本笔记来源自Youtube李宏毅老师的RNN视频归纳,主要分为以下几个知识点:
- RNN 的特点
- RNN 的几种实现方法 (Simple RNN, LSTM)
- RNN 的训练不稳定性
- RNN 的keras实现 (定长和变长输入案例)
Recurrent Neural Network
Feature of RNN
Differ from normal Neural Network, Recurrent Neural Network has memory. RNN can remember the history, named hidden state, and combine it with current state to choose the action aaa.
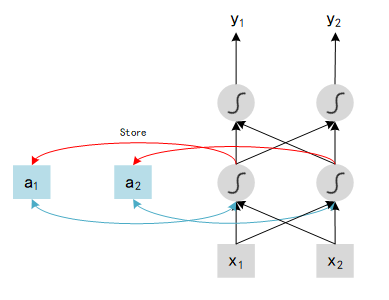
Show as above, x1x_1x1 and x2x_2x2 are tow inputs, outputs of each hidden layer will be stored in the Network after be calculated. In the next iteration, with another two inputs x1′x_1'x1′ and x2′x_2'x2′ , network will combine this new inputs {$x_1’ $, x2′x_2'x2′ } with last outputs history {a1a_1a1 , a2a_2a2 }, and compute the final value yyy. In particular, a1a_1a1 and a2a_2a2 are history data, could be explained as memory, so we can say that RNN has ability to remember history states.
Here is an example to understand the RNN: assume all weights in network is 1, no bias and all activation functions are linear. We input a sequence : [1, 1], [1, 1], [2, 2], … and see the result of output.
- At first we need to initialize the value of memory block, here we set 0 for all block. With [1, 1] input, hidden layer 1 will compute : x1x_1x1 (1) + x2x_2x2 (1) + a1a_1a1 (0) = 2. Same as other neural:
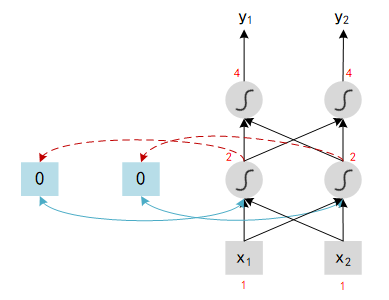
- When the first iteration finished, memory block will update its value to the output of hidden neural. Now we input the second tuple in the sequence : [1, 1], the output should be : x1x_1x1 (1) + x2x_2x2 (1) + a1a_1a1 (2) = 4.
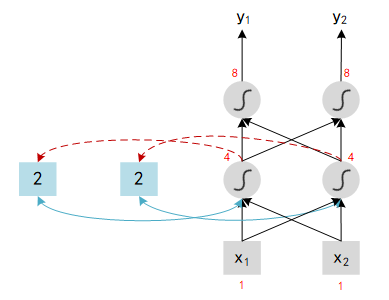
Note
: Change the sequence order will change the output.
So the total work flow of RNN looks like:
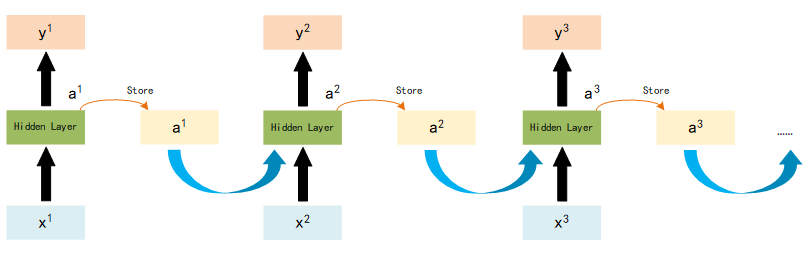
Different forms of RNN
RNN has a lot of different types, here introduce three of most common methods: Elman Network, Jordan Network and Bidirectional RNN.
