数字经济的核心是对大数据
“Information is the oil of the 21st century, and analytics is the combustion engine”.
“信息是21世纪的石油,分析是内燃机”。
— Peter Sondergaard, Senior Vice President of Gartner Research.
— Gartner研究部高级副总裁Peter Sondergaard。
来自太空的大数据和“概述效应” (Big Data from Space and the “overview effect”)
“I used to tell people I was from Cleveland, Ohio, because that was where I was born. Today, I simply say I am from Earth”.
“我曾经告诉人们我来自俄亥俄州的克利夫兰,因为那是我的出生地。 今天,我只是说我来自地球”。
That’s the world view of former Nasa astronaut Don Thomas who has orbited Earth 692 times. Known as the “Overview Effect”, many astronauts come back from space with a completely different fundamental view of our planet. A new perspective in the space sector has been possible thanks to many analysis tools that offer visualization of data, and have proven to be beneficial, since they make us understand our planet Earth better and unravel the mysteries of the universe.
那就是前美国国家航空航天局宇航员唐·托马斯(Don Thomas)绕地球旋转692次的世界观。 被称为“概述效应”的许多宇航员从太空回来时,对我们的星球有了完全不同的基本看法。 由于许多分析工具可提供数据可视化,并且已被证明是有益的,因为它们使我们更好地了解了地球并揭开了宇宙的奥秘,因此有可能在航天领域提出新的观点。
Big data technology is the product of information technology that aims to meet the challenges faced by increasing the amount of information in various fields. If we think of all the times we’ve used our phone or computer, how many apps have we logged in to? Have we checked Facebook, Twitter, Instagram, Reddit, or LinkedIn? Do we regularly use Amazon, YouTube, Tinder, Buzzfeed, or Pinterest? Every one of those app stores or websites that many of us use on a daily basis collect user data to improve user experience and help companies to make educated business decisions. But that’s not all data can help us do.
大数据技术是信息技术的产物,旨在通过增加各个领域的信息量来应对所面临的挑战。 如果我们一直在考虑使用手机或计算机,那么我们登录了多少个应用程序? 我们是否检查过Facebook,Twitter,Instagram,Reddit或LinkedIn? 我们是否经常使用Amazon,YouTube,Tinder,Buzzfeed或Pinterest? 我们许多人每天使用的这些应用商店或网站中的每一个,都收集用户数据以改善用户体验并帮助公司做出有根据的业务决策。 但这并不是所有数据都能帮助我们。
Right now, satellites are performing 2 billion instructions per second and delivering data that could help us prevent natural disasters and use natural resources wisely. There have been several data-driven initiatives to make better decisions and improve operational efficiency in sectors including agriculture, forestry, mapping, shipping, or energy.
目前,卫星正在每秒执行20亿条指令,并提供可帮助我们预防自然灾害并明智地使用自然资源的数据。 在农业,林业,制图,航运或能源等部门,有数项以数据为依据的计划可以做出更好的决策并提高运营效率。
使用数据改善地球上的生命 (Using Data to improve life on Earth)
More and more companies are starting to open to the space sector as the ever-growing number of affordable satellite services keeps increasing. Considering one industry — agriculture — the implications are enormous. Farmers can use image data to better understand what factors affect the growth of crops, and there are factors that can be detected from space, such as weather patterns, exposure to sunlight, air quality or pest activity, so optimum conditions can be determined.
随着负担得起的卫星服务数量的不断增长,越来越多的公司开始向太空领域开放。 考虑到一个产业-农业,其影响是巨大的。 农民可以使用图像数据更好地了解哪些因素会影响农作物的生长,并且可以从太空中检测到一些因素,例如天气模式,暴露在阳光下,空气质量或害虫活动,因此可以确定最佳条件。
In a few short decades, the world’s population is on pace to grow 50 percent by 2100. Now more than ever, farmers need access to tools that support the decisions they make every day to maximize their return on every acre. The Climate Corporation processes its satellite data to enable farmers to find more sustainable ways to grow more food. This company’s project’s key aspects can deliver benefits to humanity in the long term.
