简单线性回归 (Simple Linear Regression)
A simple regression model could be a linear approximation of a causative relationship between two or additional variables. Regressions models are extremely valuable, as they're one in every of the foremost common ways that to create inferences and predictions.
一个简单的回归模型可以是两个或其他变量之间的因果关系的线性近似。 回归模型非常有价值,因为它们是创建推论和预测的所有最常见方式之一。
The process goes like this. You get sample data, come back up with a model that explains the data and so create predictions for the total population supported the model you've developed.
这个过程是这样的。 您将获得样本数据,然后使用一个解释数据的模型,从而为支持您开发的模型的总人口创建预测。
There is a variable, labeled Y, being foreseen, and freelance variables, tagged x1, x2, so forth. These are the predictors. Y could be a perform of the X variables, and also the regression model could be a linear approximation of this perform.
可以预见有一个标记为Y的变量,还有一个标记为x1 , x2的自由变量。 这些是预测因素。 Y可以是X变量的执行,并且回归模型可以是该变量的线性近似。
The easiest regression model is that the straightforward linear regression: Y is up to beta zero and beta one-time x plus epsilon.
最简单的回归模型是简单的线性回归: Y最高为beta 0和beta的x乘以 epsilon。
Let's see what these values mean. Y is that the variable we tend to are attempting to predict and is termed the variable. X is a variable quantity. Once exploitation multivariate analysis, we wish to predict the worth of Y, provided we have the worth of X.
让我们看看这些值的含义。 Y是我们倾向于尝试预测的变量,称为变量。 X是一个变量。 一旦进行了多变量分析,我们希望预测Y的价值,前提是我们拥有X的价值。
But to possess a regression, Y should depend upon X in some causative manner. Whenever there's a modification in X, such modification should translate into a change in Y.
但是要拥有回归, Y应该以某种原因依赖于X。 每当X中有修改时,此类修改应转换为Y中的更改。
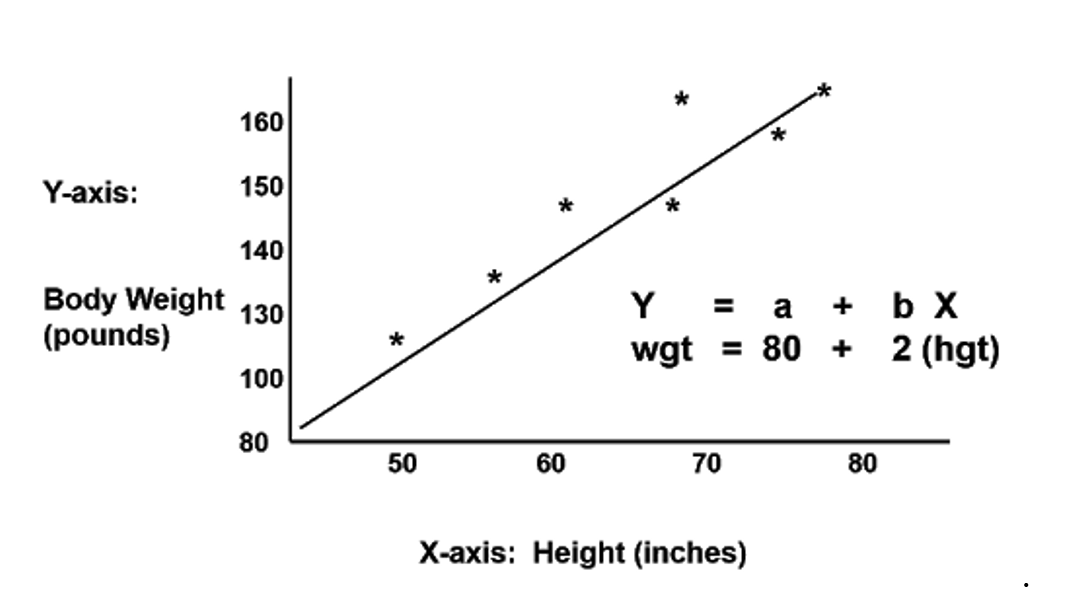
Think about the subsequent equation: the financial gain an individual receives depends on the number of years of education that a person has received. The variable is financial gain, whereas the variable quantity is years of education. There's a causative relationship between the 2. The additional education you get, the upper the financial gain you're possible to receive. This relationship is therefore trivial that it's in all probability the explanation you're observing this course, right now. You would like to urge better financial gain, therefore you're increasing your education.
