上一篇文章我记录了自己在入门 Python 学习的一些基础内容以及实际操作代码时所碰到的一些问题。
这篇我将会记录我在学习和运用 Python 进行数据分析的过程:
- 介绍 Numpy 和 Pandas 两个包
- 运用 Numpy 和 Pandas 分析一维、二维数据
- 数据分析的基本过程
- 实战项目【用 Python 分析朝阳医院2018季度的药物销售数据】
一、简单介绍 Numpy 和 Pandas 两个包
NumPy 和 pandas 是 Python 常见的两个科学运算的包,提供了比 Python 列表更高级的数组对象且运算效率更高。常用于处理大量数据并从中提取、分析有用指标。
NumPy 是 Numerical Python 的简称, 它是目前 Python 数值计算中最为重要的基础包。大多数计算包都提供了基于 NumPy 的科学函数功能,将 NumPy 的数组对象作为数据交换的通用语。NumPy 的核心是 ndarray 对象,它封装了 Python 的原生数据类型的N维数组。NumPy 创建的数组在创建时就要有固定大小,数组元素需要有相同的数据类型,NumPy 也可以像Python 数组一样使用切片。矢量化和广播是 Numpy 的特性。
pandas 所包含的数据结构和数据梳理工具的设计使得在 Python 中 进行数据清晰和分析非常快捷。pandas 经常是和其它数值计算工具,比如 NumPy 和 SciPy,以及数据可视化工具比如 matplotlib 一起使用的。 pandas 支持大部分 NumPy 语言风格的数组计算。pandas 可以直观的描述一维和二维数据结构,分别是 Series 对象和 DataFrame 对象,理解起来很直观清晰。pandas 可以处理多种不同的数据类型,可以处理缺失数据,可以分组和聚合,也支持切片功能。
二、运用 NumPy 和 pandas 分析一维、二维数据
首先在 conda 中安装这两个包,安装命令:
conda install numpy, pandas
'''
Install two packages in conda, installation command:
conda install numpy, pandas
'''
# import numpy package
import numpy as np
# import pandas package
import pandas as pd
- 运用 NumPy 分析一维数据
1.1 定义一维数组:
定义一维数组 array,参数传入的是一个列表 [2,3,4,5]
'''
Definition:
One dimension array, parameters passed was a list[2,3,4,5]
'''
a = np.array([2,3,4,5])
1.2 查询:
# check items
a[0]
2
1.3 切片访问 - 获取指定序号范围的元素
# section acess: Acquired items from designated range series number
# a[1:3] Acquired items from series no. 1 to series no.3
a[1:3]
array([3, 4])
1.4 查询数据类型:
'''
dtype detail info link reference:
https://docs.scipy.org/doc/numpy-1.10.1/reference/arrays.dtypes.html
'''
# Check data types
a.dtype
dtype('int32')
1.5 统计计算 - 平均值
# Statistical caculation
# mean
a.mean()
3.5
1.6 统计计算 - 标准差
# standard deviation
a.std()
1.118033988749895
1.7 向量化运行 - 乘以标量
# vectorization: multiply scalar
b = np.array([1,2,3])
c = b * 4
c
array([ 4, 8, 12])
2. 运用 NumPy 分析二维数据
2.1 定义二维数组:
'''
Numpy Two-dimensional data structure:
Array
'''
# Define Two-dimensional data array
a = np.array([[1,2,3,4],[5,6,7,8],[9,10,11,12]
])
2.2 获取元素:
获取行号是0,列号是2的元素
# Acquire the items that row number is 0, and Column number is 2
a[0,2]
3
2.3 获取行:
获取第1行
# Acquire first row items
a[0,:]
array([1, 2, 3, 4])
2.4 获取列:
获取第1列
# Acquire first column items
a[:,0]
array([1, 5, 9])
2.5 NumPy数轴参数:axis
1) 如果没有指定数轴参数,会计算整个数组的平均值
'''
If the axis parameters is not designated,
the mean of the entire array will be calculated
'''
a.mean()
6.5
2) 按轴计算:axis=1 计算每一行
# caculate according to axis: axis = 1 , caculate evey single row
a.mean(axis = 1)
array([ 2.5, 6.5, 10.5])
3) 按轴计算:axis=0 计算每一列
a.mean(axis = 0)
array([5., 6., 7., 8.])
3. 运用 pandas 分析一维数据
3.1 定义 Pandas 一维数据结构:
定义 Pandas 一维数据结构 - Series
'''
Definition:
Pandas One Dimension Data Analysis: Series
''''''
One day stock price saved for 6 companies(USD),
Tenent 427 HKD equal to 54.74 USD.
'''
stockS = pd.Series([54.74, 190.9, 173.14, 1050.3, 181.86, 1139.49],index = ['tencent','alibaba','apple','google','facebook','amazon'])
3.2 查询
查询 stockS
stockS
tencent 54.74
alibaba 190.90
apple 173.14
google 1050.30
facebook 181.86
amazon 1139.49
dtype: float64
3.3 获取描述统计信息:
# Acquired describe statistical info
stockS.describe()
count 6.000000
mean 465.071667
std 491.183757
min 54.740000
25% 175.320000
50% 186.380000
75% 835.450000
max 1139.490000
dtype: float64
3.4 iloc属性用于根据索引获取值
stockS.iloc[0]
54.74
3.5 loc属性用于根据索引获取值
# loc attribution: used to acquire value according to the index
stockS.loc['tencent']
54.74
3.6 向量化运算 - 向量相加
# vectorization: vectors addition
s1 = pd.Series([1,2,3,4], index = ['a','b','c','d'])
s2 = pd.Series([10,20,30,40], index = ['a','b','e','f'])
s3 = s1 + s2
s3
a 11.0
b 22.0
c NaN
d NaN
e NaN
f NaN
dtype: float64
3.7 删除缺失值
# Method 1: Delete missing value
s3.dropna()
a 11.0
b 22.0
dtype: float64
3.8 填充缺失值
# Filled up the missing values
s3 = s2.add(s1, fill_value = 0)
s3
a 11.0
b 22.0
c 3.0
d 4.0
e 30.0
f 40.0
dtype: float64
4. 运用 pandas 分析二维数据
pandas 二维数组:数据框(DataFrame)
4.1 定义数据框
'''
Pandas Two-dimensional array: DataFrame
'''
# Step1: Define a dict, Mapping names and corresponding values
salesDict = {'medecine purchased date':['01-01-2018 FRI','02-01-2018 SAT','06-01-2018 WED'],'social security card number':['001616528','001616528','0012602828'],'commodity code':[236701,236701,236701],'commodity name':['strong yinqiao VC tablets', 'hot detoxify clearing oral liquid','GanKang compound paracetamol and amantadine hydrochloride tablets'],'quantity sold':[6,1,2],'amount receivable':[82.8,28,16.8],'amount received':[69,24.64,15]
}# import OrdererDict
from collections import OrderedDict# Define an OrderedDict
salesOrderDict = OrderedDict(salesDict)# Define DataFrame: passing Dict, list name
salesDf = pd.DataFrame(salesOrderDict)
4.2 查看
salesDf

