前言
最近看了很多大模型,也使用了很多大模型。对于大模型理论似乎很了解,但是好像又缺点什么,思来想去决定自己动手实现一个 toy 级别的模型,在实践中加深对大语言模型的理解。
在这个系列的文章中,我将通过亲手实践,构建一个 1.2B
的模型,完成模型搭建、tokenizer
训练、模型预训练和指令微调这些流程。记录整个开发过程和其中遇到的各种挑战和对应解决方案。
最后里面所有的内容都是我对于大模型的理解形成的,如果您发现有任何过时或不准确的地方,请不吝指出。
模型结构
现在大模型都是以选择 transformer
中 decoder
作为网络主体。对于 transformer
的解读可以参考我的这篇博客[《Attention Is All You Need 阅读详记-结合代码实现》]
模型配置
这里简要总结一下模型结构和每个网络层的关键参数。
以 Causal Language Model
为例,它主要包括如下结构:
-
Embedding Layer:将输入的离散的
token id
序列映射到连续、稠密的向量空间中,这里姑且将映射后的向量称为hidden_state
。他的关键参数为词表大小(token id 的取值范围) 和 映射后的维度。 -
Decoder Layer:网络的主体,多层
Decoder Layer
堆叠而成,单个Decoder Layer
一般由MultiHeadAttetion
、FeedForwardNetwork
和LayerNorm
组成,当然结构上还有残差连接。MultiHeadAttention
:多头注意力,关键参数为隐藏层维度、注意力头数。FeedForwardNetwork
:前馈神经网络,关键参数为隐藏层维度、上投影维度。LayerNorm
:在Llama
中采用RMSNorm
,关键参数为隐藏层维度 和 更新权重。
-
LanguageModelHead
:分类头,将hidden_state
转换为词表中token
选择概率,更严谨一点是logits
,关键参数为隐藏层维度 和 词表大小。
为了后面模型配置方便,我们先编写配置类,它包含了上面所有关键参数,还有一些没有提到的例如 Decoder Layer
层数,这样方便我们后面控制模型大小。
参数配置类实现较为简单,这里直接给出:
class CustomConfig:def __init__(self,vocab_size=151936,hidden_size=4096,intermediate_size=22016,num_hidden_layers=32,num_attention_heads=32,max_position_embeddings=32768,initializer_range=0.02,rms_norm_eps=1e-6,rope_theta=10000.0,attention_dropout=0.0,pad_token_id=1,) -> None:self.vocab_size = vocab_size# 方便初始化位置编码self.max_position_embeddings = max_position_embeddingsself.hidden_size = hidden_sizeself.intermediate_size = intermediate_sizeself.num_hidden_layers = num_hidden_layersself.num_attention_heads = num_attention_headsself.initializer_range = initializer_rangeself.rms_norm_eps = rms_norm_epsself.rope_theta = rope_thetaself.attention_dropout = attention_dropoutself.pad_token_id = pad_token_id
模型实现
下面开始依次完成模型结构。
Embedding Layer
在 Pytorch
中有实现,这里不单独抽出作为一个类。我们从 Decoder Layer
开始实现,首先实现最简单的部分 RMSNorm
。
RMSNorm
相较于 LayerNorm
没有去中心化操作,或者可以理解成输入数据的均值为0,然后进行归一化,他的公式如下:
RMSNorm(x)=xRMS(x)+ϵ∗WRMSNorm(x)=\frac{x}{\sqrt{RMS(x)+\epsilon}} * WRMSNorm(x)=RMS(x)+ϵx∗W
其中 RMS(x)
公式如下:
RMS(x)=1n∑xi2RMS(x)=\sqrt{\frac{1}{n}\sum{x_i^2}}RMS(x)=n1∑xi2
加入 ϵ\epsilonϵ 为了数值稳定,防止分母太小导致除零操作,其代码实现如下:
class CustomRMSNorm(nn.Module):"""实现 RMSNorm 层。LayerNorm 是减去样本均值,除以样本方差,然后乘以缩放参数。RMSNorm 可以看作均值为0的特殊情况。"""def __init__(self, hidden_size: int, eps: float = 1e-6) -> None:super().__init__()self.weight = nn.Parameter(torch.ones(hidden_size))self.eps = epsdef forward(self, hidden_states: torch.Tensor) -> torch.Tensor:input_dtype = hidden_states.dtypehidden_states = hidden_states.to(torch.float32)variance = hidden_states.pow(2).mean(-1, keepdim=True)hidden_states = hidden_states * torch.rsqrt(variance + self.eps)return self.weight * hidden_states.to(input_dtype)
下面实现 FeedForwardNetwork
,这个网络操作比较简单,就是将隐藏层状态升维后再降维,依次捕捉不同特征。现在的 FFN 通常会替换成带有门控的 FFN,其实现如下:
