医疗大数据处理流程
Note: the fictitious examples and diagrams are for illustrative purposes ONLY. They are mainly simplifications of real phenomena. Please consult with your physician if you have any questions.
注意:虚拟示例和图表仅用于说明目的。 它们主要是真实现象的简化。 如有任何疑问,请咨询您的医生。
Scale is one of the main challenges in public health services. Specialized treatments are hard to track when applied to thousands of patients. Fortunately, we can now identify bottlenecks and errors in the flow of these processes at scale. Through the combination of Process Modelling and Probabilistic Modelling, precision medicine and stratified healthcare has achieved state-of-the-art results like parallel process consolidation, task connections, and task pruning.
规模是公共卫生服务的主要挑战之一。 当应用于数以千计的患者时,难以追踪专业治疗。 幸运的是,我们现在可以大规模地识别这些流程中的瓶颈和错误。 通过将过程建模和概率建模相结合,精密医学和分层医疗保健已取得了最新成果,例如并行过程合并,任务连接和任务修剪。
Process modeling helps structure a series of steps to perform a task.
流程建模有助于构造一系列步骤以执行任务。
In the real world, tasks can run in parallel or series. Processes can have loops, divergent and convergent paths, and unnecessary steps.
在现实世界中,任务可以并行或串行运行。 流程可以具有循环,分歧和收敛的路径以及不必要的步骤。
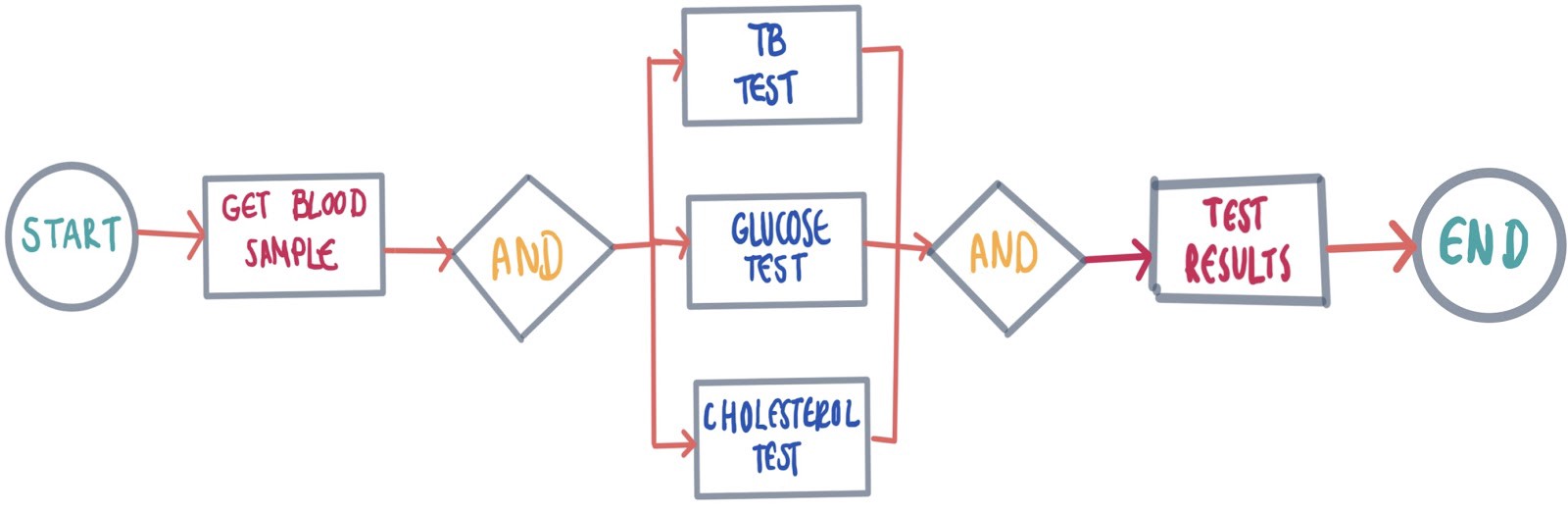
Process modeling discovers such complexities and improves upon them. Improving our process models is important since the main objective of stratified healthcare and precision medicine is to achieve an individual, precise and complete treatment for each patient. Stratified healthcare refers to the practice of offering a robust infrastructure to treat patients, while precision medicine seeks to improve the health of individual patients through personalized treatments.
流程建模发现了这种复杂性并对其进行了改进。 改进我们的过程模型非常重要,因为分层医疗保健和精密医学的主要目标是为每位患者提供个性化,精确和完整的治疗。 分层医疗保健是指提供强大的基础设施来治疗患者的实践,而精密医学则致力于通过个性化治疗来改善单个患者的健康。
Personalized treatments are a reality, thanks to the Human Genome Project. The Human Genome Project demonstrates that no human is made the same. Therefore, prescriptions and treatments cause different reactions in each individual. Modeling processes allow us to reflect this reality in paper. However, this method is not perfect.
得益于人类基因组计划,个性化治疗成为现实。 人类基因组计划表明,没有任何一个人类是一样的。 因此,处方和治疗在每个人中引起不同的React。 建模过程使我们能够在纸上反映这一现实。 但是,这种方法并不完美。
While processes in health go through rigorous statistical tests, they are by no means rid of bias. This bias can impact the flow of steps or parallelism that could be applied to optimize positive outcomes or the speed of the treatments. Additionally, the tools and tests in operations and treatments evolve with time, which shortens steps or reduces the time overhead in these tasks. Updating each process model every time a breakthrough is validated by experts consumes a lot of time.
