简述yolo1-yolo3
In the last part, we understood what YOLO is and how it works. In this section, let us understand how to apply it using pre-trained weights and obtaining the results. This article is greatly inspired by Andrew Ng’s Deep Learning Specialization course. I’ve also tried to gather information from various other articles/resources to make the concept easier to understand.
在最后一部分中,我们了解了YOLO是什么以及它如何工作。 在本节中,让我们了解如何使用预先训练的权重应用它并获得结果。 本文的灵感来自Andrew Ng的深度学习专业课程。 我还尝试从其他各种文章/资源中收集信息,以使该概念更易于理解。
Now it’s time to implement what we’ve understood using Python. You can do this with the help of a Jupyter Notebook (or any other IDE of your choice). The implementation of YOLO has been taken from Andrew Ng’s Github Repository. You’ll also have to download this zip file which contains the pre-trained weights and packages to implement YOLO. Here’s a link to my GitHub repository where you can find the Jupyter Notebook.
现在是时候使用Python来实现我们已经了解的内容了。 您可以在Jupyter Notebook(或您选择的任何其他IDE)的帮助下执行此操作。 YOLO的实现取自Andrew Ng的Github存储库 。 您还必须下载此zip文件 ,其中包含用于实施YOLO的预先训练的砝码和软件包。 这是我的GitHub存储库的链接 ,您可以在其中找到Jupyter Notebook。
I’ve tried to comment on as many lines of code as possible for better understanding.
我试图评论尽可能多的代码行,以便更好地理解。
导入库: (Importing Libraries:)
Let us first import all the required libraries.
让我们首先导入所有必需的库。
import os
import imageio
import matplotlib.pyplot as plt
from matplotlib.pyplot import imshow
import scipy.io
import scipy.misc
import numpy as np
import pandas as pd
import PIL
import tensorflow as tf
from skimage.transform import resize
from keras import backend as K
from keras.layers import Input, Lambda, Conv2D
from keras.models import load_model, Model
from yolo_utils import read_classes, read_anchors, generate_colors, preprocess_image,draw_boxes, scale_boxes
from yad2k.models.keras_yolo import yolo_head, yolo_boxes_to_corners, preprocess_true_boxes, yolo_loss, yolo_body
%matplotlib inline
应用过滤器: (Applying Filter:)
First, we are going to apply a filter by thresholding. We can do this by getting rid of those boxes which have a score less than the chosen threshold.
首先,我们将通过阈值应用过滤器。 我们可以通过摆脱得分小于所选阈值的框来做到这一点。
The model contains 80 different classes for detection. It gives a total of 19x19x5x85 numbers where:
该模型包含80种不同的检测类别。 它总共给出19x19x5x85个数字,其中:
19x19: the shape of the grid
19x19:网格的形状
5: number of anchor boxes
5:锚框数量
85: each box containing 85 numbers (Pc, bx, by, bh, bw, c1,c2…..c80)
85:每个盒子包含85个数字(Pc,bx,by,bh,bw,c1,c2…..c80)
def yolo_filter_boxes(box_confidence, boxes, box_class_probs, threshold = .6):'''
box confidence: tensor of shape (19,19,5,1) containing Pc
boxes: tensor of shape (19,19,5,4)
box_class_probs: tensor of shape (19,19,5,80)
threshold: if Pc<threshold, get rid of that box
'''
#Computing box scores
box_scores = box_confidence*box_class_probs #Finding the index of the class with maximum box score
box_classes = K.argmax(box_scores, -1) #Getting the corresponding box score
box_class_scores = K.max(box_scores,-1) #Creating a filtering mask. The mask will be true for all the boxes we intend to keep (pc >= threshold) and false for the rest
filtering_mask = box_class_scores>threshold #Applying the mask to scores, boxes and classes
scores = tf.boolean_mask(box_class_scores, filtering_mask)
boxes = tf.boolean_mask(boxes, filtering_mask)
classes = tf.boolean_mask(box_classes, filtering_mask)'''
scores: contains class probability score for the selected boxes
boxes: contains (bx,by,bh,bw) coordinates of selected boxes
classes: contains the index of class detected by the selected boxes
'''
return scores, boxes, classes
实施交叉口联盟(IoU): (Implementing Intersection Over Union (IoU):)
Now we are going to implement IoU. This will be used to evaluate the bounding boxes.
