软件测试框架课程考试
By Levi Petty
李维·佩蒂(Levi Petty)
This project uses a public, synthesized exam scores dataset from Kaggle to analyze average scores in Math, Reading, and Writing subject areas, relative to the student’s parents’ level of education and whether the student took a preparation course before the exam. I used Deephaven’s R integration, which gave me access to cool plotting libraries. Additionally, users can see the code and plots step-by-step, yielding more detailed information.
Ť他的项目采用的是公开的,合成的考试成绩,从数据集Kaggle分析数学,阅读和写作学科领域的平均成绩,相对于学生的父母的受教育程度,以及是否该学生拿了准备课程在考试前。 我使用了Deephaven的R集成,这使我可以访问很酷的绘图库。 此外,用户可以查看代码并逐步进行绘制,从而获得更详细的信息。
我的过程 (My Process)
配置变量 (Configuration Variables)
We begin by defining some configuration variables:
我们首先定义一些配置变量:
home: Your home directory
home :您的主目录
system: Deephaven system to connect to (configured in the launcher)
系统 :要连接的Deephaven系统(在启动器中配置)
keyfile: Key file used to authenticate when connecting to the Deephaven system
keyfile :连接到Deephaven系统时用于认证的密钥文件
workerHeapGB: Gigabytes of heap for the Deephaven query worker
workerHeapGB :Deephaven查询工作者的千兆字节堆
jvmHeapGB: Gigabytes of heap for the local Java Virtual Machine (JVM)
jvmHeapGB :本地Java虚拟机(JVM)的千兆字节堆
workerHost: host to run the Deephaven query worker on
workerHost :在其上运行Deephaven查询worker的主机
连接到Deephaven查询工作者 (Connecting to the Deephaven Query Worker)