尽管健康过程经过严格的统计检验,但绝不能消除偏见。 这种偏见会影响可用于优化阳性结果或治疗速度的步骤或并行性流程。 此外,手术和治疗中的工具和测试会随着时间而发展,从而缩短了步骤或减少了这些任务的时间开销。 每当专家验证突破性进展时,更新每个流程模型都会花费大量时间。
For example, imagine we currently have these personalized brain imaging processes for 5 patients with a brain tumor:
例如,假设我们目前有针对5位脑肿瘤患者的以下个性化大脑成像过程:
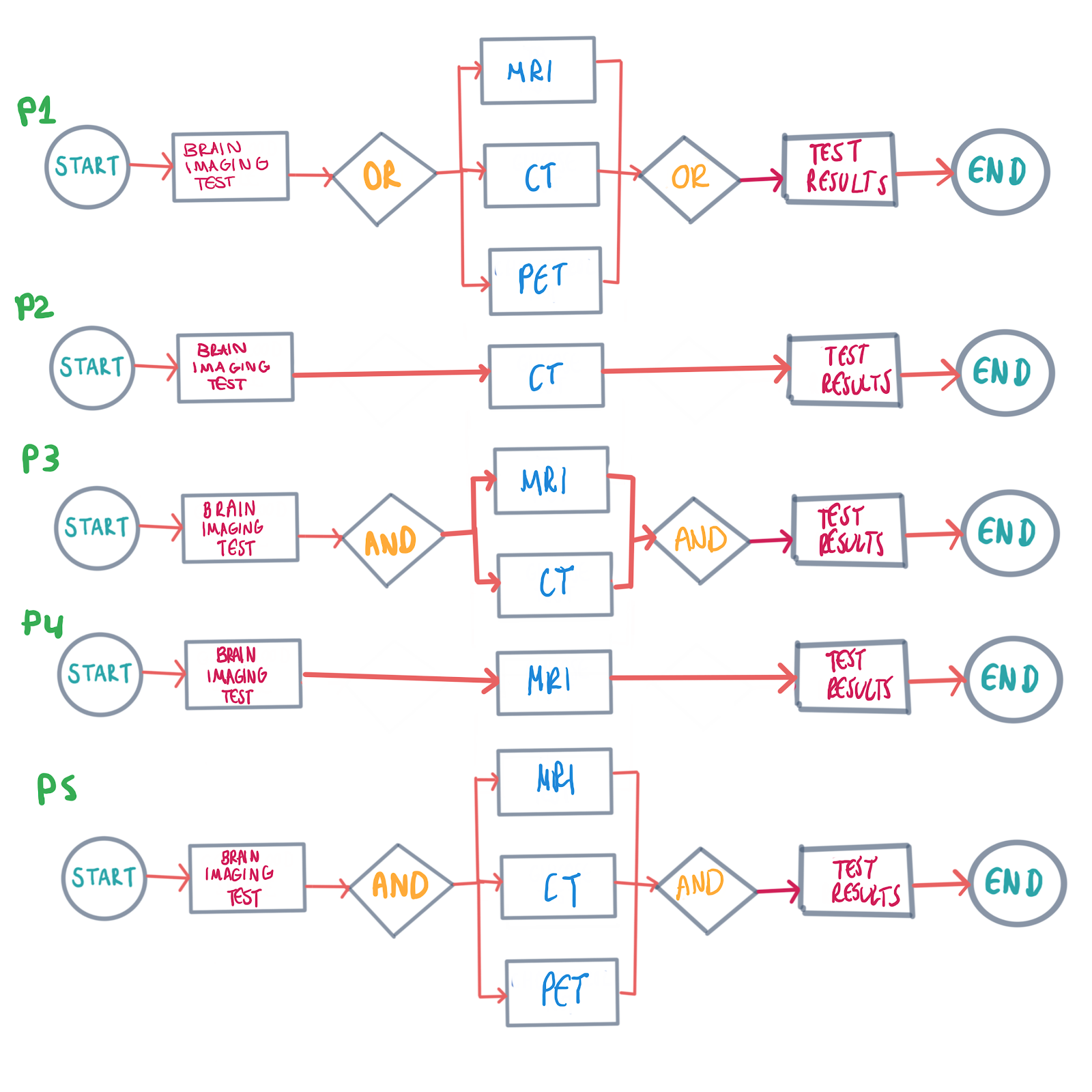
Let’s say we have a new technology called BrainImagingx2, which scans a patient’s tumor size two times faster than the current brain imaging technology, but it’s only precise for patients that can handle both MRI and CT scans periodically:
假设我们拥有一项名为BrainImagingx2的新技术,该技术可扫描患者的肿瘤大小,其速度是当前脑部成像技术的两倍,但这仅适用于可同时处理MRI和CT扫描的患者:
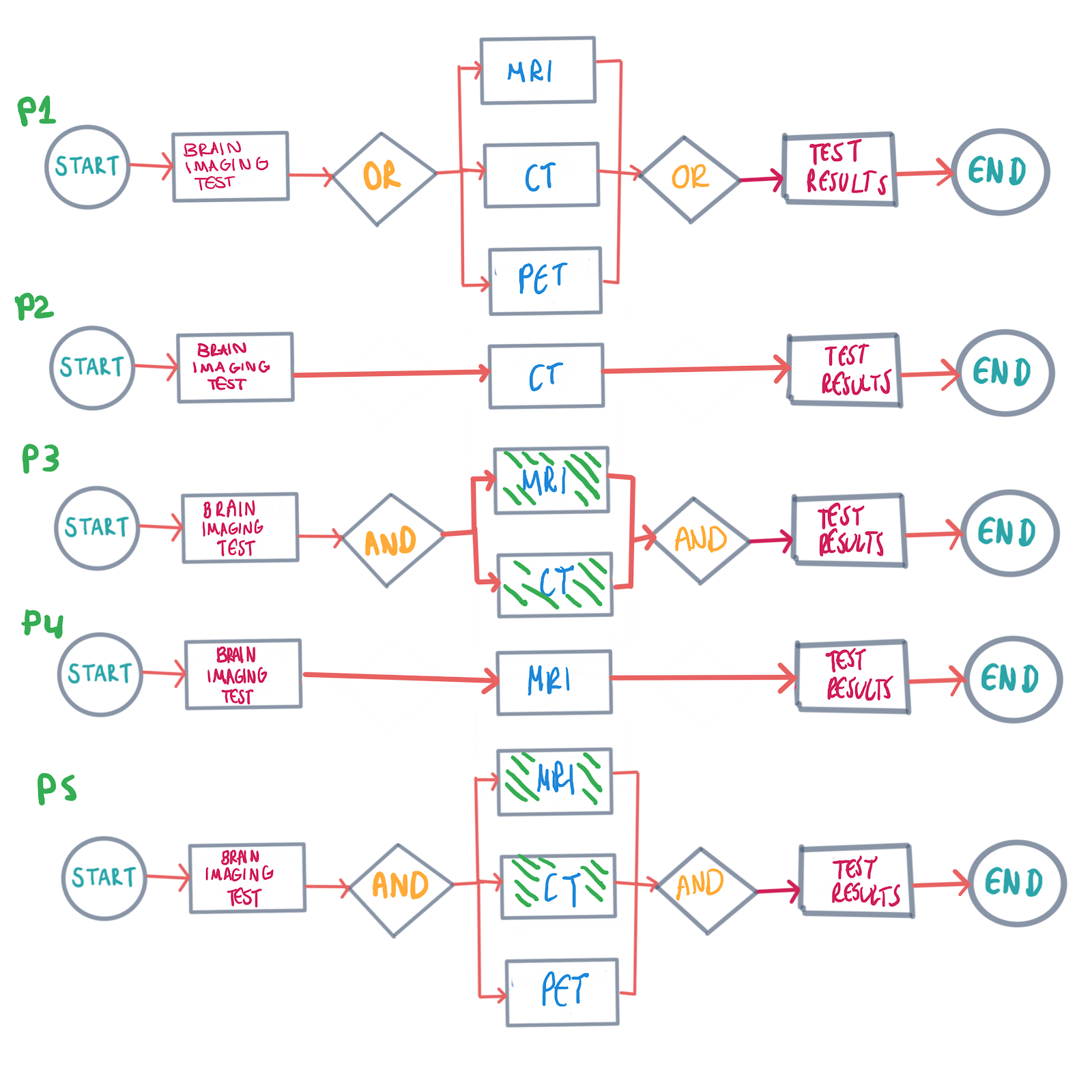
Naturally, we want to update our 5 model processes. This might be easy to do since we’re dealing with only 5 patients at this time. We can review each case manually to evaluate the risk of updating the process model and weigh it against the benefit:
自然,我们要更新5个模型流程。 这可能很容易实现,因为我们目前仅处理5名患者。 我们可以手动检查每个案例,以评估更新流程模型的风险,并将其与收益进行权衡:
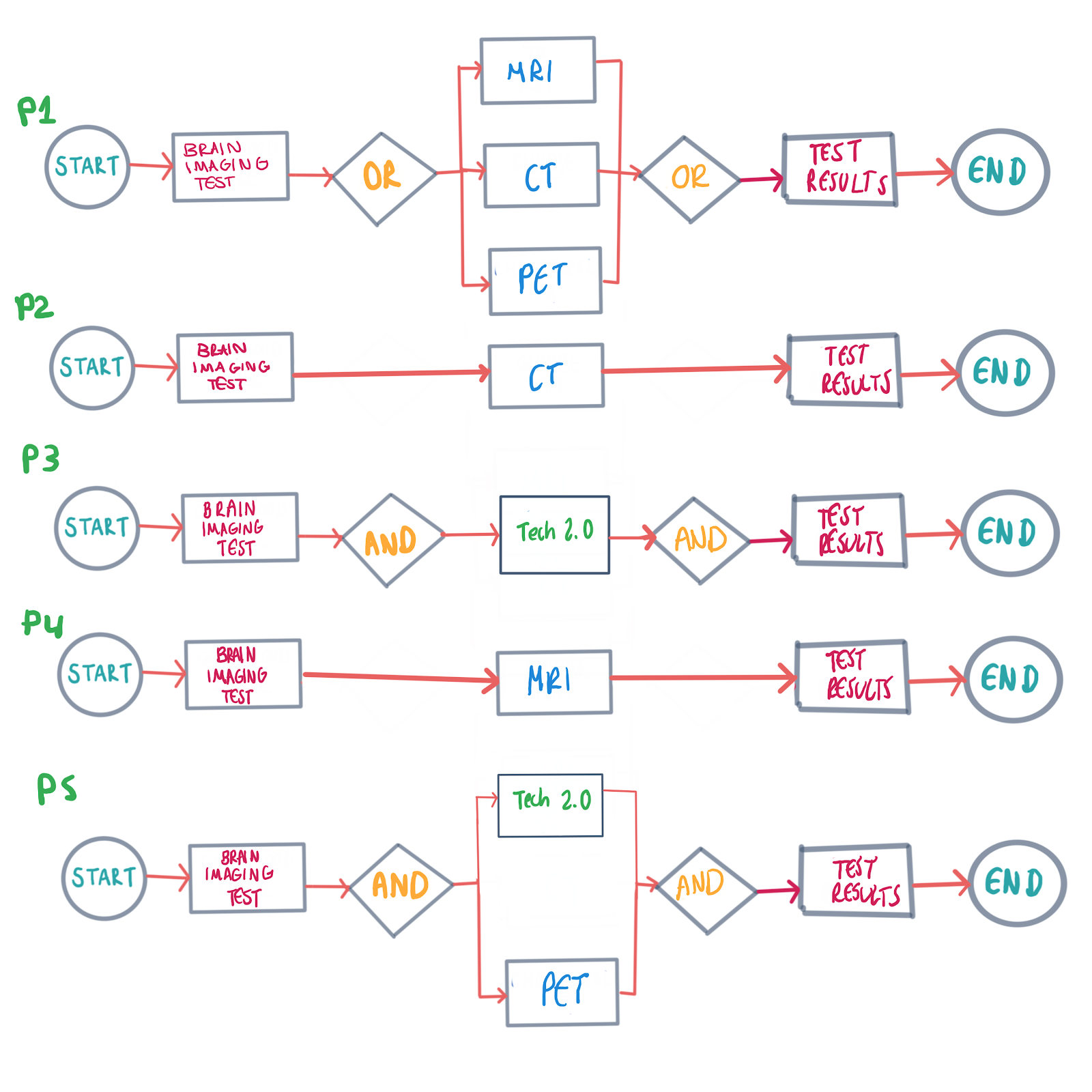
But what happens if suddenly our 5 patients turn into 1,000 different and unique patients, each with their medical history and background? The cost of evaluating each process model manually skyrockets. Even worse, maybe one of our 1,000 patients has a great risk of damage if exposed to the new technology in BrainImagingx2, like electromagnetic fields. Under these conditions, we can’t generalize or skip updating models for time and effort optimization. In summary, process modeling’s key issue is time and effort from analyzing existing models thoroughly and updating models with time. This gets worse when the number of patient profiles, and therefore the processes modeled increase with scale.