现在我们将实现IoU。 这将用于评估边界框。
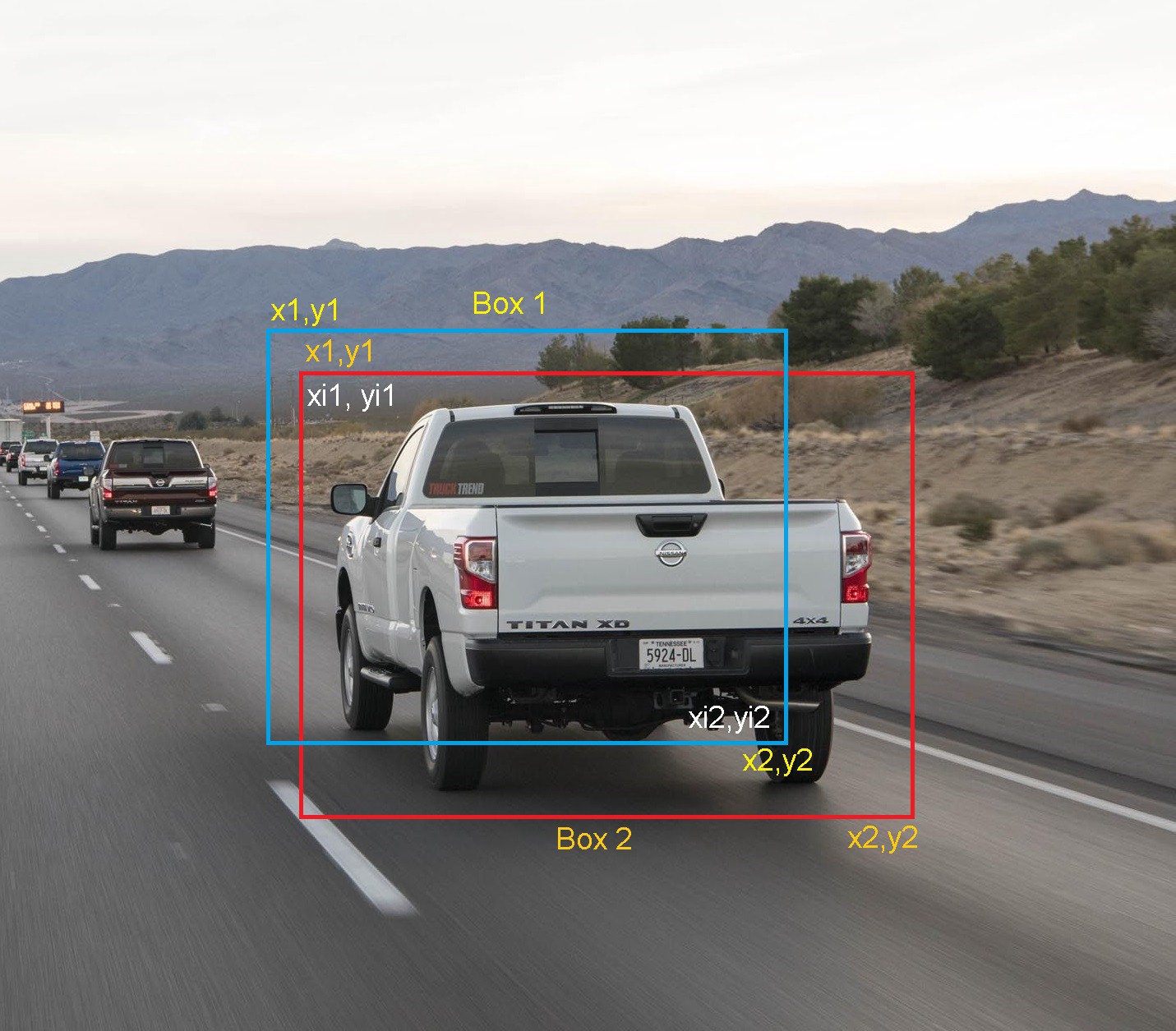
We will be defining a box using its two corners (upper left and lower right). The coordinates can be named as (x1,y1,x2,y2).
