基于plotly数据可视化
The amount of data in the world is growing every second. From sending a text to clicking a link, you are creating data points for companies to use. Insights that can be drawn from this collection of data can be extremely valuable. Every business has their own storage of data that they need to examine. One of the most important ways this examination is done is by visualizing the data.
吨他达到世界数据的增长每一秒。 从发送文本到单击链接,您正在创建供公司使用的数据点。 从这些数据收集中得出的见解可能非常有价值。 每个企业都有自己需要检查的数据存储。 进行此检查的最重要方法之一是可视化数据。
为什么要可视化数据? (Why Visualize Data?)
Simply put — “a picture is worth a thousand words”. In the entire history of business, data visualization has remained a necessary component. The reason it is so necessary is ultimately because we are visual creatures. Why else do you think a majority of us would prefer to watch a movie adaptation of a book than read the book itself? In terms of business presentations, a graph or chart of sales data may prove more insightful than just plain text. It is easy to draw insights from visual mediums rather than word documents.
简而言之-“ 一张图片值得一千个字 ”。 在整个业务历史中,数据可视化仍然是必不可少的组成部分。 之所以如此必要,是因为我们最终是视觉生物。 您为什么还认为我们大多数人宁愿看电影改编电影也不愿看书本身? 就业务演示而言,销售数据的图形或图表可能会证明比纯文本更具洞察力。 从视觉媒介而不是单词文档中汲取见解很容易。
By visualizing the data you are making the data more accessible to a wider audience. This can help draw more insights because someone else might have an insight or two that you may never have thought of. The more people that see your visualization, then the more insights can potentially be made.