考虑下面的等式 :一个人获得的经济收益取决于一个人受教育的年限。 变量是财务收益,变量是教育年限。 两人之间存在因果关系。您获得的额外教育越多,您可以获得的经济收益就越高。 因此,这种关系是微不足道的,因为它很可能是您目前正在观察的此过程的解释。 您想敦促获得更好的经济收益,因此您正在增加学历。
Now, let's pause for a second and have faith in the reverse relationship. What if education depends on financial gain. This might mean the upper your financial gain, the additional years you pay educating yourself. Golf shot high tuition fees aside, wealthier people don't pay additional years in class. And, highschool and faculty take a similar range of years, regardless of your income bracket. Therefore, a causative relationship like this one is faulty, if not plain wrong. Hence, it's unfit for multivariate analysis.
现在,让我们暂停片刻,并对反向关系充满信心。 如果教育取决于经济收益该怎么办。 这可能意味着您的经济收益越高,您自学的额外年限就越多。 高尔夫除了高额的学费外,较富裕的人无需再上课。 而且,无论您的收入水平如何,高中和教师的年限都差不多。 因此,即使不是完全错误的,这种因果关系也是错误的。 因此,它不适合进行多元分析。
Let's return to the initial example. Financial gain could be a performance of education. The additional years you study, the upper financial gain you'll receive. This sounds regarding right.
让我们回到最初的示例。 经济收益可能是教育的表现。 学习了额外的几年,您将获得最高的经济收益。 这听起来是对的。
好的 (Alright)
What we haven't mentioned, so far, is that, in our model, there are coefficients. Beta one is the constant that stands before the variable quantity. It quantifies the result of education on financial gain. If beta one is fifty, then for every further year of education, your financial gain would grow by $50. In the USA, the amount is way larger, somewhere around three to 5,000 bucks. So, for every further year you pay on education, your yearly financial gain is predicted to rise by 3 to 5 thousand bucks. And that's not considering pedagogy or tailored courses, like this one.
到目前为止,我们还没有提到的是,在我们的模型中,存在系数。 Beta 1是位于变量前的常数。 它量化了关于经济收益的教育结果。 如果beta 1是50,则每一年的教育,您的经济收益将增加50美元。 在美国,这笔钱要大得多,大约三到五千美元。 因此,您为教育付出的每一年,预计您的年度财务收益将增加3到5,000美元。 而且,这并不是在考虑像这样的教学法或量身定制的课程。
The different 2 other parts are the constant beta zero and also the error – epsilon.
In this example, you'll be able to consider the constant beta zero because of the pay. Regardless of your education, if you have got employment, you'll get the pay. This is often a secured quantity.
其他2个部分分别是常数beta 0和误差– epsilon 。
在此示例中,由于报酬,您将能够考虑常数beta 0。 无论您受过什么教育,如果您有工作,就可以获得报酬。 这通常是安全数量。
So, if you have never visited the college and plug an education worth of zero years within the formula, the regression can predict that your financial gain is going to be the pay smart, right?
因此,如果您从未上过大学,并在公式中加入了零年制的教育费用,则回归分析可以预测您的财务收益将是明智的报酬,对吗?
The last term is epsilon. This represents the error of estimation. The error is that the actual distinction between the determined financial gain and also the income the regression foreseen. On average, across all observations, the error is zero. If you earn over what the regression has foreseen, then somebody earns but what the regression has foreseen. Everything evens out.
最后一个词是epsilon。 这代表估计误差。 错误是确定的财务收益与预计的回归收益之间的实际区别。 平均而言,在所有观察中,误差为零。 如果您获得的收益超过了回归的预期,那么有人会赚钱,但回归的收益却是预期的。 一切都变得平稳。
式 (Formula)
The original formula was written with Greek letters. What will this tell us? it was the population formula. However, we all know statistics are all regarding sample information. In follow, we tend to use the statistical regression equation.
原始公式用希腊字母书写。 这将告诉我们什么? 这是人口公式。 但是,我们都知道统计数据都是关于样本信息的。 接下来,我们倾向于使用统计回归方程。
It is merely y hat equals b zero plus b one time x.
仅仅是y等于b零加b 乘以x 。
You detected right. The y here is noted as y hat. Whenever we have a hat image, it's calculable or a foreseen worth.
您检测正确。 此处的y表示为y帽子。 每当我们有帽子图像时,它都是可以计算或可以预见的。
b zero is that the estimate of the regression constant beta zero, whereas b one is that the estimate of beta one, and x is that the sample information for the variable quantity.
b零是回归常数β0的估计,而b 1是β1的估计, x是变量的样本信息。
翻译自: https://www.includehelp.com/data-science/simple-linear-regression.aspx