4.3 平均值
是按每列来求平均值
# mean: caculating according to columns
salesDf.mean()
commodity code 236701.000000
quantity sold 3.000000
amount receivable 42.533333
amount received 36.213333
dtype: float64
4.4 查询数据 - iloc属性用于根据位置获取值
1) 查询第1行第2列的元素
'''
iloc attributes used to acquired value according to position
'''
# check items at 1st row and 2nd column
salesDf.iloc[0,1]
'001616528'
2) 获取第1行 - 代表所有列
# Acquired all items of first row - collect every single colum
salesDf.iloc[0,:]
medecine purchased date 01-01-2018 FRI
social security card number 001616528
commodity code 236701
commodity name strong yinqiao VC tablets
quantity sold 6
amount receivable 82.8
amount received 69
Name: 0, dtype: object
3) 获取第1列 - 代表所有行
# Acquired all items of first column - collect every single row
salesDf.iloc[:,0]
0 01-01-2018 FRI
1 02-01-2018 SAT
2 06-01-2018 WED
Name: medecine purchased date, dtype: object
4.5 查询数据 - loc属性用于根据索引获取值
1) 获取第1行
'''
loc attributes used to acquired value according to index
'''
# Check items from first row first column
salesDf.loc[0,'medecine purchased date']
'01-01-2018 FRI'
2) 获取“商品编码”这一列
# Acquired all items of column 'commodity code'
# Method 1:
salesDf.loc[:,'commodity code']
0 236701
1 236701
2 236701
Name: commodity code, dtype: int64
3) 简单方法:获取“商品编码”这一列
# Acquired all items of column 'commodity code'
# Method 2: Easy way
salesDf['commodity code']
0 236701
1 236701
2 236701
Name: commodity code, dtype: int64
4.6 数据框复杂查询 - 切片功能
1) 通过列表来选择某几列的数据
# Select a few column data via list
salesDf[['commodity name','quantity sold']]
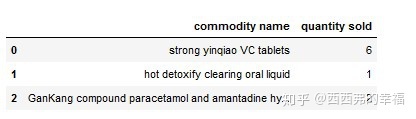
2)通过切片功能,获取指定范围的列
# Acquired data from define range of column via section
salesDf.loc[:,'medecine purchased date':'quantity sold']

4.7 数据框复杂查询 - 条件判断
1) 通过条件判断筛选 - 第1步:构建查询条件
# Select via condition test
# Step 1: Establish query condition
querySer = salesDf.loc[:,'quantity sold'] > 1
type(querySer)
pandas.core.series.Series
querySer
0 True
1 False
2 True
Name: quantity sold, dtype: bool
salesDf.loc[querySer,:]

4.8 查看数据集描述统计信息
1 ) 读取 Ecxcel 数据
# Read data from Excel
fileNameStr = 'C:UsersUSERDesktop#3Python3_The basic process of data analysisSales data of Chaoyang Hospital in 2018 - Copy.xlsx'
xls = pd.ExcelFile(fileNameStr)
salesDf = xls.parse('Sheet1')
2) 打印出前3行,以确保数据运行正常
# Print first three row to make sure data can work properly
salesDf.head(3)

3) 查询行、列总数
salesDf.shape
(6578, 7)
4)查看某一列的数据类型
# Check the data type of one column
salesDf.loc[:,'quantity sold'].dtype
dtype('float64')
5)查看每一列的统计数值
# Check the statistics for each column
salesDf.describe()
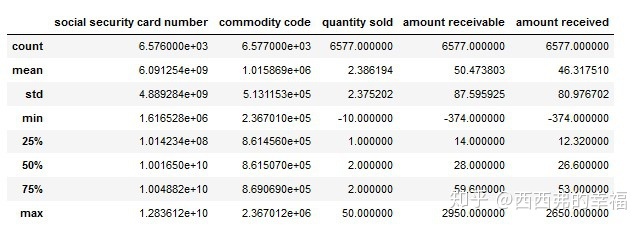
下一篇我将继续后半部分的学习
- 数据分析的基本过程
- 实战项目【用 Python 分析朝阳医院2018季度的药物销售数据】