背景:数据科学领域中,数据一直都是主要驱动力,特征工程作为其中重要一环,成为无论是kaggle类的数据竞赛,还是工业界应用中关注的重点。特征工程中有重要的一个环节叫做特征融合,好的特征融合能帮助构造当前模型不能学习到的知识,通常产生新的特征会很依赖于专家知识,当在缺乏专家知识的情况下,我们就需要一款工具帮我们自动生成特征。因此gplearn就主要产生了。
简介:待补充
gplearn特征生成使用案例:以官方给出的boston房屋数据为例
- 安装
pip install gplearn #python3.7版本
pip install gplearn==0.3.0 #python2.7版本,当前0.4版本的gplearn不再支持python2.7
- 引入库
from sklearn.datasets import load_boston
from gplearn.genetic import SymbolicTransformer›
import pandas as pd
import numpy as np
import gplearn as gp
- 数据导入
def data_prepare():boston = load_boston()boston_feature = pd.DataFrame(boston.data, columns=boston.feature_names)boston_label = pd.Series(boston.target).to_frame("TARGET")boston = pd.concat([boston_label, boston_feature], axis=1)return bostondata = data_prepare()
- 自定义可计算的算子:logical算子使用官方给定案例,自定义算子通过make_function()实现,这里我自定义一个box-cox算子(lamda = 2),注意一定要有报错机制,比如np.errstate,不然不会通过。官方自定义的‘add’等算子可以直接使用
def _logical(x1,x2,x3,x4):return np.where(x1 > x2,x3,x4)
logical = gp.functions.make_function(function = _logical,name = 'logical',arity = 4)
def _boxcox2(x1):with np.errstate(over='ignore', under='ignore'):return (np.power(x1,2)-1)/2
binary = gp.functions.make_function(function = _binary,name = 'binary',arity = 1)
function_set = ['add', 'sub', 'mul', 'div', 'log', 'sqrt', 'abs', 'neg','inv','sin','cos','tan', 'max', 'min',boxcox2,logical]
- 初始化
gp1 = SymbolicTransformer(generations=1, population_size=1000,hall_of_fame=600, n_components=100,function_set=function_set,parsimony_coefficient=0.0005,max_samples=0.9, verbose=1,random_state=0, n_jobs=3)
- 生成新特征
label = data['TARGET']
train = data.drop(columns=['TARGET'])
gp1.fit(train,label)
new_df2 = gp1.transform(train)
- 查看新生成的特征
from IPython.display import Image
import pydotplus
graph = gp1._best_programs[0].export_graphviz()
graph = pydotplus.graphviz.graph_from_dot_data(graph)
Image(graph.create_png())
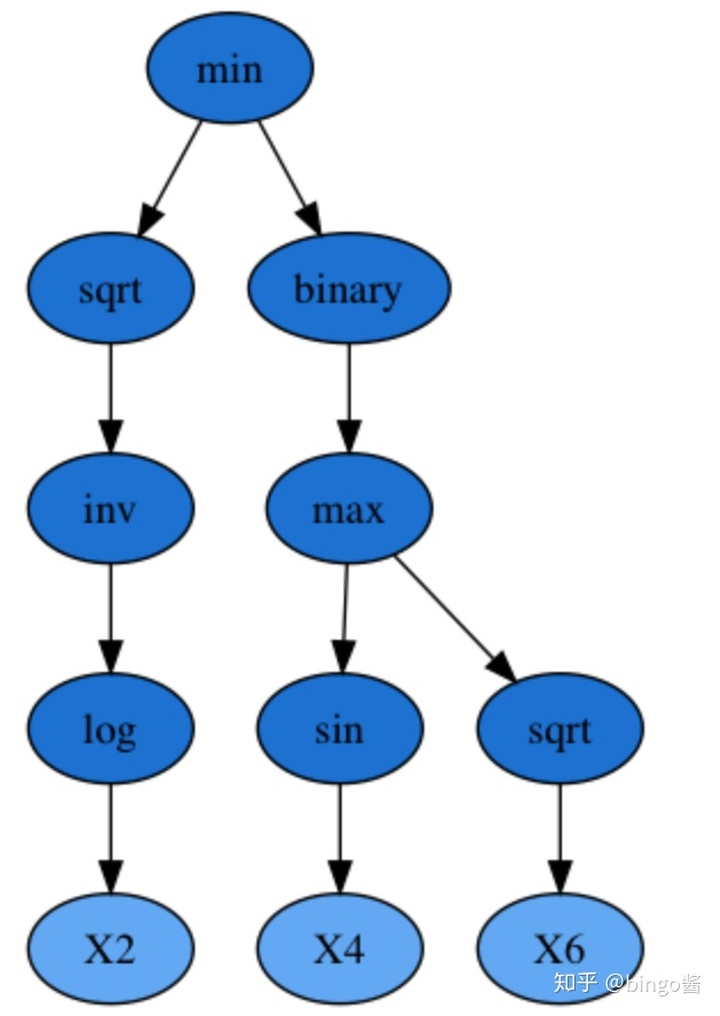
gplearn参数的介绍
- unction_set。用于构成程式的函数(算子)集合
- tournament_size。参与遗传算法计算下一代特征的特征数量(控制特征之间的相关性)
- stopping_criteria。遗传算法停止条件
- init_depth。初始化的树深度
- parsimony coefficient。简化系数
- p_hoist_mutation。提升变异系数
- max_samples。最大采样比例
- hall_of_fame。备选数量
- const_range。混入程式的常数范围
- init_method。初始化方法
- metric。适应度标准
- p_crossover。交叉变异系数
- warm_start。热启动
- n_jobs。并行计算使用的核心数量
- verbose。显示信息控制
- random_state。随机种子
- p_subtree_mutation。子树变异系数
- p_point_mutation。点变异系数
参考:
gplearn官网
一个github代码例子