用户细分
介绍 (Introduction)
The goal of this analysis was to identify different user groups based on the deals they have availed, using a discount app, in order to re-target them with offers similar to ones they have availed in the past.
该分析的目的是使用折扣应用程序基于他们所获得的交易来识别不同的用户组,以便以与他们过去所获得的类似的报价来重新定位他们。
Machine learning algorithm K-means was used to identify user segments based on their purchase behavior. Here is a 3-D illustration of what algorithm extracted.
机器学习算法K-means用于根据用户细分的购买行为识别用户细分。 这是所提取算法的3D图。
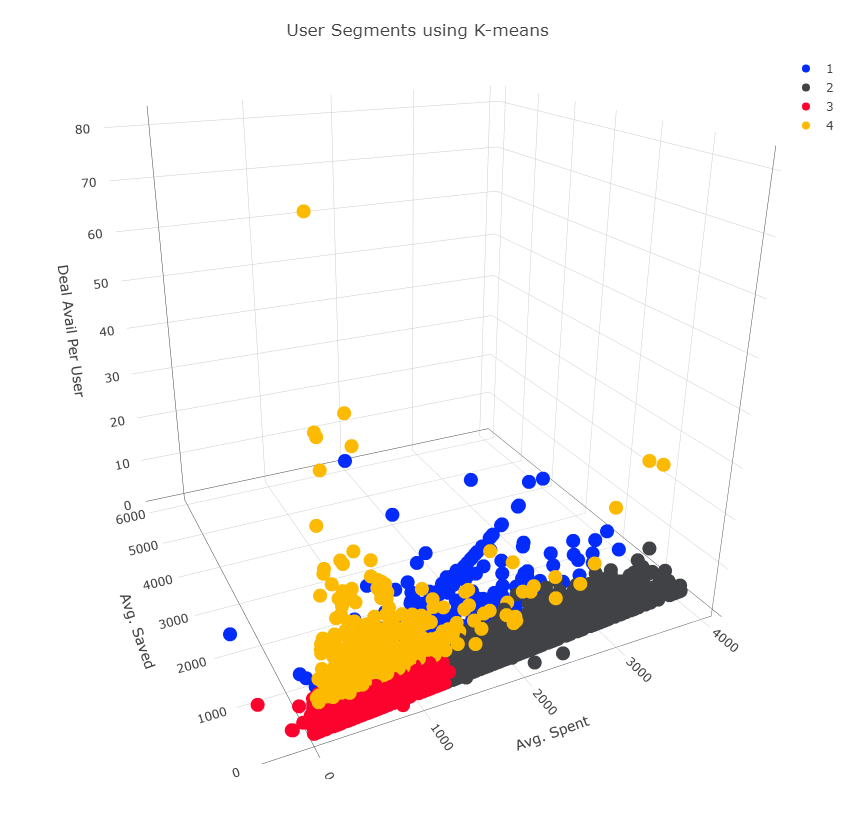
术语: (Terminologies:)
Before going deeper into the analysis, let’s define some keywords being used.
在深入分析之前,让我们定义一些正在使用的关键字。
Deal Avail: When user avails discount using app.Spent: Discounted price user pays while buying an item.Saved: Amount user saved through the app.Brands: Vendors for which discounts are being offered such as Pizza Hut, GreenODeals: Discounts offered to users on different outlets and brands.
交易无效:当用户使用应用程序享受折扣时。 已用:用户在购买商品时支付的折扣价。 已保存:通过应用保存的用户数量。 品牌:为其提供折扣的供应商,例如必胜客,GreenO 交易:为不同商店和品牌的用户提供折扣。
分析 (Analysis)
资料集 (Data sets)
The behavior data set was extracted from Mixpanel using JQL. Following was used for this analysis
使用JQL从Mixpanel提取行为数据集。 以下用于此分析
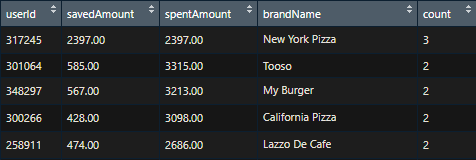
userId: unique id of usersaveAmount: amount saved by user on deal availspentAmount: amount spent by user on deal availbrandName: brand for which deal was availedcount: number of deals availed by user
userId:用户的唯一ID saveAmount :用户在交易有效时所节省的金额costAmount :用户在交易有效时所消耗的金额brandName :已进行交易的品牌数 :用户所进行的交易数量
Using the above data set averageSpentAmount, averageSavedAmount and dealAvailCount was calculated for each user as seen below
使用上面的数据集,为每个用户计算了averageSpentAmount , averageSavedAmount和dealAvailCount ,如下所示
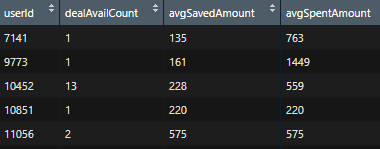
Machine Learning — K-means ClusteringThe first step of the k-mean algorithm was to find an optimal number of clusters for segmentation. There are a number of methods out there for this purpose, one of which is the elbow method using within-cluster sum square (wcss).
