视觉 Transformer 中位置嵌入背后的数学和代码简介。
自从 2017 年推出《Attention is All You Need》以来,Transformer 已成为自然语言处理 (NLP) 领域最先进的技术。 2021 年,An Image is Worth 16x16 Words² 成功地将 Transformer 应用于计算机视觉任务。从那时起,人们提出了许多基于Transformer的计算机视觉架构。
本文[1]研究了为什么位置嵌入是视觉Transformer的必要组成部分,以及不同的论文如何实现位置嵌入。它包括位置嵌入的开源代码以及概念解释。所有代码都使用 PyTorch 包。
为什么使用位置嵌入?
Attention is All You Need 指出,Transformer由于缺乏递归或卷积,无法学习有关一组标记顺序的信息。如果没有位置嵌入,Transformer对于标记的顺序是不变的。对于图像,这意味着可以对图像的补丁进行加扰,而不会影响预测的输出。
让我们看一下 Luis Zuno 的像素艺术《黄昏山》中补丁顺序的示例。原始艺术作品已被裁剪并转换为单通道图像。这意味着每个像素都有一个介于 0 和 1 之间的值。单通道图像通常以灰度显示;但是,我们将以紫色配色显示它,因为它更容易看到。
mountains = np.load(os.path.join(figure_path, 'mountains.npy'))
H = mountains.shape[0]
W = mountains.shape[1]
print('Mountain at Dusk is H =', H, 'and W =', W, 'pixels.')
print('\n')
fig = plt.figure(figsize=(10,6))
plt.imshow(mountains, cmap='Purples_r')
plt.xticks(np.arange(-0.5, W+1, 10), labels=np.arange(0, W+1, 10))
plt.yticks(np.arange(-0.5, H+1, 10), labels=np.arange(0, H+1, 10))
plt.clim([0,1])
cbar_ax = fig.add_axes([0.95, .11, 0.05, 0.77])
plt.clim([0, 1])
plt.colorbar(cax=cbar_ax);
#plt.savefig(os.path.join(figure_path, 'mountains.png'), bbox_inches='tight')
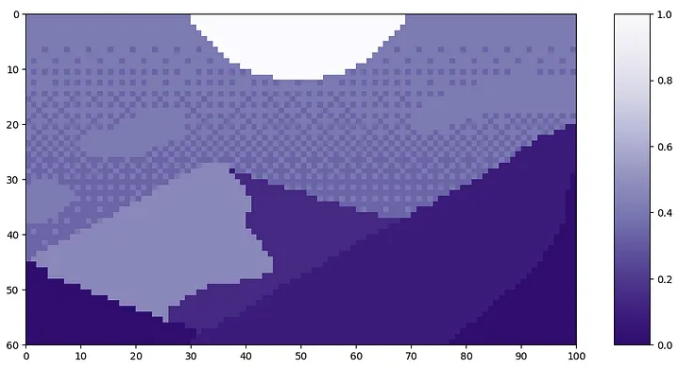
我们可以将此图像分割成大小为 20 的块。
P = 20
N = int((H*W)/(P**2))
print('There will be', N, 'patches, each', P, 'by', str(P)+'.')
