lime 模型
Article outline
文章大纲
- Introduction 介绍
- Data Background 资料背景
- Aim of the article 本文的目的
- Exploratory analysis 探索性分析
- Training a Random Forest Model 训练随机森林模型
- Global Importance 全球重要性
- Local Importance 当地重要性
介绍 (Introduction)
In the supervised machine learning world, there are two types of algorithmic task often performed. One is called regression (predicting continuous values) and the other is called classification (predicting discrete values). Black box algorithms such as SVM, random forest, boosted trees, neural networks provide better prediction accuracy than conventional algorithms. The problem starts when we want to understand the impact (magnitude and direction) of different variables. In this article, I have presented an example of Random Forest binary classification algorithm and its interpretation at the global and local level using Local Interpretable Model-agnostic Explanations (LIME).
在有监督的机器学习世界中,经常执行两种类型的算法任务。 一种称为回归(预测连续值),另一种称为分类(预测离散值)。 与传统算法相比,诸如SVM,随机森林,增强树,神经网络之类的黑匣子算法提供了更好的预测精度。 当我们想了解不同变量的影响(大小和方向)时,问题就开始了。 在本文中,我提供了一个使用本地可解释模型不可知解释(LIME)在全球和本地级别进行随机森林二进制分类算法及其解释的示例。
资料背景 (Data Background)
In this example, we are going to use the Pima Indian Diabetes 2 data set obtained from the UCI Repository of machine learning databases (Newman et al. 1998).
在本示例中,我们将使用从机器学习数据库的UCI存储库中获得的Pima Indian Diabetes 2数据集( Newman等,1998 )。
This data set is originally from the National Institute of Diabetes and Digestive and Kidney Diseases. The objective of the data set is to diagnostically predict whether or not a patient has diabetes, based on certain diagnostic measurements included in the data set. Several constraints were placed on the selection of these instances from a larger database. In particular, all patients here are females at least 21 years old of Pima Indian heritage.
该数据集最初来自美国国立糖尿病与消化与肾脏疾病研究所。 数据集的目的是根据数据集中包含的某些诊断测量值来诊断性预测患者是否患有糖尿病。 从较大的数据库中选择这些实例受到一些限制。 特别是,这里的所有患者均为皮马印第安人血统至少21岁的女性。
The Pima Indian Diabetes 2 data set is the refined version (all missing values were assigned as NA) of the Pima Indian diabetes data. The data set contains the following independent and dependent variables.
Pima印度糖尿病2数据集是Pima印度糖尿病数据的精炼版本(所有缺失值均指定为NA)。 数据集包含以下独立变量和因变量。
Independent variables (symbol: I)
自变量(符号:I)
I1: pregnant: Number of times pregnant
I1: 怀孕 :怀孕次数
I2: glucose: Plasma glucose concentration (glucose tolerance test)
I2: 葡萄糖 :血浆葡萄糖浓度(葡萄糖耐量试验)
I3: pressure: Diastolic blood pressure (mm Hg)
I3: 压力 :舒张压(毫米汞柱)
I4: triceps: Triceps skin fold thickness (mm)
I4: 三头肌 :三头肌的皮肤折叠厚度(毫米)
I5: insulin: 2-Hour serum insulin (mu U/ml)
I5: 胰岛素 :2小时血清胰岛素(mu U / ml)
I6: mass: Body mass index (weight in kg/(height in m)\²)
I6: 质量 :体重指数(重量,单位:kg /(身高,单位:m)\²)
I7: pedigree: Diabetes pedigree function
I7: 谱系 :糖尿病谱系功能
I8: age: Age (years)
I8: 年龄 :年龄(年)
Dependent Variable (symbol: D)
因变量(符号:D)
D1: diabetes: diabetes case (pos/neg)
D1: 糖尿病 :糖尿病病例(正/负)
建模目的 (Aim of the Modelling)
- fitting a random forest ensemble binary classification model that accurately predicts whether or not the patients in the data set have diabetes 拟合随机森林综合二元分类模型,该模型可准确预测数据集中的患者是否患有糖尿病
- understanding the global influence of variables on diabetes prediction 了解变量对糖尿病预测的全球影响
- understanding the influence of variables on the local level for the individual patient 了解变量对个体患者局部水平的影响
加载库 (Loading Libraries)
The very first step will be to load relevant libraries.
第一步将是加载相关的库。
import pandas as pd # data mnipulation
import numpy as np # number manipulation/crunching
import matplotlib.pyplot as plt # plotting# Classification report
from sklearn.metrics import classification_report # Train Test split
from sklearn.model_selection import train_test_split# Random forest classifier
from sklearn.ensemble import RandomForestClassifier
Reading dataset
读取数据集
After data loading, the next essential step is to perform an exploratory data analysis which helps in data familiarization. Use the head( ) function to view the top five rows of the data.
数据加载后,下一个基本步骤是执行探索性数据分析,这有助于数据熟悉。 使用head()函数查看数据的前五行。
diabetes = pd.read_csv("diabetes.csv")
diabetes.head()