在短短的几十年中,到2100年,世界人口的增长速度将达到50%。如今,农民比以往任何时候都需要更多的工具来支持他们每天做出的决定,以使每英亩土地的收益最大化。 气候公司处理其卫星数据,以使农民能够找到更可持续的方式来种植更多的粮食。 该公司项目的关键方面可以长期为人类带来好处。
Another company, Planet, provides geospatial insights equipping users with the data necessary to make informed, timely decisions offering a diverse selection of imagery and analytic solutions, all made available online through their platform and web-based tools. From agriculture and emergency response to natural resource protection and security, global imagery and foundational analytics will empower informed, deliberate, and meaningful stewardship of our planet.
另一家公司Planet则提供地理空间洞察力,为用户提供必要的数据,以便他们能够及时做出明智的决定,从而提供各种图像和分析解决方案,所有这些都可以通过其平台和基于Web的工具在线获得。 从农业和应急响应到自然资源保护和安全,全球图像和基础分析将使我们星球的知情,深思熟虑和有意义的管理工作变得更加重要。
Earth observation satellites provide important data that allows the rapid detection of changes to the environment and climate, or measurements of the movement or shrinking of glaciers. Up-to-date maps can be provided to the emergency services in the event of disasters such as flooding or earthquakes. This, however, requires the accumulation of very large quantities of data. The European Union (EU) Copernicus Program satellites are among the biggest producers of data in the world. Their high-resolution instruments currently generate approximately 20 terabytes of data every day. This is equivalent to an HD film that would run for about one-and-a-half years. In addition to this, data is also provided by German missions such as TerraSAR-X and TanDEM-X, as well as an increasing number of other sources, such as the internet and measurement stations. The processing and analysis of these very large and heterogeneous data sets are among the Big Data challenges facing an increasingly digital society.
地球观测卫星提供重要的数据,可以快速检测环境和气候的变化,或者测量冰川的运动或萎缩。 如果发生洪水或地震等灾害,可以将最新地图提供给紧急服务。 但是,这需要积累大量数据。 欧盟(EU)哥白尼计划卫星是世界上最大的数据生产商之一。 他们的高分辨率仪器目前每天大约产生20 TB的数据。 这相当于一部高清电影,播放时间约为一年半。 除此之外,TerraSAR-X和TanDEM-X等德国特派团还提供了数据,以及越来越多的其他来源(例如互联网和测量站)也提供了数据。 这些越来越大的异构数据集的处理和分析是数字社会日益面临的大数据挑战之一。

New ideas and concepts are needed in order to be able to process data and turn it into information. Artificial intelligence plays a major role in this, as such processes are extremely powerful, especially where large amounts of data are involved. DLR scientist Xiaoxiang Zhu, based at the Technical University of Munich, is conducting research into the use of such methods. Together with her team, Zhu is developing exploratory algorithms from signal processing and artificial intelligence (AI), particularly machine learning, to significantly improve the acquisition of global geoinformation from satellite data and achieve breakthroughs in geosciences and environmental sciences. Novel data science algorithms allow scientists to go one step further with the merging of petabytes of data from complementary geo-relevant sources, ranging from Earth observation satellites to social media networks. Their findings have the potential to address previously insoluble challenges, such as recording and mapping global urbanization — one of the most important megatrends in global change.
为了能够处理数据并将其转变为信息,需要新的想法和概念。 人工智能在其中起着重要作用,因为这种过程非常强大,尤其是在涉及大量数据的情况下。 来自慕尼黑工业大学的DLR科学家Zhu Xiaoxiang Zhu正在研究这种方法的使用。 Zhu与她的团队一起,正在开发信号处理和人工智能(AI)(尤其是机器学习)的探索性算法,以显着改善从卫星数据中获取全球地理信息的过程,并在地球科学和环境科学方面取得突破。 新颖的数据科学算法使科学家可以更进一步地合并来自地理相关的补充资源(从地球观测卫星到社交媒体网络)的PB级数据。 他们的发现有可能解决以前无法解决的挑战,例如记录和绘制全球城市化进程-这是全球变化中最重要的大趋势之一。
Yet the field of satellite remote sensing is not alone in grappling with this challenge. Investigating phenomena, the other way round — looking from Earth into space — also generates enormous amounts of data. Telescopes such as the Square Kilometre Array (SKA) in South Africa and Australia provide large quantities of data, as do ESA’s space-based telescopes, for example, Gaia and Euclid. The systematic analysis of archive data by self-learning AI programs is thus becoming increasingly important in astronomical research.