print('\n')
fig = plt.figure(figsize=(10,6))
plt.imshow(mountains, cmap='Purples_r')
plt.clim([0,1])
plt.hlines(np.arange(P, H, P)-0.5, -0.5, W-0.5, color='w')
plt.vlines(np.arange(P, W, P)-0.5, -0.5, H-0.5, color='w')
plt.xticks(np.arange(-0.5, W+1, 10), labels=np.arange(0, W+1, 10))
plt.yticks(np.arange(-0.5, H+1, 10), labels=np.arange(0, H+1, 10))
x_text = np.tile(np.arange(9.5, W, P), 3)
y_text = np.repeat(np.arange(9.5, H, P), 5)
for i in range(1, N+1):
plt.text(x_text[i-1], y_text[i-1], str(i), color='w', fontsize='xx-large', ha='center')
plt.text(x_text[2], y_text[2], str(3), color='k', fontsize='xx-large', ha='center');
#plt.savefig(os.path.join(figure_path, 'mountain_patches.png'), bbox_inches='tight')
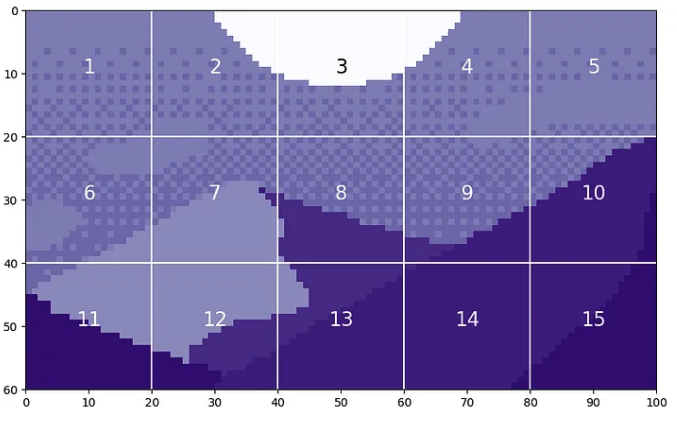
据称,视觉Transformer将无法区分原始图像和补丁被打乱的版本。
np.random.seed(21)
scramble_order = np.random.permutation(N)
left_x = np.tile(np.arange(0, W-P+1, 20), 3)
right_x = np.tile(np.arange(P, W+1, 20), 3)
top_y = np.repeat(np.arange(0, H-P+1, 20), 5)
bottom_y = np.repeat(np.arange(P, H+1, 20), 5)
scramble = np.zeros_like(mountains)
for i in range(N):
t = scramble_order[i]
scramble[top_y[i]:bottom_y[i], left_x[i]:right_x[i]] = mountains[top_y[t]:bottom_y[t], left_x[t]:right_x[t]]
fig = plt.figure(figsize=(10,6))
plt.imshow(scramble, cmap='Purples_r')
plt.clim([0,1])
plt.hlines(np.arange(P, H, P)-0.5, -0.5, W-0.5, color='w')
plt.vlines(np.arange(P, W, P)-0.5, -0.5, H-0.5, color='w')
plt.xticks(np.arange(-0.5, W+1, 10), labels=np.arange(0, W+1, 10))
plt.yticks(np.arange(-0.5, H+1, 10), labels=np.arange(0, H+1, 10))
x_text = np.tile(np.arange(9.5, W, P), 3)
y_text = np.repeat(np.arange(9.5, H, P), 5)
for i in range(N):
plt.text(x_text[i], y_text[i], str(scramble_order[i]+1), color='w', fontsize='xx-large', ha='center')
