数据可视化分析票房数据报告
Welcome back to my 100 Days of Data Science Challenge Journey. On day 4 and 5, I work on TMDB Box Office Prediction Dataset available on Kaggle.
欢迎回到我的100天数据科学挑战之旅。 在第4天和第5天,我将研究Kaggle上提供的TMDB票房预测数据集。
I’ll start by importing some useful libraries that we need in this task.
我将从导入此任务中需要的一些有用的库开始。
import pandas as pd# for visualizations
import matplotlib.pyplot as plt
import seaborn as sns
%matplotlib inline
plt.style.use('dark_background')
数据加载与探索 (Data Loading and Exploration)
Once you downloaded data from the Kaggle, you will have 3 files. As this is a prediction competition, you have train, test, and sample_submission file. For this project, my motive is only to perform data analysis and visuals. I am going to ignore test.csv and sample_submission.csv files.
从Kaggle下载数据后,您将拥有3个文件。 由于这是一场预测比赛,因此您具有训练,测试和sample_submission文件。 对于这个项目,我的动机只是执行数据分析和视觉效果。 我将忽略test.csv和sample_submission.csv文件。
Let’s load train.csv in data frame using pandas.
让我们使用熊猫在数据框中加载train.csv。
%time train = pd.read_csv('./data/tmdb-box-office-prediction/train.csv')# output
CPU times: user 258 ms, sys: 132 ms, total: 389 ms
Wall time: 403 ms
关于数据集: (About the dataset:)
id: Integer unique id of each moviebelongs_to_collection: Contains the TMDB Id, Name, Movie Poster, and Backdrop URL of a movie in JSON format.budget: Budget of a movie in dollars. Some row contains 0 values, which mean unknown.genres: Contains all the Genres Name & TMDB Id in JSON Format.homepage: Contains the official URL of a movie.imdb_id: IMDB id of a movie (string).original_language: Two-digit code of the original language, in which the movie was made.original_title: The original title of a movie in original_language.overview: Brief description of the movie.popularity: Popularity of the movie.poster_path: Poster path of a movie. You can see full poster image by adding URL after this link → https://image.tmdb.org/t/p/original/production_companies: All production company name and TMDB id in JSON format of a movie.production_countries: Two-digit code and the full name of the production company in JSON format.release_date: The release date of a movie in mm/dd/yy format.runtime: Total runtime of a movie in minutes (Integer).spoken_languages: Two-digit code and the full name of the spoken language.status: Is the movie released or rumored?tagline: Tagline of a movietitle: English title of a movieKeywords: TMDB Id and name of all the keywords in JSON format.cast: All cast TMDB id, name, character name, gender (1 = Female, 2 = Male) in JSON formatcrew: Name, TMDB id, profile path of various kind of crew members job like Director, Writer, Art, Sound, etc.revenue: Total revenue earned by a movie in dollars.
Let’s have a look at the sample data.
让我们看一下样本数据。
train.head()
As we can see that some features have dictionaries, hence I am dropping all such columns for now.
如我们所见,某些功能具有字典,因此我暂时删除所有此类列。
train = train.drop(['belongs_to_collection', 'genres', 'crew',
'cast', 'Keywords', 'spoken_languages', 'production_companies', 'production_countries', 'tagline','overview','homepage'], axis=1)
Now it time to have a look at statistics of the data.
现在该看一下数据统计了。
print("Shape of data is ")
train.shape# OutputShape of data is
(3000, 12)
Dataframe information.
数据框信息。
train.info()# Output
<class 'pandas.core.frame.DataFrame'>
RangeIndex: 3000 entries, 0 to 2999
Data columns (total 12 columns):
# Column Non-Null Count Dtype
--- ------ -------------- -----
0 id 3000 non-null int64
1 budget 3000 non-null int64
2 imdb_id 3000 non-null object
3 original_language 3000 non-null object
4 original_title 3000 non-null object
5 popularity 3000 non-null float64
6 poster_path 2999 non-null object
7 release_date 3000 non-null object
8 runtime 2998 non-null float64
9 status 3000 non-null object
10 title 3000 non-null object
11 revenue 3000 non-null int64
dtypes: float64(2), int64(3), object(7)
memory usage: 281.4+ KB
Describe dataframe.
描述数据框。
train.describe()
Let’s create new columns for release weekday, date, month, and year.
让我们为发布工作日,日期,月份和年份创建新列。
train['release_date'] = pd.to_datetime(train['release_date'], infer_datetime_format=True)train['release_day'] = train['release_date'].apply(lambda t: t.day)train['release_weekday'] = train['release_date'].apply(lambda t: t.weekday())train['release_month'] = train['release_date'].apply(lambda t: t.month)
train['release_year'] = train['release_date'].apply(lambda t: t.year if t.year < 2018 else t.year -100)
数据分析与可视化 (Data Analysis and Visualization)
问题1:哪部电影的收入最高? (Question 1: Which movie made the highest revenue?)
train[train['revenue'] == train['revenue'].max()]
train[['id','title','budget','revenue']].sort_values(['revenue'], ascending=False).head(10).style.background_gradient(subset='revenue', cmap='BuGn')# Please note that output has a gradient style, but in a medium, it is not possible to show.
The Avengers movie has made the highest revenue.
复仇者联盟电影的收入最高。
问题2:哪部电影的预算最高? (Question 2 : Which movie has the highest budget?)
train[train['budget'] == train['budget'].max()]
train[['id','title','budget', 'revenue']].sort_values(['budget'], ascending=False).head(10).style.background_gradient(subset=['budget', 'revenue'], cmap='PuBu')
Pirates of the Caribbean: On Stranger Tides is most expensive movie.
加勒比海盗:惊涛怪浪是最昂贵的电影。
问题3:哪部电影是最长的电影? (Question 3: Which movie is longest movie?)
train[train['runtime'] == train['runtime'].max()]
plt.hist(train['runtime'].fillna(0) / 60, bins=40);
plt.title('Distribution of length of film in hours', fontsize=16, color='white');
plt.xlabel('Duration of Movie in Hours')
plt.ylabel('Number of Movies')