After setting up the configuration variables, the first step is to connect R to a Deephaven query worker. To determine the proper value for JAVA_HOME, run R CMD javareconf from the command line.
设置配置变量后,第一步是将R连接到Deephaven查询工作程序。 要确定JAVA_HOME的正确值,请从命令行运行R CMD javareconf 。
在表中加载 (Loading in the Table)
Prior to writing my code, I downloaded the StudentsPerformance.csv file from Kaggle and created a new user table so that I could access the table without needing to reload the file into a variable every time I executed my code.
在编写代码之前,我从Kaggle下载了StudentsPerformance.csv文件并创建了一个新的用户表,这样我就可以访问该表,而无需每次执行代码时都将文件重新加载到变量中。
创建初始表 (Creating The Initial Table)
After all this setup, my next step is to use Deephaven’s filtering tools to get the relevant data from this new user table and create an initial data table with each student’s parental level of education, whether they took a test prep course, and their scores in each of three subjects: Math, Reading, and Writing.
完成所有这些设置后,我的下一步就是使用Deephaven的过滤工具从此新用户表中获取相关数据,并使用每个学生的父母的受教育程度,他们是否参加了考试预备课程以及他们的分数来创建初始数据表。三个科目中的每一个:数学,阅读和写作。
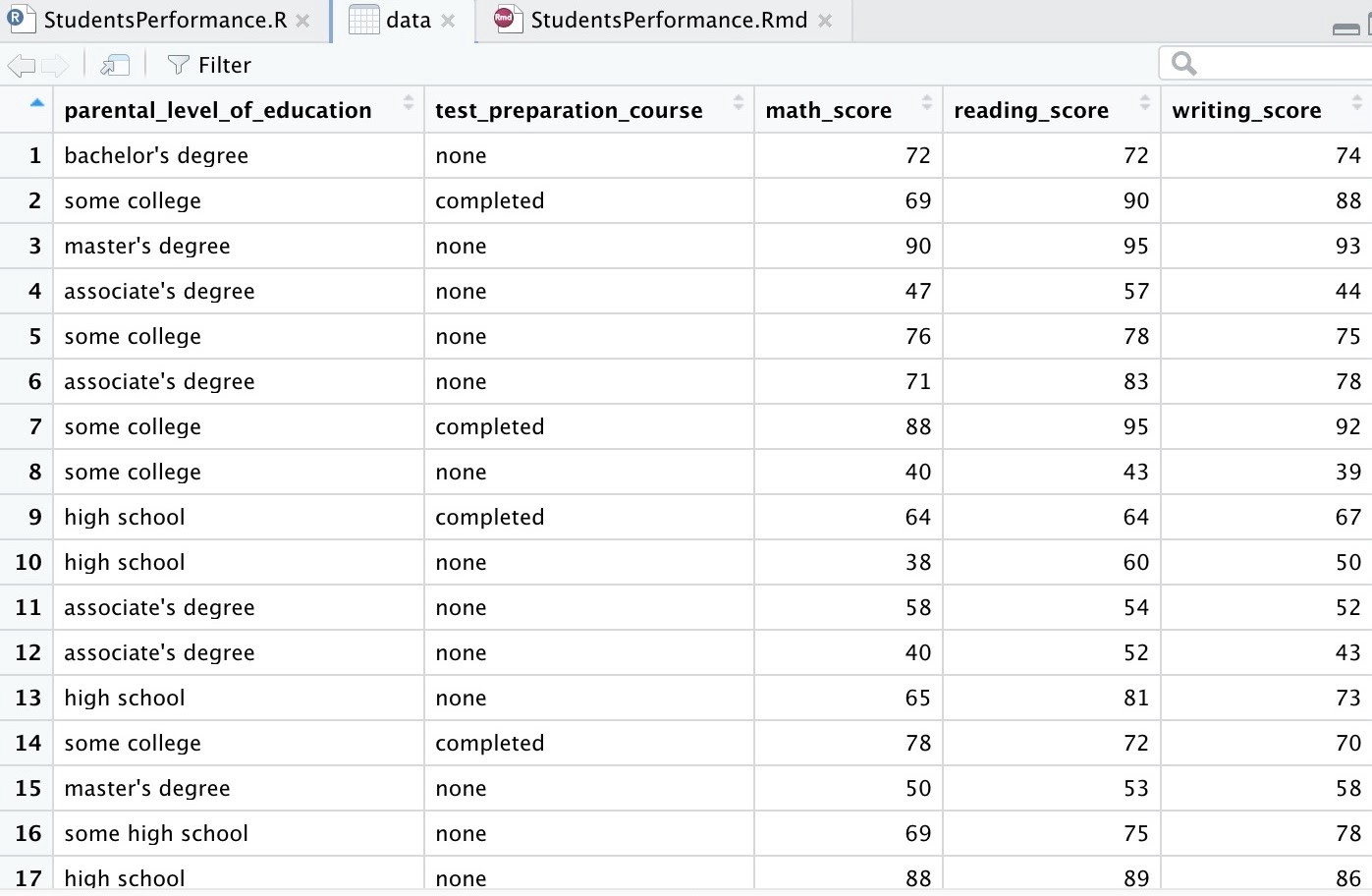
增加教育水平的排名 (Adding Rankings for Education Levels)
Next, I use a map to add rankings for each education level to the data table so that I can order the plots by education level instead of alphabetically. Each education level is given its own corresponding ranking from 0–5, with higher education levels having higher numbers for their ranking.
