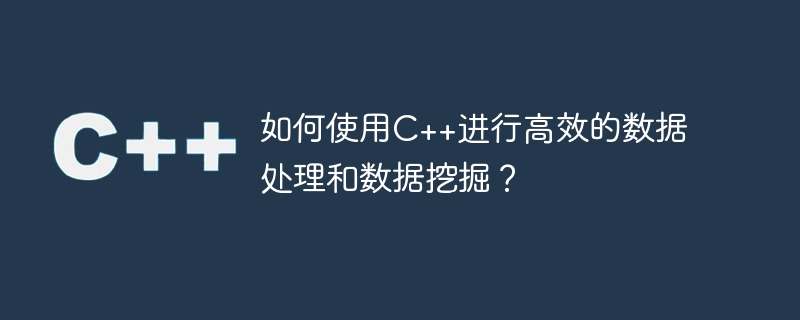
如何使用C++进行高效的数据处理和数据挖掘?
数据处理和数据挖掘在如今信息爆炸的时代变得越来越重要。为了快速、高效地处理和分析大量的数据,选择合适的编程语言非常重要。C++作为一种高性能的编程语言,在数据处理和数据挖掘领域也有广泛的应用。本文将介绍如何使用C++进行高效的数据处理和数据挖掘,并提供一些代码示例。
一、数据处理
- 文件读写
在数据处理中,文件的读写是非常常见的操作。C++提供了标准库中的fstream来实现文件读写。下面是一个读取文件内容的示例代码:
1 2 3 4 5 6 7 8 9 10 11 12 13 14 15 16 | #include <fstream> #include <iostream> int main() { std::ifstream file( "data.txt" ); // 打开文件 if (file.is_open()) { std::string line; while (std::getline(file, line)) { // 逐行读取文件内容 std::cout << line << std::endl; // 处理每一行数据 } file.close(); // 关闭文件 } else { std::cout << "无法打开文件" << std::endl; } return 0; } |
- 字符串处理
在数据处理中,字符串处理也是非常重要的一块。C++提供了std::string类来处理字符串,同时还提供了一些能够方便地操作字符串的函数。下面是一个字符串分割的示例代码:
1 2 3 4 5 6 7 8 9 10 11 12 13 14 15 16 17 18 19 20 21 22 23 | #include <iostream> #include <sstream> #include <string> #include <vector> std::vector<std::string> split( const std::string& str, char delimiter) { std::vector<std::string> result; std::stringstream ss(str); std::string token; while (std::getline(ss, token, delimiter)) { result.push_back(token); } return result; } int main() { std::string str = "Hello,World,!" ; std::vector<std::string> tokens = split(str, ',' ); for ( const auto & token : tokens) { std::cout << token << std::endl; } return 0; } |
- 数据结构
在数据处理中,合适的数据结构对于高效地存储和处理数据至关重要。C++提供了多种数据结构,如数组、向量、链表、哈希表等。选择合适的数据结构能够提高程序的执行效率。下面是一个数组排序的示例代码:
1 2 3 4 5 6 7 8 9 10 11 12 13 | #include <algorithm> #include <iostream> #include <vector> int main() { std::vector< int > numbers = {5, 1, 3, 2, 4}; std::sort(numbers.begin(), numbers.end()); // 数组排序 for ( const auto & number : numbers) { std::cout << number << " " ; } std::cout << std::endl; return 0; } |
二、数据挖掘
- 特征提取
在数据挖掘中,特征提取是一个非常重要的环节。合适的特征可以大大提高数据挖掘的准确性。C++提供了多种特征提取的方法和函数库,如OpenCV、Dlib等。下面是一个使用OpenCV提取图像特征的示例代码:
1 2 3 4 5 6 7 8 9 10 11 12 13 14 15 | #include <iostream> #include <opencv2/opencv.hpp> int main() { cv::Mat image = cv::imread( "image.jpg" ); // 读取图像 cv::SiftFeatureDetector detector; std::vector<cv::KeyPoint> keypoints; detector.detect(image, keypoints); // 提取特征点 cv::Mat descriptors; cv::SiftDescriptorExtractor extractor; extractor.compute(image, keypoints, descriptors); // 计算特征描述子 std::cout << "特征点数:" << keypoints.size() << std::endl; std::cout << "特征描述子维度:" << descriptors.cols << std::endl; return 0; } |
- 模型训练与预测
在数据挖掘中,模型训练和预测是一个非常重要的环节。C++提供了多种机器学习和深度学习库,如MLPACK、Tensorflow等。下面是一个使用MLPACK进行线性回归的示例代码:
1 2 3 4 5 6 7 8 9 10 11 12 13 14 15 16 17 | #include <iostream> #include <mlpack/methods/linear_regression/linear_regression.hpp> #include <mlpack/core/data/scaler_methods/mean_normalization.hpp> int main() { arma::mat X = arma::randu<arma::mat>(100, 2) * 10; // 生成训练数据 arma::vec y = 2 * X.col(0) + 3 * X.col(1) + arma::randn<arma::vec>(100); // 生成标签 mlpack::data::NormalizeParam normParams; // 特征归一化 mlpack::regression::LinearRegression lr(normParams); // 初始化线性回归模型 lr.Train(X, y); // 训练模型 arma::mat testX = arma::randu<arma::mat>(10, 2) * 10; // 生成测试数据 arma::vec testY; lr.Predict(testX, testY); // 预测结果 std::cout << "预测结果:" << std::endl; std::cout << testY << std::endl; return 0; } |
总结:
通过使用C++进行高效的数据处理和数据挖掘,我们可以更高效地处理和分析大量的数据。本文介绍了C++在数据处理和数据挖掘中的一些常用操作和技巧,并提供了相应的代码示例。希望本文对您在使用C++进行数据处理和数据挖掘方面有所帮助。