class CustomMLP(nn.Module):"""实现升维和降维"""def __init__(self, config) -> None:super().__init__()self.config = configself.hidden_size = config.hidden_sizeself.intermediate_size = config.intermediate_sizeself.gate_proj = nn.Linear(self.hidden_size, self.intermediate_size, bias=False)self.up_proj = nn.Linear(self.hidden_size, self.intermediate_size, bias=False)self.down_proj = nn.Linear(self.intermediate_size, self.hidden_size, bias=False)self.act_fn = nn.functional.geludef forward(self, x: torch.Tensor):gate = self.act_fn(self.gate_proj(x))up = self.up_proj(x) * gatereturn self.down_proj(up)
下面开始实现 MultiHeadAttention
,在实现这个层之前,先看一下经典公式
Attention(Q,K,V)=softmax(QKTdk)VAttention(Q,K,V)=softmax(\frac{QK^T}{\sqrt{d_k}})VAttention(Q,K,V)=softmax(dkQKT)V
在 Transformer
中 Q=WqXQ=W_{q}XQ=WqX ,其中 X=WE+PEX=W_E+P_EX=WE+PE。这样可以得到如下推导:
QKT=Wq(WE+PE)(WET+PET)WkT=Wq(WEWET+WEPET+PEWET+PEPET)WkTQKT=W_q(W_E+P_E)(W_E{T}+P_E{T})W_kT \\ =W_q(W_EW_ET+W_EP_ET+P_EW_ET+P_EP_ET)W_k^TQKT=Wq(WE+PE)(WET+PET)WkT=Wq(WEWET+WEPET+PEWET+PEPET)WkT
其中 WEWETW_EW_E^TWEWET 不携带位置信息,会破坏整体一致性(之前看到的一个观点,已经找不到出处了)。
而苏神提出旋转位置编码,很好解决了这一问题,使得 QKTQK^TQKT 结果很好注意到不同位置 token 之间的相对距离,在这里简要说明一下。
假设存在一个位于 m 位的 token 记作 xmx_mxm,对于这个 token,他的 Q 值计算记作 fq(xm)f_q(x_m)fq(xm),则在 Transformer
中是这样计算的:
fq(xm)=Wq(xm+pm)f_q(x_m) = W_q(x_m + p_m)fq(xm)=Wq(xm+pm)
在旋转位置编码中是这样计算的:
fq(xm)=(Wqxm)eimθf_q(x_m) = (W_qx_m)e^{im \theta}fq(xm)=(Wqxm)eimθ
因为没有加法运算,避免了对位置信息的破坏,下面来看内积如何携带相对位置信息。这里首先假设 xmx_mxm 是一个二维向量,即 xm=(xm1,xm2)Tx_m=(x_m1,x_m2)^Txm=(xm1,xm2)T。
则 WqW_qWq 是一个 2x2 矩阵,WqxmW_qx_mWqxm 的结果为二维向量,记作 qmq_mqm 。
由于我们可以使用复数表示一个二维向量,则 qm=qm1+iqm2q_m = q_m^1 + iq_m^2qm=qm1+iqm2。
再由欧拉公式可以得到 eimθ=cos(mθ)+isin(mθ)e^{im \theta}=cos(m \theta) + i sin(m \theta)eimθ=cos(mθ)+isin(mθ)
则旋转位置编码公式可以变为
fq(xm)=qmeimθ=(qm1+iqm2)(cos(mθ)+isin(mθ))=qm1cos(mθ)+iqm1sin(mθ)+iqm2cos(mθ)−qm2sin(mθ)=(qm1cos(mθ)−qm2sin(mθ))+i(qm1sin(mθ)+qm2cos(mθ))=(qm1cos(mθ)−qm2sin(mθ)qm1sin(mθ)+qm2cos(mθ))=(cos(mθ)−sin(mθ)sin(mθ)cos(mθ))(qm1qm2)f_q(x_m)=q_me^{im \theta}=(q_m1+iq_m2)(cos(m \theta) + i sin(m \theta)) \\ =q_m^1cos(m \theta) + iq_m^1sin(m \theta)+iq_m^2cos(m \theta)-q_m^2sin(m \theta) \\ =(q_m^1cos(m \theta)-q_m^2sin(m \theta))+i(q_m^1sin(m \theta)+q_m^2cos(m \theta)) \\ =\begin{pmatrix} q_m^1cos(m \theta)-q_m^2sin(m \theta) \\ q_m^1sin(m \theta)+q_m^2cos(m \theta) \end{pmatrix} \\ =\begin{pmatrix} cos(m\theta) & -sin(m\theta) \\ sin(m\theta) & cos(m\theta) \\ \end{pmatrix}\begin{pmatrix} q_m^1 \\ q_m^2\end{pmatrix}fq(xm)=qmeimθ=(qm1+iqm2)(cos(mθ)+isin(mθ))=qm1cos(mθ)+iqm1sin(mθ)+iqm2cos(mθ)−qm2sin(mθ)=(qm1cos(mθ)−qm2sin(mθ))+i(qm1sin(mθ)+qm2cos(mθ))=(qm1cos(mθ)−qm2sin(mθ)qm1sin(mθ)+qm2cos(mθ))=(cos(mθ)sin(mθ)−sin(mθ)cos(mθ))(qm1qm2)
同理