i3 = np.where(scramble_order==2)[0][0]
plt.text(x_text[i3], y_text[i3], str(scramble_order[i3]+1), color='k', fontsize='xx-large', ha='center');
#plt.savefig(os.path.join(figure_path, 'mountain_scrambled_patches.png'), bbox_inches='tight')
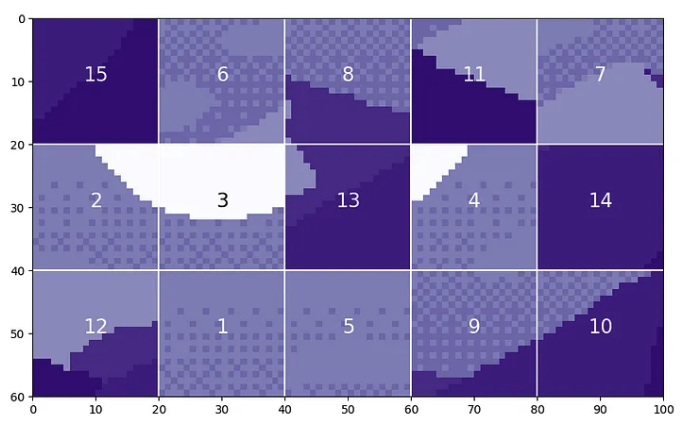
显然,这是与原始图像非常不同的图像,并且您不希望视觉Transformer将这两个图像视为相同。
排列的注意力不变性
让我们研究一下视觉Transformer对于标记顺序不变的说法。Transformer中对 token 顺序不变的组件是注意力模块。
注意力是根据三个矩阵(查询、键和值)计算得出的,每个矩阵都是通过将token传递到线性层而生成的。生成 Q、K 和 V 矩阵后,将使用以下公式计算注意力。

其中 Q、K、V 分别是查询、键和值; dₖ 是缩放值。为了证明注意力对 token 顺序的不变性,我们将从三个随机生成的矩阵开始来表示 Q、K 和 V。Q、K 和 V 的形状如下:
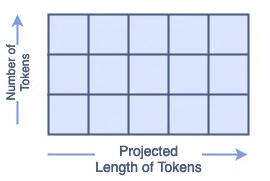
在此示例中,我们将使用 4 个预计长度为 9 的标记。矩阵将包含整数以避免浮点乘法错误。生成后,我们将交换token 0 和token 2 在所有三个矩阵中的位置。具有交换标记的矩阵将用下标 s 表示。
n_tokens = 4
l_tokens = 9
shape = n_tokens, l_tokens
mx = 20 #max integer for generated matricies
# Generate Normal Matricies
np.random.seed(21)
Q = np.random.randint(1, mx, shape)
K = np.random.randint(1, mx, shape)
V = np.random.randint(1, mx, shape)
# Generate Row-Swapped Matricies
swapQ = copy.deepcopy(Q)
swapQ[[0, 2]] = swapQ[[2, 0]]
swapK = copy.deepcopy(K)
swapK[[0, 2]] = swapK[[2, 0]]
swapV = copy.deepcopy(V)
swapV[[0, 2]] = swapV[[2, 0]]
# Plot Matricies
fig, axs = plt.subplots(nrows=3, ncols=2, figsize=(8,8))
fig.tight_layout(pad=2.0)
plt.subplot(3, 2, 1)
mat_plot(Q, 'Q')
plt.subplot(3, 2, 2)
mat_plot(swapQ, r'$Q_S$')
plt.subplot(3, 2, 3)
mat_plot(K, 'K')
plt.subplot(3, 2, 4)
mat_plot(swapK, r'$K_S$')
plt.subplot(3, 2, 5)
mat_plot(V, 'V')
plt.subplot(3, 2, 6)
mat_plot(swapV, r'$V_S$')
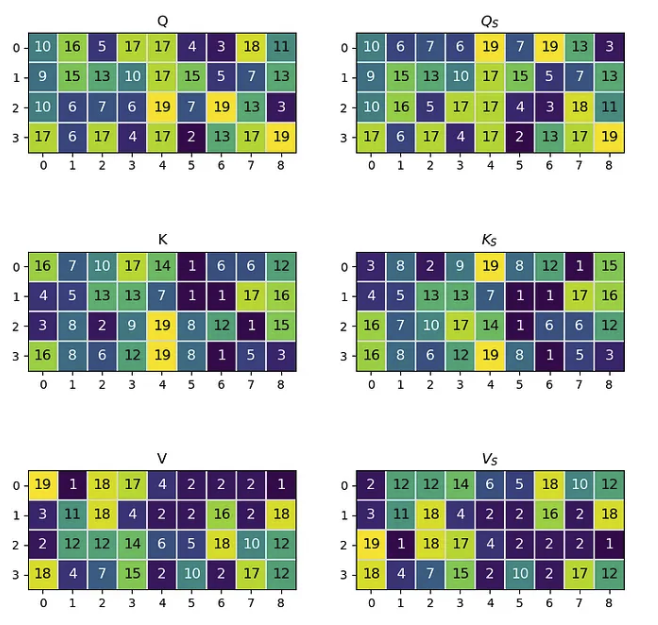
注意力公式中的第一个矩阵乘法是 Q·Kᵀ=A,其中得到的矩阵 A 是一个大小等于 token 数量的正方形。当我们用 Qₛ 和 Kₛ 计算 Aₛ 时,得到的 Aₛ 的行 [0, 2] 和列 [0,2] 都与 A 交换。
A = Q @ K.transpose()
swapA = swapQ @ swapK.transpose()