机器学习-K均值聚类 k均值算法的第一步是找到用于分割的最佳聚类数。 为此,存在许多方法,其中一种是使用簇内和平方(wcss)的肘方法。
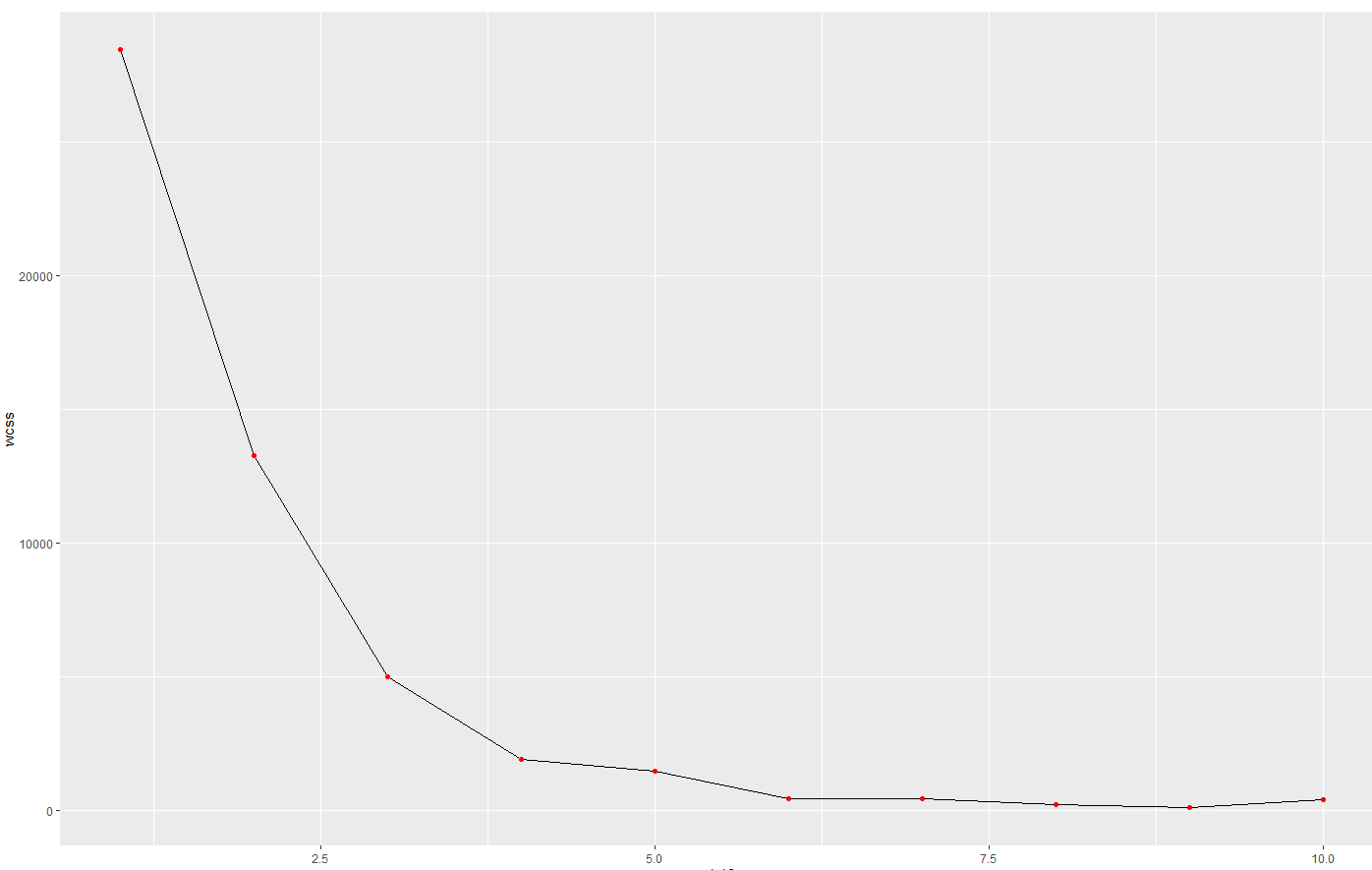
Based on the elbow method, 4, 5, and 6 clusters were used to explore the segments and 4 clusters were picked as best for the given data set.