然而,并非只有卫星遥感领域能够应对这一挑战。 反之,从地球到太空,调查现象也会产生大量数据。 诸如南非和澳大利亚的平方公里阵列(SKA)之类的望远镜提供了大量数据,ESA的天基望远镜也是如此,例如盖亚(Gaia)和欧几里得(Euclid)。 因此,通过自学AI程序对档案数据进行系统的分析在天文研究中变得越来越重要。
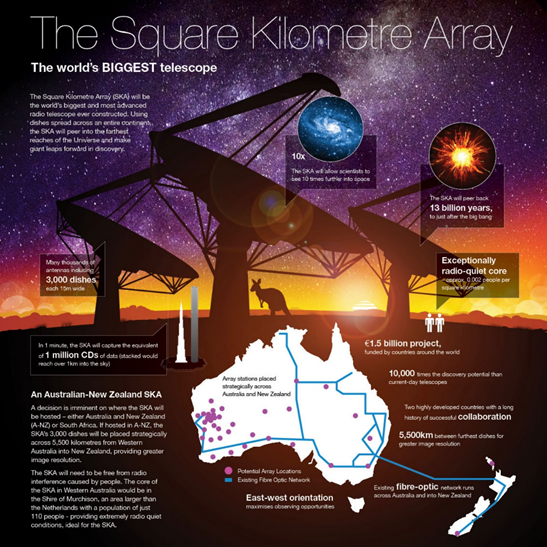
“We’ve been talking about Big Data for a long time, and this takes us on the journey to start understanding space data and space analytics. Not too many people in the commercial sector have got their hands around it yet, they don’t fully understand the implications of all of this data” said Sparks & Honey CEO Terry Young. “The idea was to look at the innovations that are going to be created over the next 15 years on our journey to Mars and beyond, and to find from those innovations — which are very science or engineering-focused — what the implications are for organizations and consumers, back here on Earth”.
“我们讨论大数据已经很长时间了,这使我们踏上了开始理解空间数据和空间分析的旅程。 商业领域的人还不是很多,他们还不完全理解所有这些数据的含义。” Sparks&Honey首席执行官Terry Young说。 “我们的想法是研究在接下来的15年中,在我们前往火星及以后的旅程中将要创造的创新,并从那些非常注重科学或工程学的创新中找出对组织的影响和消费者,回到地球上来”。
In the past, space data applications have been mainly carried out by Governments because of the sky-high cost of launching satellites and keeping them in space, where they could generate data with cameras, sensors and scanners, or used to monitor conflicts, track the flow of refugees and gather terrestrial and space data for research purposes. Thanks to the likes of SpaceX, founded by Tesla entrepreneur Elon Musk, as well as hundreds of startups, billions will be spent in the coming decade on creating infrastructure. The exciting part for the industry is that much of this data will become available for organizations whose business is not primarily space-based.
过去,空间数据的应用主要由各国政府执行,这是因为发射卫星并将其保持在太空的成本很高,在这里它们可以利用照相机,传感器和扫描仪生成数据,或用于监测冲突,跟踪卫星和卫星。难民的流动,并收集地面和空间数据以供研究。 得益于由特斯拉企业家埃隆·马斯克(Elon Musk)创立的SpaceX以及数百家初创公司,未来十年,数十亿美元将用于创建基础架构。 该行业令人兴奋的部分是,这些数据中的大部分将可用于其业务主要不是基于空间的组织。
“Something which is hovering above the Earth and providing a perspective from above is really creating a unique dataset. Roughly 35% of the satellites in orbit right now are there for commercial purposes, and those satellites have been driven by venture capital money. A lot of startups are providing low-orbit satellites for a wide range of different uses”. “We covered ideas like being able to observe things like water shortage, as it relates to manufacturing processes, traffic patterns in large cities as we are looking towards building cities of the future and their infrastructure. We can even translate it to big retail, where all of a sudden, we can capture real-time data on hundreds of stores simultaneously and use it to look at foot traffic patterns,” Young said.