通过可视化数据,您可以使更广泛的受众更容易访问数据。 这可以帮助您获得更多的见解,因为其他人可能有您可能从未想过的一两个见识。 看到您的可视化内容的人越多,则可以潜在地获得更多的见解。
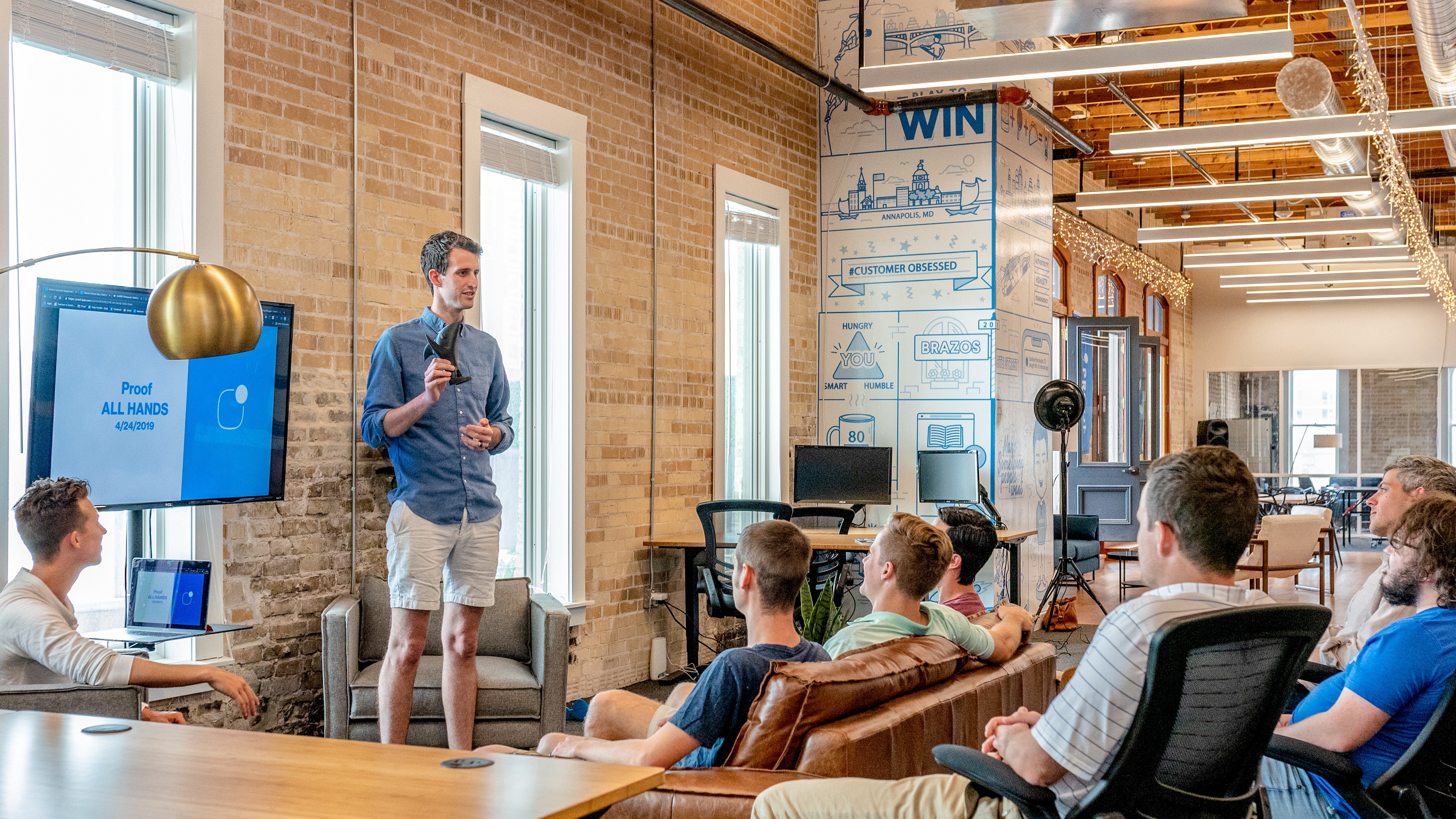
Visualizations also play a key role when presenting to crucial decision makers such as board members or shareholders. As you are constructing your numerous graphs and plots to highlight key data points, the visuals you decide to make can help push these decision makers in one direction or another. If the data visuals are presented with a select narrative in mind, then these decision makers will be inclined to make specific decisions based on your presentation.
在向关键决策者(例如董事会成员或股东)展示时,可视化也起着关键作用。 在构建大量图形和图表以突出显示关键数据点时,您决定制作的视觉效果可以帮助将这些决策者推向一个方向或另一个方向。 如果在呈现数据视觉效果时考虑了特定的叙述,那么这些决策者将倾向于根据您的呈现做出特定的决策。
数据可视化工具 (Tools for Data Visualization)
Pie charts, bar charts, line graphs, and so on are all effective visuals when presenting data. These visuals are the tried and true forms for data presentation and we have made it even easier to create them. What we once use to do by hand can now be done with a couple of clicks on a computer.
饼图,条形图,折线图等都是呈现数据时的有效视觉效果。 这些视觉效果是经过验证的真实数据展示形式,我们使创建它们变得更加容易。 现在,我们曾经手动执行的操作现在只需在计算机上单击几下即可完成。
Nowadays, we have access to multiple programs to construct beautiful looking charts and graphs. These tools range from more technically based applications of visualization like Python’s Matplotlib or Plotly to more user-friendly ones like Tableau or Microsoft Power BI. Data visualizations tools are now more accessible than ever before.