Elman Network and Jordan Network
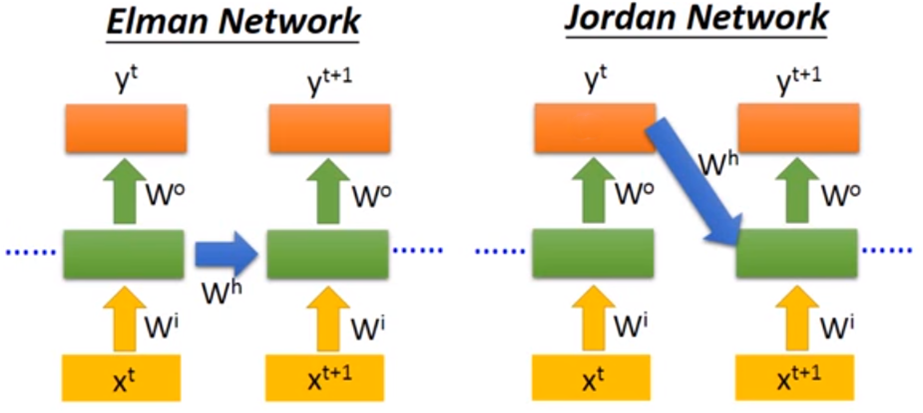
The main difference of these two method is : Elman Network stores all parameters of hidden layers, use last parameters to compute the value; Jordan Network stores the target output value, and combined this output with next input to compute the value.
Bidirectional RNN
Bidirectional RNN is train both Forward and Backward model, use the combination of both model to decide the result of xtx^txt, shown as below :
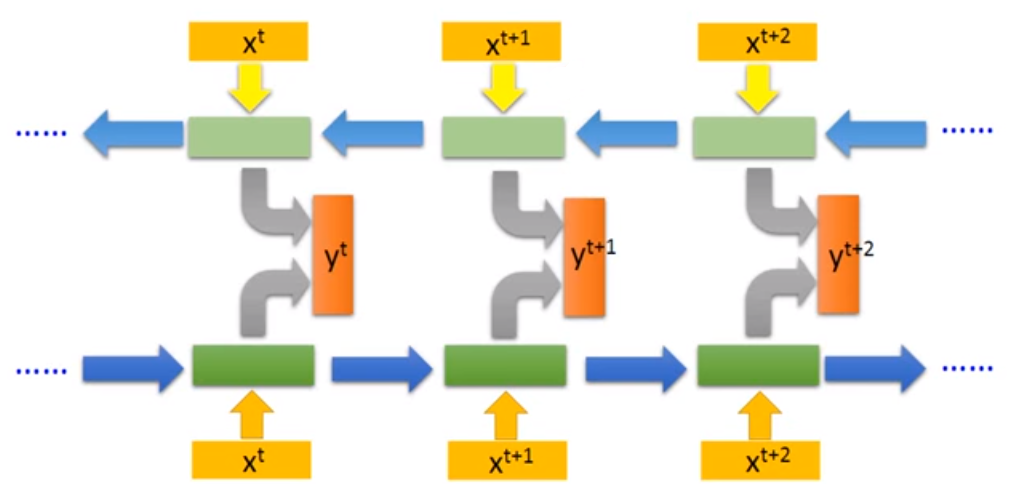
The advantage of this model is that it will decide the value of xtx^txt according to the entire state not only the history state (in forward rnn, value of xtx^txt is only decided by the state history before ttt while there exists backward model in bidirectional rnn which can consider the history between ttt to NNN, NNN is the final state of this sequence).
★ Long Short-term Memory (LSTM)
LSTM focus on the structure of Memory cell, which contains three parts in the controller of memory cell: Input Gate, Output Gate and Forget Gate.
-
Input Gate: memory cell stores the parameters to remember the history, Input Gate decides if these parameters update or not in each step. New parameters of current step can pass into memory cell only if Input Gate is open while the state of Input Gate is controlled by Control Signal.
-
Output Gate: output of this model is the parameters in the memory cell, Output Gate which controlled by Output Gate Signal decides these parameters can output or not.
-
Forget Gate: memory cell stores a lot of history states, which means there may exist some old and outdated data. Forget Gate controlled by Forget Gate Signal decides which data need to be forgot.