但是,如果我们的5名患者突然变成1000名不同且独特的患者,每个患者都有其病史和背景,会发生什么? 手动评估每个流程模型的成本激增。 更糟糕的是,如果暴露于BrainImagingx2的新技术(例如电磁场)中,我们的1000名患者中可能会有一个遭受损坏的风险很高。 在这种情况下,我们无法概括或跳过更新模型以节省时间和精力。 总而言之,流程建模的关键问题是从彻底分析现有模型并随时间更新模型中所花费的时间和精力。 当患者档案的数量增加时,情况变得更糟,因此建模的过程随规模的增加而增加。
Process mining achieves an automated “understanding” of the processes through the analysis of event logs and expert reviews to approximate processes. Rather than attempting to update existing models, it builds models from the data it is fed. The process of generating updated process models is thus faster. However, it’s not a perfect approach. Process mining outputs a greater number of personalized healthcare models. However, generalization or aggregation can still happen since most process mining techniques rely on process analysis, which automatically filters, sorts and compresses the logs fed to the process. We need to avoid generalizing the aggregation of our information to not obscure the edge case illnesses and response our patients could have as a result of their unique genetic composition like we discussed as a fact through the breakthrough of the Human Genome Project.
流程挖掘通过事件日志分析和专家评审来近似流程,从而实现流程的自动化“理解”。 与其尝试更新现有模型,不如从馈送的数据构建模型。 因此,生成更新的过程模型的过程更快。 但是,这不是一个完美的方法。 流程挖掘输出了大量个性化的医疗保健模型。 但是,由于大多数流程挖掘技术都依赖于流程分析,因此一般化或聚合仍然可能发生,流程分析会自动过滤,分类和压缩馈送到流程的日志。 我们需要避免概括我们的信息汇总,以免掩盖边缘病例的疾病和患者由于其独特的遗传组成而可能产生的React,就像我们通过人类基因组计划的突破所讨论的那样。
Another limitation to take into account is that this is an automated method that relies on data, and the quality of the process models exported is directly related to the quality of the information it is fed. The combined data of patients around the world have variance and noise. The different scales (the what), and sometimes even standards of the measurements (the how) impact what we see. Additionally, Electronic Health Records are not always available across the world, so inconsistencies between global records are highly likely. Missing data greatly degrades the quality of these models. Language disparity obscures the understanding learned through these data mining-based approaches.
要考虑的另一个限制是,这是一种依赖数据的自动化方法,并且导出的流程模型的质量直接与其所馈送信息的质量有关。 世界各地患者的综合数据存在差异和噪音。 不同的比例(什么),有时甚至是度量标准(如何)也会影响我们所看到的。 此外,电子病历在世界范围内并不总是可用的,因此全球病历之间很可能存在不一致之处。 数据丢失会大大降低这些模型的质量。 语言差异掩盖了通过这些基于数据挖掘的方法学到的理解。
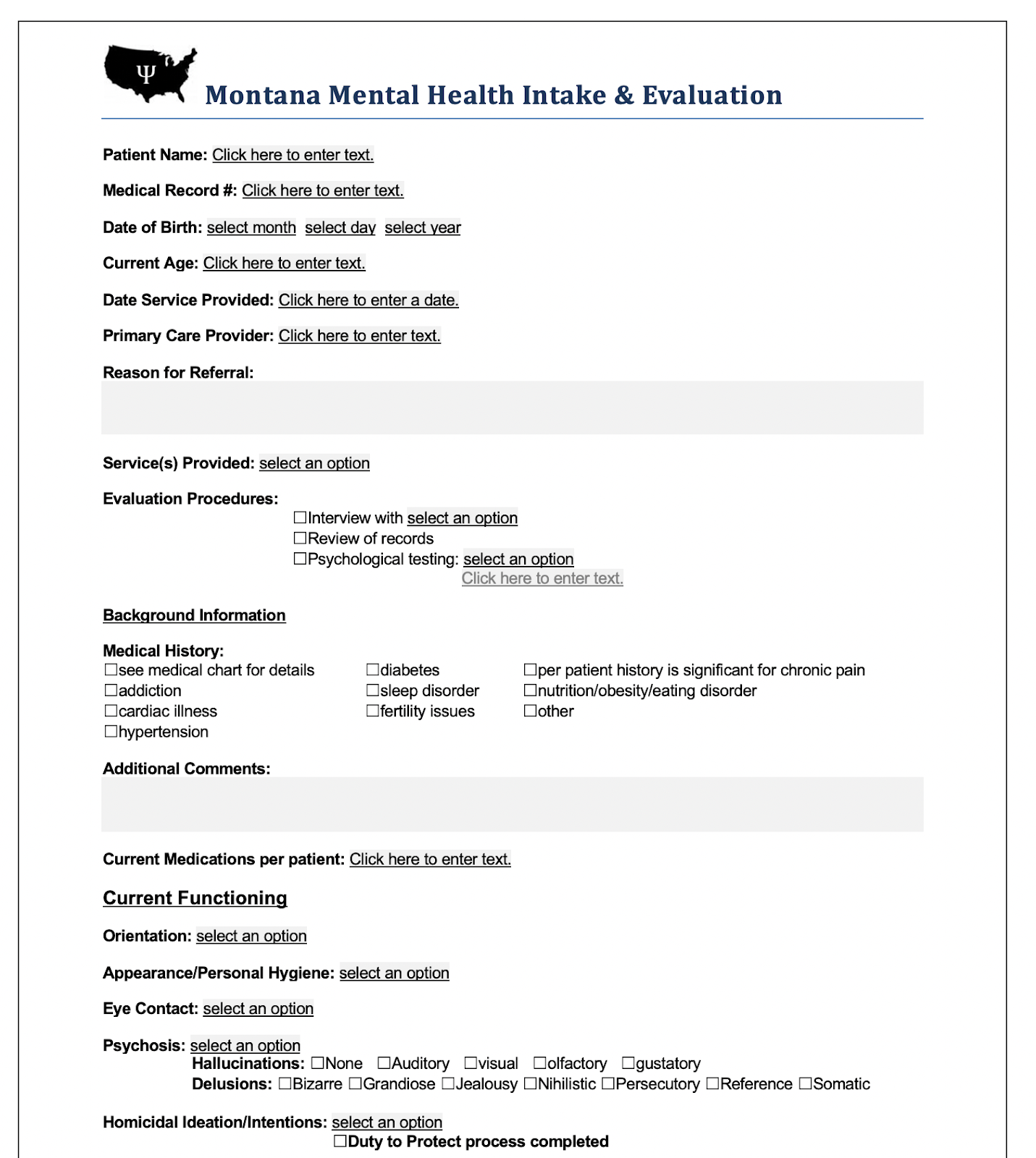
Source: https://www.apadivisions.org/division-31/publications/records/intake
资料来源: https : //www.apadivisions.org/division-31/publications/records/intake
We can combine Process Modelling, namely Process Mining, with Probabilistic Modelling to better mitigate these issues. Probabilistic modeling takes uncertainty into account, treating it as noise. A probabilistic model gives a distribution of possible outcomes. By modeling outcomes as likelihoods, the uncertainty is quantified to be further addressed and corrected by statistical methods.