如今,我们可以使用多个程序来构建美观的图表。 这些工具的范围从更基于技术的可视化应用程序(例如Python的Matplotlib或Plotly)到更加用户友好的工具(如Tableau或Microsoft Power BI) 。 数据可视化工具现在比以往任何时候都更易于访问。
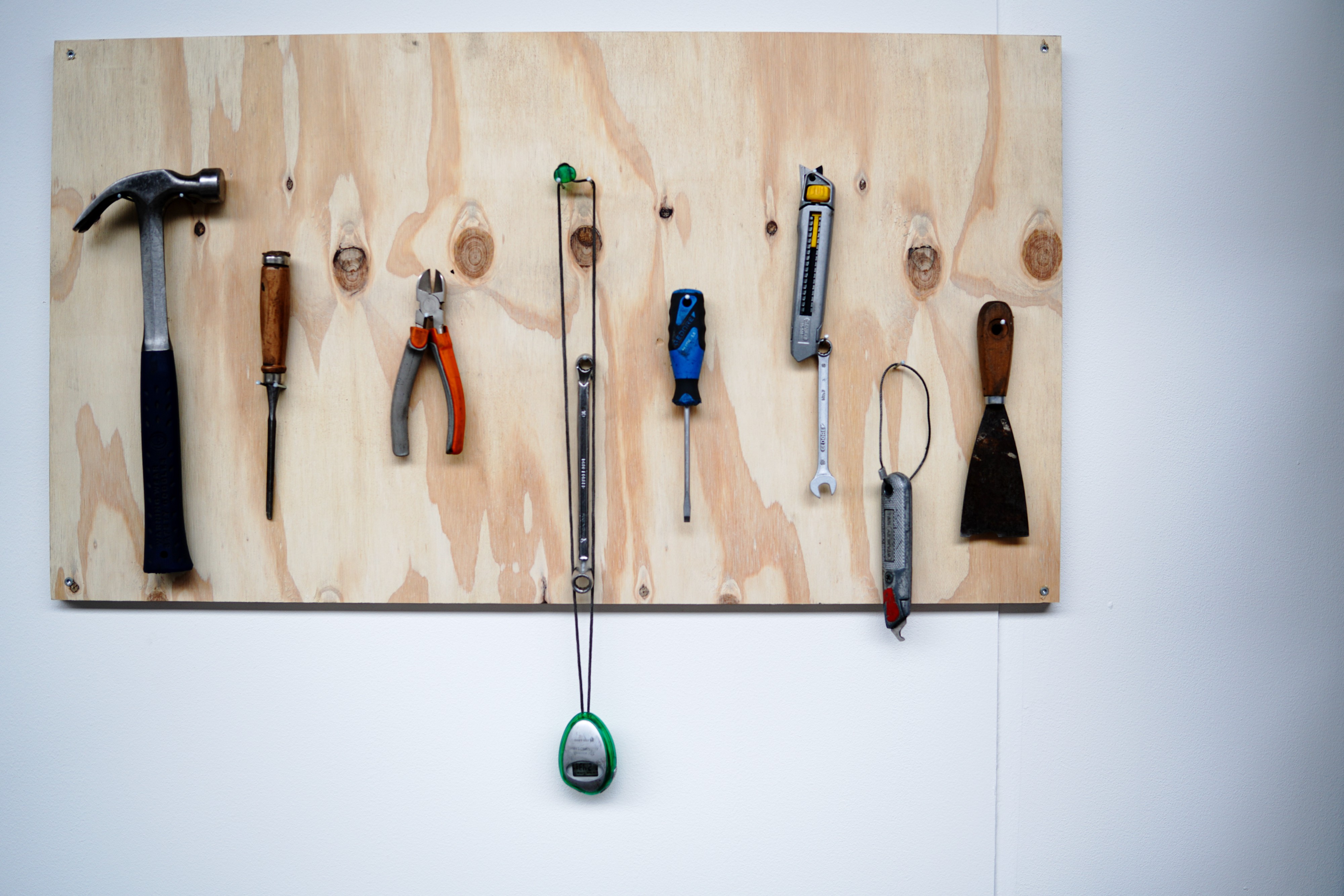
Within the realm of Python programming, there are many different libraries you could use to craft data visualizations. These libraries include, but are not limited, to Altair, Seaborn, and Plotly. There is no superior Python library because it all depends on what you are comfortable with and the problem or data you are trying to visualize.
在Python编程领域内,可以使用许多不同的库来制作数据可视化。 这些库包括但不限于Altair , Seaborn和Plotly 。 没有出色的Python库,因为这一切都取决于您是否熟悉什么以及要可视化的问题或数据。
学习如何使用情节 (Learning How to Use Plotly)
One of the tools we mentioned before is called Plotly. Plotly is a graphing and plotting library in Python similar to Matplotlib. The difference between the two is the fact that Plotly creates dynamically, interactive charts and graphs.