There are 4 inputs (Input Gate Signal, Output Gate Signal, Forget Gate Signal and Input Data) and 1 output in this model, structure shown as below:
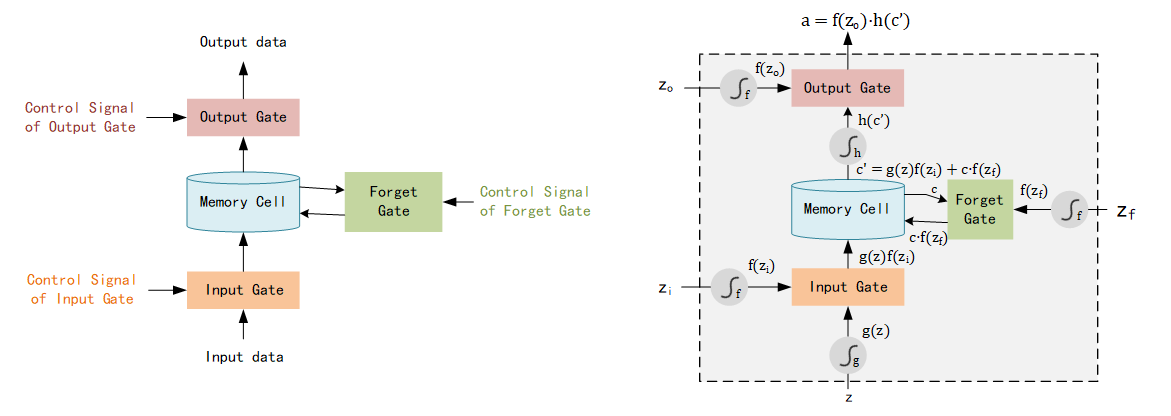
Here we input data as zzz, pass the activate function ggg and become g(z)g(z)g(z). Input Gate Signal ziz_izi pass the activate function fff and become f(zi)f(z_i)f(zi), output of Input Gate is f(zi)⋅g(z)f(z_i)·g(z)f(zi)⋅g(z). ccc is the origin parameters stored in Memory Cell, zfz_fzf is the Forget Gate Signal and the output of Forget Gate is f(zf)⋅cf(z_f)·cf(zf)⋅c, which named c′c'c′. The final output aaa equals f(z0)⋅h(c′)f(z_0)·h(c')f(z0)⋅h(c′).
Note: Activation Function fff often be sigmoid function, output is 0 or 1, which expresses the Gate is opened or not. For Input Gate, If value equals 1, the input zzz is fully passed through this gate while if value equals 0, none of input zzz could be passed.
A clear example could be found here (29:50) :
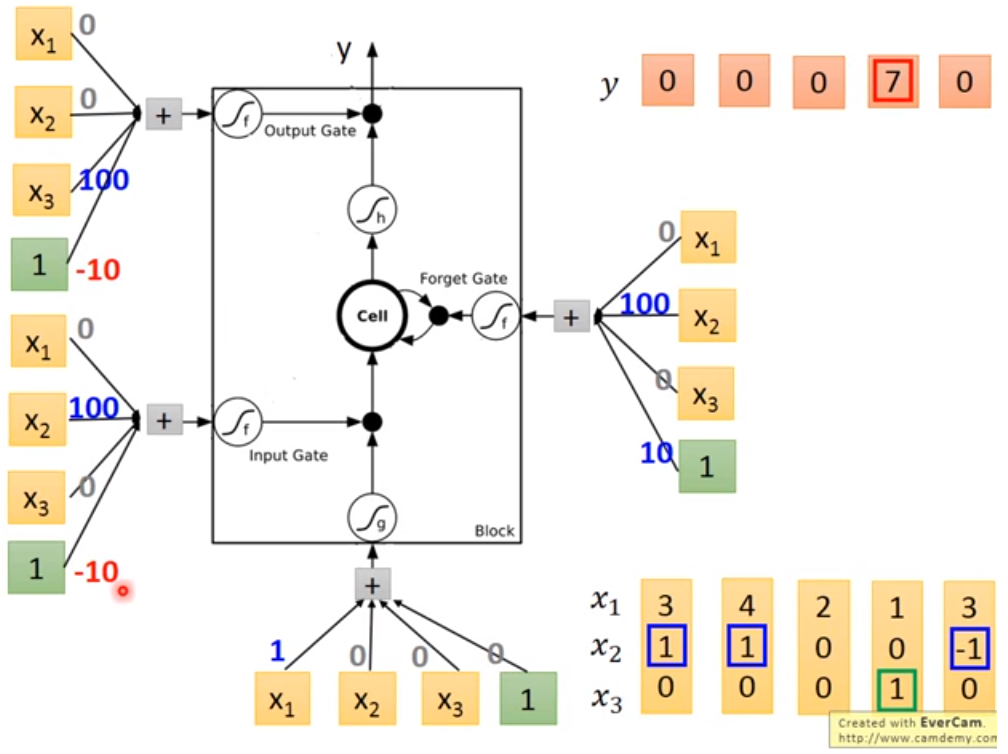
Now we know the structure of Memory Cell. In LSTM, each Memory Cell expresses a neural which means each neural needs 4 different inputs. Typically we have only 1 input, but these 4 inputs are all computed from that 1 input ( by multiple different vector ).