我们可以将过程建模(即过程挖掘)与概率建模相结合,以更好地缓解这些问题。 概率建模将不确定性考虑在内,将其视为噪声。 概率模型给出了可能结果的分布。 通过将结果建模为可能性,可以量化不确定性,以便通过统计方法进一步解决和纠正。
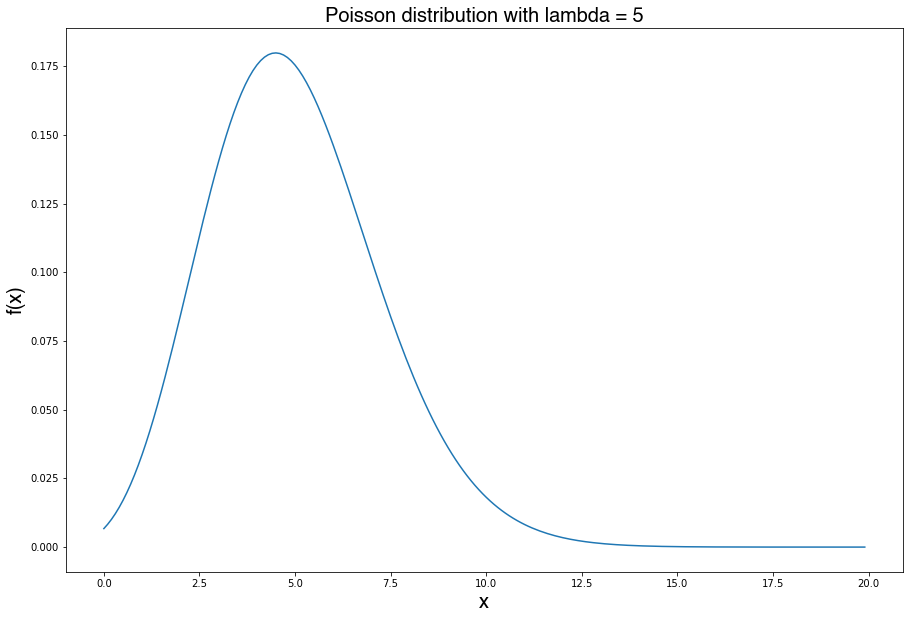
Probabilistic Modelling assumes a relationship between the outcome and the independent variable, however, this is something that needs to be proven. Correlation coefficients and regression analysis are used to perform hypothesis tests. While correlation measures the degree of association between two variables, other methods like regression measures the predictive power of the independent variable should the relationship be represented by a mathematical model. Probabilistic methods assume a model to account for noise and to make inferences based on these models.
概率建模假设结果与自变量之间存在关系,但是,这需要加以证明。 相关系数和回归分析用于执行假设检验。 虽然相关性度量两个变量之间的关联度,但其他方法(如回归)可以度量自变量的预测能力(如果该关系由数学模型表示)。 概率方法假设一个模型来考虑噪声并基于这些模型进行推断。
To combine both Process Modelling and Probabilistic Modelling, we can represent similar healthcare processes through a single diagram, which is the result of grouping similar patient task histories. In this diagram, each state transitions to multiple states. Each transition has a probability based on the data that was grouped. This way, even if the models are inconsistent with reality, they can be reviewed easily.
结合过程建模和概率建模,我们可以通过单个图表表示相似的医疗过程,这是对相似的患者任务历史进行分组的结果。 在此图中,每个状态都转换为多个状态。 每个转换都有一个基于分组数据的概率。 这样,即使模型与现实不一致,也可以轻松地对其进行检查。
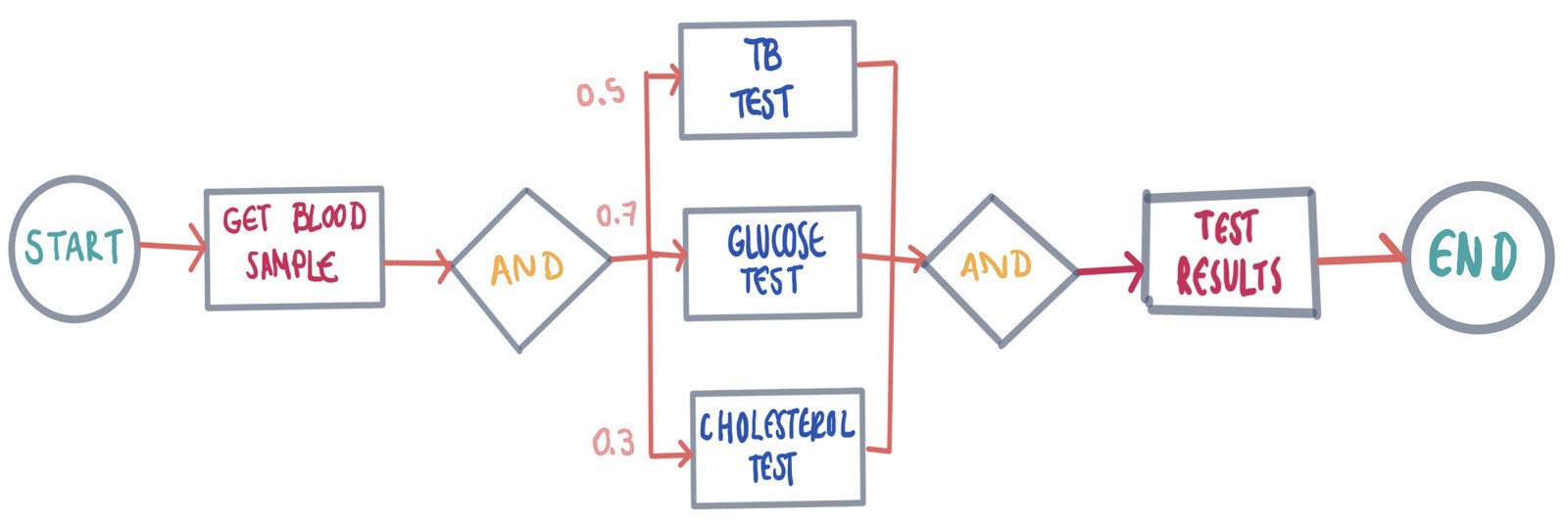
Additionally, statistical methods probability distribution fitting can be used to find the distribution that best fits the process modeled. Some examples of these distributions include the Poisson distribution for discrete variables like the task of vaccinating a patient and the Exponential and Gamma distributions for continuous variables like the process of assessing the levels of cholesterol in the body of a patient. Systems with queues, like those of a coffee shop, a computer server, and more specifically a health clinic can be modeled as probabilities through the theory of Queuing Systems, which incorporate the aforementioned probability distributions. By fitting a distribution, you can determine to which degree the outcomes in your processes are due to chance or not, which helps trim down or update the tasks in processes that matter in healthcare.
