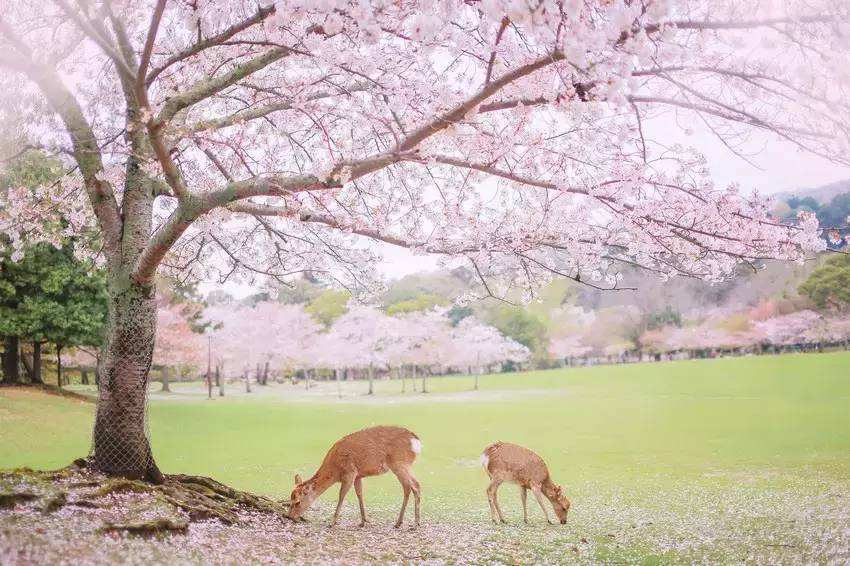
代码出处
吃水不忘打井人,分析github上的基于keras的实现:
xuannianz/keras-CenterNetgithub.com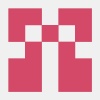
代码主体结构
模型训练的主函数流程如下所示,该流程也是使用keras的较为标准的流程。其中代码篇幅较大的是数据准备的部分,通常的代码也亦如此。下面按照不同的部分分别进行说明。
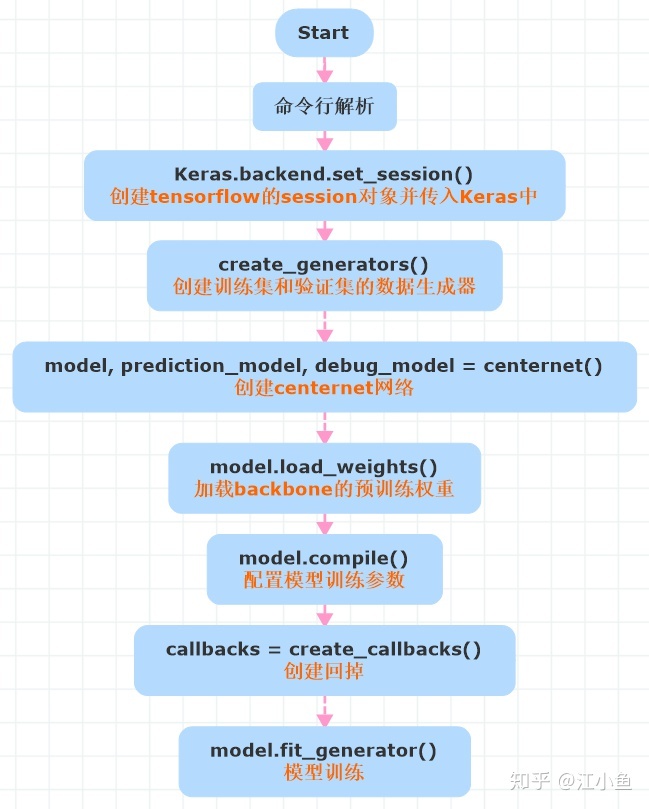
create_generators 数据集准备
该代码支持Pascal VOC格式、COCO格式以及CSV格式。keras中有三个函数可以用来进行模型的训练:分别是fit,fit_generator和train_on_batch。
fit(train_x, train_y, batchsize, epochs)
在使用fit进行模型训练时,通常假设整个训练集都可以放入RAM,并且没有数据增强(即不需要keras生成器)。常用于简单小型的数据集训练。
fit_generator;常常使用的模型训练函数
fit_generator适用于大数据集无法直接全部放入内存中,以及标注数据较少需要使用数据增强来增加训练模型的泛化能力。fit_generator需要传入一个数据生成器,数据生成器可以每次动态的生成一个batchsize的训练数据,通常我们也将数据增强放入数据生成器中,这样便可以动态的生成增强后的数据。在使用fit_generator时,需要传入steps_per_epoch的值,而fit函数则不需要,这是因为fit函数的steps_per_epoch默认等于总的训练数据/batchsize,而对于fit_generator来说,如果采用了数据增强,则可以产生无限的batchsize训练数据,因此需要指定该参数。
By the way,数据生成器可以使用keras的API或者直接自己手码python的代码,因为其本质上也就是python的函数。
train_on_batch(batchX, batchY)
train_on_batch用于需要对训练迭代进行精细控制,给其传入一批数据即可(数据大小任意),不需要提供batchsize的大小。通常很少使用该函数进行模型训练。
- 本算法的实现过程就是采用的fit_generator进行的模型训练。因此需要为其构建数据生成器。common.py文件:class Generator(keras.utils.Sequence)构建数据生成器的基类,咱们先说道说道keras.utils.Sequence这个类。
keras.utils.Sequence:这个基类通常应用于数据集生成一个数据序列。使用时需构建一个python类继承自该
基类,并必须实现__len__和__getitem__两个函数,如果要在每个epoch间修改数据集则需要实现on_epoch_end
方法。
NOTE:特别注意,__getitem__要返回一个完整的batchsize数据,__len__统计的也是有多少个batch
Generator类可以当成一个抽象基类,其中主要实现的是batch的划分、数据增强的处理、以及标注数据的转换(将bounding box的标注形式转换成高斯分布的标注)。而真正使用的数据集的生成器如下所示。主要按照不同的数据集生成的类,并均都继承于Generator抽象类,这里区分不同的数据集主要为了能方便区分其不同的数据标注格式,使用起来更为方便。主要是load_annotations()和load_image()函数的实现。至此数据生成器便构建完成了。
class PascalVocGenerator(Generator)
class CocoGenerator(Generator)
centernet网络构建
算法实现采用的Resnet50作为网络的backbone,采用下述引用网络。网络构建这里相对就比较简单了,取出Resnet的C5,先添加了一层dropout,然后进行了上采样,然后分别构建网络head,主要有三支:中心点预测、中心点偏移值预测以及bouding box的size预测。
from keras.applications.resnet50 import ResNet50
最后构建model,使用keras的Lambda层构建loss,作为model的output
loss_ = Lambda(loss, name='centernet_loss')([y1, y2, y3, hm_input, wh_input, reg_input, reg_mask_input, index_input])
model = Model(inputs=[image_input, hm_input, wh_input, reg_input, reg_mask_input, index_input], outputs=[loss_])
预训练模型权重加载
keras的模型加载可以使用load_weights来实现,其模型加载可以按照模型结构加载,此时by_name需设置为False。否则将按照网络层的名字来加载,此时通常将skip_mismatch也设置成True,即仅加载名字相同的层,其他名字不同的层直接跳过。因此可以利用这个特性,对已训练好的网络局部进行修改,然后再加载之前训练好的模型,方便进行模型的调优。
model.load_weights(args.snapshot, by_name=True, skip_mismatch=True)
模型配置
其中loss参数的传递有几种形式。
- 目标函数/损失函数的字符串,比如keras内置的一些损失函数
- 目标函数/损失函数,通常为自定义的损失函数