fk(xn)=(cos(nθ)−sin(nθ)sin(nθ)cos(nθ))(kn1kn2)f_k(x_n)=\begin{pmatrix} cos(n\theta) & -sin(n\theta) \\ sin(n\theta) & cos(n\theta) \\ \end{pmatrix}\begin{pmatrix} k_n^1 \\ k_n^2\end{pmatrix}fk(xn)=(cos(nθ)sin(nθ)−sin(nθ)cos(nθ))(kn1kn2)
可以看到编码后的向量实际上是编码前的向量乘了一个旋转矩阵,因此叫做旋转位置编码。
上面是二维情况,对于更高维可以进行两两分组,旋转矩阵进行拼接,这样得到高维旋转矩阵。但是这样得到的旋转矩阵是很稀疏的,推荐使用下面的方式实现旋转位置编码:
R(k)x=(cos(mθ0)cos(mθ0)cos(mθ1)cos(mθ1)…cos(mθd/2−1)cos(mθd/2−1))∘(x0x1x2x3…xd−2xd−1)+(sin(mθ0)sin(mθ0)sin(mθ1)sin(mθ1)…sin(mθd/2−1)sin(mθd/2−1))∘(−x1x0−x3x2…−xd−1xd−2)R(k)x= \begin{pmatrix} cos(m\theta_0) \\ cos(m\theta_0) \\ cos(m\theta_1) \\ cos(m\theta_1) \\ … \\ cos(m\theta_{d/2-1}) \\ cos(m\theta_{d/2-1}) \end{pmatrix} \circ \begin{pmatrix} x_0 \\ x_1 \\ x_2 \\ x_3 \\ … \\ x_{d-2} \\ x_{d-1} \end{pmatrix} + \begin{pmatrix} sin(m\theta_0) \\ sin(m\theta_0) \\ sin(m\theta_1) \\ sin(m\theta_1) \\ … \\ sin(m\theta_{d/2-1}) \\ sin(m\theta_{d/2-1}) \end{pmatrix} \circ \begin{pmatrix} -x_1 \\ x_0 \\ -x_3 \\ x_2 \\ … \\ -x_{d-1} \\ x_{d-2} \end{pmatrix} R(k)x=cos(mθ0)cos(mθ0)cos(mθ1)cos(mθ1)…cos(mθd/2−1)cos(mθd/2−1)∘x0x1x2x3…xd−2xd−1+sin(mθ0)sin(mθ0)sin(mθ1)sin(mθ1)…sin(mθd/2−1)sin(mθd/2−1)∘−x1x0−x3x2…−xd−1xd−2
上面不难看出核心思想是两两分组,在乘正弦的时候一半分组值取负数,因此具体实现时可以按照如下公式实现:
R(k)x=(cos(mθ0)cos(mθ1)…cos(mθd/2−1)cos(mθ0)cos(mθ1)…cos(mθd/2−1))∘(x0x1…xd/2−1xd/2xd/2+1…xd−1)+(sin(mθ0)sin(mθ1)…sin(mθd/2−1)sin(mθ0)sin(mθ1)…sin(mθd/2−1))∘(−xd/2−xd/2+1…−xd−1x0x1…xd/2−1)R(k)x= \begin{pmatrix} cos(m\theta_0) \\ cos(m\theta_1) \\ … \\ cos(m\theta_{d/2-1}) \\ cos(m\theta_0) \\ cos(m\theta_1) \\ … \\ cos(m\theta_{d/2-1}) \end{pmatrix} \circ \begin{pmatrix} x_0 \\ x_1 \\ … \\ x_{d/2-1} \\ x_{d/2} \\ x_{d/2+1} \\ … \\ x_{d-1} \end{pmatrix} + \begin{pmatrix} sin(m\theta_0) \\ sin(m\theta_1) \\ … \\ sin(m\theta_{d/2-1}) \\ sin(m\theta_0) \\ sin(m\theta_1) \\ … \\ sin(m\theta_{d/2-1}) \\ \end{pmatrix} \circ \begin{pmatrix} -x_{d/2} \\ -x_{d/2+1} \\ … \\ -x_{d-1} \\ x_0 \\ x_1 \\ … \\ x_{d/2-1} \end{pmatrix} R(k)x=cos(mθ0)cos(mθ1)…cos(mθd/2−1)cos(mθ0)cos(mθ1)…cos(mθd/2−1)∘x0x1…xd/2−1xd/2xd/2+1…xd−1+sin(mθ0)sin(mθ1)…sin(mθd/2−1)sin(mθ0)sin(mθ1)…sin(mθd/2−1)∘−xd/2−xd/2+1…−xd−1x0x1…xd/2−1
对应实现代码如下,我们关注查询和键的距离,所有对 q
和 k
进行旋转位置编码:
def rotate_half(x: torch.Tensor):"""将隐藏层一半维度旋转"""x1 = x[..., : x.shape[-1] // 2]x2 = x[..., x.shape[-1] // 2 :]return torch.cat((-x2, x1), dim=-1)def apply_rotary_pos_emb(q: torch.Tensor,k: torch.Tensor,cos: torch.Tensor,sin: torch.Tensor,position_ids: torch.Tensor,
) -> torch.Tensor:"""对 q 和 k 进行旋转位置编码:param q: 查询向量:param k: 关键词向量:param cos: 旋转位置编码余弦部分:param sin: 旋转位置编码正弦部分:param position_ids: 位置索引:return 使用旋转位置编码后的 q 和 k"""cos = cos[position_ids].unsqueeze(dim=1)sin = sin[position_ids].unsqueeze(dim=1)q_embed = (q * cos) + rotate_half(q) * sink_embed = (k * cos) + rotate_half(k) * sinreturn q_embed, k_embed
对应的旋转位置编码就有了如下实现:
