皮尔逊相关性
Today we would be using a statistical concept i.e. Pearson's correlation to help us understand the relationships between the feature values (independent values) and the target value (dependent value or the value to be predicted ) which will further help us in improving our model’s efficiency.
今天,我们将使用统计概念(即Pearson的相关性)来帮助我们理解特征值(独立值)与目标值(独立值或要预测的值)之间的关系,这将进一步帮助我们提高模型的效率。
Mathematically pearson's correlation is calculated as:
在数学上, 皮尔逊的相关性计算如下:
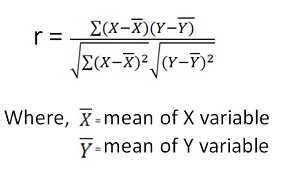
Image source: https://businessjargons.com/wp-content/uploads/2016/04/Karl-Pearson-final.jpg
图片来源: https : //businessjargons.com/wp-content/uploads/2016/04/Karl-Pearson-final.jpg
So now the question arises, what should be stored in the variable X and what should be stored in variable Y. We generally store the feature values in X and target value in the Y. The formula written above will tell us whether there exists any correlation between the selected feature value and the target value.
所以现在出现了一个问题,什么应该存储在变量X中,什么应该存储在变量Y中。我们通常将特征值存储在X中,将目标值存储在Y中。上面写的公式将告诉我们是否存在任何相关性在所选特征值和目标值之间。
Before we code there are few basic things that we should keep in mind about correlation:
在进行编码之前,关于关联我们应该牢记一些基本的知识:
The value of Correlation will always lie between 1 and -1
关联的值将始终在1到-1之间
Correlation=0, it means there is absolutely no relationship between the selected feature value and the target value.
Correlation = 0 ,这意味着所选特征值和目标值之间绝对没有关系。
Correlation=1, it means that there is a perfect relationship between the selected feature value and the target value and this would mean that the selected feature is appropriate for our model to learn.
Correlation = 1 ,表示所选特征值与目标值之间存在完美的关系,这意味着所选特征适合我们的模型学习。
Correlation=-1, it means that there exists a negative relationship between the selected feature value and the target value, generally, the use of the feature value having a negative value of low magnitude is discouraged for e.g. -0.1 0r -0.2.
Correlation = -1 ,意味着在所选择的特征值与目标值之间存在负的关系,通常,对于例如-0.1 0r -0.2,不鼓励使用具有低幅度的负值的特征值。
So, guys let us now write the code to implement that we have just learned:
所以,伙计们让我们现在编写代码以实现刚刚学习的代码:
The data set used can be downloaded from here: headbrain3.CSV
可以从此处下载使用的数据集: headbrain3.CSV
"""
# -*- coding: utf-8 -*-
"""
Created on Sun Jul 29 22:21:12 2018
@author: Raunak Goswami
"""
import numpy as np
import pandas as pd
import matplotlib.pyplot as plt
"""
#reading the data
"""
here the directory of my code and the headbrain3.csv file
is same make sure both the files are stored in same folder
or directory
"""
data=pd.read_csv('headbrain3.csv')
#this will show the first five records of the whole data
data.head()
w=data.iloc[:,0:1].values
y=data.iloc[:,1:2].values
#this will create a variable x which has the feature values i.e head size
x=data.iloc[:,2:3].values
#this will create a variable y which has the target value i.e brain weight
z=data.iloc[:,3:4].values
print(round(data['Gender'].corr(data['Brain Weight(grams)'])))
plt.scatter(w,z,c='red')
plt.title('scattered graph for coorelation between Gender and brainweight' )
plt.xlabel('age')
plt.ylabel('brain weight')
plt.show()
print(round(data['Age Range'].corr(data['Brain Weight(grams)'])))
plt.scatter(x,z,c='red')
plt.title('scattered graph for coorelation between age and brainweight' )
plt.xlabel('age range')
plt.ylabel('brain weight')
plt.show()
print(round((data['Head Size(cm^3)'].corr(data['Brain Weight(grams)']))))
plt.scatter(x,z,c='red')
plt.title('scattered graph for coorelation between head size and brainweight' )
plt.xlabel('head size')
plt.ylabel('brain weight')
plt.show()
data.info()
data['Head Size(cm^3)'].corr(data['Brain Weight(grams)'])
k=data.corr()
print("The table for all possible values of pearson's coefficients is as follows")
print(k)
After you run your code in Spyder tool provided by anaconda distribution just go to your variable explorer and search for the variable named as k and double-click to see the values in that variable and you’ll see something like this
在anaconda发行版提供的Spyder工具中运行代码之后,转到变量资源管理器并搜索名为k的变量,然后双击以查看该变量中的值,您将看到类似以下的内容
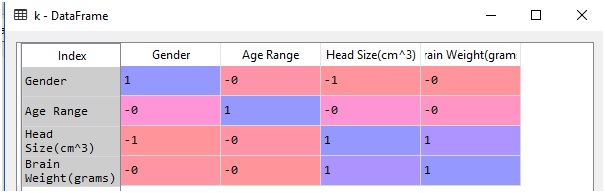
The table above shows the correlation values here 1 means perfect correlation,0 is for no correlation and -1 stands for negative correlation.
上表显示了相关值,此处1表示完全相关,0表示无相关,-1表示负相关。
Now let us understand these values using the graphs:
现在,让我们使用图形来了解这些值:
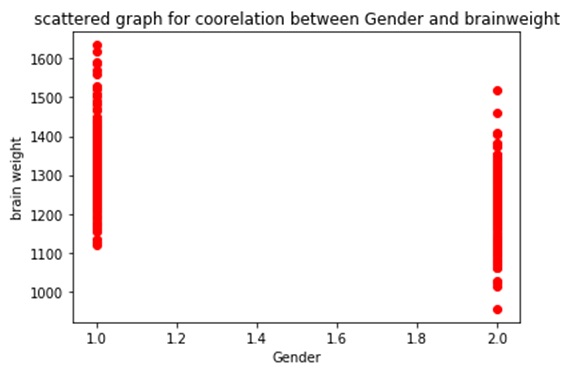
The reason for getting this abruptly looking graph is that there is no correlation between gender and brain weight, that is why we cannot use gender as a feature value in our prediction model.Let us try drawing graph for brain weight using another feature value, what about head size?
得到这张看起来很突然的图的原因是性别和大脑重量之间没有相关性,这就是为什么我们不能在预测模型中使用性别作为特征值的原因。让我们尝试使用另一个特征值绘制大脑重量的图关于头的大小?
As you can see in the table, there exists a perfect correlation between between brain weight and head size so as a result we a getting a definite graph this signifies that there exists a perfect linear relationship between brain weight and head size so we can use head size as one of the feature value in our model.
如您在表格中所见,大脑重量和头部大小之间存在完美的关联,因此,我们得到一个确定的图,这表明大脑重量和头部大小之间存在完美的线性关系,因此我们可以使用头部大小作为模型中的特征值之一。
That is all for this article if you have any queries just write in the comment section I would be happy to help you. Have a great day ahead, keep learning.
如果您有任何疑问,只需要在评论部分中编写,这就是本文的全部内容,我们很乐意为您提供帮助。 祝您有美好的一天,继续学习。
翻译自: https://www.includehelp.com/ml-ai/pearsons-correlation-and-its-implication-in-machine-learning.aspx
皮尔逊相关性