modA = copy.deepcopy(A)
modA[[0,2]] = modA[[2,0]] #swap rows
modA[:, [2, 0]] = modA[:, [0, 2]] #swap cols
fig, axs = plt.subplots(nrows=1, ncols=3, figsize=(8,3))
fig.tight_layout(pad=1.0)
plt.subplot(1, 3, 1)
mat_plot(A, r'$A = Q*K^T$')
plt.subplot(1, 3, 2)
mat_plot(swapA, r'$A_S = Q_S * K_S^T$')
plt.subplot(1, 3, 3)
mat_plot(modA, 'A\nwith rows [0,2] swaped\n and cols [0,2] swaped')
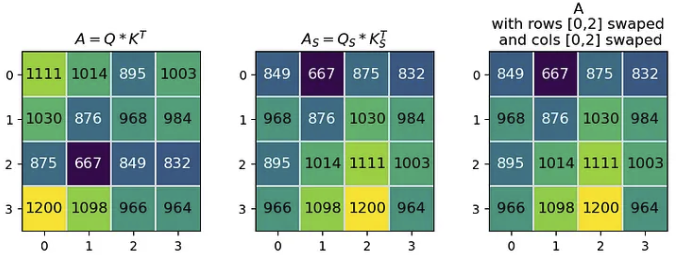
下一个矩阵乘法是 A·V=A,其中生成的矩阵 A 与初始 Q、K 和 V 矩阵具有相同的形状。当我们用 Aₛ 和 Vₛ 计算 Aₛ 时,得到的 Aₛ 的行 [0,2] 与 A 交换。
A = A @ V
swapA = swapA @ swapV
modA = copy.deepcopy(A)
modA[[0,2]] = modA[[2,0]] #swap rows
fig, axs = plt.subplots(nrows=2, ncols=2, figsize=(12, 7))
fig.tight_layout(pad=1.0)
plt.subplot(2, 2, 1)
mat_plot(A, r'$A = A*V$')
plt.subplot(2, 2, 2)
mat_plot(swapA, r'$A_S = A_S * V_S$')
plt.subplot(2, 2, 4)
mat_plot(modA, 'A\nwith rows [0,2] swaped')
axs[1,0].axis('off')
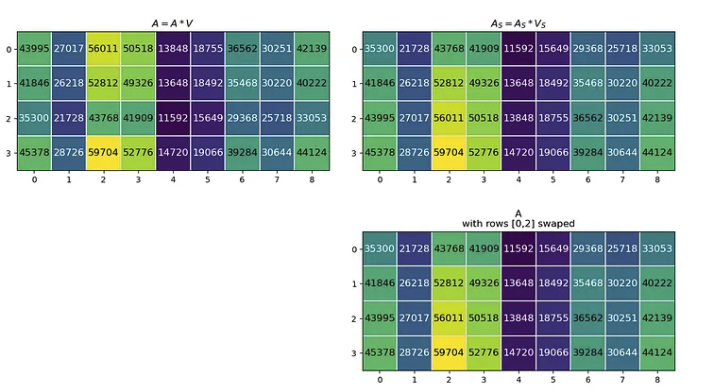
这表明,更改注意力层输入中标记的顺序会导致输出注意矩阵的相同标记行发生变化。因为注意力是对标记之间关系的计算。如果没有位置信息,更改token顺序不会改变token的关联方式。
示例
定义位置嵌入
现在,我们可以看看正弦位置嵌入的细节。该代码基于 Tokens-to-Token ViT 的公开可用 GitHub 代码。从功能上来说,位置嵌入是一个与 token 形状相同的矩阵。这看起来像:
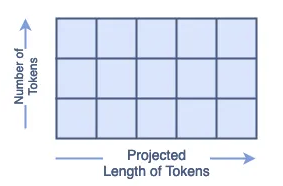
正弦位置嵌入公式如下所示
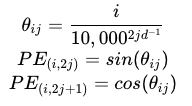
其中 PE 是位置嵌入矩阵,i 是沿着标记的数量,j 是沿着标记的长度,d 是标记长度。代码实现:
def get_sinusoid_encoding(num_tokens, token_len):
""" Make Sinusoid Encoding Table
Args:
num_tokens (int): number of tokens
token_len (int): length of a token
Returns:
(torch.FloatTensor) sinusoidal position encoding table
"""
def get_position_angle_vec(i):
return [i / np.power(10000, 2 * (j // 2) / token_len) for j in range(token_len)]
sinusoid_table = np.array([get_position_angle_vec(i) for i in range(num_tokens)])
sinusoid_table[:, 0::2] = np.sin(sinusoid_table[:, 0::2])