class CustomRotaryEmbedding(nn.Module):"""实现旋转位置编码。"""def __init__(self,dim,max_position_embeddings: int = 2048,base: int = 10000,device: Union[str, torch.device] = None,) -> None:super().__init__()self.dim = dimself.max_position_embeddings = max_position_embeddingsself.base = baseinv_freq = 1.0 / (self.base ** (torch.arange(0, self.dim, 2).float().to(device) / self.dim))# 保存固定状态,但不成为模型参数self.register_buffer("inv_freq", inv_freq, persistent=False)self._set_cos_sin_cache(seq_len=self.max_position_embeddings,device=self.inv_freq.device,dtype=torch.get_default_dtype(),)def _set_cos_sin_cache(self, seq_len, device, dtype):"""设置 cos 和 sin 缓存。"""self.max_seq_len_cached = seq_lent = torch.arange(self.max_seq_len_cached, device=device, dtype=self.inv_freq.dtype)freqs = torch.outer(t, self.inv_freq)emb = torch.cat((freqs, freqs), dim=-1)# cos_cached / sin_cached 的 shape 为 (seq_len, dim)self.register_buffer("cos_cached", emb.cos().to(dtype), persistent=False)self.register_buffer("sin_cached", emb.sin().to(dtype), persistent=False)def forward(self, x: torch.Tensor, seq_len=None):if seq_len > self.max_position_embeddings:self._set_cos_sin_cache(seq_len=seq_len, device=x.device, dtype=x.dtype)return (self.cos_cached[:seq_len].to(x.dtype),self.sin_cached[:seq_len].to(x.dtype),)
有了旋转位置编码之后,多头注意力实现就很容易了。总体来说输入经过投影成为 q
、k
和 v
,然后 q
和 k
进行旋转位置编码后计算注意力权重,与 v
计算后经过一次投影输出。实现代码如下:
class CustomAttention(nn.Module):"""多头注意力机制"""def __init__(self, config) -> None:super().__init__()self.config = configself.hidden_size = self.config.hidden_sizeself.num_heads = self.config.num_attention_headsself.head_dim = self.hidden_size // self.num_headsself.max_position_embeddings = self.config.max_position_embeddingsself.rope_theta = self.config.rope_thetaself.attention_dropout = self.config.attention_dropoutif self.head_dim * self.num_heads != self.hidden_size:raise ValueError(f"hidden_size must be divisible by num_heads (got `hidden_size`: {self.hidden_size}"f" and `num_heads`: {self.num_heads}).")self.q_proj = nn.Linear(self.hidden_size, self.num_heads * self.head_dim, bias=True)self.k_proj = nn.Linear(self.hidden_size, self.num_heads * self.head_dim, bias=True)self.v_proj = nn.Linear(self.hidden_size, self.num_heads * self.head_dim, bias=True)self.o_proj = nn.Linear(self.num_heads * self.head_dim, self.hidden_size, bias=False)self.rotary_emb = CustomRotaryEmbedding(dim=self.head_dim,max_position_embeddings=self.max_position_embeddings,base=self.rope_theta,)def forward(self,hidden_states: torch.Tensor,attention_mask: Optional[torch.Tensor] = None,position_ids: Optional[torch.Tensor] = None,) -> torch.Tensor:bsz, seq_len, _ = hidden_states.size()query_states = self.q_proj(hidden_states)key_states = self.k_proj(hidden_states)value_states = self.v_proj(hidden_states)query_states = query_states.view(bsz, seq_len, self.num_heads, self.head_dim).transpose(1, 