此外,可以使用统计方法概率分布拟合来找到最适合建模过程的分布。 这些分布的一些示例包括离散变量的Poisson分布(如为患者接种疫苗的任务)和连续变量的指数和Gamma分布(如评估患者体内胆固醇水平的过程)。 可以通过排队系统的理论将带有队列的系统(例如咖啡店,计算机服务器的系统,更具体地说是健康诊所的系统)建模为概率,该系统结合了上述概率分布。 通过拟合分布,您可以确定过程中的结果是否由于偶然而导致,这有助于缩减或更新对医疗保健至关重要的过程中的任务。
Some of the latest applications of Probability Modelling in Process Modelling include dealing with invisible prime tasks through rules and equations utilizing the probability of state transition of Coupled Hidden Markov and double time-stamped in event logs. Invisible prime tasks are usually run in parallel, and it’s hard to map this parallelism from raw logs back to XOR or AND gates in the diagrams without manual reviews. Through Coupled Hidden Markov Models, “wherein all of states from each Hidden Markov Model are dependent on the states of all Hidden Markov Model in previous time slice”, mapping logs back to process diagrams can now include such parallel tasks. (Sarno & Sungkono, 2016)
流程建模中概率建模的一些最新应用包括通过规则和方程式处理不可见的主要任务,这些规则和方程式利用耦合隐马尔可夫状态转换的概率和事件日志中的双重时间戳记。 看不见的主要任务通常并行运行,并且在没有人工检查的情况下,很难将这种并行性从原始日志映射回图中的XOR或AND门。 通过耦合隐马尔可夫模型,“其中每个隐马尔可夫模型的所有状态都取决于先前时间片中所有隐马尔可夫模型的状态”,映射回流程图的流程现在可以包括此类并行任务。 (Sarno和Sungkono,2016)
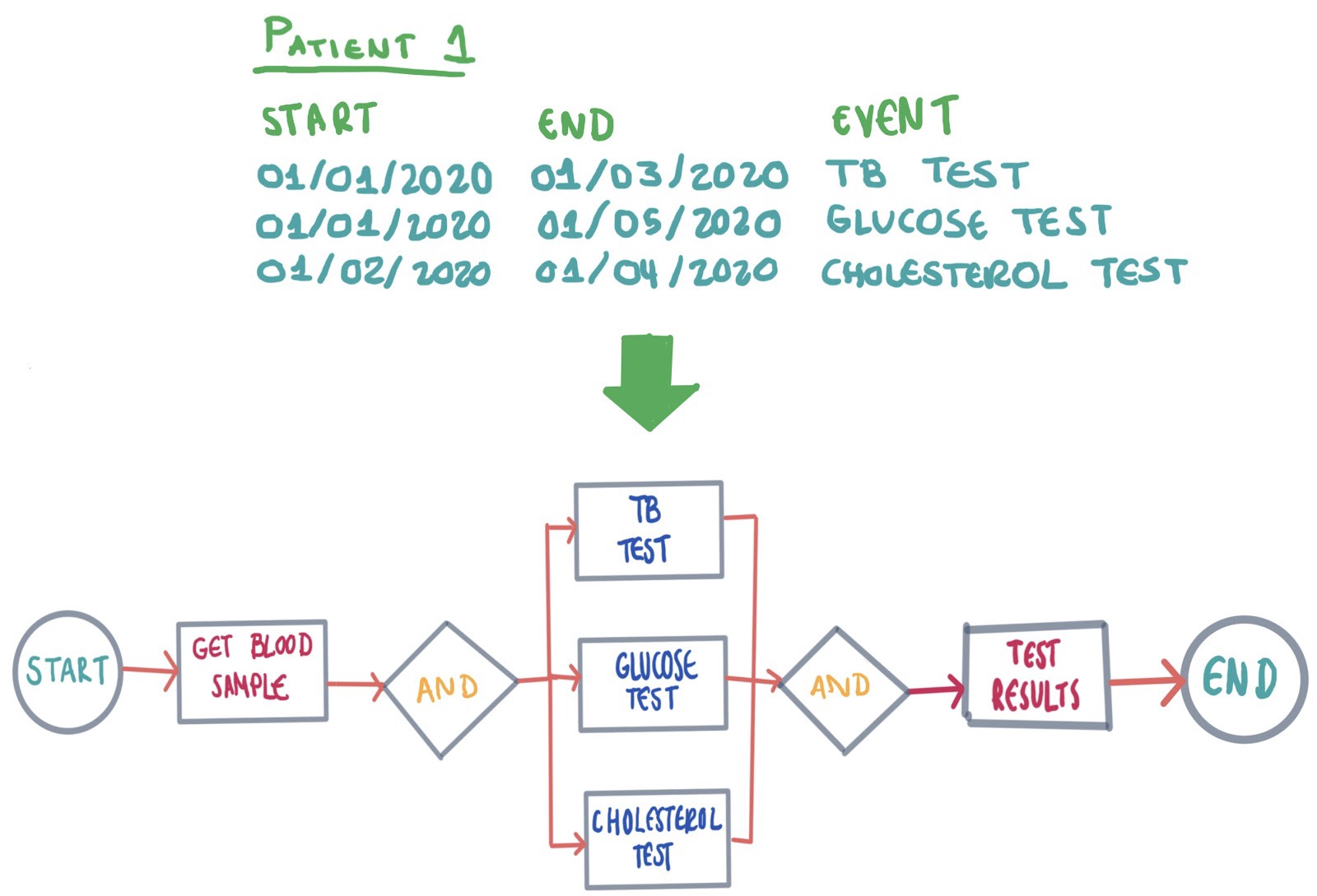
New learning methods for process mining based on probability modeling include using a logistic regression model for the discovery of direct connections between events in event logs with noise. (Maruster et al., 2002) Logistic regression addresses noise by treating the occurrence of events as a binary outcome calculated with the independent variable mapped to the sigmoid function, that for extreme positive values still gives a 1 and for extreme negative values still gives a 0. Even so, this function exports a probability as a continuous variable which allows us to show experts the probability of connecting events A and B, allowing further assessment if needed.