The below table showed that the diabetes data set includes 392 observations and 9 columns/variables. The independent variables include integer 64 and float 64 data types, whereas dependent/response (diabetes) variable is of string (neg/pos) data type also known as an object.
下表显示糖尿病数据集包括392个观察值和9列/变量 。 自变量包括整数64和浮点64数据类型,而因变量/响应(糖尿病)变量为字符串(neg / pos)数据类型,也称为对象 。

Let's print the column names
让我们打印列名
diabetes.columns

将输出变量映射到0和1 (Mapping output variable into 0 and 1)
Before proceeding to model fitting, it is often essential to ensure that the data type is consistent with the library/package that you are going to use. In diabetes, data set the dependent variable (diabetes) consists of strings/characters i.e., neg/pos, which need to be converted into integers by mapping neg: 0 and pos: 1 using the .map( ) method.
在进行模型拟合之前,通常必须确保数据类型与要使用的库/包一致。 在糖尿病中,数据集因变量(糖尿病)由字符串/字符(即neg / pos )组成,需要使用.map()方法通过映射neg:0和pos:1将其转换为整数。
diabetes["diabetes"] = diabetes["diabetes"].map({"neg":0, "pos":1})diabetes["diabetes"].value_counts()
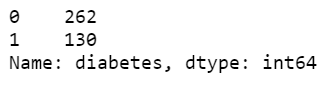
Now you can see that the dependent variable “diabetes” is converted from object to an integer 64 type.
现在您可以看到因变量“ diabetes ”从对象转换为整数64类型。

The next step is to gaining knowledge about basic data summary statistics using .describe( ) method, which computes count, mean, standard deviation, minimum, maximum and percentile (25th, 50th and 75th) values. This helps you to detect any anomaly in your dataset. Such as variables with high variance or extremely skewed data.
下一步是使用.describe()方法获取有关基本数据摘要统计信息的知识,该方法计算计数,均值,标准差,最小值,最大值和百分位数(第25、50和75位)。 这可以帮助您检测数据集中的任何异常。 例如具有高方差的变量或数据严重偏斜。

训练射频模型 (Training RF Model)
The next step is splitting the diabetes data set into train and test split using train_test_split of sklearn.model_selection module and fitting a random forest model using the sklearn package/library.
下一步是分裂糖尿病数据集到列车和使用sklearn.model_selection模块的train_test_split以及使用该sklearn包/库拟合随机森林模型试验分裂。
训练和测试拆分 (Train and Test Split)
The whole data set generally split into 80% train and 20% test data set (general rule of thumb). The 80% train data is being used for model training, while the rest 20% could be used for model generalized and local model interpretation.
整个数据集通常分为80%的训练数据集和20%的测试数据集(一般经验法则)。 80%的训练数据用于模型训练,而其余20%的数据可用于模型概括和局部模型解释。
Y = diabetes['diabetes']
X = diabetes[['pregnant', 'glucose', 'pressure', 'triceps', 'insulin', 'mass',
'pedigree', 'age']]X_featurenames = X.columns# Split the data into train and test data:
X_train, X_test, Y_train, Y_test = train_test_split(X, Y, test_size = 0.2)
In order to fit a Random Forest model, first, you need to install sklearn package/library and then you need to import RandomForestClassifier from sklearn.ensemble. Here, have fitted around 10000 trees with a max depth of 20.
为了适应随机森林模型,首先,您需要安装sklearn包/库,那么你需要从sklearn.ensemble进口RandomForestClassifier。 这里, 已安装约10000棵树,最大深度为20。
# Build the model with the random forest regression algorithm:
model = RandomForestClassifier(max_depth = 20, random_state = 0, n_estimators = 10000)
model.fit(X_train, Y_train)
分类报告 (Classification Report)
Let’s predict the test data class labels using predict( ) and generate a classification report. The classification report revealed that the micro average of F1 score (used for unbalanced data) is about 0.71, which indicates that the trained model has a classification strength of 71%.
让我们使用predict()预测测试数据类标签并生成分类报告。 分类报告显示,F1评分的微观平均值(用于不平衡数据)约为0.71,这表明训练后的模型的分类强度为71%。
y_pred = model.predict(X_test)print(classification_report(Y_test, y_pred, target_names=["Diabetes -ve", "Diabetes +ve"]))