2)key_states = key_states.view(bsz, seq_len, self.num_heads, self.head_dim).transpose(1, 2)value_states = value_states.view(bsz, seq_len, self.num_heads, self.head_dim).transpose(1, 2)cos, sin = self.rotary_emb(value_states, seq_len=seq_len)query_states, key_states = apply_rotary_pos_emb(query_states, key_states, cos, sin, position_ids)attn_weights = torch.matmul(query_states, key_states.transpose(-1, -2)) / math.sqrt(self.head_dim)if attn_weights.size() != (bsz, self.num_heads, seq_len, seq_len):raise ValueError(f"Attention weights should be of size {(bsz, self.num_heads, seq_len, seq_len)}, but is"f" {attn_weights.size()}")if attention_mask is not None:if attention_mask.size() != (bsz, 1, seq_len, seq_len):raise ValueError(f"Attention mask should be of size {(bsz, 1, seq_len, seq_len)}, but is {attention_mask.size()}")# 使用混合精度时 -1e9 会报错 RuntimeError: value cannot be converted to type at::Half without overflow# attn_weights.masked_fill_(attention_mask, -1e4)# 设置为 float(-inf) 损失可能变成 nanattn_weights.masked_fill_(attention_mask, -1e9)attn_weights = nn.functional.softmax(attn_weights, dim=-1, dtype=torch.float32).to(query_states.dtype)attn_weights = nn.functional.dropout(attn_weights, p=self.attention_dropout, training=self.training)attn_output = torch.matmul(attn_weights, value_states)if attn_output.size() != (bsz, self.num_heads, seq_len, self.head_dim):raise ValueError(f"`attn_output` should be of size {(bsz, self.num_heads, seq_len, self.head_dim)}, but is"f" {attn_output.size()}")attn_output = attn_output.transpose(1, 2).contiguous()attn_output = attn_output.reshape(bsz, seq_len, self.hidden_size)attn_output = self.o_proj(attn_output)return attn_output
有了上面的组件,就可以组成一个 DecoderLayer
,基本流程就是先经过多头注意力,然后经过前馈网络,中间穿插着残差连接。因此可以有如下实现:
class CustomDecoderLayer(nn.Module):def __init__(self, config) -> None:super().__init__()self.hidden_size = config.hidden_sizeself.self_attn = CustomAttention(config)self.mlp = CustomMLP(config)self.input_layernorm = CustomRMSNorm(hidden_size=config.hidden_size, eps=config.rms_norm_eps)self.post_attention_layernorm = CustomRMSNorm(hidden_size=config.hidden_size, eps=config.rms_norm_eps)def forward(self,hidden_states: torch.Tensor,attention_mask: Optional[torch.Tensor] = None,position_ids: Optional[torch.Tensor] = None,) -> torch.Tensor:residual = hidden_states# layernorm 归一化hidden_states = self.input_layernorm(hidden_states)# 自注意力hidden_states = self.self_attn(hidden_states=hidden_states,attention_mask=attention_mask,position_ids=position_ids,)# 残差连接hidden_states += residual# 前馈网络部分residual = hidden_stateshidden_states = self.post_attention_layernorm(hidden_states)hidden_states = self.mlp(hidden_states)hidden_states += residualreturn hidden_states
