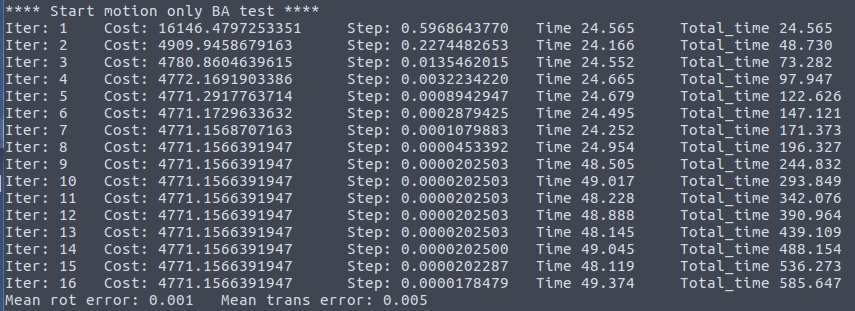
SLAM问题的后端有主要有滤波和优化两种方案。目前,普遍认为优化的方法在精度上要超过滤波方法,因为它可以进行多次的线性化。近年来出现的SLAM算法也大都是基于优化的算法(如ORB-SLAM、DSO等)。优化问题的核心便是Bundle Adjustment。本文对V-SLAM中纯视觉的Bundle Adjustment问题进行了介绍,给出了简单的实现,并利用仿真数据进行了测试。
1. 基本原理
关于Bundle Adjustment是什么,大家应该都比较熟悉,这里就不多做介绍了(可以参考文献[1][2])。本文主要给出一些实现细节。在基于特征点的视觉SLAM中,我们一般通过最小化重投影误差,来优化相机的位姿和地图点的位置。下面针对这个问题展开。
1.1 地图点的参数化方式
地图点的参数化方式主要有两种:一种是用三维向量
1.2 相机模型
相机模型也有很多啦,这里我们同样是使用最简单的一种:针孔相机模型。一个3D地图点
其中
1.3 误差、最小二乘问题
我们需要求解的最小二乘优化问题为
需要优化的量为相机的位姿
为了便于操作,这里我们将其转换为一个权重
那么,权重
至此,我们需要求解的最小二乘优化问题,变为
1.4 雅克比Jacobian
求解最小二乘问题,需要提供cost function:
1)cost function关于相机位姿
其中,
2)cost function关于地图点
1.5 增量方程的求解
无论使用Gauss-Netwon还是Levenburg-Marquadt方法求解最小二乘问题(7),都会求解一个关于增量的线性方程:
Bundle Adjustment中的
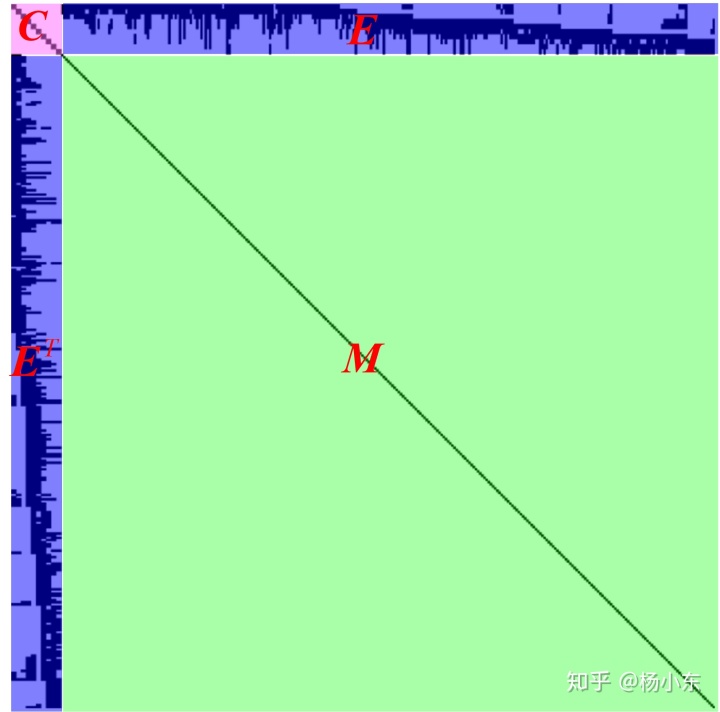
其中只与相机位姿相关的矩阵
利用舒尔补消元,我们首先求解
然后,再去求解
由于
1.6 固定部分状态
对于一个Bundle Adjustment问题,我们必须固定一部分状态,或者给一部分状态一个先验。不然,就会有无穷多解。可以想象一下,一个网络的误差已经达到了最小后,可以整体在空间内任意移动,而不会对误差造成任何影响。我们只要固定这个网络中的任意1个以上的节点,其他的节点都会有确定的状态。(我们通常会把第一个位姿固定)
怎么固定呢?一种可以加一个先验约束,就是加一个先验cost function。另外一种就是直接把状态fix住,不让它参与优化就好了。 我们采用后一种方法。
2. 实现
我们的实现比较简单:ydsf16/vslamgithub.com
1. 仅针对上述优化问题;
2. 不考虑扩展性;
3. 不考虑内存问题(不考虑稀疏矩阵存储,十分占内存,只能用来解小规模问题。TODO);
4. 不考虑稀疏矩阵的加速;
5. 只实现了LM优化算法;
6. 不支持先验约束,有motion only BA, struct only BA 和 Full BA三种形式。
7. 没有考虑鲁棒性的问题,错误的输入可能直接就崩掉了。
8. 速度慢(TODO)
实现的过程我们参考了g2o的做法。全部工程代码请见:
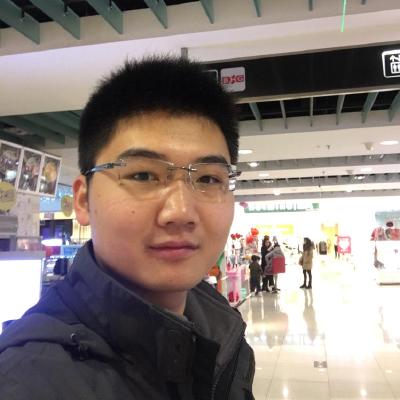
我们首先定义几个类,分别是地图点,相机位姿,CostFunction。地图点和相机位姿类用来存储需要优化的状态,通过id进行标识。CostFunction用来存储代价函数、观测,计算雅克比、残差等。
2.1 地图点类
存储待优化的地图点,同时定义了位置的加法。如果设置了fixed_标志,则不加入状态向量。
class MapPoint{
public:MapPoint(const Eigen::Vector3d& position, int id, bool fixed = false);const Eigen::Vector3d& getPosition();void setPosition(const Eigen::Vector3d& position);int getId();void setId(int id);void setFixed();bool isFixed();void addDeltaPosition(const Eigen::Vector3d& delta_position);int state_index_;
