项目经济规模的估算方法
On June 23 2016, the United Kingdom narrowly voted in a country-wide referendum to leave the European Union (EU). Economists at the time warned of economic losses; the Bank of England produced estimates that that GDP could be as much as 10.5% lower than the previous trend.
2016年6月23日,英国在全国范围的公民投票中以微弱的票数离开了欧盟(EU)。 当时的经济学家警告经济损失。 英格兰银行(BoE) 估计 ,GDP可能比以前的趋势低10.5%。

The latest update from the Bank of England has lowered estimates: a 5.5% loss of GDP is now expected if a no-deal Brexit were to occur.
英格兰银行的最新消息降低了预期:如果无协议脱欧,现在预计GDP将下降5.5%。
The divorce has yet to happen of course, dragging on for three and a half years with no clear end in sight. However, the uncertainty and expectation that the UK will eventually succeed in leaving the EU is able to cause substantial harm to the economy even before the official withdrawal occurs. Here, I will be using Synthetic Control Method to produce a model that can estimate the economic impacts of Brexit so far. If you are simply interested in seeing the results and don’t care for the methodology, skip to the Conclusion section at the bottom.
离婚当然还没有发生,拖延了三年半,没有明确的结局。 但是,即使英国正式退出欧盟,英国最终能否成功退出欧盟的不确定性和期望仍可能对经济造成重大损害。 在这里,我将使用综合控制方法生成一个模型,该模型可以估计到目前为止英国脱欧的经济影响。 如果您只是对查看结果感兴趣,而不关心方法论,请跳至底部的结论部分。
方法 (Methodology)
For a sample of potential donor countries to form the synthetic control, I used all current OECD countries. The OECD consists of 36 mostly developed countries. Using OECD countries allows me to pull from OECD Data.
为了对潜在的捐助国进行综合控制,我使用了所有经合组织国家作为样本。 经合组织由36个最发达国家组成。 使用OECD国家可以使我从OECD数据中受益。
In constructing the synthetic control, I will be using the Synth package for R.
在构建综合控件时,我将使用Synth包用于R。
Selection of Predictors
预测变量的选择
To form the synthetic control, we need to include several variables that are predictive of our outcome variable (Real Gross Domestic Product per capita). I collected data on the following variables:
为了形成综合控制,我们需要包括几个可以预测结果变量(人均实际国内生产总值)的变量。 我收集了以下变量的数据:
- Exports as a percentage of GDP 出口占GDP的百分比
- Employment rate 就业率
- Working age population as a percentage of the total population. The working age population is defined as aged 15–64. 劳动年龄人口占总人口的百分比。 劳动年龄人口定义为15-64岁。
- Human capital. Specifically, the percentage of 25–34 year old’s with tertiary education. 人力资本。 具体来说,是25-34岁的大专以上学历的百分比。
Selection of Donor States
选择捐助国
In this process, any other countries that underwent a similar intervention should be removed. Luckily, no other countries have left the EU. As the OECD is mostly formed of relatively similar developed countries, I will not remove any from the sample.
在此过程中,任何接受过类似干预的国家都应删除。 幸运的是,没有其他国家离开欧盟。 由于经合组织主要由相对类似的发达国家组成,因此我不会从样本中删除任何内容。
Optimization Algorithm
优化算法
I will leave this as the default setting, which takes the best result from Nelder-Mead and BFGS. Nelder-Mead produces a better result in this case.
我将其保留为默认设置,它将获得Nelder-Mead和BFGS的最佳效果。 在这种情况下,Nelder-Mead会产生更好的结果。
I will optimize the model from 2000 to 2015.
我将从2000年到2015年对模型进行优化。
综合控制 (The Synthetic Control)
After running the function, we can review the synthetic control it has produced. The function has selected the following weights for our predictors:
运行该函数后,我们可以查看它产生的综合控件。 该函数为我们的预测变量选择了以下权重:

Note the synthetic is virtually identical to the UK in our predictor variables:
请注意,在我们的预测变量中,合成实际上与英国相同:
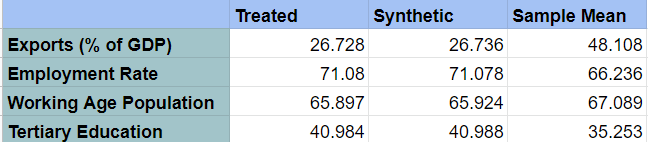
The synthetic is primarily composed of Japan (35%), Iceland (21.5%), and the US (14.4%) with smaller weights coming from several other countries. We can now see that our synthetic does a fairly good job of following the trends of the UK.
合成纤维主要由日本(35%),冰岛(21.5%)和美国(14.4%)组成,其重量较小来自其他几个国家。 现在我们可以看到,我们的合成材料在追随英国趋势方面做得相当不错。
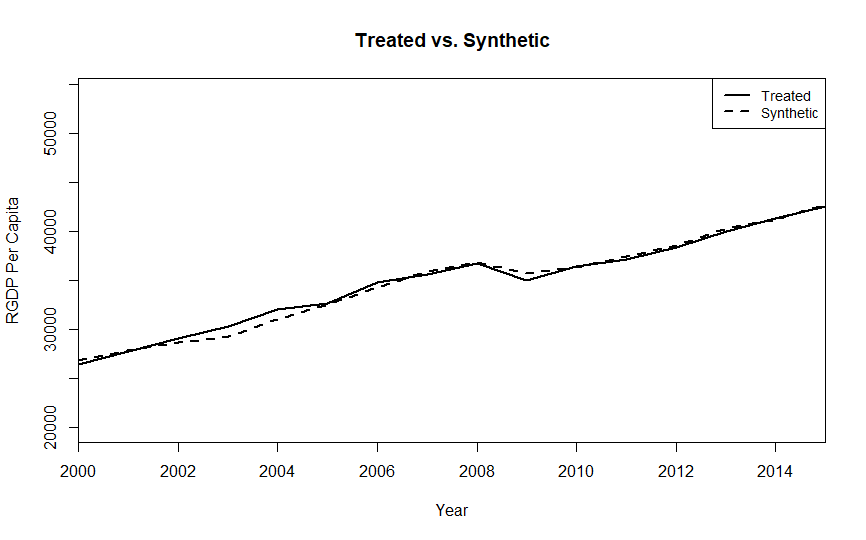
The period from 2002 to 2005 shows some deviation, but overall the result looks okay. The model has a Mean Squared Prediction Error (MSPE) of 214,588.
从2002年到2005年这段时期显示出一些偏差,但总体而言结果还不错。 该模型的均方预测误差(MSPE)为214,588。
结果 (Results)
We can now see a plot of the UK against the synthetic control extended to 2018.
现在我们可以看到英国针对合成控制的情节延至2018年。