“悬停在地球上方并从上方提供视角的事物确实在创建一个独特的数据集。 目前,在轨卫星中约有35%用于商业目的,而这些卫星是由风险投资推动的。 许多初创公司正在提供低轨道卫星,以用于各种不同的用途。” “我们涵盖了诸如能够观察到缺水之类的想法,因为它与制造过程,大城市的交通方式有关,我们正致力于建设未来的城市及其基础设施。 我们甚至可以将其转换为大型零售店,突然之间,我们可以同时在数百家商店中捕获实时数据,并使用它来查看人流情况。”
为什么空间数据是新的大数据 (Why Space Data is the new Big Data)
Data analytics can be used to improve sports performance, to help us better understand and build cures for disease, to aid in the development of artificial intelligence, to improve infrastructure in your city, and to expand the reach of what science can do. NASA has recently used data gathered over years of exploration to launch an amazing interactive map of Mars. Called, “Mars Trek,” the map is an educational tool NASA has available to the public as part of their Mars Exploration Program. Here’s the link: https://trek.nasa.gov/mars/
数据分析可用于改善运动表现,帮助我们更好地理解和建立疾病治疗方法,帮助开发人工智能,改善城市基础设施以及扩大科学工作范围。 美国国家航空航天局(NASA)最近使用了经过多年探索收集的数据,以发射出惊人的火星互动式地图。 该地图被称为“火星迷航”,是NASA作为其“火星探索计划”的一部分向公众提供的一种教育工具。 这是链接: https : //trek.nasa.gov/mars/
According to NASA’s official Mars Trek site, “This portal showcases data collected by NASA at various landing sites. It features an easy-to-use browsing tool which provides layering and viewing of high-resolution Mars data products in 2D and Globe view allowing users to fly over the surface of Mars. It also provides a set of tools including 3D printing, elevation profiles, sun angle calculations, Sun and Earth position, as well as bookmarks for the exploration area by NASA missions”. These “missions” which have supplied the majority of the data for the map to date are specifically the MSL (Mars Science Laboratory) mission, which involved the Curiosity Rover, the MER (Mars Exploration Rovers) mission, which included Spirit and Opportunity, the Phoenix mission, and the Pathfinder mission. NASA plans to continue to update the map as new data becomes available.
根据NASA的火星迷航官方网站,“该门户网站展示了NASA在各个着陆点收集的数据。 它具有易于使用的浏览工具,可在2D和Globe视图中对高分辨率火星数据产品进行分层和查看,从而使用户可以在火星表面上飞行。 它还提供了一套工具,包括3D打印,高程剖面图,太阳角度计算,太阳和地球位置,以及NASA任务在探索区域的书签。” 这些“任务”迄今已提供了大部分地图数据,特别是MSL(火星科学实验室)任务,其中包括好奇号火星车,MER(火星探索漫游者)任务,包括“精神与机遇”,凤凰任务和探路者任务。 NASA计划在有新数据可用时继续更新地图。
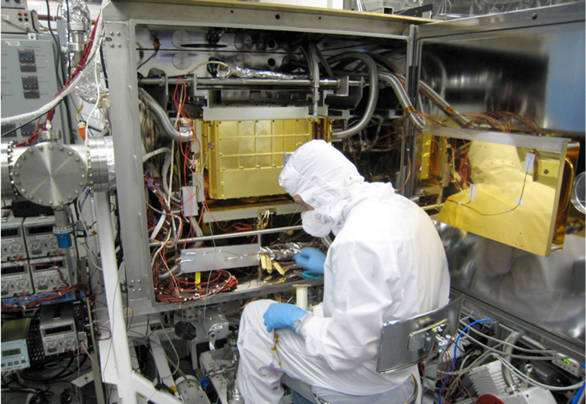
This is especially exciting as the Mars 2020 rover should be bringing us a whole new supply of data to add to the map by 2021. Modeled after the Curiosity, which has been a breakthrough unmanned system for NASA, the 2020 rover which launched on 30 July 2020 at 11:50 UTC will explore the habitability of Mars, hopefully paving the way for NASA’s manned missions tentatively planned for 2030.