private:Eigen::Vector3d position_; int id_;bool fixed_;
}; //class MapPoint
2.2 相机类
存储待优化的相机,同时定义了位姿的加法,其实就是左乘啦。如果设置了fixed_标志,则不加入状态向量。
class Camera{
public:Camera(const Sophus::SE3& pose, int id, bool fixed = false);const Sophus::SE3& getPose();void setPose(const Sophus::SE3& pose);int getId();void setId(int id);void setFixed();bool isFixed();void addDeltaPose(const Eigen::Matrix<double, 6, 1>& delta_pose);void addDeltaPose(const Sophus::SE3& delta_pose);int state_index_;
private:Sophus::SE3 pose_;int id_;bool fixed_;
}; // class CameraPose
2.3 CostFunction类
用来存储cost function,计算cost, 计算雅克比,计算Huber weight等作用。总之,跟cost function相关的操作都放在了这里。
class CostFunction{
public:CostFunction(MapPoint* map_point, Camera* camera, double fx, double fy, double cx, double cy, const Eigen::Vector2d& ob_z);void setHuberParameter(double b = 1.0);void setCovariance(const Eigen::Matrix2d& cov);void computeInterVars(Eigen::Vector2d& e, Eigen::Matrix2d& weighted_info, double& weighted_e2);void computeJT(Eigen::Matrix<double, 2, 6>& JT);void computeJX(Eigen::Matrix<double, 2, 3>& JX);MapPoint* map_point_;Camera* camera_;
private:double fx_, fy_, cx_, cy_;Eigen::Vector2d ob_z_;Eigen::Matrix2d info_matrix_;double huber_b_;
}; // class CostFunction
2.4 BundleAdjustment类
整个BA问题的类。利用map结构存储地图点和相机,主要是为了方便快速查找。利用set结构存储cost function。在这个类里面,将所有cost function的雅克比、信息矩阵整合成大的雅克比、信息矩阵、Hassian矩阵等。在这里面,我们实现了LM优化算法。
class BundleAdjustment{
public:BundleAdjustment();~BundleAdjustment(); // free memory.void addMapPoint(MapPoint* mpt);void addCamera(Camera* cam);void addCostFunction(CostFunction* cost_func);MapPoint* getMapPoint(int id);Camera* getCamera(int id);void setConvergenceCondition(int max_iters, double min_delta, double min_error);void setVerbose(bool flag);void optimize();private:void optimizationInit(); // init