接下来,我使用地图将每个受教育程度的排名添加到数据表,以便我可以按受教育程度而不是按字母顺序对图进行排序。 每个教育级别都有自己从0-5的相应排名,而高等教育级别的排名则更高。
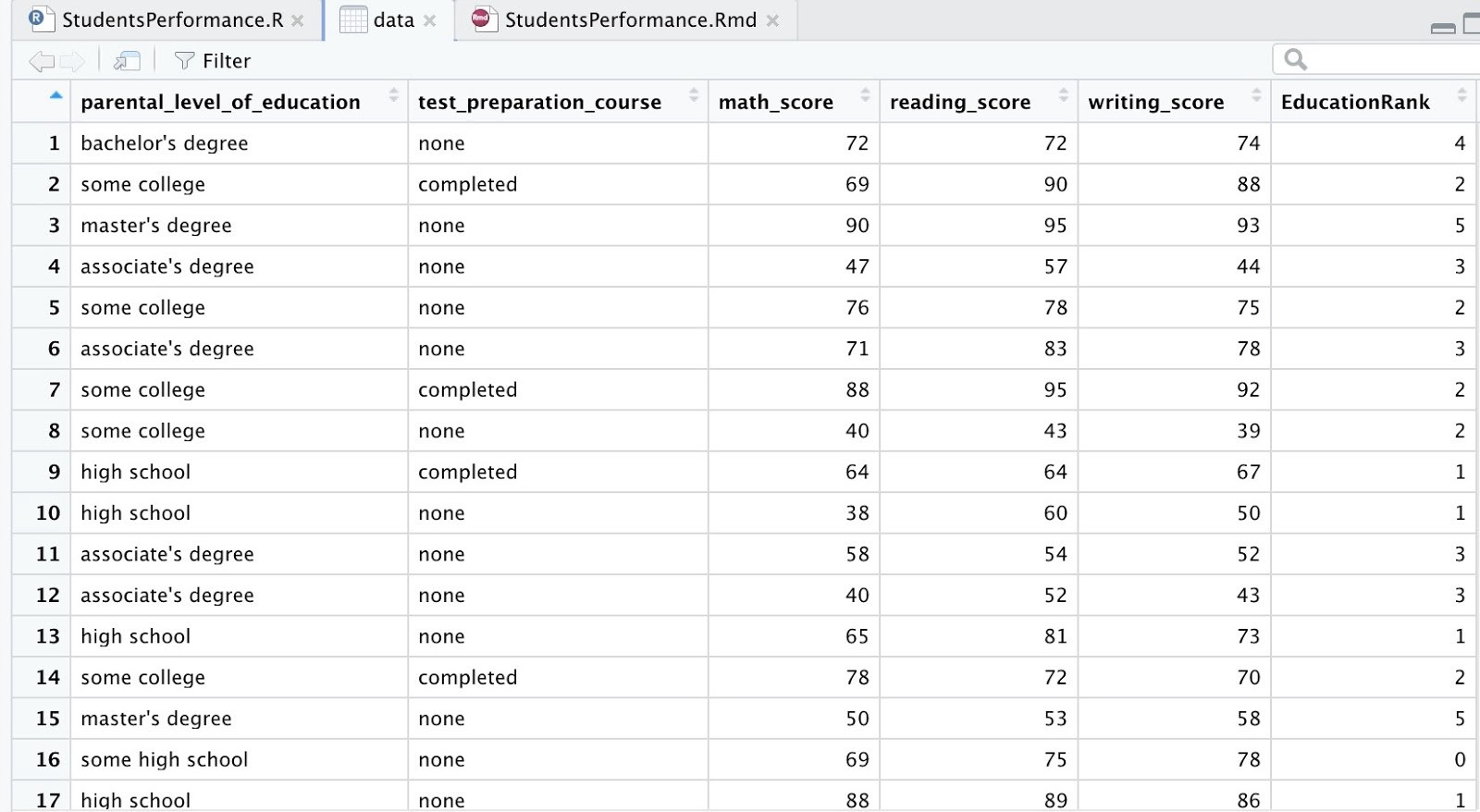
初始图 (Initial Plots)
Next, I use R’s ggplot2 and gridExtra libraries to plot the students’ scores with regard to their parents’ levels of education, and the students’ scores with regard to whether they took a test prep course. You’ll need to run install.packages(“ggplot2”, “gridExtra”) in RStudio to install these packages first.
