numpy 线性代数
Machine learning and deep learning models are data-hungry. The performance of them is highly dependent on the amount of data. Thus, we tend to collect as much data as possible in order to build a robust and accurate model. Data is collected in many different formats from numbers to images, from text to sound waves. However, we need to convert the data to numbers in order to analyze and model it.
机器学习和深度学习模型需要大量数据。 它们的性能高度依赖于数据量。 因此,我们倾向于收集尽可能多的数据,以建立可靠而准确的模型。 数据以多种不同的格式收集,从数字到图像,从文本到声波。 但是,我们需要将数据转换为数字,以便对其进行分析和建模。
It is not enough just to convert data to scalars (single numbers). As the amount of data increases, the operations done with scalars start to be inefficient. We need vectorized or matrix operations to make computations efficiently. That’s where linear algebra comes into play.
仅将数据转换为标量(单个数字)是不够的。 随着数据量的增加,使用标量执行的操作开始效率低下。 我们需要向量化或矩阵运算来有效地进行计算。 那就是线性代数起作用的地方。
Linear algebra is one of the most important topics in data science domain. In this post, we will cover the basic concepts in linear algebra with examples using NumPy.
线性代数是数据科学领域中最重要的主题之一。 在本文中,我们将使用NumPy的示例介绍线性代数的基本概念。
NumPy is a scientific computing library for Python and forms the basis of many libraries such as Pandas.
NumPy是用于Python的科学计算库,它构成了许多库(例如Pandas)的基础。
线性代数中的对象类型 (Types of Objects in Linear Algebra)
Types of objects (or data structures) in linear algebra:
线性代数中的对象(或数据结构)类型:
- Scalar: Single number 标量:单个数字
- Vector: Array of numbers 向量:数字数组
- Matrix: 2-dimensional array of numbers 矩阵:二维数字数组
- Tensor: N-dimensional array of numbers where n > 2 张量:N维数数组,其中n> 2
A scalar is just a number. It can be used in vectorized operations as we will see in the following examples.
标量只是一个数字。 如下面的示例所示,它可以用于矢量化操作。
A vector is an array of numbers. For instance, following is a vector with 5 elements:
向量是数字数组。 例如,下面是一个包含5个元素的向量:

We can use scalars in vectorized operations. The specified operation is done on each element of the vector and scalar.
我们可以在向量化运算中使用标量。 对向量和标量的每个元素执行指定的操作。

A matrix is a 2-dimensional vector.
矩阵是二维向量。

It seems like a pandas dataframe with rows and columns. Actually, pandas dataframes are converted to matrices and then fed into machine learning models.
好像是一个带有行和列的熊猫数据框。 实际上,熊猫数据帧会转换为矩阵,然后输入到机器学习模型中。
A tensor is an N-dimensional array of numbers where N is greater than 2. Tensors are mostly used in deep learning models where the input data is 3-dimensional.
张量是数字的N维数组,其中N大于2。张量通常用于输入数据为3维的深度学习模型中。

It is hard easy to represent with numbers but think of T as 3 matrices with a shape of 3x2.
很难用数字表示,但是将T视为3x2形状的3个矩阵。
The shape method can be used to check the shape of a numpy array.
shape方法可用于检查numpy数组的形状 。

The size of an array is calculated by multiplying the size in each dimension.
数组的大小是通过将每个维度的大小相乘得出的。
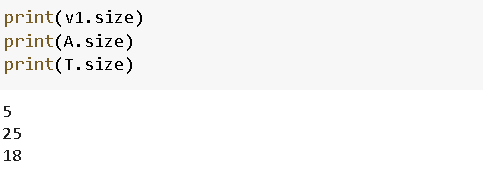
通用矩阵术语 (Common Matrix Terms)
A matrix is called square if number of rows is equal to the number of columns. thus, the matrix A above is a square matrix.
如果行数等于列数,则矩阵称为正方形 。 因此,上面的矩阵A是正方形矩阵。
Identity matrix, denoted as I, is a square matrix that have 1’s on the diagonal and 0’s at all other positions. Identity function of NumPy can be used create identity matrices of any size.
单位矩阵,表示为I,是一个对角线为1且在所有其他位置为0的方阵。 NumPy的身份函数可用于创建任何大小的身份矩阵。