train[['id','title','runtime', 'budget', 'revenue']].sort_values(['runtime'],ascending=False).head(10).style.background_gradient(subset=['runtime','budget','revenue'], cmap='YlGn')
Carlos is the longest movie, with 338 minutes (5 hours and 38 minutes) of runtime.
卡洛斯(Carlos)是最长的电影,有338分钟(5小时38分钟)的运行时间。
问题4:大多数电影在哪一年发行的? (Question 4: In which year most movies were released?)
plt.figure(figsize=(20,12))
edgecolor=(0,0,0),
sns.countplot(train['release_year'].sort_values(), palette = "Dark2", edgecolor=(0,0,0))
plt.title("Movie Release count by Year",fontsize=20)
plt.xlabel('Release Year')
plt.ylabel('Number of Movies Release')
plt.xticks(fontsize=12,rotation=90)
plt.show()
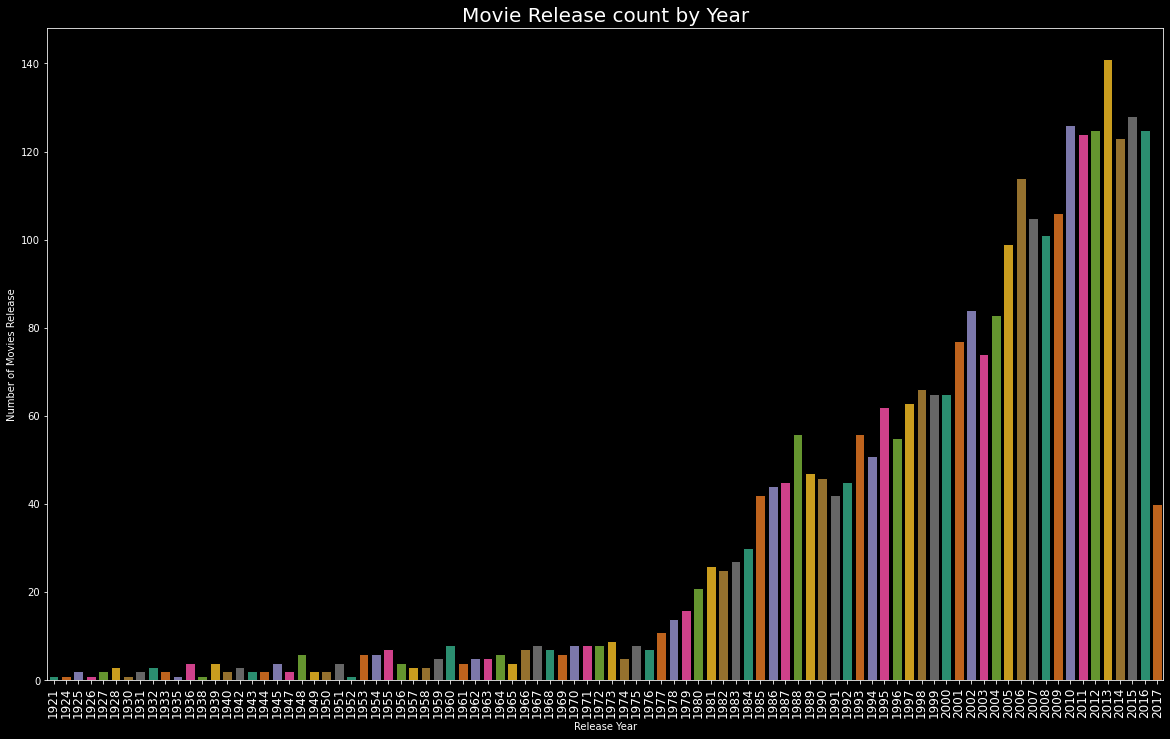
train['release_year'].value_counts().head()# Output2013 141
2015 128
2010 126
2016 125
2012 125
Name: release_year, dtype: int64
In 2013 total 141 movies were released.
2013年,总共发行了141部电影。
问题5:最受欢迎和最低人气的电影。 (Question 5 : Movies with Highest and Lowest popularity.)
Most popular Movie:
最受欢迎的电影:
train[train['popularity']==train['popularity'].max()][['original_title','popularity','release_date','revenue']]
Least Popular Movie:
最不受欢迎的电影:
train[train['popularity']==train['popularity'].min()][['original_title','popularity','release_date','revenue']]
Lets create popularity distribution plot.
让我们创建人气分布图。
plt.figure(figsize=(20,12))
edgecolor=(0,0,0),
sns.distplot(train['popularity'], kde=False)
plt.title("Movie Popularity Count",fontsize=20)
plt.xlabel('Popularity')
plt.ylabel('Count')
plt.xticks(fontsize=12,rotation=90)
plt.show()

