使用管道符组合使用命令
Surely you have heard of pipelines or ETL (Extract Transform Load), or seen some method in a library, or even heard of any tool to create pipelines. However, you aren’t using it yet. So, let me introduce you to the fantastic world of pipelines.
当然,您听说过管道或ETL(提取转换负载),或者在库中看到了一些方法,甚至听说过任何用于创建管道的工具。 但是,您尚未使用它。 因此,让我向您介绍梦幻般的管道世界。
Before understanding how to use them, we have to understand what it is.
在了解如何使用它们之前,我们必须了解它的含义。
A pipeline is a way to wrap and automatize a process, which means that the process will always be executed in the same way, with the same functions and parameters and the outcome will always be in the predetermined standard.
管道是包装和自动化过程的一种方式,这意味着该过程将始终以相同的方式执行,并具有相同的功能和参数,并且结果将始终符合预定的标准。
So, as you may guess, the goal is to apply pipelines in every development stage to try to guarantee that the designed process never ends up different from the one idealized.
因此,您可能会猜到,目标是在每个开发阶段都应用管道,以确保设计过程永远不会与理想化过程不同。
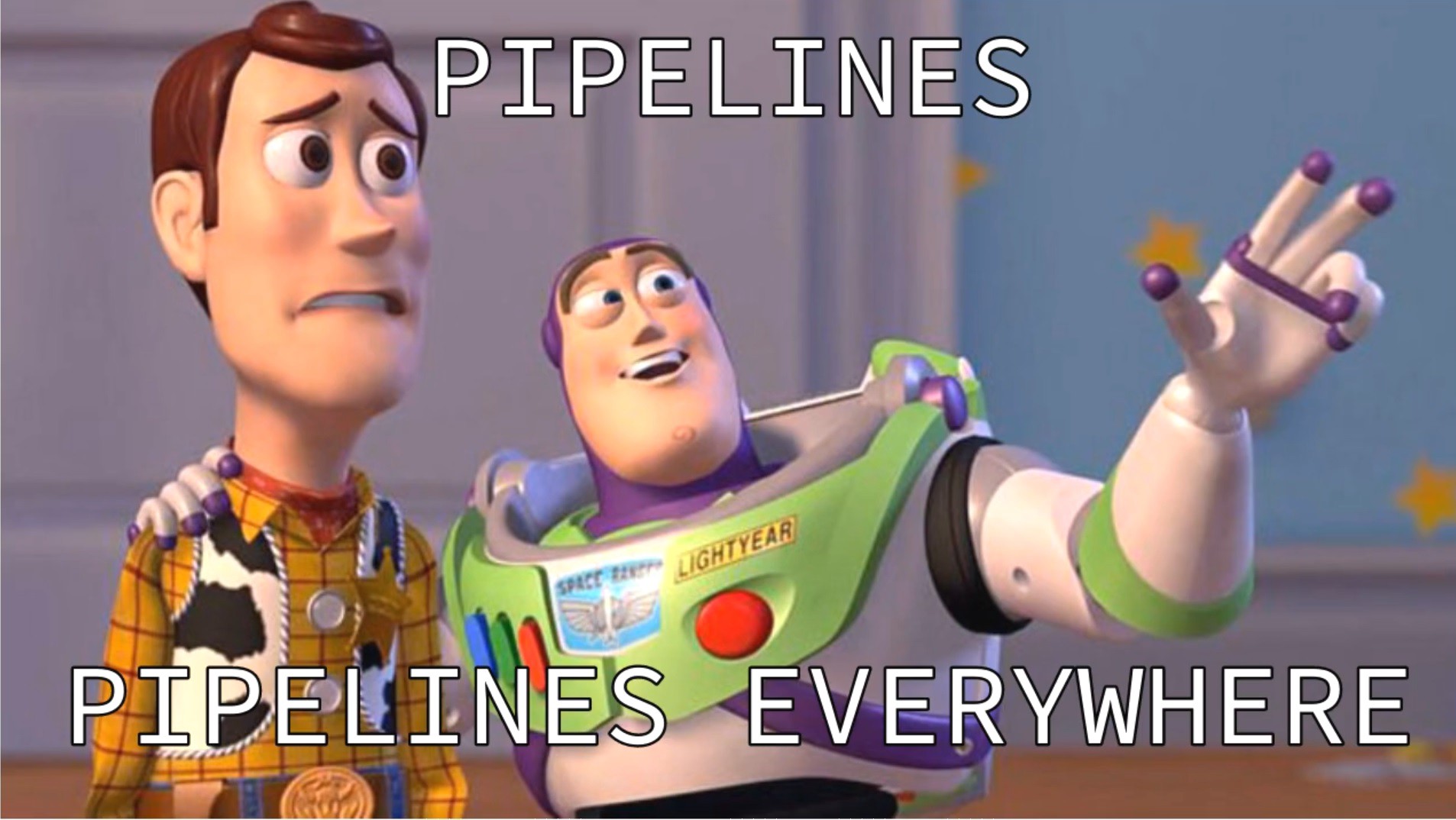
There are in particular two uses of pipelines in data science, either in production or during the modelling/exploration, that have a huge importance. Furthermore, it makes our life much easier.
在数据科学中,无论是在生产中还是在建模/探索期间,管道都有两种特别重要的用途。 此外,它使我们的生活更加轻松。
The first one is the data ETL. During production, the ramifications are way greater, and consequently, the level of detail spent in it, however, it can be summed up as:
第一个是数据ETL。 在生产过程中,后果会更加严重,因此花费在其中的详细程度也可以总结为:
E (Extract) — How am I going to collect the data? If I am going to collect them from one or several sites, one or more databases, or even a simple pandas csv. We can think of this stage as the data reading phase.
E(摘录)—我将如何收集数据? 如果我要从一个或多个站点,一个或多个数据库甚至一个简单的熊猫csv收集它们。 我们可以将此阶段视为数据读取阶段。