这尤其令人兴奋,因为2020年火星探测器应该为我们带来全新的数据供应,以便在2021年之前添加到地图上。该模型仿效了好奇号(这是NASA的突破性无人驾驶系统),于7月30日发射的2020年火星探测器。 2020年世界标准时间(UTC)将探索火星的可居住性,希望为暂定于2030年进行的NASA载人飞行铺平道路。
数据,更多数据和PB级数据 (Data, more data, and petabytes of data)
Even in the healthcare sector data are largely mentioned. Pathologists have been diagnosing disease the same way for the past 100 years, by manually reviewing images under a microscope. Now, computers help doctors improve accuracy and significantly change the way cancer and other diseases are diagnosed.
即使在医疗保健领域,也大量提及数据。 在过去的100年中,病理学家通过在显微镜下手动查看图像来以相同的方式诊断疾病。 现在,计算机可以帮助医生提高准确性,并显着改变诊断癌症和其他疾病的方式。
Artificial intelligence (AI) methods have been developed by a research team from Harvard Medical School and Beth Israel Deaconess Medical Center that aimed at training computers to interpret pathology images, with the long-term goal of building AI-powered systems to make pathologic diagnoses more accurate.
哈佛医学院和贝斯以色列女执事医学中心的研究团队开发了人工智能(AI)方法,旨在培训计算机以解释病理图像,其长期目标是构建AI驱动的系统以进行更多的病理诊断。准确。
“Our AI method is based on deep learning, a machine-learning algorithm used for a range of applications including speech recognition and image recognition,” explained pathologist Andrew Beck, HMS associate professor of pathology and director of bioinformatics at the Cancer Research Institute at Beth Israel Deaconess. “This approach teaches machines to interpret the complex patterns and structure observed in real-life data by building multi-layer artificial neural networks, in a process which is thought to show similarities with the learning process that occurs in layers of neurons in the brain’s neocortex, the region where thinking occurs”.
“我们的AI方法基于深度学习,这是一种机器学习算法,可用于包括语音识别和图像识别在内的一系列应用,” HMS病理学副教授兼Beth癌症研究所生物信息学负责人病理学家Andrew Beck解释说。以色列女执事。 “这种方法教机器通过构建多层人工神经网络来解释现实数据中观察到的复杂模式和结构,这一过程被认为与大脑新皮层神经元层中发生的学习过程相似。 ,即发生思考的区域”。
“Identifying the presence or absence of metastatic cancer in a patient’s lymph nodes is a routine and critically important task for pathologists,” Beck explained. “Peering into the microscope to sift through millions of normal cells to identify just a few malignant cells can prove extremely laborious using conventional methods. We thought this was a task that the computer could be quite good at — and that proved to be the case”. In an objective evaluation in which researchers were given slides of lymph node cells and asked to determine whether they contained cancer, the team’s automated diagnostic method proved accurate approximately 92 percent of the time, said Khosla, adding, “This nearly matched the success rate of a human pathologist, whose results were 96 percent accurate”.