基于弯头方法,使用4、5和6个聚类来探索这些段,并且对于给定的数据集,最好选择4个聚类。
I would recommend these courses on Data camp and Coursera if you want to learn more about user clustering and user segmentation.
如果您想了解有关用户集群和用户细分的更多信息, 我将在 数据营 和 Coursera 上推荐这些课程 。
K均值提取了哪些细分? (What Segments K-means extracted?)
Following were average stats of four identified segments:
以下是四个确定的细分市场的平均统计信息:
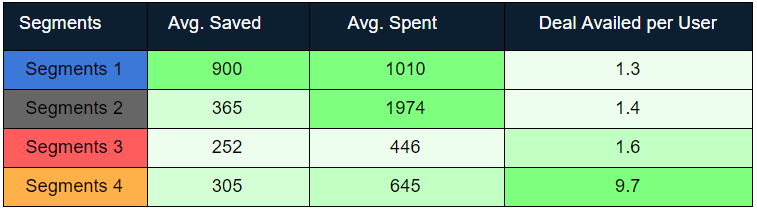
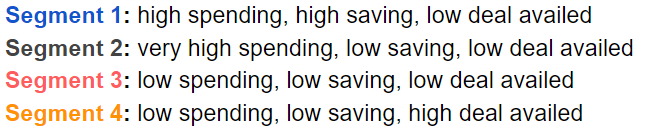
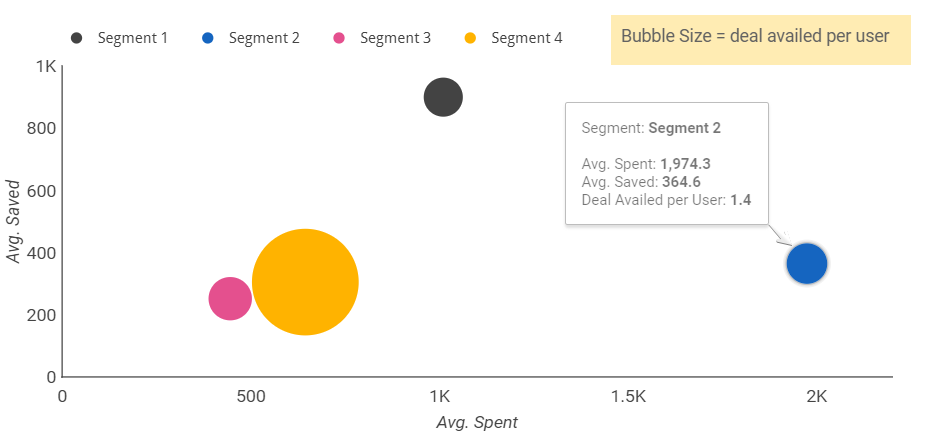
Users in segment 1 and 2 were high paying users with segment 1 users also had saved equally high per deal(probably availed buy 1 get 1 offers). However, the number of deals availed by these users were less than 2 (i.e. 1.3 and 1.4 respectively).
第1段和第2段的用户是高薪用户,第1段的用户每笔交易也节省了同样高的费用(可能使用“买一送一”的优惠)。 但是,这些用户获得的交易数量少于2(即分别为1.3和1.4)。
On the other hand, segment 3 and segment 4 users spent less and hence, saved less as well. However, segment 4 users had the greatest deal availed per user ratio (on average more than 9 deals availed by each user) in all 4 segments. It was the most converted cohort of users.
另一方面,第3段和第4段的用户花费较少,因此节省的也较少。 但是,在所有4个细分受众群中,细分受众群4用户的每位用户交易比例最高(平均每位用户超过9笔交易)。 这是转化率最高的用户群体。
每个细分市场中的用户总数和交易数量是多少? (What were the total number of users and the number of deals availed in each segment?)
Here is the total number of users and deals each segment users had availed.