接下来,我使用R的ggplot2和gridExtra库来绘制学生对他们父母的教育水平的评分,以及学生对他们是否参加考试预备课程的评分。 您需要在RStudio中运行install.packages(“ ggplot2”,“ gridExtra”)才能首先安装这些软件包。
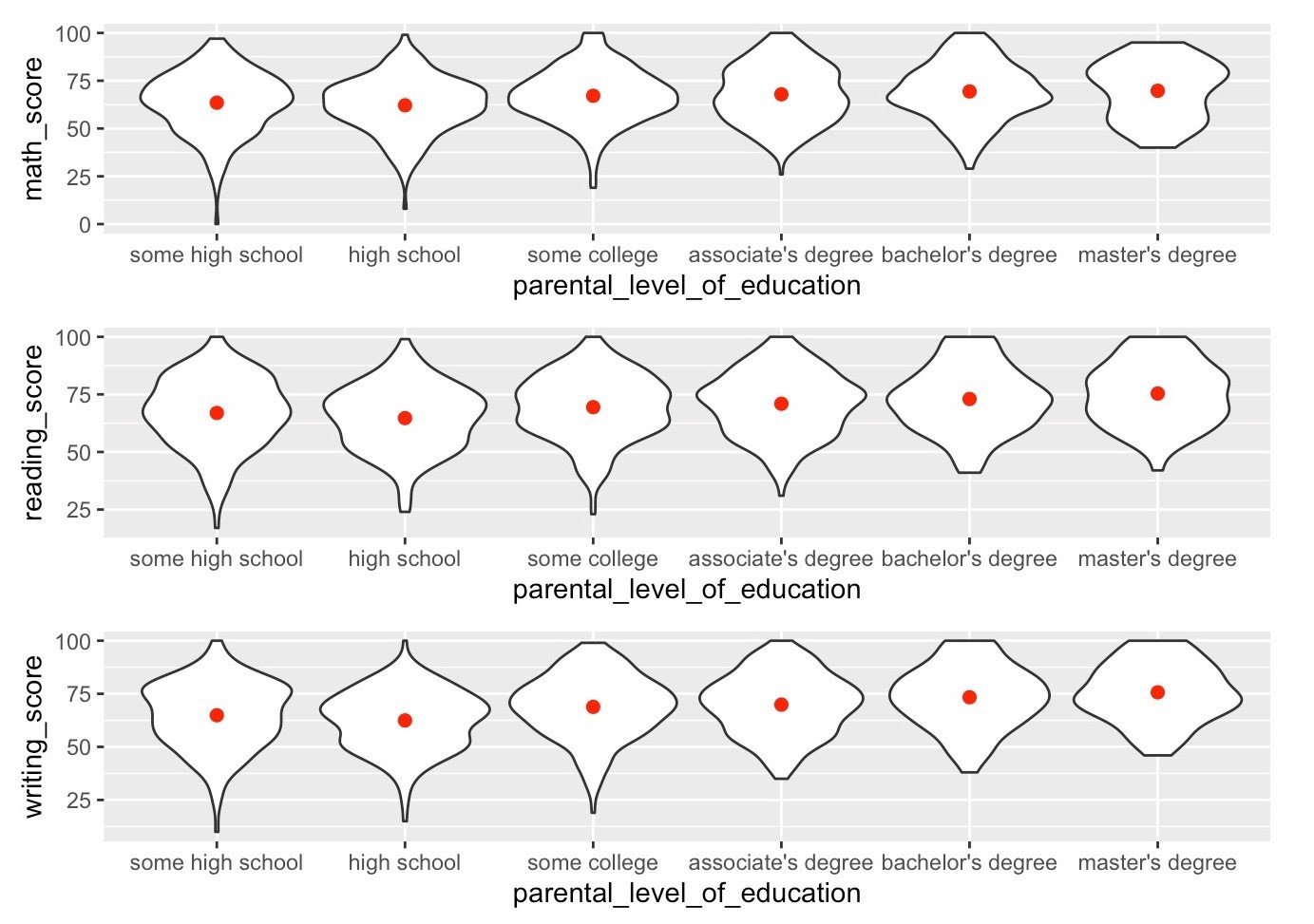
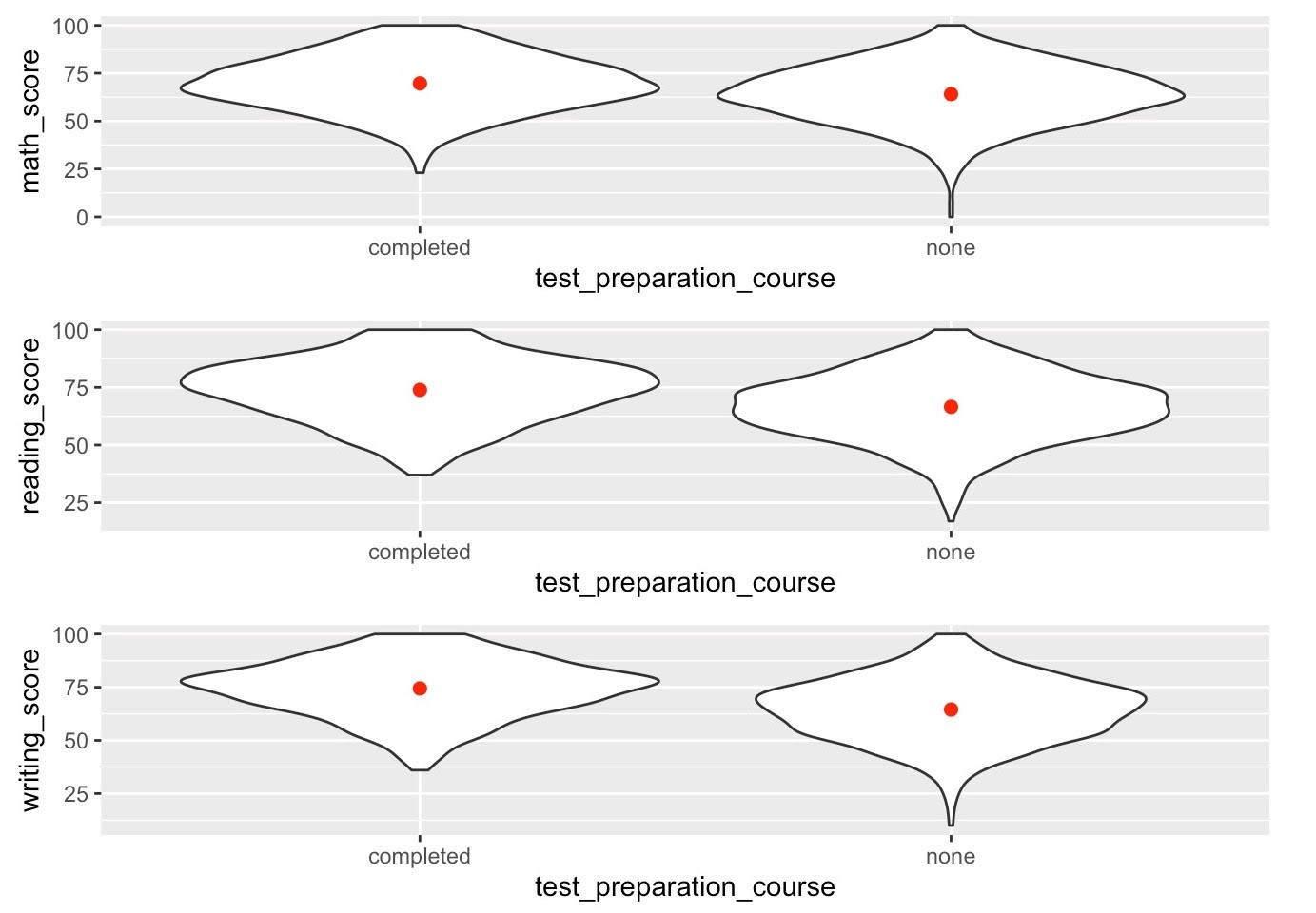
获取标准偏差和总体平均值 (Get Standard Deviations and Overall Averages)
Next, I use Deephaven’s filtering and aggregation tools to calculate the overall standard deviations and average scores for each subject, and store the results in constants. I do this because the standard deviations above average are actually more important information than just raw values, because knowing the standard deviation gives you a better idea of the magnitude of the impact of each variable.
接下来,我使用Deephaven的过滤和汇总工具来计算每个主题的总体标准偏差和平均分数,并将结果存储在常量中。 我这样做是因为高于平均值的标准偏差实际上比原始值更重要,因为知道标准偏差可以使您更好地了解每个变量的影响幅度。
将高于总体平均值的标准偏差添加到数据表中 (Add Standard Deviations Above Overall Average to the Data Table)
Lastly, I use Deephaven’s table updating functions to add the number of standard deviations each student’s score was above the overall average for each subject to the data table.