到此,基本上完成了所有基础组件的搭建,下面开始组成预训练模型的基座。他的组成也很简单,就是 EmbeddingLayer
加上若干 DecoderLayer
,下面是实现代码。
由于大模型在训练中存储中间激活值需要占用大量显存,为了节省训练时候的显存,给出了梯度检查点方式,前向传播时只保存中间几个节点的激活值,在反向传播时,根据最近的保存点重新计算激活值,从而进行反向传播。
class CustomPreTrainedModel(nn.Module):def __init__(self, config) -> None:super().__init__()self.config = configself.padding_idx = config.pad_token_idself.vocab_size = config.vocab_sizeself.embed_tokens = nn.Embedding(config.vocab_size, config.hidden_size, padding_idx=self.padding_idx)self.layers = nn.ModuleList([CustomDecoderLayer(config) for _ in range(config.num_hidden_layers)])self.norm = CustomRMSNorm(config.hidden_size, eps=config.rms_norm_eps)self.graident_checkpoint = False_init_weights(config, self.modules())def forward(self,input_ids: Optional[torch.Tensor] = None,attention_mask: Optional[torch.Tensor] = None,position_ids: Optional[torch.Tensor] = None,input_embeds: Optional[torch.FloatTensor] = None,) -> torch.Tensor:# 对于输入的处理if input_ids is not None and input_embeds is not None:raise ValueError("You cannot specify both decoder_input_ids and decoder_inputs_embeds at the same time")elif input_ids is not None:_, seq_len = input_ids.shapeelif input_embeds is not None:_, seq_len, _ = input_embeds.shapeelse:raise ValueError("You have to specify either decoder_input_ids or decoder_inputs_embeds")# 位置索引if position_ids is None:device = input_ids.device if input_ids is not None else input_embeds.deviceposition_ids = torch.arange(seq_len, dtype=torch.long, device=device)position_ids = position_ids.unsqueeze(0).view(-1, seq_len)else:position_ids = position_ids.view(-1, seq_len).long()if input_embeds is None:input_embeds = self.embed_tokens(input_ids)attention_mask = _update_causal_mask(attention_mask, input_embeds)hidden_states = input_embedsfor decoder_layer in self.layers:if self.training and self.graident_checkpoint:layer_outputs = checkpoint(decoder_layer, hidden_states, attention_mask, position_ids)else:layer_outputs = decoder_layer(hidden_states,attention_mask=attention_mask,position_ids=position_ids,)hidden_states = layer_outputshidden_states = self.norm(hidden_states)return hidden_states
初始化模型参数可以采用 normal
或者 xavier_normal
方式进行初始化,这里给一个简单实现:
def _init_weights(config, modules):"""初始化权重,对 embedding 层进行特殊处理"""std = config.initializer_rangefor m in modules:if isinstance(m, nn.Linear):# nn.init.xavier_normal_(m.weight)m.weight.data.normal_(mean=0.0, std=std)if m.bias is not None:m.bias.data.zero_()elif isinstance(m, nn.Embedding):m.weight.data.normal_(mean=0.0, std=std)if m.padding_idx is not None:m.weight.data[m.padding_idx].zero_()
同时注意对于 attention_mask
也需要进行处理,因为输入是一个批次一起输入进来,对于较短的句子会进行 padding
操作,因此 attention_mask
需要考虑到因果注意力和填充的注意力。因果注意力是当前词不能注意到后面的词,填充注意力是指当前词不能注意到填充的无意义token。
def _update_causal_mask(attention_mask: torch.LongTensor, input_tensor: torch.FloatTensor
