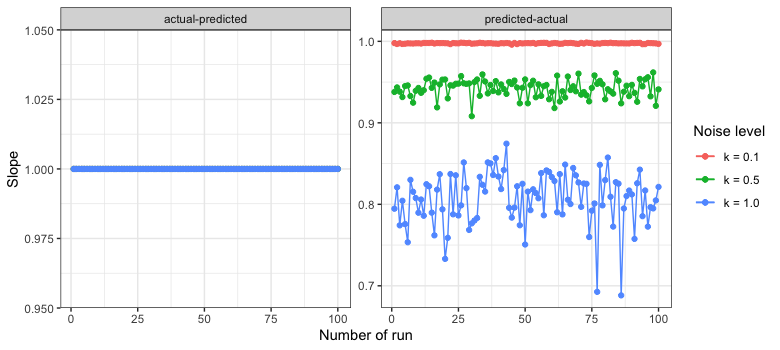
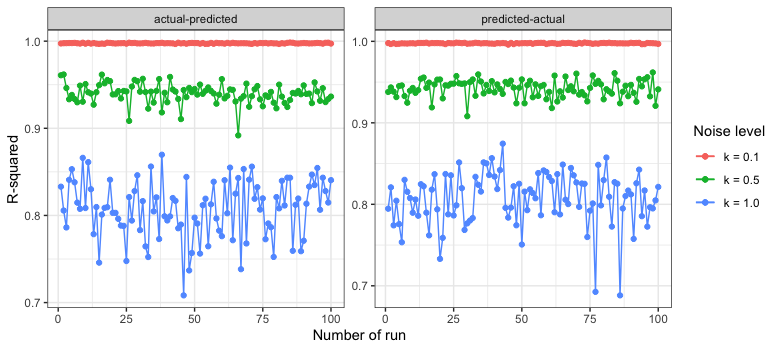
One can see that independently of the noise level, the values for the intercept and slope are respectively 0 and 1 when plotted as actual vs. predicted. On the other hand, when plotted as predicted vs. actual, the intercept increases with an increase in noise level, while the slope decreases. Hence, the distribution of the slope and intercept differs considerably between the two cases as the noise increases. However, R-squared has a similar behavior regardless of which axis the predicted data are plotted. Both exhibit almost identical distribution.
可以看到,与实际噪声和预测值相比,与噪声水平无关,截距和斜率的值分别为0和1。 另一方面,当按预测值与实际值作图时,截距随噪声水平的增加而增加,而斜率则减小。 因此,两种情况下,随着噪声的增加,斜率和截距的分布也有很大不同。 但是,R-平方具有相似的行为,而与绘制预测数据的轴无关。 两者都表现出几乎相同的分布。
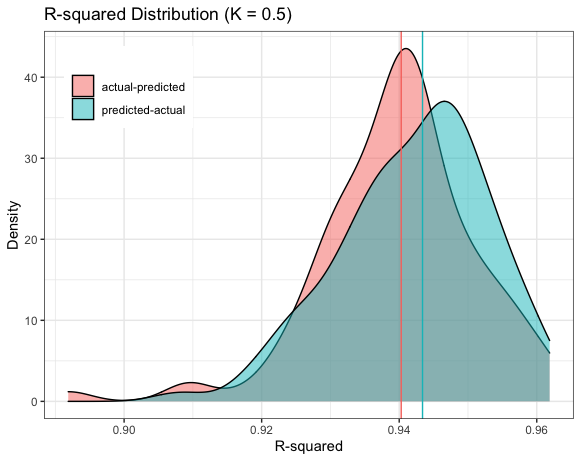
Thus, in summary, there is only one correct way to plot results from a model, which is the actual data plotted on the y-axis and the predicted data plotted on the x-axis, especially for noisy data. For any further and detailed information, the interested reader is referred to the reference below, a mathematical proof is provided by Piñeiro et al.
因此,总而言之,只有一种正确的方法可以绘制模型的结果,即在y轴上绘制的实际数据和在x轴上绘制的预测数据,特别是对于噪声数据。 对于任何进一步和详细的信息,有兴趣的读者可以参考以下参考文献,Piñeiro 等人提供了数学证明。
Reference: G. Piñeiro, S. Perelman, J. P. Guerschman, J. M. Paruelo, Ecological Modelling, How to evaluate models: Observed vs. predicted or predicted vs. observed?, 216, 2008, 316–322.
参考: G.Piñeiro,S. Perelman,JP Guerschman,JM Paruelo,生态模型, 如何评估模型:观察与预测还是预测与观察? ,216,2008,316-322。
翻译自: https://towardsdatascience.com/should-we-plot-actual-vs-predicted-predicted-vs-actual-or-it-doesnt-matter-13648ee163f5
安卓代码还是xml绘制页面
本文来自互联网用户投稿,该文观点仅代表作者本人,不代表本站立场。本站仅提供信息存储空间服务,不拥有所有权,不承担相关法律责任。如若转载,请注明出处:http://www.mzph.cn/news/388186.shtml
如若内容造成侵权/违法违规/事实不符,请联系多彩编程网进行投诉反馈email:809451989@qq.com,一经查实,立即删除!