最后,我使用Deephaven的表格更新功能将每个学生的分数的标准差加到数据表中每个学科的总体平均水平之上。
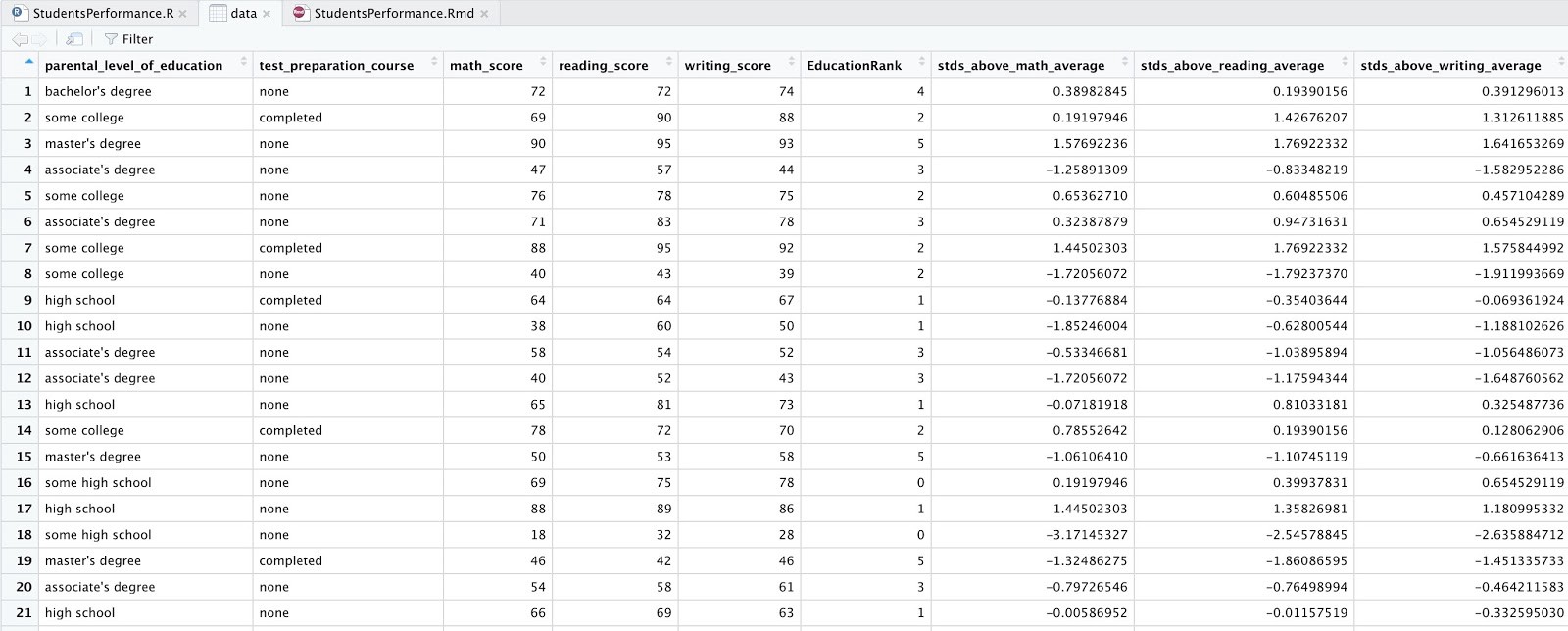
最终情节 (Final Plots)
Finally, I add the standard deviations above average to both plots.
最后,我将高于平均值的标准偏差添加到两个图中。
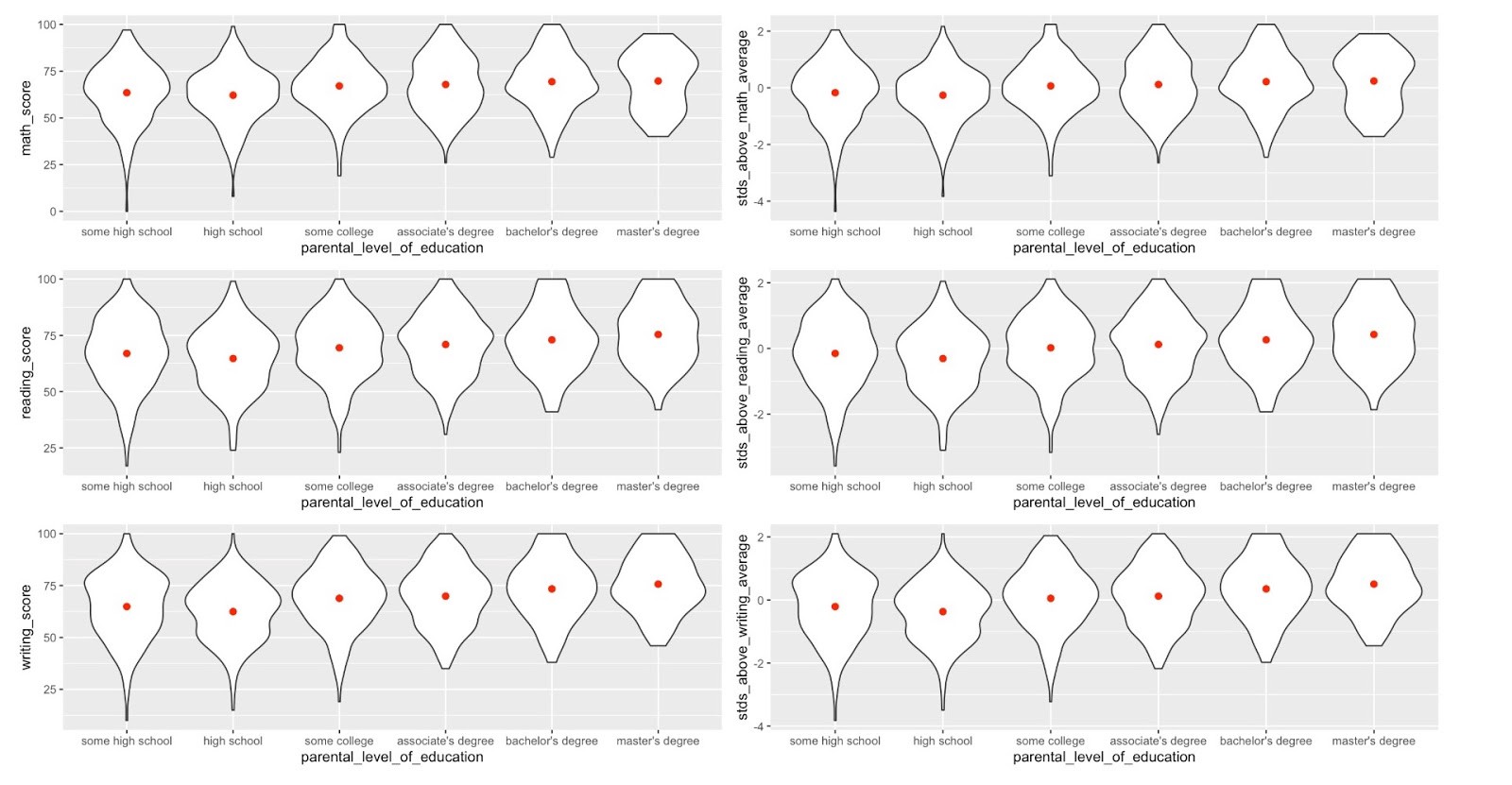
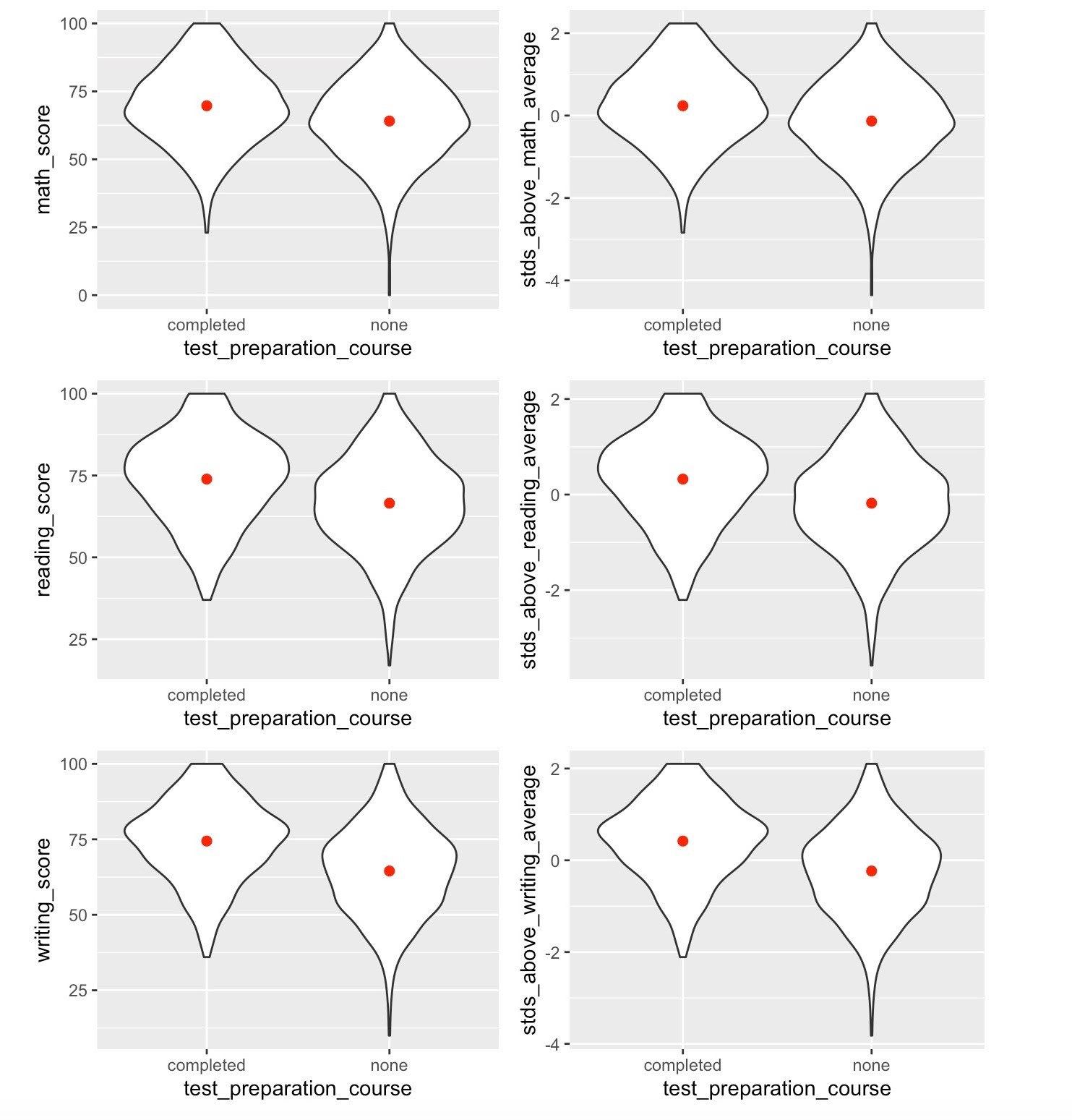
结论 (Conclusion)
As expected, a higher parental level of education correlated with higher scores in every subject, and generally resulted in less varying distributions. Students with a master’s degree parental education level in particular had top-heavy score distributions in math and writing.