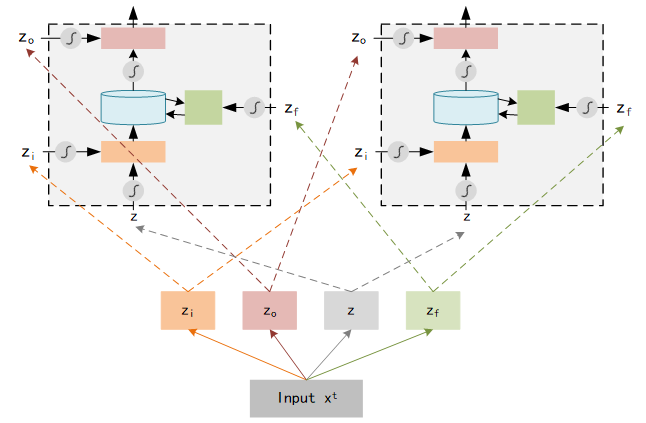
Furthermore, we compute the output using 4 inputs in each neural :
yt=h[f(zf)⋅ct−1+f(zi)⋅z]⋅f(zo)y^t = h[f(z_f)·c_{t-1} + f(z_i)·z]·f(z_o) yt=h[f(zf)⋅ct−1+f(zi)⋅z]⋅f(zo)
Picture below shows the computation logic :
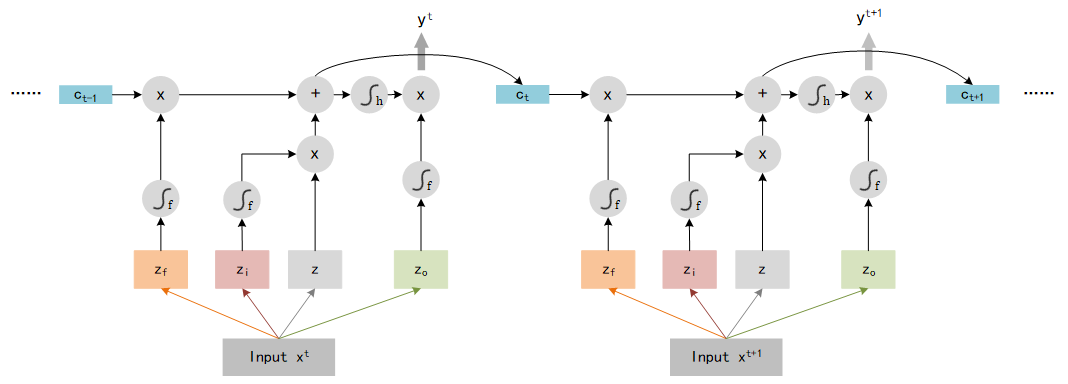
That is not the end of LSTM, we need to add some extra data as input. LSTM use the output yty^tyt and the memory ctc_tct as the input of next step t+1t+1t+1, so the entire structure of LSTM should look like:
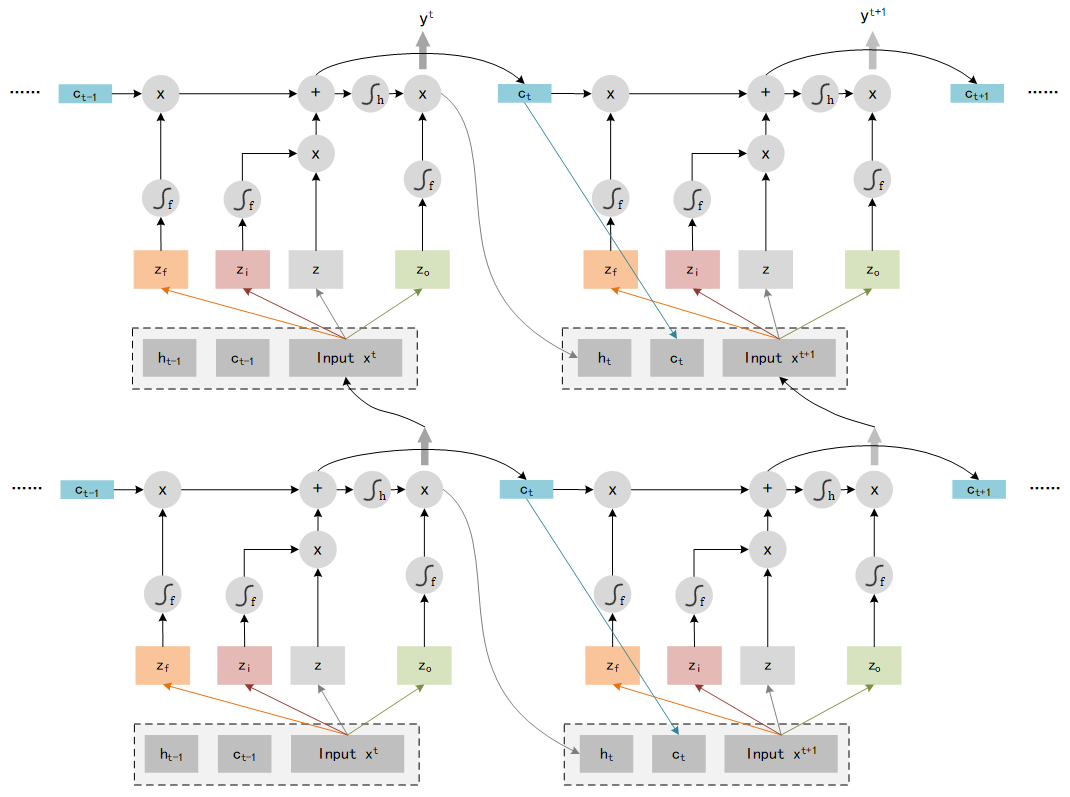
GRU (Gated recurrent unit)
A method based on LSTM, which delete 1 gate (only 2 gates used) but has the same performance as LSTM.