T (Transform) — What do I need to do for the data to become usable? This can be thought of as the conclusion of the exploratory data analysis, which means after we know what to do with the data (remove features, transform categorical variables into binary data, cleaning strings, etc.), we compile it all in a function that guarantees that cleaning will always be done in the same way.
T(转换)—要使数据变得可用我需要做什么? 这可以被认为是探索性数据分析的结论,这意味着在我们知道如何处理数据(删除功能,将分类变量转换为二进制数据,清理字符串等)之后,我们将其全部编译为一个函数这样可以确保始终以相同的方式进行清洁。
L (Load) — This is simply to save the data in the desired format (csv, data base, etc.) somewhere, either cloud or locally, to use anytime, anywhere.
L(负载)—这只是将数据以所需的格式(csv,数据库等)保存在云端或本地的任何地方,以便随时随地使用。
The simplicity of the creation of this process is such that it can be done only by grabbing that exploratory data analysis notebook, put that pandas read_csv inside a funcion; write the several functions to prepare the data and compile them in one; and finally create a function saving the result of the previous one.
创建此过程非常简单,只需抓住该探索性数据分析记录本,然后将熊猫read_csv放入函数中即可完成 。 编写几个函数来准备数据并将它们合而为一; 最后创建一个保存上一个结果的函数。
Having this, we can create the main function in a python file and with one line of code executes the created ETL, without risking any changes. Not to mention the advantages of changing/updating everything in a single place.
有了这一点,我们可以在python文件中创建main函数,并用一行代码执行创建的ETL,而无需冒险进行任何更改。 更不用说在单个位置更改/更新所有内容的优势。
And the second, and likely the most advantageous pipeline, helps solve one of the most common problems in machine learning: the parametrization.
第二,可能是最有利的管道,有助于解决机器学习中最常见的问题之一:参数化。
How many times have we faced these questions: which model to choose? Should I use normalization or standardization?
我们已经面对这些问题多少次了:选择哪种模型? 我应该使用标准化还是标准化?