贝克解释说:“确定患者淋巴结中是否存在转移性癌症是一项常规且至关重要的任务,”病理学家说。 使用传统方法,在显微镜下窥视以筛选数百万个正常细胞以鉴定出少数恶性细胞可能非常费力。 我们认为这是计算机可以非常擅长的一项任务,事实证明确实如此。” Khosla表示,在一项客观评估中,研究人员被给予了淋巴结细胞切片并被要求确定它们是否含有癌症,该团队的自动诊断方法在大约92%的时间内被证明是准确的。一位人类病理学家,其结果准确率为96%”。
“But the truly exciting thing was when we combined the pathologist’s analysis with our automated computational diagnostic method, the result improved to 99.5 percent accuracy,” said Beck. “Combining these two methods yielded a major reduction in errors”.
贝克说:“但是真正令人兴奋的是,当我们将病理学家的分析与我们的自动化计算诊断方法结合在一起时,结果的准确性提高到了99.5%。” “将这两种方法结合起来可以大大减少错误”。
The team trained the computer to distinguish between cancerous tumor regions and normal regions based on a deep, multilayer convolutional network. To accomplish this, researchers had to amass huge amounts of data from which they could train their machine learning models.
该团队训练了计算机,以基于深度的多层卷积网络区分癌性肿瘤区域和正常区域。 为此,研究人员必须积累大量数据,他们可以从中训练机器学习模型。
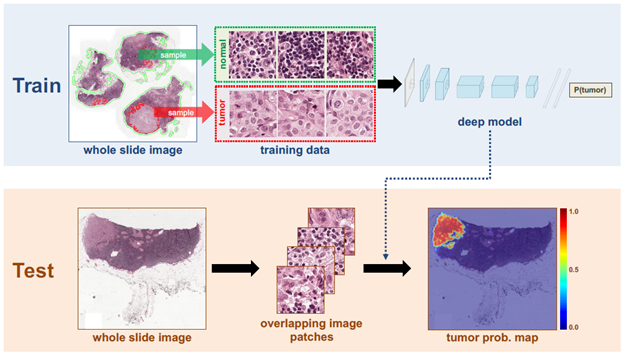
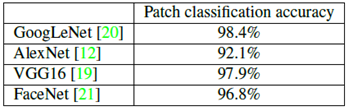
And it isn’t just radiology. The emerging field of gene therapy maps pathologies to specific genetic mutations. This means that newly diagnosed cancer patients now routinely have their genes sequenced so oncologists can prescribe the most effective treatment.
这不仅是放射学。 基因治疗的新兴领域将病理学映射到特定的基因突变。 这意味着现在对新诊断的癌症患者常规进行基因测序,以便肿瘤科医生可以开出最有效的治疗方案。
The key to both of these life-saving advances? Petabytes and petabytes of data.
这两项救生措施的关键是什么? PB和PB的数据。
未来的前景以及全球开放访问数据的努力 (What the future holds and the global effort for open access to data)
Back in 2016, Piero Scaruffi, cognitive scientist and author of “History of Silicon Valley” said: “The difference between oil and data is that the product of oil does not generate more oil (unfortunately), whereas the product of data (self-driving cars, drones, wearables) will generate more data (where do you normally drive, how fast/well you drive, who is with you)”.
早在2016年,认知科学家兼《硅谷历史》的作者Piero Scaruffi说:“石油与数据之间的区别在于,石油产品不会产生更多的石油(不幸的是),而数据产品(自驾驶汽车,无人驾驶飞机,可穿戴设备)将生成更多数据(您通常在哪里驾驶,驾驶速度/速度有多快,与谁在一起)。

Open data, big data and technology revolutions are stimulating for businesses, governments, and citizens.
开放数据,大数据和技术革命正在刺激企业,政府和公民。
Today, the industry is witnessing a wide variety of downsized technologies — miniaturization of sensors and satellites; a high number of private entrepreneurial missions, and adoption of new technologies such as AR/VR, artificial intelligence and machine learning, cloud, etc. How do we make all this data accessible for everyone? By making it open. Providing better environmental satellite data sharing policies and making practical recommendations for increasing global data sharing.