How to train RNN?
The method of RNN training is also Backpropagation, but exists slight difference: we need to sum the loss of all steps.

In RNN training, the loss values are often jump sharply, show as below picture:
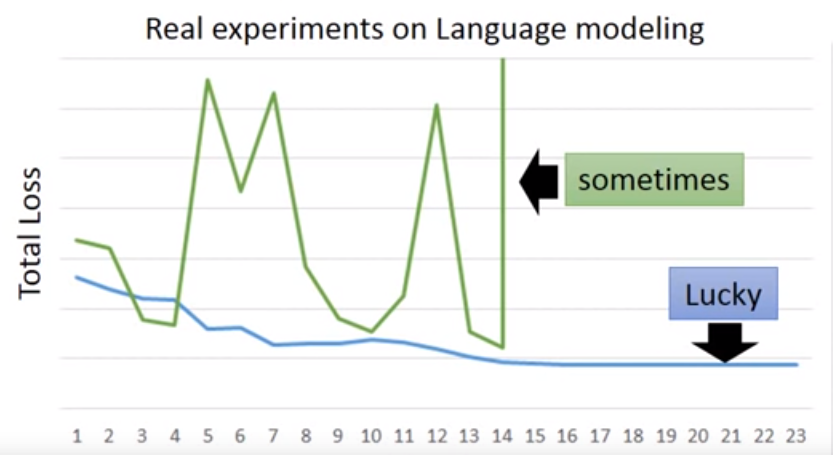
What is the reason of this strange problem? Visualize the loss value of w1w_1w1 and w2w_2w2, found there exists a sharply changing. When orange point jump from 2 to 3, it will get a big loss.
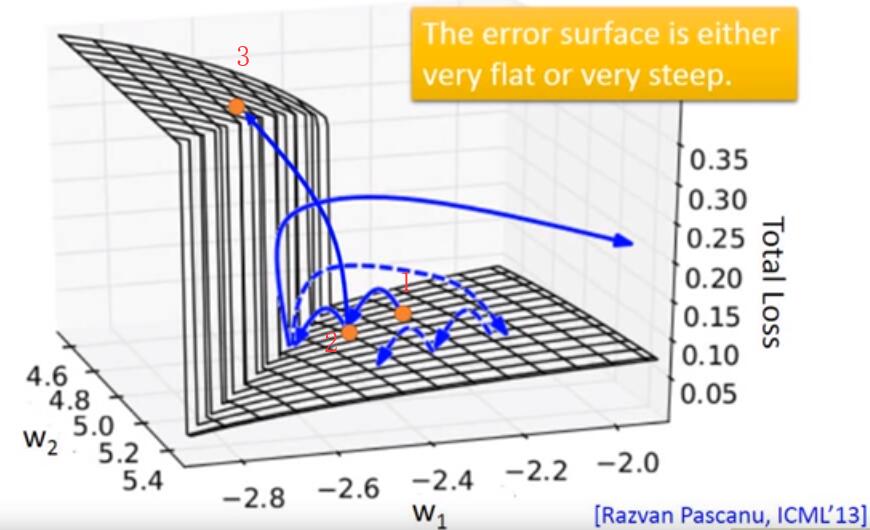
The resolution of this problem is Clipping: if the loss value is bigger than a threshold, then let the loss value equal to this threshold.