- 将目标函数/损失函数定义成model的一个层,类似本代码的实现。本代码实现时,因为直接把loss作为model的输出,因此输入y_true和y_pred,实际使用y_pred即输出loss,对其进行优化。
model.compile(optimizer=Adam(lr=1e-3), loss={'centernet_loss': lambda y_true, y_pred: y_pred})def compile(self, optimizer,loss=None,metrics=None,loss_weights=None,sample_weight_mode=None,weighted_metrics=None,target_tensors=None,**kwargs):"""Configures the model for training.# Argumentsoptimizer: String (name of optimizer) or optimizer instance.See [optimizers](/optimizers).loss: String (name of objective function) or objective function.See [losses](/losses).If the model has multiple outputs, you can use a different losson each output by passing a dictionary or a list of losses.The loss value that will be minimized by the modelwill then be the sum of all individual losses.metrics: List of metrics to be evaluated by the modelduring training and testing.Typically you will use `metrics=['accuracy']`.To specify different metrics for different outputs of amulti-output model, you could also pass a dictionary,such as `metrics={'output_a': 'accuracy'}`.loss_weights: Optional list or dictionary specifying scalarcoefficients (Python floats) to weight the loss contributionsof different model outputs.The loss value that will be minimized by the modelwill then be the *weighted sum* of all individual losses,weighted by the `loss_weights` coefficients.If a list, it is expected to have a 1:1 mappingto the model's outputs. If a dict, it is expected to mapoutput names (strings) to scalar coefficients.sample_weight_mode: If you need to do timestep-wisesample weighting (2D weights), set this to `"temporal"`.`None` defaults to sample-wise weights (1D).If the model has multiple outputs, you can use a different`sample_weight_mode` on each output by passing adictionary or a list of modes.weighted_metrics: List of metrics to be evaluated and weightedby sample_weight or class_weight during training and testing.target_tensors: By default, Keras will create placeholders for themodel's target, which will be fed with the target data duringtraining. If instead you would like to use your owntarget tensors (in turn, Keras will not expect externalNumpy data for these targets at training time), youcan specify them via the `target_tensors` argument. It can bea single tensor (for a single-output model), a list of tensors,or a dict mapping output names to target tensors.**kwargs: When using the Theano/CNTK backends, these argumentsare passed into `K.function`.When using the TensorFlow backend,these arguments are passed into `tf.Session.run`.