) -> torch.Tensor:"""创建 causal_mask:param attention_mask: (bsz, seq_len):param input_tensor: (bsz, seq_len, hidden_size)"""device = input_tensor.deviceif input_tensor.dim() == 3:bsz, seq_len, _ = input_tensor.shapeelif input_tensor.dim() == 2:bsz, seq_len = input_tensor.shapeelse:raise ValueError(f"Input tensor should have 2 or 3 dimensions, but has {input_tensor.dim()}")assert (bsz == attention_mask.shape[0]), f"batch size should be equal, but got {bsz} and {attention_mask.shape[0]}"# 处理 causal_maskcausal_mask = torch.triu(torch.ones(seq_len, seq_len), diagonal=1).bool().to(device)# 处理 padding maskif attention_mask.dim() == 2:padding_mask = attention_mask[:, None, None, :] # (bsz, 1, 1, seq_len)elif attention_mask.dim() == 4:padding_mask = attention_maskelse:raise ValueError(f"Attention mask dim should be `2` or `4`, but is {attention_mask.dim()}")padding_mask = (padding_mask == 0).to(device)combined_mask = padding_mask | causal_maskreturn combined_mask
最后我们的语言模型就是在基座上面加入一个分类头,输出为词表大小的概率分布,这样我们可以根据概率选择下一个词是什么。
class CustomForCausalLM(nn.Module):def __init__(self, config) -> None:super().__init__()self.model = CustomPreTrainedModel(config)self.vocab_size = config.vocab_sizeself.lm_head = nn.Linear(config.hidden_size, config.vocab_size, bias=False)_init_weights(config, self.modules())def enable_gradient_checkpoint(self):self.model.graident_checkpoint = Truedef forward(self,input_ids: torch.Tensor,attention_mask: Optional[torch.Tensor] = None,position_ids: Optional[torch.Tensor] = None,input_embeds: Optional[torch.FloatTensor] = None,labels: Optional[torch.LongTensor] = None,) -> Tuple[torch.Tensor, torch.Tensor]:outputs = self.model(input_ids=input_ids,attention_mask=attention_mask,position_ids=position_ids,input_embeds=input_embeds,)logits: torch.Tensor = self.lm_head(outputs)loss = Noneif labels is not None:shift_logits = logits[..., :-1, :].contiguous()shift_labels = labels[..., 1:].contiguous()loss_fct = nn.CrossEntropyLoss()shift_logits = shift_logits.view(-1, self.vocab_size) # [bsz, seq_len, vocab] => [bsz * seq_len, vocab]shift_labels = shift_labels.view(-1) # [bsz, seq_len] => [bsz * seq_len]shift_labels = shift_labels.to(shift_logits.device)loss = loss_fct(shift_logits, shift_labels)return (logits, loss)
注意我们的训练目标是预测下一个词,例如输入是“我有一个苹果”,模型是按照如下步骤进行预测:
输入 | 标签 | 模型输出 |
---|---|---|
我 | 有 | token1 |
我有 | 一 | token2 |
我有一 | 个 | token3 |
我有一个 | 苹 | token4 |
我有一个苹 | 果 | token5 |
我有一个苹果 | NULL | token6 |
由于 attention_mask
的存在,我们输入“我有一个苹果”,上述过程可以并行发生。因此实际上的标签是 “有一个苹果”,对应有标签的模型输出是 token1 ~ token5。所以我们在计算损失的时候有个位移操作,这样才能正确对齐模型预测和标签。
结语
至此,我们终于实现了一个自己的小语言模型,现在他有了骨骼但是还没有肌肉,想要有语言能力还需要对他进行训练。对他进行训练前,首先我们要准备文本数据,然后进行切词,转换成向量,最后才能输入模型并且进行训练。下一篇我们实现一个分词器,有了分词器,模型就可以接受外部知识了。
那么,我们该如何学习大模型?