sinusoid_table[:, 1::2] = np.cos(sinusoid_table[:, 1::2])
return torch.FloatTensor(sinusoid_table).unsqueeze(0)
让我们生成一个示例位置嵌入矩阵。我们将使用 176 个tokens。每个token的长度为 768,这是 T2T-ViT代码中的默认长度。一旦生成了矩阵,我们就可以绘制它。
PE = get_sinusoid_encoding(num_tokens=176, token_len=768)
fig = plt.figure(figsize=(10, 8))
plt.imshow(PE[0, :, :], cmap='PuOr_r')
plt.xlabel('Along Length of Token')
plt.ylabel('Individual Tokens');
cbar_ax = fig.add_axes([0.95, .36, 0.05, 0.25])
plt.clim([-1, 1])
plt.colorbar(label='Value of Position Encoding', cax=cbar_ax);
#plt.savefig(os.path.join(figure_path, 'fullPE.png'), bbox_inches='tight')
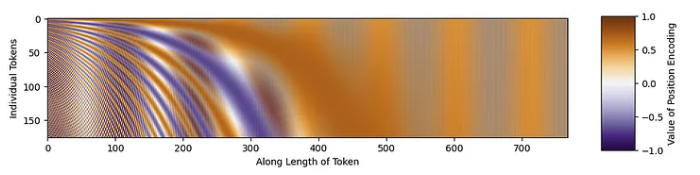
放大标记的开头。
fig = plt.figure()
plt.imshow(PE[0, :, 0:301], cmap='PuOr_r')
plt.xlabel('Along Length of Token')
plt.ylabel('Individual Tokens');
cbar_ax = fig.add_axes([0.95, .2, 0.05, 0.6])
plt.clim([-1, 1])
plt.colorbar(label='Value of Position Encoding', cax=cbar_ax);
#plt.savefig(os.path.join(figure_path, 'zoomedinPE.png'), bbox_inches='tight')
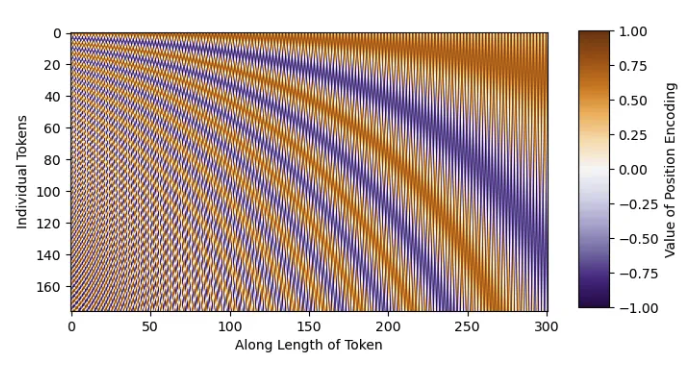
具有正弦结构!
将位置嵌入应用于tokens
现在,我们可以将位置嵌入添加到我们的tokens中!我们将使用《Mountain at Dusk》,并具有与上述相同的补丁标记化。这将为我们提供 15 个长度为 20²=400 的token。
fig = plt.figure(figsize=(10,6))
plt.imshow(mountains, cmap='Purples_r')
plt.hlines(np.arange(P, H, P)-0.5, -0.5, W-0.5, color='w')
plt.vlines(np.arange(P, W, P)-0.5, -0.5, H-0.5, color='w')
plt.xticks(np.arange(-0.5, W+1, 10), labels=np.arange(0, W+1, 10))
plt.yticks(np.arange(-0.5, H+1, 10), labels=np.arange(0, H+1, 10))
x_text = np.tile(np.arange(9.5, W, P), 3)
y_text = np.repeat(np.arange(9.5, H, P), 5)
for i in range(1, N+1):
plt.text(x_text[i-1], y_text[i-1], str(i), color='w', fontsize='xx-large', ha='center')
plt.text(x_text[2], y_text[2], str(3), color='k', fontsize='xx-large', ha='center')