我们将使用两个角(左上和右下)定义一个框。 坐标可以命名为(x1,y1,x2,y2)。
We will also have to find out the coordinates of the intersection of two boxes.
我们还必须找出两个盒子相交的坐标。
xi1: maximum of the x1 coordinates of the two boxes.
xi1:两个框的x1坐标的最大值。
yi1: maximum of the y1 coordinates of the two boxes.
yi1:两个框的y1坐标的最大值。
xi2: minimum of the x2 coordinates of the two boxes.
xi2:两个框的x2坐标的最小值。
yi2: minimum of the y2 coordinates of the two boxes.
yi2:两个框的y2坐标的最小值。
The area of the rectangle formed after intersection can be calculated using the formula: (xi2 — xi1)*(yi2 — yi1)
交点后形成的矩形的面积可以使用以下公式计算:(xi2 — xi1)*(yi2 — yi1)
The formula for finding IoU is:
查找IoU的公式是:
(Intersection area)/(Union area)
Now let us define a function to calculate IoU.
现在让我们定义一个函数来计算IoU。
def iou(box1, box2): #Calculating (xi1,yi1,xi2,yi2) of the intersection of box1 and box2
xi1 = max(box1[0], box2[0])
yi1 = max(box1[1], box2[1])
xi2 = min(box1[2], box2[2])
yi2 = min(box1[3], box2[3])
#Calculating the area of intersection
inter_area = (yi2-yi1)*(xi2-xi1) #Calculating the areas of box1 and box2 using the same formula
box1_area = (box1[3] - box1[1])*(box1[2] - box1[0])
box2_area = (box2[3] - box2[1])*(box2[2] - box2[0])
#Calculating the union area by using the formula: union(A,B) = A+B-Inter(A,B)
union_area = box1_area + box2_area - inter_area #Calculating iou
iou = inter_area/union_area
return iou
实施非最大抑制: (Implementing Non-Max Suppression:)
Next, we will be implementing non-max suppression to remove all the duplicate bounding boxes for the same object. The steps involved are:
接下来,我们将实现非最大抑制,以移除同一对象的所有重复的边界框。 涉及的步骤是:
- Select the box with the highest score. 选择得分最高的框。
- Compute its IoU with all other boxes and remove those boxes which have IoU greater than the threshold mentioned. 用其他所有框计算其IoU,并删除IoU大于上述阈值的框。
- Repeat until there are no more boxes with a lower score than the selected box. 重复进行操作,直到没有比所选框得分更低的框。
Let us define the function
让我们定义功能
def yolo_non_max_suppression(scores, boxes, classes, max_boxes = 10, iou_threshold = 0.5): #tensor used in tf.image.non_max_suppression()of size 'max_boxes'
max_boxes_tensor = K.variable(max_boxes, dtype = 'int32') #initiating the tensor
K.get_session().run(tf.variables_initializer([max_boxes_tensor])) #Using the tensorflow function tf.image.non_max_suppression to get the indices of boxes kept
nms_indices = tf.image.non_max_suppression(boxes, scores, max_boxes, iou_threshold) #Using K.gather to individually access scores, boxes and classes from nms_indices
scores = K.gather(scores, nms_indices)
boxes = K.gather(boxes, nms_indices)
classes = K.gather(classes, nms_indices)
return scores, boxes, classes
上面定义的调用函数: (Calling Functions Defined Above:)
Now it’s time to implement a function that takes the output of deep CNN and then filters the boxes using the above functions.
现在是时候实现一个功能,该功能获取深层CNN的输出,然后使用上述功能过滤框。
Note that there are a few ways by which a bounding box can be represented i.e via their corners or their midpoints and height/width. YOLO converts between a few such formats for which there is a function named “yolo_boxes_to_corners”.