The vertical red line represents the last year before the intervention (when the referendum took place).
垂直的红线表示干预前的最后一年(举行公民投票时)。
The size of the graph makes it it difficult to assess, so we are also able to view a plot of the gaps between the synthetic and the UK to view more easily view the differences:
该图的大小使其难以评估,因此我们还可以查看合成图和英国之间的差距图,从而更轻松地查看差异:
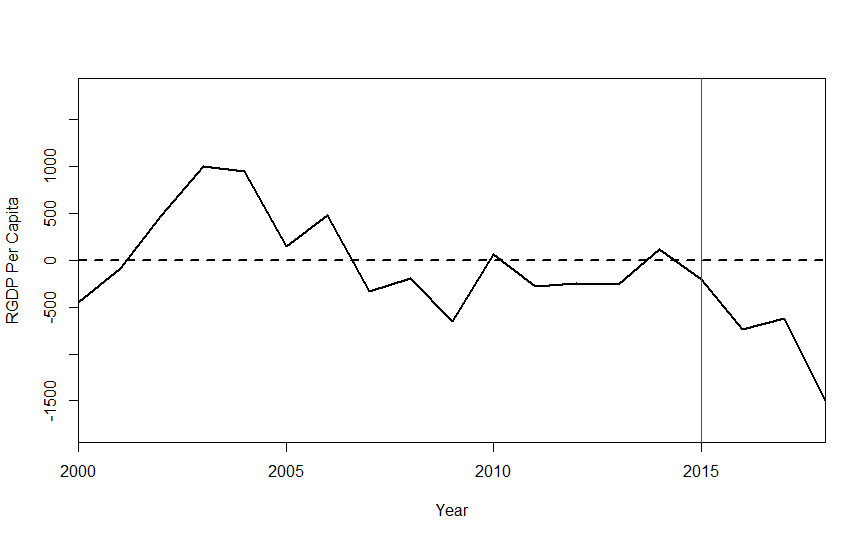
The model uses annual GDP, where the last year is 2018. As of this date, the UK has lost approximately $1500 per capita according to this estimate. While this model does suggest UK GDP is lower due to Brexit, the fact that the UK and the synthetic control don’t perfectly track each other means we can’t be certain of the magnitude. However, given weak GDP growth so far in 2019, we are likely to see the damage continue to grow.
该模型使用的年度GDP(去年是2018年)。根据该估计,截至该日期,英国人均损失了大约1500美元。 尽管该模型确实表明英国脱欧导致英国GDP下降,但英国和综合控制机构之间无法很好地相互追踪这一事实意味着我们无法确定其幅度。 但是,鉴于2019年迄今为止GDP增长疲软 ,我们很可能看到损失继续增加。
These results are broadly in line with results from most experts; two economists from the London School of Economics noted the UK has experienced slow-downs in GDP, investment, productivity growth, and a weakened currency since the referendum.
这些结果与大多数专家的结果基本一致; 伦敦经济学院的两位经济学家指出 ,自公投以来, 英国的 GDP,投资,生产率增长和货币走弱都经历了放缓。
结论 (Conclusion)
- This analysis suggests the UK has already experienced a significant economic hit due to the Brexit referendum 该分析表明,由于英国退欧公投,英国已经遭受了重大的经济打击
- The UK has likely lost about $1500 per person of GDP from the impacts of Brexit so far 迄今为止,英国可能因英国脱欧的影响而使每人GDP损失约1500美元
- If the UK had not voted to leave the EU, UK GDP per capita would likely be about 3.25% higher than it is right now 如果英国没有投票决定退出欧盟,那么英国人均GDP可能会比目前高出约3.25%。
- The economic damages are likely to get worse as the saga continues, and 2019 was very possibly the worst year for the UK economy yet 随着传奇的继续,经济损失可能会变得更糟,2019年很可能是英国经济最糟糕的一年
翻译自: https://medium.com/economic-watch/estimating-the-economic-impact-of-brexit-5fbbf7258790
项目经济规模的估算方法
本文来自互联网用户投稿,该文观点仅代表作者本人,不代表本站立场。本站仅提供信息存储空间服务,不拥有所有权,不承担相关法律责任。如若转载,请注明出处:http://www.mzph.cn/news/391466.shtml
如若内容造成侵权/违法违规/事实不符,请联系多彩编程网进行投诉反馈email:809451989@qq.com,一经查实,立即删除!