cbar_ax = fig.add_axes([0.95, .11, 0.05, 0.77])
plt.clim([0, 1])
plt.colorbar(cax=cbar_ax);
#plt.savefig(os.path.join(figure_path, 'mountain_patches_w_colorbar.png'), bbox_inches='tight')
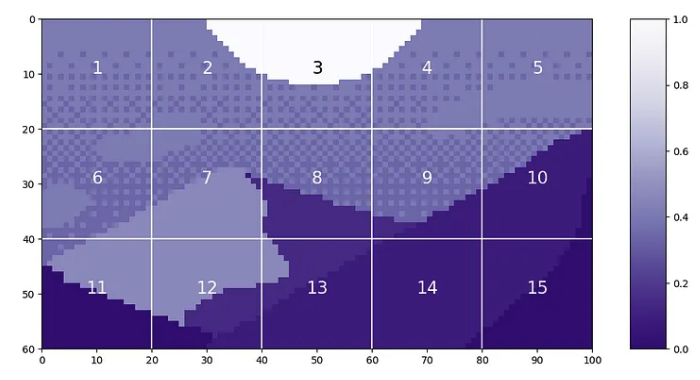
当我们将这些补丁转换为token时,它看起来像
tokens = np.zeros((15, 20**2))
for i in range(15):
patch = gray_mountains[top_y[i]:bottom_y[i], left_x[i]:right_x[i]]
tokens[i, :] = patch.reshape(1, 20**2)
tokens = tokens.astype(int)
tokens = tokens/255
fig = plt.figure(figsize=(10,6))
plt.imshow(tokens, aspect=5, cmap='Purples_r')
plt.xlabel('Length of Tokens')
plt.ylabel('Number of Tokens')
cbar_ax = fig.add_axes([0.95, .36, 0.05, 0.25])
plt.clim([0, 1])
plt.colorbar(cax=cbar_ax)
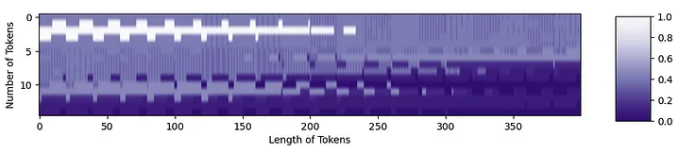
现在,我们可以以正确的形状进行位置嵌入:
PE = get_sinusoid_encoding(num_tokens=15, token_len=400).numpy()[0,:,:]
fig = plt.figure(figsize=(10,6))
plt.imshow(PE, aspect=5, cmap='PuOr_r')
plt.xlabel('Length of Tokens')
plt.ylabel('Number of Tokens')
cbar_ax = fig.add_axes([0.95, .36, 0.05, 0.25])
plt.clim([0, 1])
plt.colorbar(cax=cbar_ax)
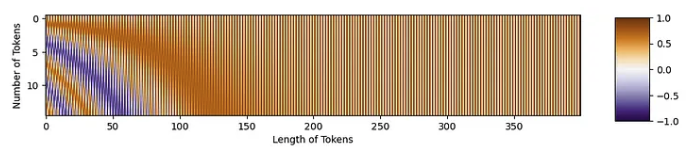
我们现在准备将位置嵌入添加到标记中。位置嵌入中的紫色区域将使令牌变暗,而橙色区域将使它们变亮。
mountainsPE = tokens + PE
resclaed_mtPE = (position_mountains - np.min(position_mountains)) / np.max(position_mountains - np.min(position_mountains))
fig = plt.figure(figsize=(10,6))
plt.imshow(resclaed_mtPE, aspect=5, cmap='Purples_r')
plt.xlabel('Length of Tokens')
plt.ylabel('Number of Tokens')
cbar_ax = fig.add_axes([0.95, .36, 0.05, 0.25])
plt.clim([0, 1])
plt.colorbar(cax=cbar_ax)
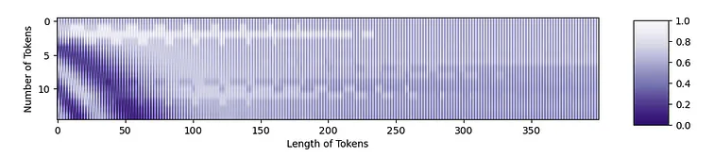
您可以从原始token中看到结构,以及位置嵌入中的结构!这两条信息都将被转发到Transformer中。
Source: https://towardsdatascience.com/position-embeddings-for-vision-transformers-explained-a6f9add341d5
本文由 mdnice 多平台发布