特征重要性图 (Feature Importance Plot)
The advantage of tree-based algorithms is that it provides global variable importance, which means you can rank them based on their contribution to the model. Here, you can observe that the glucose variable has the highest influence in the model, followed by Insulin. The problem with global importance is that it gives an average overview of variable contributing to the model.
基于树的算法的优势在于它提供了全局变量重要性,这意味着您可以根据它们对模型的贡献对其进行排名。 在这里,您可以观察到葡萄糖变量在模型中影响最大,其次是胰岛素 。 具有全球重要性的问题是,它给出了有助于模型的变量的平均概图。
feat_importances = pd.Series(model.feature_importances_, index = X_featurenames)feat_importances.nlargest(5).plot(kind = 'barh')

From the BlackBox model, it is nearly impossible to get a feeling for its inner functioning. This brings us to a question of trust: do you trust that a certain prediction from the model is correct? Or do you even trust that the model is making sound predictions?
从BlackBox模型中,几乎不可能对它的内部功能有所了解。 这给我们带来了一个信任问题:您是否相信模型中的某个预测是正确的? 还是您甚至相信模型可以做出合理的预测?
创建一个模型解释器 (Creating a model explainer)
LIME is short for Local Interpretable Model-Agnostic Explanations. Local refers to local fidelity — i.e., we want the explanation to really reflect the behaviour of the classifier “around” the instance being predicted. This explanation is useless unless it is interpretable — that is, unless a human can make sense of it. Lime is able to explain any model without needing to ‘peak’ into it, so it is model-agnostic.
LIME是本地可解释模型不可知的解释的缩写。 本地是指本地保真度-即,我们希望解释能真正反映分类器在“预测”实例周围的行为。 除非可以解释 ,否则这种解释是无用的,也就是说,除非人类可以理解。 Lime能够解释任何模型而无需“说话”,所以它与模型无关 。
Behind the workings of LIME lies the assumption that every complex model is linear on a local scale and asserting that it is possible to fit a simple model around a single observation that will mimic how the global model behaves at that locality (Pedersen and Benesty, 2016)
LIME的工作原理是,假设每个复杂模型在局部范围内都是线性的,并断言有可能在单个观测值附近拟合一个简单模型,以模拟全局模型在该位置的行为(Pedersen和Benesty,2016年) )
LIME explainer fitting steps
LIME解释器安装步骤
- import the lime library 导入石灰库
- import lime.lime_tabular 导入lime.lime_tabular
Fit an explainer using LimeTabularExplainer( ) function
使用LimeTabularExplainer()函数拟合解释器
Supply the x_train values, feature names and class names as ‘Diabetes -ve’, ‘Diabetes +ve’
提供x_train值, 特征名称和类名称,例如' Diabetes -ve ',' Diabetes + ve '
Here we used the lasso_path for feature selection
这里我们使用lasso_path进行特征选择
binned continuous variable into discrete values (discretize_continuous = True) based on “quartile”
根据“ 四分位数 ”将连续变量分为离散值( discretize_continuous = True )
Select mode as classification
选择模式作为分类
import limeimport lime.lime_tabularexplainer = lime.lime_tabular.LimeTabularExplainer(X_train.values, feature_names = X_featurenames, class_names = ['Diabetes -ve', 'Diabetes +ve'], feature_selection = "lasso_path", discretize_continuous = True, discretizer = "quartile", verbose = True, mode = 'classification')
For local level explanation let’s pick an observation from test data who is diabetes +ve. Let’s select the 3rd observation (index 254). Here are the first 5 observations from X_test dataset including 3rd observation (index number 254)
对于局部级别的解释,让我们从测试数据中选择一个观察者,即糖尿病+ ve。 让我们选择第三个观察值(索引254)。 这是X_test数据集中的前5个观察值,包括第3个观察值(索引号254)
X_test.iloc[0:5]
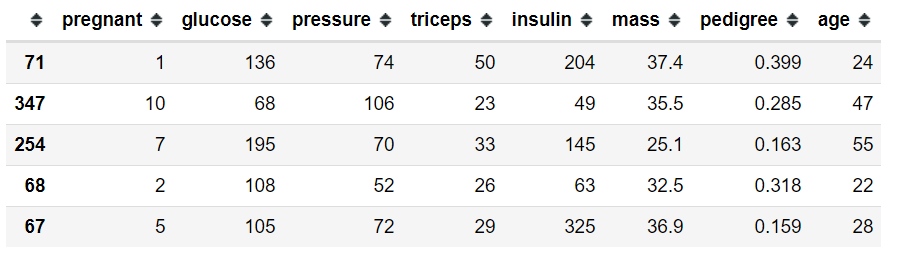
Let’s observe the output variable. You can observe the 3rd observation (index 254) has a value of 1 which indicates it is diabetes +ve.
让我们观察一下输出变量。 您可以观察到第三个观察值(索引254)的值为1,表示糖尿病+ ve。
Y_test.iloc[0:5]