不出所料,父母的受教育程度越高,每门科目的得分越高,通常分配的差异就越小。 尤其是具有父母教育程度的硕士学位的学生在数学和写作方面的成绩分配最高。
Also unsurprisingly, whether the student took a test prep course had a larger impact on the scores in each subject. The students who took a test prep course had more top-heavy scores in every subject than those who didn’t, especially writing. Surprisingly, the math scores were the least impacted by test prep courses. Neither variable had a large influence on the scores, though, as the scores were only impacted by <1 standard deviation. However, because this data is simulated, we must take the results with a grain of salt.
同样也就不足为奇的是,学生是否参加了考试预备课程对每个科目的成绩都有较大的影响。 参加考试预备课程的学生与没有考试的学生相比,在各个学科上的成绩最高,尤其是写作。 令人惊讶的是,数学分数受考试准备课程的影响最小。 但是,这两个变量都不会对分数产生很大影响,因为分数仅受<1个标准偏差的影响。 但是,由于此数据是模拟的,因此必须对结果进行细微的估计。
If you’re interested, you can read my full code below. If you want to see the html file, you can run the code in RStudio.
如果您有兴趣,可以在下面阅读我的完整代码。 如果要查看html文件,可以在RStudio中运行代码。
翻译自: https://medium.com/swlh/is-that-test-prep-course-worth-it-a18011524394
软件测试框架课程考试
本文来自互联网用户投稿,该文观点仅代表作者本人,不代表本站立场。本站仅提供信息存储空间服务,不拥有所有权,不承担相关法律责任。如若转载,请注明出处:http://www.mzph.cn/news/388002.shtml
如若内容造成侵权/违法违规/事实不符,请联系多彩编程网进行投诉反馈email:809451989@qq.com,一经查实,立即删除!