Wonder Woman movie have highest popularity of 294.33 whereas Big Time movie have lowest popularity which is 0.
《神奇女侠》电影的最高人气为294.33,而《大时代》电影的最低人气为0。
问题6:从1921年到2017年,大多数电影在哪个月发行? (Question 6 : In which month most movies are released from 1921 to 2017?)
plt.figure(figsize=(20,12))
edgecolor=(0,0,0),
sns.countplot(train['release_month'].sort_values(), palette = "Dark2", edgecolor=(0,0,0))
plt.title("Movie Release count by Month",fontsize=20)
plt.xlabel('Release Month')
plt.ylabel('Number of Movies Release')
plt.xticks(fontsize=12)
plt.show()

train['release_month'].value_counts()# Output
9 362
10 307
12 263
8 256
4 245
3 238
6 237
2 226
5 224
11 221
1 212
7 209
Name: release_month, dtype: int64
In september month most movies are relesed which is around 362.
在9月中,大多数电影都已发行,大约362。
问题7:大多数电影在哪个月上映? (Question 7 : On which date of month most movies are released?)
plt.figure(figsize=(20,12))
edgecolor=(0,0,0),
sns.countplot(train['release_day'].sort_values(), palette = "Dark2", edgecolor=(0,0,0))
plt.title("Movie Release count by Day of Month",fontsize=20)
plt.xlabel('Release Day')
plt.ylabel('Number of Movies Release')
plt.xticks(fontsize=12)
plt.show()

train['release_day'].value_counts().head()#Output
1 152
15 126
12 122
7 110
6 107
Name: release_day, dtype: int64
首次发布影片的最高数量为152。 (On first date highest number of movies are released, 152.)
问题8:大多数电影在一周的哪一天发行? (Question 8 : On which day of week most movies are released?)
plt.figure(figsize=(20,12))
sns.countplot(train['release_weekday'].sort_values(), palette='Dark2')
loc = np.array(range(len(train['release_weekday'].unique())))
day_labels = ['Mon', 'Tue', 'Wed', 'Thu', 'Fri', 'Sat', 'Sun']
plt.xlabel('Release Day of Week')
plt.ylabel('Number of Movies Release')
plt.xticks(loc, day_labels, fontsize=12)
plt.show()

train['release_weekday'].value_counts()# Output
4 1334
3 609
2 449
1 196
5 158
0 135
6 119
Name: release_weekday, dtype: int64
星期五上映的电影数量最多。 (Highest number of movies released on friday.)
最后的话 (Final Words)
I hope this article was helpful to you. I tried to answer a few questions using data science. There are many more questions to ask. Now, I will move towards another dataset tomorrow. All the codes of data analysis and visuals can be found at this GitHub repository or Kaggle kernel.
希望本文对您有所帮助。 我尝试使用数据科学回答一些问题。 还有更多问题要问。 现在,我明天将移至另一个数据集。 可以在此GitHub存储库或Kaggle内核中找到所有数据分析和可视化代码。
Thanks for reading.
谢谢阅读。
I appreciate any feedback.
我感谢任何反馈。
If you like my work and want to support me, I’d greatly appreciate it if you follow me on my social media channels:
如果您喜欢我的工作并希望支持我,那么如果您在我的社交媒体频道上关注我,我将不胜感激:
The best way to support me is by following me on Medium.
支持我的最佳方法是在Medium上关注我。
Subscribe to my new YouTube channel.
订阅我的新YouTube频道 。
Sign up on my email list.
在我的电子邮件列表中注册。
翻译自: https://towardsdatascience.com/box-office-revenue-analysis-and-visualization-ce5b81a636d7
数据可视化分析票房数据报告
本文来自互联网用户投稿,该文观点仅代表作者本人,不代表本站立场。本站仅提供信息存储空间服务,不拥有所有权,不承担相关法律责任。如若转载,请注明出处:http://www.mzph.cn/news/390897.shtml
如若内容造成侵权/违法违规/事实不符,请联系多彩编程网进行投诉反馈email:809451989@qq.com,一经查实,立即删除!