for the optimization.void computeStateIndexes(); // compute index for every state.void computeHAndbAndError();void solveNormalEquation();void inverseM(const Eigen::MatrixXd& M, Eigen::MatrixXd& M_inv);void updateStates();void recoverStates();std::map<int, MapPoint*> mappoints_; // all mappointsstd::map<int, Camera*> cameras_; // all cameras.std::set<CostFunction*> cost_functions_; // all cost functions.Eigen::MatrixXd J_; // Jocabian matrix.Eigen::MatrixXd JTinfo_;Eigen::MatrixXd H_; // Hassian matrix.Eigen::MatrixXd r_; // residual vector.Eigen::MatrixXd b_;Eigen::MatrixXd info_matrix_; // information matrix.Eigen::MatrixXd Delta_X_; // Delta_X_Eigen::MatrixXd I_;int n_cam_state_; // number of cameras in the state vector.int n_mpt_state_; // number of map points in the state vector./* Convergence condition */int max_iters_;double min_delta_;double min_error_;double sum_error2_;double last_sum_error2_;bool verbose_;
}; // BundleAdjustment
2.5 BA问题的构建
这个过程跟g2o几乎一样。
step 1. 构造地图点、相机,加入到BundleAdjustment类。
step 2. 构造cost function,加入到BundleAdjustment类。
step 3. 开始迭代优化
step 4. 根据id将结果取出
BundleAdjustment ba_mba;ba_mba.setConvergenceCondition(20, 1e-5, 1e-10);ba_mba.setVerbose(true);// add mappointsfor(size_t i = 0; i < noise_mappoints.size(); i ++){const Eigen::Vector3d& npt = noise_mappoints.at(i);MapPoint* mpt = new MapPoint(npt, i);mpt->setFixed(); // fixed all mappoints.ba_mba.addMapPoint(mpt);} // add mappoints// add cameras.for(size_t i = 0; i < noise_cameras.size(); i ++){const Sophus::SE3& ncam = noise_cameras.at(i);Camera* cam = new Camera(ncam, i);ba_mba.addCamera(cam);} // add cameras.// add observationsfor(size_t i = 0; i < noise_observations.size(); i ++){const Observation& ob = noise_observations.at(i);MapPoint* mpt = ba_mba.getMapPoint(ob.mpt_id_);Camera* cam = ba_mba.getCamera(ob.cam_id_);CostFunction* cost_func = new CostFunction(mpt, cam, fx, fy, cx, cy, ob.ob_);ba_mba.addCostFunction(cost_func);} // add observations.