我们之前提到的工具之一称为Plotly 。 Plotly是Python中的绘图和绘图库,类似于Matplotlib。 两者之间的区别在于Plotly创建动态的交互式图表的事实。
一个简单的业务问题 (A Simple Business Problem)
To get started with Plotly, we will need data to graph or plot first. So let’s say for example you work for a business that sells clothing. They want you to chart the sales for their shirts and jeans over the course of one year and have provided you with the data to do so. This problem will help us begin working with Plotly.
要开始使用Plotly,我们将需要首先绘制图形或绘图的数据。 举例来说,假设您为一家销售服装的公司工作。 他们希望您绘制一年中衬衫和牛仔裤的销售图表,并提供了相关数据。 这个问题将帮助我们开始使用Plotly。
剧情安装 (Installing Plotly)
In order to begin, we must first install Plotly by using the following command in your terminal:
首先,我们必须首先在终端中使用以下命令来安装Plotly:
$ pip install plotly
Or if you have Anaconda installed:
或者,如果您安装了Anaconda :
$ conda install -c plotly plotly
导入 (Importing Plotly)
Now that you have Plotly installed, let’s open a new file and start importing the necessary libraries for our data visualization example:
现在您已经安装了Plotly,让我们打开一个新文件,并开始为数据可视化示例导入必要的库:
import plotly.express as px
import calendar as cal
import random
import pandas as pd
Here we are using plotly.express
, which is a module within Plotly that will quickly create graphs and charts for us.
在这里,我们使用plotly.express
,它是plotly.express
中的一个模块,可以为我们快速创建图形和图表。
创建数据 (Creating the Data)
Since we are not actually given real data, we will have to create our own:
由于实际上并未提供真实数据,因此我们将必须创建自己的数据:
data = {'Months': [cal.month_name[i] for i in range(1,13)],
'Shirts': [round(random.gauss(100, 15)) for _ in range(12)],
'Jeans': [round(random.gauss(50, 20)) for _ in range(12)]}
Plotly works very well with Pandas DataFrames so we will store our newly created data into a DF:
Plotly与Pandas DataFrames配合得很好,因此我们会将新创建的数据存储到DF中:
df = pd.DataFrame(data)
This new DF looks like this:
这个新的DF看起来像这样:
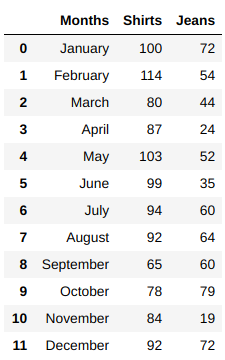
情节的条形图 (Plotly’s Bar Chart)
Now that we have our DF ready we can begin crafting our bar chart:
现在我们已经准备好DF,可以开始制作条形图了:
fig = px.bar(df,
x='Months',
y=['Shirts','Jeans'])fig.show()
Here we are using the .bar()
method and inputting the DF of our data, and specifying the x and y axes. We are crafting a stacked bar chart by making a list for the columns: ‘Shirts
’ and ‘Jeans
’. Which we’ll display by calling fig.show()
.
在这里,我们使用.bar()
方法并输入数据的DF,并指定x和y轴。 我们通过列出以下列的列表来制作堆叠式条形图:“ Shirts
”和“ Jeans
”。 我们将通过调用fig.show()
显示。
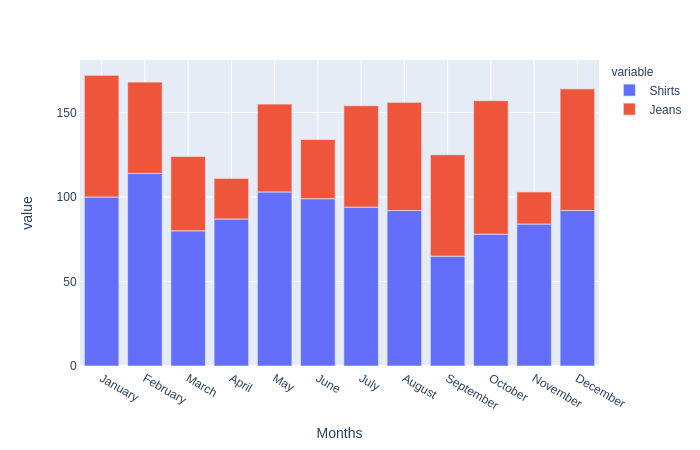
Success! That was simple enough. The cool thing about this Plotly chart is that you can start interacting with it by zooming in, panning, etc. But in regards to the overall chart, there are some things we would like to change to make this graph a little bit more descriptive like adding a title and renaming a few of the labels.