这是每个细分用户可用的用户总数和交易数。
57% of users belonged to segment 3 and only 3% of users were from the most converted segment (i.e segment 4).
57%的用户属于第3部分,而只有3%的用户来自转化率最高的部分(即第4部分)。
总体用户支出是多少? (What were overall users spending?)
Here is the spread of spending by each segment
这是每个细分受众群的支出分布
Some of the users from segment 4 had high spending (yellow dots in segment 4) similar to segment 1 and 2 but segment 3 (which comprise of 57% of the users) didn't go for high spending deals and/or brands at all.
第4部分的一些用户具有较高的支出(第4部分中的黄点),类似于第1和第2部分,但第3部分(占57%的用户)根本没有进行高支出的交易和/或品牌推广。
每个细分市场用户偏好的品牌类型? (Type of brand each segment users preferred?)
Let’s look at what type of brand these segment users avail to understand any distinction in them.
让我们看看这些细分用户可以使用哪种类型的品牌来理解他们之间的任何区别。
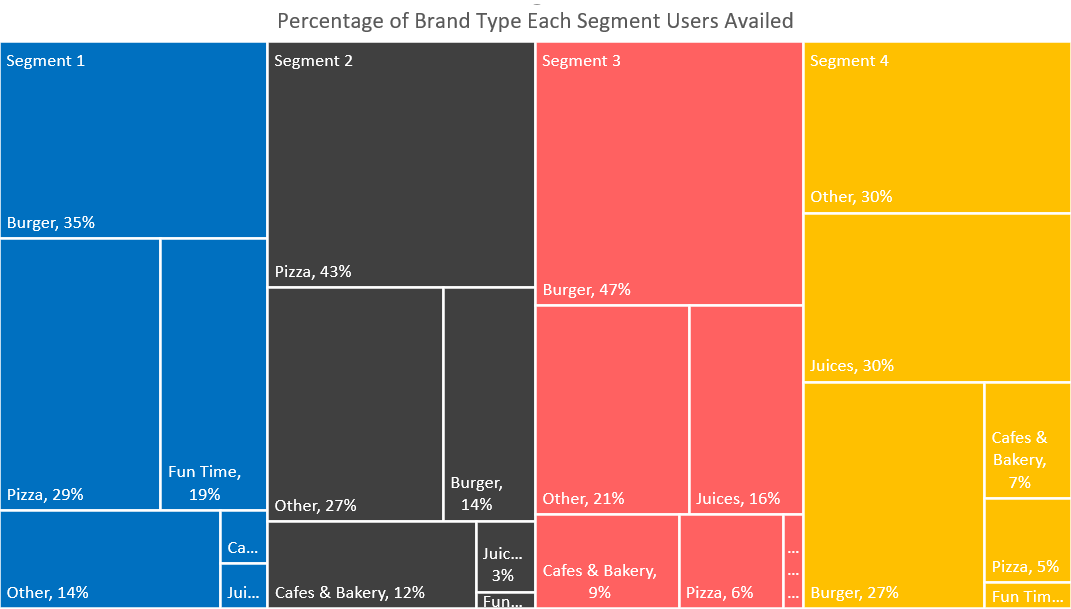
Segment 1 users had availed mix of burger, pizza and fun time, Segment 2 users had availed pizza and segment 3 users had preferred burgers. While Segment 4 users (most converted users) preferred juices and other types of brands.
第1部分用户使用了汉堡,比萨饼和娱乐时间,第2部分用户使用了比萨饼,第3部分用户则选择了汉堡。 而第4类用户(转化最多的用户)则更喜欢果汁和其他类型的品牌。
每个细分市场都有哪些品牌? (What brands each segment availed?.)
Here are the top 10 brands these segmented users had availed.