基于概率模型的过程挖掘的新学习方法包括使用逻辑回归模型来发现带有噪声的事件日志中事件之间的直接联系。 (Maruster et al。,2002)Logistic回归通过将事件的发生视为二进制结果来处理噪声,该结果使用映射到S型函数的自变量进行计算,对于极高的正值仍然给出1,对于极度的负值仍然给出1。 0。即使这样,此函数也将概率导出为连续变量,这使我们可以向专家显示将事件A和B联系起来的概率,并在需要时进行进一步评估。
There’s a classic machine learning example that illustrates the limitations of using probabilistic methods in process mining: you can’t say a cancer test classifier is good enough if it predicts that someone doesn’t have cancer 90% of the time. This applies to models learned through Probabilistic Modelling in Process Mining. If you have event logs that indicate that 90% of the time after a test you should give a negative result for cancer, what does this probability mean? Let’s assume for a second that we didn’t know what cancer was and decided that under an arbitrary threshold, and that we were looking to prune out steps in our process to invest more money in those with higher probability. What would happen if we decided to invest more money in the pathway of the process for negative cancer cases, instead of positive cancer cases? Interpreting the probabilities and steps requires a good understanding of their evaluation and implementation. To perform this automatically is a challenge that still needs to be addressed for clinical processes.
有一个经典的机器学习示例,说明了在过程挖掘中使用概率方法的局限性:如果癌症测试分类器可以预测某人90%的时间未患癌症,则不能说它足够好。 这适用于通过过程挖掘中的概率建模学习的模型。 如果您有事件日志表明在测试后90%的时间您应该给出癌症阴性结果,那么这种可能性意味着什么? 让我们假设一秒钟,我们不知道癌症是什么,并决定在一个任意阈值下进行,并且我们正在寻找简化过程的步骤,以便将更多的资金投资于那些可能性更高的患者。 如果我们决定在阴性癌症病例而不是阳性癌症病例的治疗过程中投入更多的资金,将会发生什么? 解释概率和步骤需要对它们的评估和实施有很好的理解。 要自动执行此操作是一项挑战,临床过程仍需要解决。
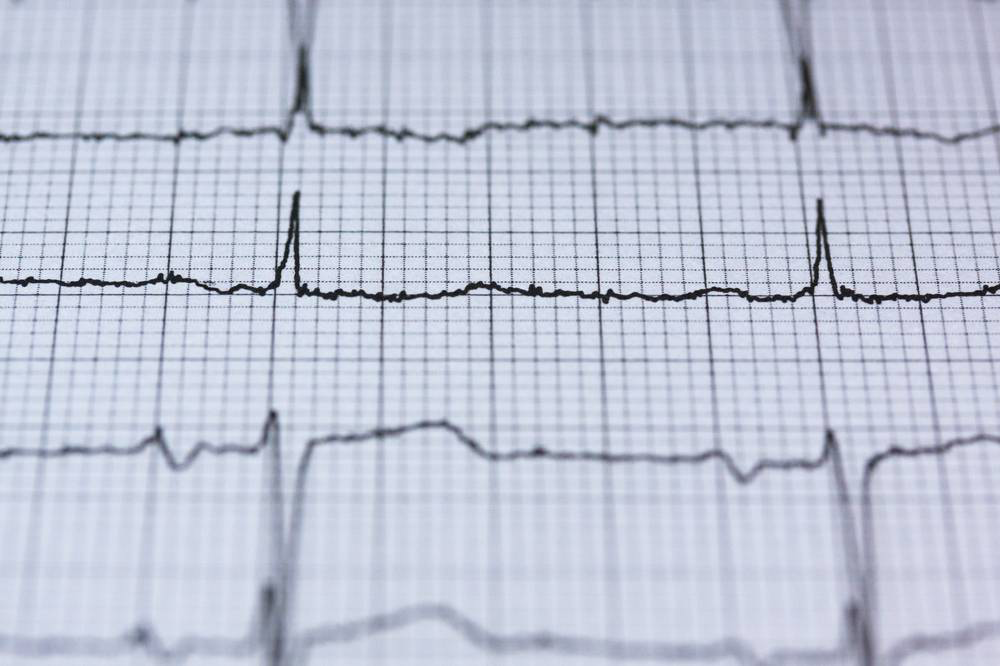
Does correlation imply prediction? The relationships found between the components of a process diagram may not be true, they may as well be false negatives. For example, if you find two consecutive event logs for a patient where one is the patient taking medication and the next one is the sickness getting worse, just looking at these isolated logs could draw the naive conclusion that the medication is to blame. However, this may not be the case. If more event logs, both in the past and the future were taken into account, effects of. That’s why correlation and regression alone are not enough to solve these cases. A potential solution for this is the use of probability chains such as Markov Chains and their extensions, that allow the probability of an event to be traced back to an earlier event than its immediate predecessor.
相关性暗示预测吗? 在流程图的各个组件之间发现的关系可能不是真实的,也可能是假阴性。 例如,如果您发现一个患者的两个连续事件日志,其中一个是正在服药的患者,下一个是病情加重,那么仅查看这些孤立的日志可能会得出天真的结论,那就是应归咎于药物。 但是,事实并非如此。 如果考虑更多的事件日志(过去和将来)的影响。 这就是仅相关性和回归不足以解决这些情况的原因。 一种可能的解决方案是使用概率链,例如马尔可夫链及其扩展,这种概率链可将事件的概率追溯到比其前身更早的事件。
Health information is highly sensible. It is a snapshot of the user’s medical, pathological, nutritional, and psychological history. In a world surrounded by taboos for mental illness and HIV, this information needs to be handled with utmost care. Patients are served so that their information is solely used for their recovery and rarely to be sold to third parties in a game of profit.