成功! 那很简单。 关于该Plotly图表,最酷的事情是您可以通过放大,平移等开始与之交互。但是对于整体图表,我们需要进行一些更改以使该图表更具描述性,例如添加标题并重命名一些标签。
fig = px.bar(df,
x='Months',
y=['Shirts','Jeans'],
title='Total Monthly Item Sales',
labels={'variable': 'Item',
'value': 'Quantity Sold (in thousands)'})fig.show()
The difference between this code and the code before is the addition of the title=
and labels={}
argument. With these new arguments we are adding in a title for the chart and under the labels
we are basically using a dictionary to replace the two current labels.
此代码与之前的代码之间的区别是添加了title=
和labels={}
参数。 通过这些新参数,我们为图表添加了一个标题,在labels
之下,我们基本上是使用字典来替换两个当前标签。
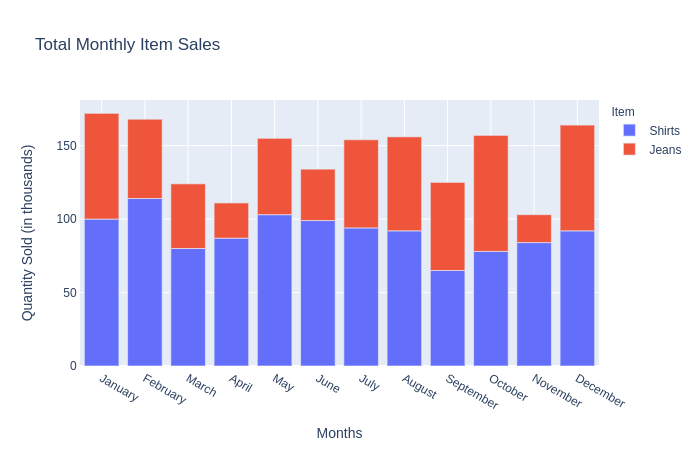
Now that the bar chart is properly labeled, we are basically finished with using Plotly for this data. But what if we wanted to do other kinds of charts or graphs in order to view different sides of the data?
现在,条形图已正确标记,我们基本上已经完成了对数据使用Plotly的操作。 但是,如果我们想做其他种类的图表来查看数据的不同方面怎么办?
情节的线图 (Plotly’s Line Graph)
Plotly allows us to create other types of visualizations too. We can easily create a line graph by using the code from before and just changing one thing:
通过Plotly,我们也可以创建其他类型的可视化。 我们可以使用之前的代码轻松地创建折线图,而只需更改一件事:
fig = px.line(df,
x='Months',
y=['Shirts','Jeans'],
title='Monthly Item Sales',
labels={'variable': 'Item',
'value': 'Quantity Sold (in thousands)'})fig.show()
All we did here was change px.bar
to px.line
. This now displays the following:
我们在这里所做的只是将px.bar
更改为px.line
。 现在将显示以下内容:
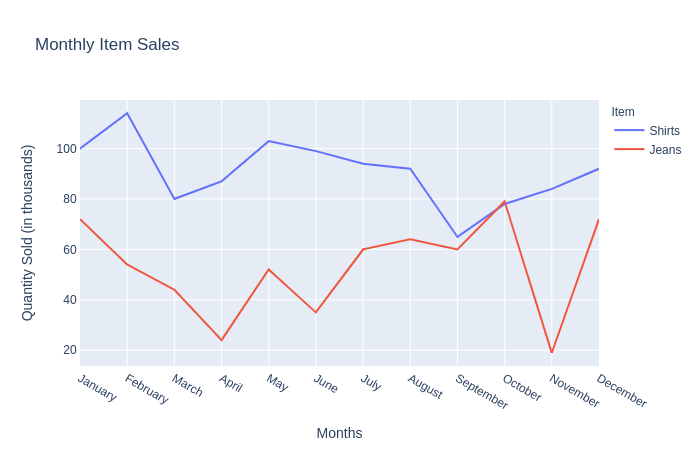
Now we have a line graph! But wait there’s more…
现在我们有了折线图! 但是,等等……
情节饼图 (Plotly’s Pie Chart)
Let’s say we wanted to compare how many shirts were sold vs how many jeans were sold in the entire year.