请注意,有几种表示边界框的方法,即通过其角或中点和高度/宽度。 YOLO在几种此类格式之间进行转换,为此存在一个名为“ yolo_boxes_to_corners”的函数。
Also, YOLO was trained on images of 608 x 608 dimensions. If the images we provide have a dimension greater than or less than the original dimension (on which YOLO was trained) then we will have to rescale the bounding boxes accordingly to fit on the image. We will be using a function called “scale_boxes” for this purpose.
此外,YOLO还接受了608 x 608尺寸图像的培训。 如果我们提供的图像的尺寸大于或小于原始尺寸(YOLO受其训练),则我们将不得不相应地重新调整边界框以适合图像。 为此,我们将使用一个名为“ scale_boxes”的函数。
def yolo_eval(yolo_outputs, image_shape = (720., 1280.), max_boxes = 10, score_threshold = .6, iou_threshold = .5): '''
yolo_outputs contains:
box_confidence, box_xy, box_wh, box_class_probs
''' #Retrieving output
box_confidence, box_xy, box_wh, box_class_probs = yolo_outputs #Converting the boxes for filtering functions
boxes = yolo_boxes_to_corners(box_xy, box_wh) #Using the function defined before to remove boxes with less confidence score
scores, boxes, classes = yolo_filter_boxes(box_confidence, boxes, box_class_probs, threshold = score_threshold) #Scaling the boxes
boxes = scale_boxes(boxes, image_shape) #Using the function defined before for non-max suppression
scores, boxes, classes = yolo_non_max_suppression(scores, boxes, classes, max_boxes, iou_threshold)
return scores, boxes, classes
加载预训练模型: (Loading Pre-Trained Model:)
Now we’re going to test the YOLO pre-trained models on images. For this, we have to create a session. Also, remember that we’re trying to detect 80 classes and using 5 anchor boxes. We have all the class information in “coco_classes.txt” and “yolo_anchors.txt” which must be present in the zip file you downloaded before inside the folder “model_data”.
现在,我们将在图像上测试YOLO预训练模型。 为此,我们必须创建一个会话。 另外,请记住,我们正在尝试检测80个类别并使用5个定位框。 我们在“ coco_classes.txt”和“ yolo_anchors.txt”中具有所有类信息,这些信息必须出现在您下载的zip文件中之前,该文件位于“ model_data”文件夹中。
The training of the YOLO model takes a long time especially if you don’t have a high spec system. So we are going to load an existing pre-trained Keras YOLO model stored in “yolo.h5”. These are the pre-trained weights from the YOLOv2 model.
YOLO模型的训练需要很长时间,特别是如果您没有高规格的系统。 因此,我们将加载存储在“ yolo.h5”中的现有预先训练的Keras YOLO模型。 这些是来自YOLOv2模型的预训练权重。
Let's create a session and load these files.
让我们创建一个会话并加载这些文件。
sess = K.get_session()
class_names = read_classes("model_data/coco_classes.txt")
anchors = read_anchors("model_data/yolo_anchors.txt")
yolo_model = load_model("model_data/yolo.h5")
Note: In some cases, a warning pops up while loading the weights. If that’s the case then just ignore the warning.
注意: 在某些情况下,加载砝码时会弹出警告。 如果是这种情况,请忽略警告。
#Converting the output of model into usable bounding box tensors
yolo_outputs = yolo_head(yolo_model.output, anchors, len(class_names))
#Filtering the boxes
scores, boxes, classes = yolo_eval(yolo_outputs, image_shape)
So far we have created a session graph that is given to yolo_model to compute output, processed by yolo_head, and goes through a filtering function yolo_eval.
到目前为止,我们已经创建了一个会话图,该会话图被赋予yolo_model以计算输出,并由yolo_head处理,并经过过滤函数yolo_eval。
在图像上应用YOLO: (Applying YOLO on an Image:)
Now we have to implement a function that runs the graph to test YOLO on an image.