// Optimizeba_mba.optimize();// Compute pose Errordouble sum_rot_error = 0.0;double sum_trans_error = 0.0;for(size_t i = 0; i < cameras.size(); i ++){Camera* cam = ba_mba.getCamera(i);const Sophus::SE3& opt_pose = cam->getPose();const Sophus::SE3& org_pose = cameras.at(i);Sophus::SE3 pose_err = opt_pose * org_pose.inverse();sum_rot_error += pose_err.so3().log().norm();sum_trans_error += pose_err.translation().norm();}std::cout << "Mean rot error: " << sum_rot_error / (double)(cameras.size())<< "tMean trans error: " << sum_trans_error / (double)(cameras.size()) << std::endl;
3. 实验
为了能够与真值对比,我采用了仿真实验。就是人为的仿真出m个相机,n个地图点和一些观测。然后给数据加一些噪声,测试算法的有效性。仿真实验有明确的ground truth,调试程序非常好用。下面给出一组数据的结果。
相机数量:6地图点数量:275观测数量:1650
3.1 motion only BA实验(固定地图点,只优化位姿)
首先,我们可以不添加任何噪声进行实验,可以发现优化后的结果与真值完全一样。
然后,添加如下所示的噪声,进行测试
mpt_noise = 0.01; // m
cam_trans_noise = 0.1; // m
cam_rot_noise = 0.1; // rad
ob_noise = 1.0; // pixel
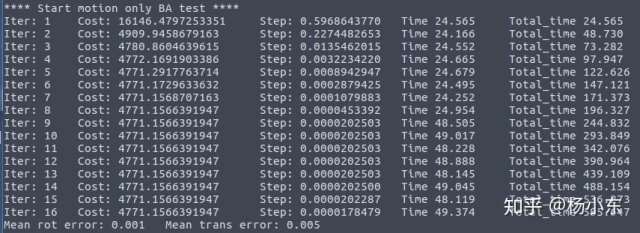
3.2 struct only BA实验(固定相机,只优化地图点)
添加如下所示的噪声,进行测试
mpt_noise = 0.1;
cam_trans_noise = 0.0;
cam_rot_noise = 0.00;
ob_noise = 1.0;
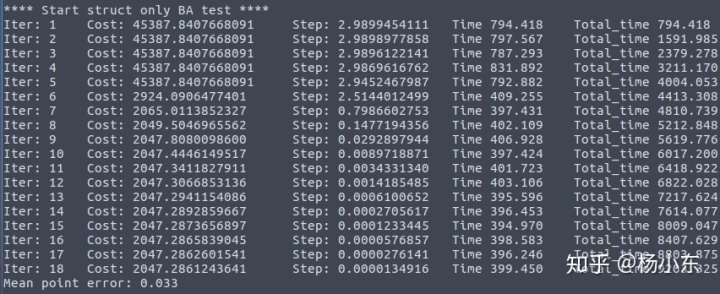
3.3 Full BA实验(只固定第一个相机,优化其余相机和地图点)
添加如下所示的噪声,进行测试
mpt_noise = 0.05;
cam_trans_noise = 0.1;
cam_rot_noise = 0.1;
ob_noise = 1.0;
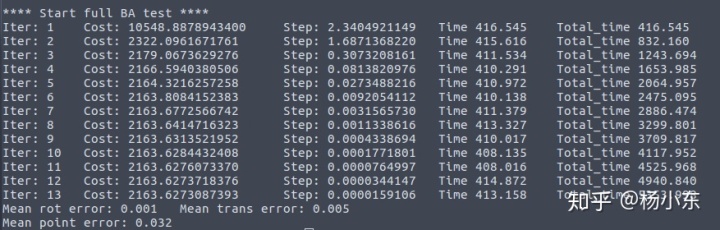
实测发现,算法可以很好的收敛。
相机位姿的估计精度会比地图点的精度更高,因为一个相机可以看到几百个地图点,而一个地图点只能被几个相机看到;相机的约束方程数量远大于地图点的约束方程数量。
还发现,计算速度很慢,主要的原因在于
1. 内存频繁拷贝
2. 增量方程求解速度很慢
3. 没有利用稀疏矩阵的性质
4. Eigen的特性不熟悉
......
总之,还有一大堆问题需要解决。这只是一个很基础的版本。以后有时间再搞吧。
参考资料
[1] Triggs B . Bundle Adjustment - A Modern Synthesis[M]// Vision Algorithms: Theory and Practice. Springer Berlin Heidelberg, 1999.
[2] 视觉SLAM十四讲
[3] Methods for Non-Linear Least Squares Problems