This unstable loss is the feature of RNN, but why? What on earth is the reason which causes this problem? Here is an easy example to show the effect of result yty_tyt by changing the www.
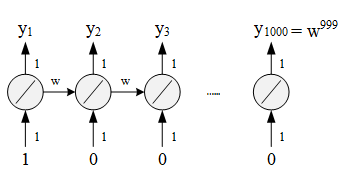
Assume only one neural in each network, weight is 1, no bias, www is transform weight (parameters in last layer needs multiply www then input into next layer).
w=1→y1000=1w=1 \quad \rightarrow \quad y_{1000}=1w=1→y1000=1
w=1.01→y1000≈20000w=1.01 \quad \rightarrow \quad y_{1000}\approx20000w=1.01→y1000≈20000
Even if www changes slightly, the output of last layer y1000y_{1000}y1000 changes a lot. This because we multiply www many times which means we magnify the effect of transform weight.
w=0.99→y1000≈0w = 0.99 \quad \rightarrow \quad y_{1000} \approx0w=0.99→y1000≈0
w=0.01→y1000≈0w = 0.01 \quad \rightarrow \quad y_{1000} \approx 0w=0.01→y1000≈0
Even if two www are total different, the output of last layer is same.
Use LSTM to solve problem —— handle gradient vanishing
The difficulty of RNN is that we don’t know how to choose a suitable learning rate: big η\etaη need for plane surface but small η\etaη for sharp changing area. LSTM could solve this problem by making those plane area more uneven so that you can safely assign a small η\etaη.
In traditional RNN, parameters in memory cell will be covered after each step which means it forget the history before. But in LSTM, parameters in memory cell not be covered, data in this step are added with history before, so the history could be remembered. Further more, gradient won’t be vanished if forget gate is not opened, so there is no gradient vanishing in LSTM.
Implementation LSTM with keras
To use keras, we need to understand following terms:
- input_dim: dimension of input vector.
- time_steps: length of Sequence.
- output_dim = dimension of output. Notice this output is not the final output of entire Neural Network, it’s just the output of LSTM Layers ( LSTM might be a part of one Neural Network, see as code below ).
Say if we want to predict the “happy feeling” or “sad feeling” of two sentence, “I am lucky!” and “I feel bad!”. Then the time_steps should be 3 ( the length of sentence is 3 ), and the output_dim should be 1 ( 0 expresses unhappy while 1 expresses happy ). Because of the different length of words ( ‘I’ has 1 character while ‘lucky’ has 5 characters ), we can’t choose a fixed input dimension. The resolution of this problem is using Embedding Layer to map the different length words to a specific dimension.
LSTM for Constant Length Input problem
Here is a simple example which use LSTM to predict the MNIST problem, key code block shown as below:
input_dim = 28 # MNIST image's width
time_steps = 28 # MNIST image's height
output_dim = 28 # output dimension for LSTM
model = Sequential()
model.add(LSTM(units=ouput_dim, input_shape=(time_steps, input_dim))) # add LSTM Layer
model.add(Dense(10, activation='softmax')) # since there are 10 classes in MNIST
LSTM for Different Length Input problem
Because of the different length between different sentences. We need to add a Masking Layer to solve the Length Problem.
First, we add 0 to make all samples have the same length, for example, here is a sample set:
X = np.array([[1, 3, 2], # sentence 1 - 3 words[2], # sentence 2 - 1 words[3, 4] # sentence 3 - 2 words
])
we use inner function of keras to change the sample length:
X = keras.preprocessing.sequence.pad_sequences(X, maxlen=3, padding='post')
Now the matrix X changes to:
array([[1, 3, 2], # sentence 1 - 3 words[2, 0, 0], # sentence 2 - 3 words[3, 4, 0] # sentence 3 - 3 words
])
Then, we add a Mask Layer to filter the 0 value which means these neural with 0 value won’t be calculated in the feed-forward process.
model = Sequential()
model.add(Masking(mask_value=0, input_shape=(3, 3))) # add Masking Layer
model.add(LSTM(units=ouput_dim, input_shape=(3, 3))) # add LSTM Layer
model.add(Dense(10, activation='softmax'))