Let’s see whether LIME able to interpret which variables contribute to +ve diabetes and what is the impact magnitude and direction for observation 3 (index number 254)
让我们看看LIME是否能够解释哪些变量导致+ ve糖尿病,以及观察的影响幅度和方向是什么(索引号254)3
解释第一个观察 (Explain the first observation)
For model explanation, one needs to supply the observation and the model predicted probabilities.
为了进行模型解释,需要提供观察值和模型预测的概率。
The output shows the local level LIME model intercept is 0.245 and LIME model prediction is 0.613 (Prediction_local). The original random forest model prediction 0.589. Now, we can plot the explaining variables to show their contribution. In the plot, the right side green bar shows support for +ve diabetes while left side red bars contradicts the support. The variable glucose > 142 shows the highest support for +ve diabetes for the selected observation. In other words for observation 3 in the test dataset having glucose> 142 primarily contributed to +ve diabetes.
输出显示本地级别的LIME模型截距为0.245,而LIME模型的预测值为0.613(Prediction_local)。 原始随机森林模型预测值为0.589。 现在,我们可以绘制解释变量以显示它们的作用。 在该图中,右侧的绿色条表示对+ ve糖尿病的支持,而左侧的红色条与支持相反。 对于选定的观察,可变葡萄糖> 142显示对+ ve糖尿病的最高支持。 换句话说,对于葡萄糖> 142的测试数据集中的观察3,其主要导致+ ve糖尿病。
exp = explainer.explain_instance(X_test.iloc[2], model.predict_proba)exp.as_pyplot_figure()

Similarly, you can plot a detailed explanation using the show_in_notebook( ) function.
同样,您可以使用show_in_notebook()函数绘制详细说明。
exp = explainer.explain_instance(X_test.iloc[2], model.predict_proba)exp.show_in_notebook(show_table = True, show_all = False)

In summary, black-box models nowadays not a black box anymore. There are plenty of algorithms that have been proposed by researchers. Some of them are LIME, Sharp values etc. The above explanation mechanism could be used for all major classification and regression algorithms, even for the deep neural networks.
总而言之,黑匣子模型如今已不再是黑匣子了。 研究人员已经提出了许多算法。 其中一些是LIME,Sharp值等。以上解释机制可用于所有主要的分类和回归算法,甚至适用于深度神经网络。
If you learned something new and liked this article, follow on Twitter, LinkedIn, YouTube or Github.
如果您学到了新知识并喜欢本文,请在Twitter , LinkedIn , YouTube 或 Github上关注 。
Note
注意
This article was first published on onezero.blog, a data science, machine learning and research related blogging platform maintained by me.
本文首次发表于onezero.blog ,数据科学,机器学习和研究相关的博客平台维护由我。
More Interesting Readings — I hope you’ve found this article useful! Below are some interesting readings hope you like them too —
更多有趣的读物 - 希望您对本文有所帮助! 以下是一些有趣的读物,希望您也喜欢 -
翻译自: https://towardsdatascience.com/diabetes-prediction-model-explanation-using-lime-onezeroblog-583d1f509d89
lime 模型
本文来自互联网用户投稿,该文观点仅代表作者本人,不代表本站立场。本站仅提供信息存储空间服务,不拥有所有权,不承担相关法律责任。如若转载,请注明出处:http://www.mzph.cn/news/391100.shtml
如若内容造成侵权/违法违规/事实不符,请联系多彩编程网进行投诉反馈email:809451989@qq.com,一经查实,立即删除!