如今,该行业正在目睹各种尺寸缩小的技术-传感器和卫星的小型化; 大量的私人企业访问,以及采用新技术(例如AR / VR,人工智能和机器学习,云计算等)。我们如何使所有人都能使用所有这些数据? 通过使其打开。 提供更好的环境卫星数据共享策略,并为增加全球数据共享提出实用建议。
Open.NASA, for example, is an open innovation program in NASA’s Innovation Division, which creates many open data programs for both space professionals and enthusiasts. The NASA Space Apps Challenge Hackathon, NASA Datanauts, and the Data Bootcamp are projects which provide opportunities for citizens to easily get access and innovate with NASA’s open data, code, and APIs. All of this and much more is becoming plausible with an increase in space investments. More private sector companies — large, medium and small — are entering the earth observation foray redefining the very meaning of the what the future holds.
例如,Open.NASA是NASA创新部的一项开放式创新计划,该计划为航天专业人员和爱好者创建许多开放式数据计划。 NASA太空应用程序挑战Hackathon,NASA Datanauts和Data Bootcamp是为公民提供机会的项目,可让他们轻松地使用NASA的开放数据,代码和API进行访问和创新。 随着空间投资的增加,所有这些以及更多的东西变得合理。 越来越多的大型,中型和小型私营部门公司正在进入地球观测之路,重新定义未来的意义。
Autonomous vehicles (AVs) are also coming too. The benefits are widely known: safer roads, a boost to the economy and less rush-hour crowding. But perhaps the biggest benefit is a reduction in greenhouse gases (GHG) coming from automobiles. Research conducted by Poznan University professors estimates that autonomous vehicles could eventually reduce GHG by 40% to 60%. In this case, it requires hundreds of petabytes of data that form the data lake from which the AV self-driving advanced machine learning solutions will come. It doesn’t stop there. Each of these modern “computing platforms that happen to be mobile” will generate terabytes of data per week per vehicle. Even assuming a 75% reduction in the number of vehicles on the roads, that’s many exabytes of data per year. If a vehicle accident occurs, you can call up the images that the vehicles involved recorded to decide what caused the accident and which AV algorithms need improvements.
无人驾驶汽车(AVs)也即将问世。 好处广为人知:更安全的道路,促进经济发展和减少高峰时间拥挤。 但是,最大的好处也许是减少了汽车产生的温室气体(GHG)。 波兹南大学教授进行的研究估计,自动驾驶汽车最终将使温室气体排放减少40%至60%。 在这种情况下,它需要数百PB的数据来形成数据湖,AV自动驾驶高级机器学习解决方案将来自该数据湖。 它不止于此。 这些现代的“可移动的计算平台”每个都会每周每辆车产生TB级的数据。 即使假设道路上的车辆数量减少了75%,每年的数据量也高达EB级。 如果发生交通事故,您可以调出有关车辆记录的图像,以确定造成事故的原因以及需要改进的AV算法。
We are on the cusp of exploring an unprecedented abundance of innovation, research, resources and technological connection. All with Earth-bound resonance. Space isn’t just a moonshot. It’s transforming life, not just in orbit, but here on Earth.
我们正处于探索创新,研究,资源和技术联系空前丰富的风口浪尖上。 全部具有与地球相关的共振。 太空不仅仅是月亮。 它正在改变生命,不仅在轨道上,而且还在地球上。
And data isn’t just shaping the way our businesses run, it is shaping our lives.
数据不仅影响着我们的业务运营方式,还影响着我们的生活。
Originally published at https://westeastspace.com on August 1, 2020.
最初于 2020年8月1日 发布在 https://westeastspace.com 上。
翻译自: https://medium.com/@alessandro.prosperi123/the-rise-of-big-data-as-the-core-lubricant-of-the-digital-world-3d647b28e3ec
数字经济的核心是对大数据
本文来自互联网用户投稿,该文观点仅代表作者本人,不代表本站立场。本站仅提供信息存储空间服务,不拥有所有权,不承担相关法律责任。如若转载,请注明出处:http://www.mzph.cn/news/391806.shtml
如若内容造成侵权/违法违规/事实不符,请联系多彩编程网进行投诉反馈email:809451989@qq.com,一经查实,立即删除!