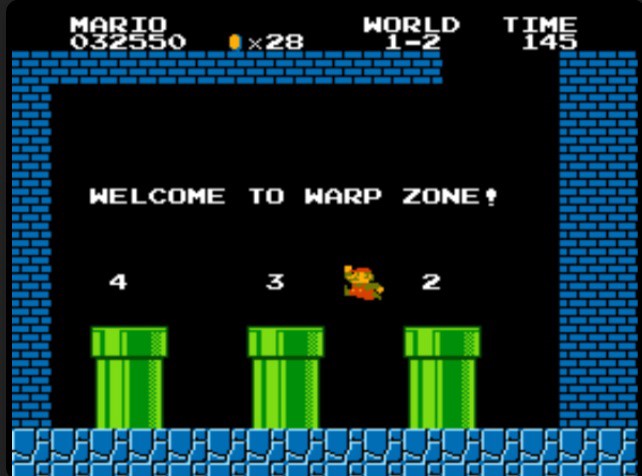
Libraries such as scikit-learn offer us the pipeline method where we can put several models, with their respective parameters’ variance, add pre-processing such as normalization, standardization or even a custom process, and even add cross-validation at the end. Afterwards, all possibilities will be tested, and the results returned, or even only the best result, like in the following code:
诸如scikit-learn之类的库为我们提供了流水线方法,我们可以放置几个具有各自参数差异的模型,添加诸如标准化,标准化甚至是自定义过程之类的预处理,甚至最后添加交叉验证。 之后,将测试所有可能性,并返回结果,甚至仅返回最佳结果,如以下代码所示:
def build_model(X,y):
pipeline = Pipeline([
('vect',CountVectorizer(tokenizer=tokenize)),
('tfidf', TfidfTransformer()),
('clf', MultiOutputClassifier(estimator=RandomForestClassifier())) ])# specify parameters for grid search parameters = {
# 'vect__ngram_range': ((1, 1), (1, 2)),
# 'vect__max_df': (0.5, 0.75, 1.0),
# 'vect__max_features': (None, 5000, 10000),
# 'tfidf__use_idf': (True, False),
# 'clf__estimator__n_estimators': [50,100,150,200],
# 'clf__estimator__max_depth': [20,50,100,200],
# 'clf__estimator__random_state': [42] }
# create grid search object
cv = GridSearchCV(pipeline, param_grid=parameters, verbose=1) return cv
At this stage, the sky is the limit! There are no parameters limits inside the pipeline. However, depending on the database and the chosen parameters it can take an eternity to finish. Even so, it is a very good tool to funnel the research.
在这个阶段,天空是极限! 管道内部没有参数限制。 但是,根据数据库和所选的参数,可能要花很长时间才能完成。 即使这样,它还是进行研究的很好工具。
We can add a function to read the data that comes out of the data ETL, and another to save the created model and we have model ETL, wrapping up this stage.
我们可以添加一个函数来读取来自数据ETL的数据,另一个函数可以保存创建的模型,并且我们拥有模型ETL,从而结束了这一阶段。
In spite of everything that we talked about, the greatest advantages of creating pipelines are the replicability and maintenance of your code that improve exponentially.
尽管我们讨论了所有问题,但是创建管道的最大优势是代码的可复制性和维护性得到了指数级的提高。
So, what are you waiting for to start creating pipelines?
那么,您还等什么来开始创建管道?
An example of these can be found in this project.
在此项目中可以找到这些示例。
翻译自: https://towardsdatascience.com/how-to-use-the-magic-of-pipelines-6e98d7e5c9b7
使用管道符组合使用命令
本文来自互联网用户投稿,该文观点仅代表作者本人,不代表本站立场。本站仅提供信息存储空间服务,不拥有所有权,不承担相关法律责任。如若转载,请注明出处:http://www.mzph.cn/news/392158.shtml
如若内容造成侵权/违法违规/事实不符,请联系多彩编程网进行投诉反馈email:809451989@qq.com,一经查实,立即删除!