What makes an identity matrix special is that it does not change a matrix when multiplied. In this sense, it is similar to number 1 in real numbers. We will do examples with identity matrix in matrix multiplication part of this post.
使单位矩阵与众不同的原因是,乘法时它不会改变矩阵。 从这个意义上讲,它与实数上的数字1相似。 我们将在本文的矩阵乘法部分中以恒等矩阵为例。
The inverse of a matrix is the matrix that gives the identity matrix when multiplied with the original matrix.
矩阵的逆矩阵是与原始矩阵相乘时给出单位矩阵的矩阵。

Not every matrix has an inverse. If matrix A has an inverse, then it is called invertible or non-singular.
并非每个矩阵都有逆。 如果矩阵A具有逆,则称其为可逆 或非奇异。
点积和矩阵乘法 (Dot Product and Matrix Multiplication)
Dot product and matrix multiplication are the building blocks of complex machine learning and deep learning models so it is highly valuable to have a comprehensive understanding of them.
点积和矩阵乘法是复杂的机器学习和深度学习模型的基础,因此全面了解它们非常有价值。
The dot product of two vectors is the sum of the products of elements with regards to their position. The first element of the first vector is multiplied by the first element of the second vector and so on. The sum of these products is the dot product. The function to compute dot product in NumPy is dot().
两个向量的点积是元素相对于其位置的乘积之和。 第一个向量的第一个元素乘以第二个向量的第一个元素,依此类推。 这些乘积之和为点积。 在NumPy中计算点积的函数是dot() 。
Let’s first create two simple vectors in the form of numpy arrays and calculate the dot product.
首先,我们以numpy数组的形式创建两个简单的向量,然后计算点积。

The dot product is calculated as (1*2)+(2*4)+(3*6) which is 28.
点积计算为(1 * 2)+(2 * 4)+(3 * 6),即28。
Since we multiply elements at the same positions, the two vectors must have same length in order to have a dot product.
由于我们在相同位置上乘以元素,因此两个向量必须具有相同的长度才能具有点积。
In the field of data science, we mostly deal with matrices. A matrix is a bunch of row and column vectors combined in a structured way. Thus, multiplication of two matrices involves many dot product operations of vectors. It will be more clear when we go over some examples. Let’s first create two 2x2 matrices with NumPy.
在数据科学领域,我们主要处理矩阵。 矩阵是以结构化方式组合的一堆行和列向量。 因此, 两个矩阵的乘法涉及向量的许多点积运算 。 当我们回顾一些示例时,将更加清楚。 我们首先使用NumPy创建两个2x2矩阵。


A 2x2 matrix has 2 rows and 2 columns. Index of rows and columns start with 0. For instance, the first row of A (row with index 0) is the array of [4,2]. The first column of A is the array of [4,0]. The element at first row and first column is 4.
2x2矩阵有2行2列。 行和列的索引以0开头。例如,A的第一行(索引为0的行)是[4,2]的数组。 A的第一列是[4,0]的数组。 第一行和第一列的元素是4。
We can access individual rows, columns, or elements as follows:
我们可以按以下方式访问单独的行,列或元素:

These are important concepts to comprehend matrix multiplication.
这些是理解矩阵乘法的重要概念。
Multiplication of two matrices involves dot products between rows of first matrix and columns of the second matrix. The first step is the dot product between the first row of A and the first column of B. The result of this dot product is the element of resulting matrix at position [0,0] (i.e. first row, first column).
两个矩阵的乘法涉及第一矩阵的行和第二矩阵的列之间的点积。 第一步是A的第一行和B的第一列之间的点积。该点积的结果是位置[0,0](即第一行,第一列)处所得矩阵的元素。

So the resulting matrix, C, will have a (4*4) + (2*1) at the first row and first column. C[0,0] = 18.
因此,所得矩阵C在第一行和第一列将具有(4 * 4)+(2 * 1)。 C [0,0] = 18
The next step is the dot product of the first row of A and the second column of B.
下一步是A的第一行和B的第二列的点积。

C will have a (4*0) + (2*4) at the first row and second column. C[0,1] = 8.
C在第一行和第二列将具有(4 * 0)+(2 * 4)。 C [0,1] = 8
First row A is complete so we start on the second row of A and follow the same steps.
第一行A已完成,因此我们从A的第二行开始并遵循相同的步骤。