作为一名热心肠的互联网老兵,我决定把宝贵的AI知识分享给大家。 至于能学习到多少就看你的学习毅力和能力了 。我已将重要的AI大模型资料包括AI大模型入门学习思维导图、精品AI大模型学习书籍手册、视频教程、实战学习等录播视频免费分享出来。
一、大模型全套的学习路线
学习大型人工智能模型,如GPT-3、BERT或任何其他先进的神经网络模型,需要系统的方法和持续的努力。既然要系统的学习大模型,那么学习路线是必不可少的,下面的这份路线能帮助你快速梳理知识,形成自己的体系。
L1级别:AI大模型时代的华丽登场
L2级别:AI大模型API应用开发工程
L3级别:大模型应用架构进阶实践
L4级别:大模型微调与私有化部署
一般掌握到第四个级别,市场上大多数岗位都是可以胜任,但要还不是天花板,天花板级别要求更加严格,对于算法和实战是非常苛刻的。建议普通人掌握到L4级别即可。
以上的AI大模型学习路线,不知道为什么发出来就有点糊,高清版可以微信扫描下方CSDN官方认证二维码免费领取【保证100%免费
】
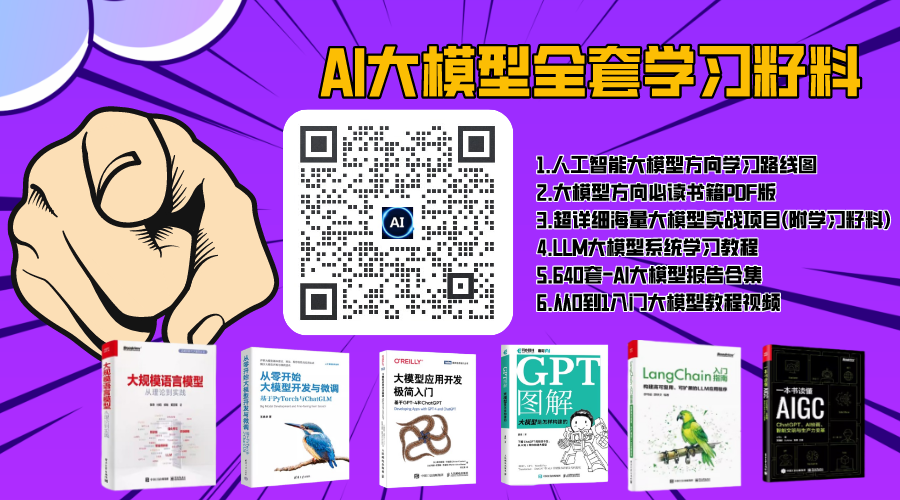
二、640套AI大模型报告合集
这套包含640份报告的合集,涵盖了AI大模型的理论研究、技术实现、行业应用等多个方面。无论您是科研人员、工程师,还是对AI大模型感兴趣的爱好者,这套报告合集都将为您提供宝贵的信息和启示。
三、大模型经典PDF籍
随着人工智能技术的飞速发展,AI大模型已经成为了当今科技领域的一大热点。这些大型预训练模型,如GPT-3、BERT、XLNet等,以其强大的语言理解和生成能力,正在改变我们对人工智能的认识。 那以下这些PDF籍就是非常不错的学习资源。
四、AI大模型商业化落地方案
作为普通人,入局大模型时代需要持续学习和实践,不断提高自己的技能和认知水平,同时也需要有责任感和伦理意识,为人工智能的健康发展贡献力量。