假设我们要比较全年售出的衬衫数量与牛仔裤的销售数量。
First, we must change our data to show the total sum of all sales for shirts and jeans:
首先,我们必须更改数据以显示衬衫和牛仔裤的所有销售总额:
pie_df = df[['Shirts','Jeans']].sum()
Here we’re just getting the sum of both Shirts
and Jeans
from the DF. Then, we will need to use px.pie()
using our new summed up DF.
在这里,我们只是从DF中获取Shirts
和Jeans
的总和。 然后,我们需要使用新的汇总DF使用px.pie()
。
fig = px.pie(values=pie_df.values,
names=pie_df.index,
title="Sales Percentage in a Year")fig.show()
The argument values
is used to determine the sizes of each portion of the pie chart. The names
are the labels for each of the portions.
参数values
用于确定饼图各部分的大小。 names
是每个部分的标签。
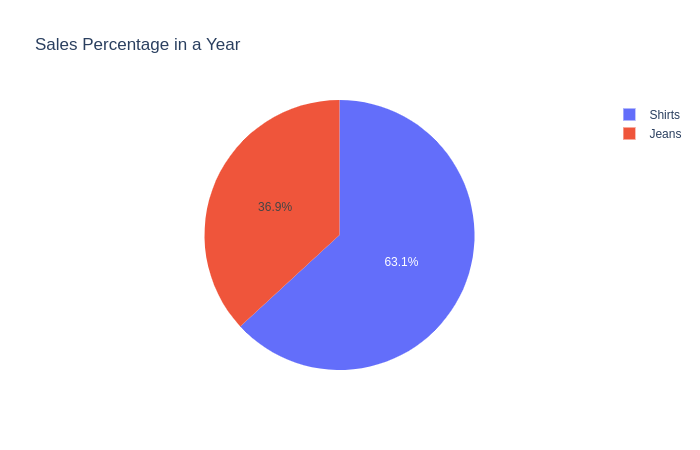
Awesome! Now we have created three different types of visualizations for our data. But you don’t have to stop — there are more options available (see here for more) if you feel the need to continue experimenting with Plotly.
太棒了! 现在,我们为数据创建了三种不同类型的可视化。 但是您不必停下来-如果您有需要继续尝试Plotly的权利,可以使用更多选项( 有关更多信息, 请参见此处 )。
数据见解和结论 (Data Insights and Conclusions)
After visualizing our data, we would need to come to some sort of insight or conclusion based on the visuals. What can you tell based on these charts? Are there some obvious conclusions that can be drawn? What about some not so obvious ones?
可视化我们的数据后,我们需要根据视觉效果得出某种见解或结论。 您可以根据这些图表看出什么? 是否可以得出一些明显的结论? 那些不太明显的东西呢?
Anyways, insights and conclusions are easier to see rather than read. If you are still wondering about the importance of visualizations, then just take a look back at the DF we created and compare it to any of the visuals we created with Plotly. Sometimes reading information is not as good as seeing the information.
无论如何,见解和结论更容易看到而不是阅读。 如果您仍然想知道可视化的重要性,那么只需回顾一下我们创建的DF,并将其与我们使用Plotly创建的任何可视化图像进行比较。 有时阅读信息不如阅读信息。
翻译自: https://towardsdatascience.com/how-to-use-plotly-for-data-visualization-f3d62bbcfd92
基于plotly数据可视化
本文来自互联网用户投稿,该文观点仅代表作者本人,不代表本站立场。本站仅提供信息存储空间服务,不拥有所有权,不承担相关法律责任。如若转载,请注明出处:http://www.mzph.cn/news/388164.shtml
如若内容造成侵权/违法违规/事实不符,请联系多彩编程网进行投诉反馈email:809451989@qq.com,一经查实,立即删除!