健康信息非常明智。 它是用户的医疗,病理,营养和心理史的快照。 在一个充满精神疾病和艾滋病毒禁忌的世界中,需要非常谨慎地处理这些信息。 为患者提供服务,以便他们的信息仅用于恢复,很少在赢利游戏中出售给第三方。
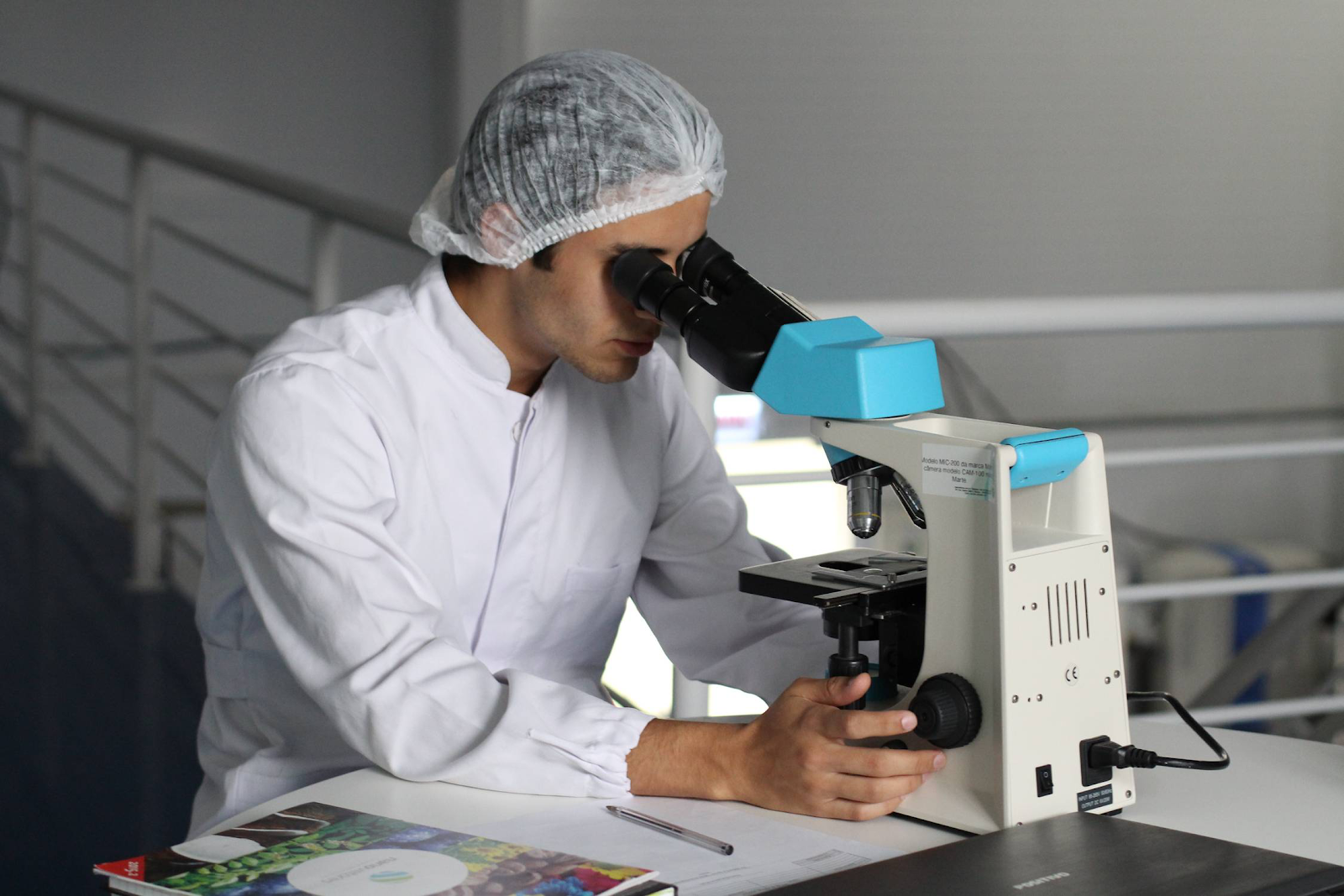
Process Modelling and Probabilistic Modelling methods aggregate from individual cases to generalize and personalize during treatments when needed. No matter how promising the results look, we need to understand the potential edge cases and caveats of the methods used. For example, the classic photo classification example classified pictures of people of color as monkeys because of the underrepresentation of their skin color in the data gathered for its training. In health, this can lead to a misunderstanding from our models for things like HIV, where most data collected have been documented for the queer community, especially gay men.
过程建模和概率建模方法从各个案例中汇总,以便在需要时在治疗期间进行概括和个性化。 无论结果看起来多么有希望,我们都需要了解所使用方法的潜在优势和注意事项。 例如,经典的照片分类示例将有色人种的图片归类为猴子,这是因为在为其训练收集的数据中皮肤颜色的表示不足。 在健康方面,这可能会导致我们对诸如艾滋病毒之类的模型产生误解,在该模型中,大多数收集到的数据已记录在酷儿社区,尤其是男同性恋者。
https://www.researchgate.net/profile/Bouchra_Marzak/publication/306046482_Clustering_in_Vehicular_Ad-Hoc_Network_Using_Artificial_Neural_Network/links/5a0c19f0a6fdccc69edaa37c/Clustering-in-Vehicular-Ad-Hoc-Network-Using-Artificial-Neural-Network.pdf#page=75
https://www.researchgate.net/profile/Bouchra_Marzak/publication/306046482_Clustering_in_Vehicular_Ad-Hoc_Network_Using_Artificial_Neural_Network/links/5a0c19f0a6fdccc69edaa37c/Clustering-in-Vehicular-Ad-Ne-Hoc-Network-Network。
https://link.springer.com/chapter/10.1007/3-540-36182-0_37
https://link.springer.com/chapter/10.1007/3-540-36182-0_37
https://neuroneurotic.net/2015/11/30/does-correlation-imply-prediction/
https://neuroneurotic.net/2015/11/30/does-correlation-imply-prediction/
翻译自: https://medium.com/swlh/we-need-data-to-improve-healthcare-processes-at-scale-d3592a44c8d4
医疗大数据处理流程
本文来自互联网用户投稿,该文观点仅代表作者本人,不代表本站立场。本站仅提供信息存储空间服务,不拥有所有权,不承担相关法律责任。如若转载,请注明出处:http://www.mzph.cn/news/388020.shtml
如若内容造成侵权/违法违规/事实不符,请联系多彩编程网进行投诉反馈email:809451989@qq.com,一经查实,立即删除!