以下是这些细分用户所使用的十大品牌。
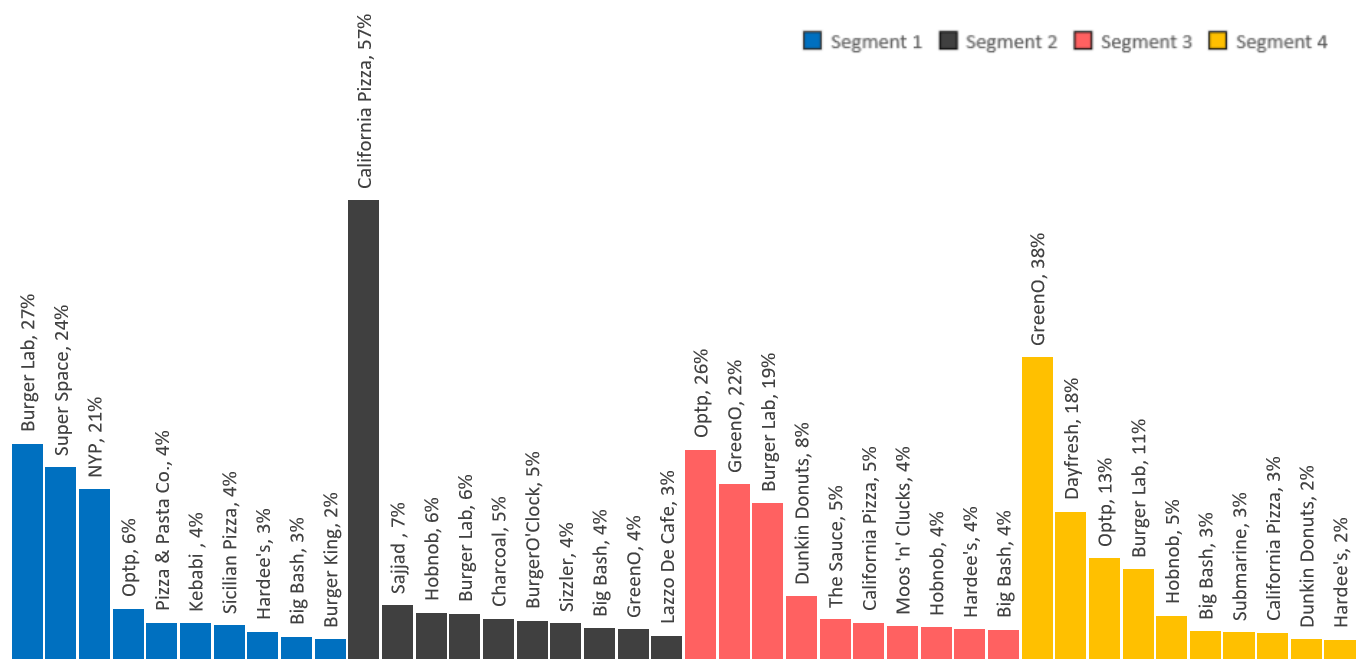
Looking at the brands we can comprehend what type of brand and deals these segment users would prefer. Segment 1 & 2 users (high paying users) had availed premium brands such as Sajjad, kababi, Charcoal, California, etc while segment 3 and 4 (low paying users) had mostly opted in for medium to low tier brands.
通过查看品牌,我们可以了解哪些类型的品牌以及这些细分用户希望的交易。 第1段和第2段用户(高收入用户)曾使用过Sajjad,kababi,木炭,加利福尼亚等高级品牌,而第3段和第4段(低收入用户)则大多选择了中低档品牌。
如何运用这些结果? (How these results can be employed?)
Based on different user segments we can:
根据不同的用户群,我们可以:
1- Targeted Ads Personalize ads for each segment would increase the conversion rate as users are more likely to convert on specific brands and offers. So, for example, show Sajjad’s ads to users with higher-paying power then to users with low paying power.
1-定位广告 每个细分受众群的个性化广告将提高转化率,因为用户更有可能转化为特定品牌和优惠。 因此,例如,向高支付能力的用户展示Sajjad的广告,然后向低支付能力的用户展示。
2- In-app RecommendationsOptimize the app to recommend deals and discounts within the app that each segment users would be more interested in.
2-应用内推荐优化应用,以推荐每个细分市场用户更感兴趣的应用内交易和折扣。
摘要 (Summary)
To sum up, with data and proper efforts we were able to identify interesting information about users and their liking and were able to strategies how to engage users more based on their preferences.
综上所述,通过数据和适当的努力,我们能够识别出有关用户及其喜好的有趣信息,并能够根据用户的喜好来制定如何与用户进行更多互动的策略。
翻译自: https://towardsdatascience.com/user-segmentation-based-on-purchase-history-490c57402d53
用户细分
本文来自互联网用户投稿,该文观点仅代表作者本人,不代表本站立场。本站仅提供信息存储空间服务,不拥有所有权,不承担相关法律责任。如若转载,请注明出处:http://www.mzph.cn/news/242150.shtml
如若内容造成侵权/违法违规/事实不符,请联系多彩编程网进行投诉反馈email:809451989@qq.com,一经查实,立即删除!