现在,我们必须实现一个运行图形的功能,以在图像上测试YOLO。
def predict(sess, image_file): #Preprocessing the image
image, image_data = preprocess_image("images/"+image_file, model_image_size = (608,608)) #Running the session and feeding the input to it
out_scores, out_boxes, out_classes = sess.run([scores, boxes, classes],feed_dict = {yolo_model.input: image_data, K.learning_phase(): 0}) #Prints the predicted information
print('Found {} boxes for {}'.format(len(out_boxes), image_file)) #Generates color for drawing bounding boxes
colors = generate_colors(class_names) #Draws bounding boxes on the image file
draw_boxes(image, out_scores, out_boxes, out_classes, class_names, colors) #Saving the predicted bounding box on the image
image.save(os.path.join("out", image_file), quality = 150) #Displaying the results in notebook
output_image = imageio.imread(os.path.join("out", image_file))
plt.figure(figsize=(12,12))
imshow(output_image) return out_scores, out_boxes, out_classes
Run the following cell on your test image to see the results.
在测试图像上运行以下单元格以查看结果。
#Loading the image
img = plt.imread('images/traffic.jpeg')#Calculating the size of image and passing it as a parameter to yolo_eval
image_shape = float(img.shape[0]),float(img.shape[1])
scores, boxes, classes = yolo_eval(yolo_outputs, image_shape)#Predicts the output
out_scores, out_boxes, out_classes = predict(sess, "traffic.jpeg")
The output is:
输出为:
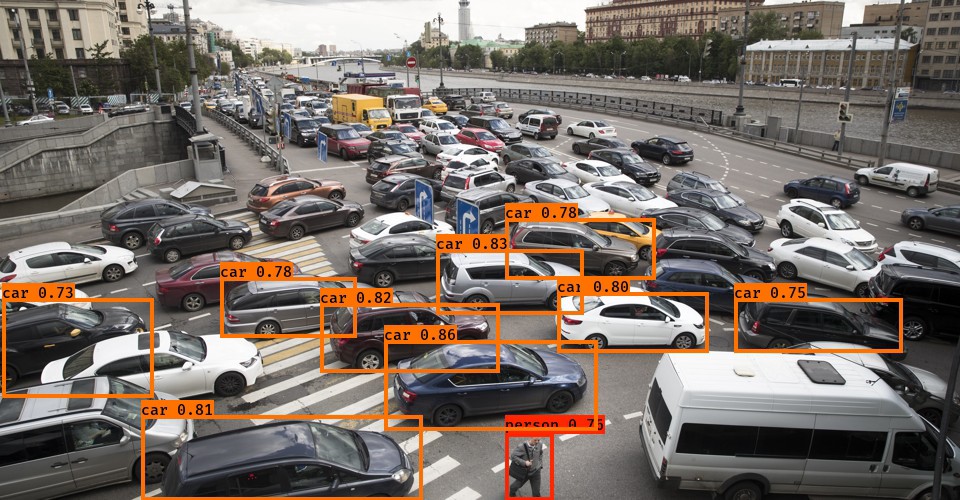
结论: (Conclusion:)
Thanks a lot if you’ve made this far. Please do note that the results may or may not be the same if you use the same image for detection. You can further customize the maximum number of bounding boxes per image, threshold values, etc. to obtain better results.
非常感谢您所做的一切。 请注意,如果使用相同的图像进行检测,结果可能会有所不同。 您可以进一步自定义每个图像的最大边界框数量,阈值等,以获得更好的结果。
If you have any suggestions to make this blog better, please do mention in the comments. I will try to make the changes.
如果您有任何建议可以改善此博客,请在评论中提及。 我将尝试进行更改。
翻译自: https://towardsdatascience.com/object-detection-part2-6a265827efe1
简述yolo1-yolo3
本文来自互联网用户投稿,该文观点仅代表作者本人,不代表本站立场。本站仅提供信息存储空间服务,不拥有所有权,不承担相关法律责任。如若转载,请注明出处:http://www.mzph.cn/news/392514.shtml
如若内容造成侵权/违法违规/事实不符,请联系多彩编程网进行投诉反馈email:809451989@qq.com,一经查实,立即删除!