C will have a (0*4) + (3*1) at the second row and first column. C[1,0] = 3.
C在第二行和第一列将具有(0 * 4)+(3 * 1)。 C [1,0] = 3。
The final step is the dot product between the second row of A and the second column of B.
最后一步是A的第二行和B的第二列之间的点积。

C will have a (0*0) + (3*4) at the second row and second column. C[1,1] = 12.
C在第二行和第二列将具有(0 * 0)+(3 * 4)。 C [1,1] = 12
We have seen how it is done step-by-step. All of these operations are done with a np.dot operation:
我们已经看到了它是如何逐步完成的。 所有这些操作都是通过np.dot操作完成的:

As you may recall, we have mentioned that identity matrix does not change a matrix when multiplied. Let’s do an example.
您可能还记得,我们已经提到了单位矩阵在相乘时不会改变。 让我们做一个例子。

We have also mentioned that when a matrix is multiplied by its inverse, the result is the identity matrix. Let’s first create a matrix and find its inverse. We can use linalg.inv() function of NumPy to find the inverse of a matrix.
我们还提到过,当矩阵乘以其逆矩阵时,结果就是单位矩阵。 首先创建一个矩阵并找到其逆矩阵。 我们可以使用NumPy的linalg.inv()函数来查找矩阵的逆。

Let’s multiply B with its inverse matrix, C :
让我们将B与其逆矩阵C相乘:

Bingo! We have the identity matrix.
答对了! 我们有单位矩阵。
As we recall from vector dot products, two vectors must have the same length in order to have a dot product. Each dot product operation in matrix multiplication must follow this rule. Dot products are done between the rows of the first matrix and the columns of the second matrix. Thus, the rows of the first matrix and columns of the second matrix must have the same length.
正如我们从向量点积中回忆起的那样, 两个向量必须具有相同的长度才能具有点积 。 矩阵乘法中的每个点积运算必须遵循此规则。 点积在第一矩阵的行和第二矩阵的列之间完成。 因此, 第一矩阵的行和第二矩阵的列必须具有相同的长度。
The requirement for matrix multiplication is that the number of columns of the first matrix must be equal to the number of rows of the second matrix.
矩阵乘法的要求是,第一个矩阵的列数必须等于第二个矩阵的行数。
For instance, we can multiply a 3x2 matrix with a 2x3 matrix.
例如,我们可以将3x2矩阵与2x3矩阵相乘。

The shape of the resulting matrix will be 3x3 because we are doing 3 dot product operations for each row of A and A has 3 rows. An easy way to determine the shape of the resulting matrix is to take the number of rows from the first one and the number of columns from the second one:
最终矩阵的形状将为3x3,因为我们对A的每一行进行了3个点积运算,而A具有3行。 确定结果矩阵形状的一种简单方法是从第一个矩阵中获取行数,从第二个矩阵中获取列数:
- 3x2 and 2x3 multiplication returns 3x3 3x2和2x3乘法返回3x3
- 3x2 and 2x2 multiplication returns 3x2 3x2和2x2乘法返回3x2
- 2x4 and 4x3 multiplication returns 2x3 2x4和4x3乘法返回2x3
We have covered basic but very fundamental operations of linear algebra. These basic operations are the building blocks of complex machine learning and deep learning models. Lots of matrix multiplication operations are done during the optimization process of models. Thus, it is highly important to understand the basics as well.
我们已经介绍了线性代数的基本但非常基本的运算。 这些基本操作是复杂的机器学习和深度学习模型的基础。 在模型优化过程中完成了许多矩阵乘法运算。 因此,了解基础知识也非常重要。
Thank you for reading. Please let me know if you have any feedback.
感谢您的阅读。 如果您有任何反馈意见,请告诉我。
翻译自: https://towardsdatascience.com/linear-algebra-for-data-scientists-explained-with-numpy-6fec26519aea
numpy 线性代数
本文来自互联网用户投稿,该文观点仅代表作者本人,不代表本站立场。本站仅提供信息存储空间服务,不拥有所有权,不承担相关法律责任。如若转载,请注明出处:http://www.mzph.cn/news/391702.shtml
如若内容造成侵权/违法违规/事实不符,请联系多彩编程网进行投诉反馈email:809451989@qq.com,一经查实,立即删除!