jvm gc策略
IBM JVM概述
正如您可能从其他文章中看到的那样,IBM JVM在某些方面与HotSpot JVM不同,因为它没有PermGen内存空间。 从垃圾回收的角度来看,它确实为您提供了可以利用多物理核心计算机的高级算法; 与HotSpot JVM类似。
从故障诊断的角度来看,IBM为您提供了许多工具。 包括从其JVM实现中获得的开箱即用的Thread Dump和Heap Dump生成功能。
例如,IBM JVM线程转储特别强大,因为它为您提供了有关JVM的额外数据,例如活动的JVM环境变量,GC策略,每个活动的类加载器中的已加载类等。我们将在本部分中对此进行更详细的探讨。我们的线程转储培训计划的4 。
IBM VM –默认GC行为
现在回到我们的主要主题,了解IBM JVM垃圾收集器(版本1.5和1.6)的默认行为非常重要。 默认情况下,使用终身记忆只例如,它不会创建一个单独的YoungGen(幼儿园)空间中创建了Java堆空间。 这意味着任何内存分配都将进入保管空间(短期和长期对象),该空间随后由默认收集器(通过Full GC)收集。
在下面找到详细的GC快照,其中显示了默认的GC内存故障,并带有解释:
<af type="tenured" id="5" timestamp="Mar 01 13:40:30 2012" intervalms="0.000"><minimum requested_bytes="48" /><time exclusiveaccessms="0.106" meanexclusiveaccessms="0.106" threads="0" lastthreadtid="0x000000011A846B00" /><tenured freebytes="20131840" totalbytes="2013265920" percent="0" ><soa freebytes="0" totalbytes="1993134080" percent="0" /><loa freebytes="20131840" totalbytes="20131840" percent="100" /></tenured><gc type="global" id="8" totalid="2492" intervalms="2017588.587"><finalization objectsqueued="199" /><timesms mark="808.286" sweep="9.341" compact="0.000" total="818.292" /><tenured freebytes="1362331024" totalbytes="2013265920" percent="67" ><soa freebytes="1344212368" totalbytes="1995147264" percent="67" /><loa freebytes="18118656" totalbytes="18118656" percent="100" /></tenured></gc><tenured freebytes="1362330976" totalbytes="2013265920" percent="67" ><soa freebytes="1344212320" totalbytes="1995147264" percent="67" /><loa freebytes="18118656" totalbytes="18118656" percent="100" /></tenured><time totalms="818.750" /></af>
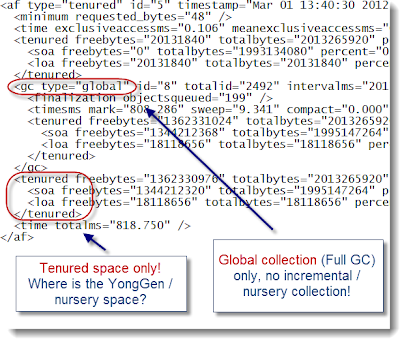
好的,默认的IBM JVM GC策略是不同的……问题是什么?
此默认JVM策略的问题在于,所有Java对象都将复制到使用权空间并通过全局集合(完整GC)进行收集。 对于许多Java EE应用程序,短期对象与长期对象的比率要高得多。 这意味着您的JVM将需要执行很多主要的收集工作,以清理短期对象。 结果:Full GC的频率增加,JVM暂停时间增加,CPU利用率增加和性能下降!
这正是我们在使用默认GC策略迁移到JVM HotSpot 1.5(使用增量和并行GC)到IBM JVM 1.6进行负载测试之后观察到的结果。 根据上述说明,重度GC过程被确定为根本原因。
请解决!
好消息是,IBM JVM 从版本1.5开始引入了世代和并发GC收集器 。 该GC政策正提供我们想要的:
- 它确实将Java Heap空间在苗圃空间和保育空间之间进行了划分
- 托儿所(YoungGen)空间对象通过清除剂GC收集器分别收集
- 通过全局GC收集器收集长期空间对象
- GC收集器是并发的,并且可以利用任何多物理核计算机
结果:
- 降低主要采集频率(全GC)
- 减少了全GC消耗的时间和暂停时间
- 增加JVM吞吐量
- 提高应用程序的性能和容量
您可以通过在下面添加此JVM参数来启用它:
-Xgcpolicy:gencon
在启用此GC策略后,在下面的详细GC日志中可以找到什么:
<af type="nursery" id="15" timestamp="Mar 08 05:34:06 2012" intervalms="1289096.227"><minimum requested_bytes="40" /><time exclusiveaccessms="0.085" meanexclusiveaccessms="0.085" threads="0" lastthreadtid="0x0000000118113900" /><refs soft="18043" weak="204057" phantom="27" dynamicSoftReferenceThreshold="32" maxSoftReferenceThreshold="32" /><nursery freebytes="0" totalbytes="530716672" percent="0" /><tenured freebytes="1887422016" totalbytes="2013265920" percent="93" ><soa freebytes="1786758720" totalbytes="1912602624" percent="93" /><loa freebytes="100663296" totalbytes="100663296" percent="100" /></tenured><gc type="scavenger" id="15" totalid="15" intervalms="1289097.271"><flipped objectcount="1486449" bytes="129908000" /><tenured objectcount="1176" bytes="184144" /><finalization objectsqueued="3082" /><scavenger tiltratio="73" /><nursery freebytes="364304408" totalbytes="495208448" percent="73" tenureage="10" /><tenured freebytes="1886766656" totalbytes="2013265920" percent="93" ><soa freebytes="1786103360" totalbytes="1912602624" percent="93" /><loa freebytes="100663296" totalbytes="100663296" percent="100" /></tenured><time totalms="233.886" /></gc><nursery freebytes="364238872" totalbytes="495208448" percent="73" /><tenured freebytes="1886766656" totalbytes="2013265920" percent="93" ><soa freebytes="1786103360" totalbytes="1912602624" percent="93" /><loa freebytes="100663296" totalbytes="100663296" percent="100" /></tenured><refs soft="17992" weak="5344" phantom="27" dynamicSoftReferenceThreshold="32" maxSoftReferenceThreshold="32" /><time totalms="236.858" /></af>
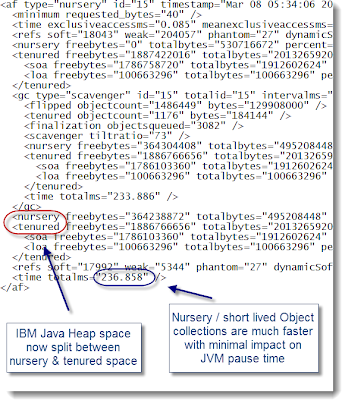
请记住,您的应用程序仍可能无法从该GC策略中受益(长寿命对象的占用空间更大等),因此,我对您的建议是始终进行尽职调查并执行适当的容量规划和负载测试您的应用程序,然后再执行任何主要的调整建议。
结论
我希望本文能帮助您了解默认的IBM JVM 1.5 / 1.6 GC策略以及Java EE应用程序如何从该GC策略gencon调整建议中受益。
参考: IBM JVM调优–来自我们JCG合作伙伴 Pierre-Hugues Charbonneau的gencon GC策略 ,位于Java EE支持模式和Java教程博客。
翻译自: https://www.javacodegeeks.com/2012/04/ibm-jvm-tuning-gencon-gc-policy.html
jvm gc策略