搜索引擎优化学习原理
Search Engine Optimisation (SEO) is the discipline of using knowledge gained around how search engines work to build websites and publish content that can be found on search engines by the right people at the right time.
搜索引擎优化(SEO)是一门学科,它使用有关搜索引擎如何工作的知识来构建网站和发布内容,这些内容可以由合适的人在正确的时间在搜索引擎上找到。
Some people say that you don’t really need SEO and they take a Field of Dreams ‘build it and they shall come’ approach. The size of the SEO industry is predicted to be $80 billion by the end of 2020. There are at least some people who like to hedge their bets.
有人说您真的不需要SEO,而他们却选择了“梦想之场 ”来构建它,然后他们就会来。 到2020年底,SEO行业的规模预计将达到800亿美元。至少有些人喜欢对冲自己的赌注。
An often-quoted statistic is that Google’s ranking algorithm contains more than 200 factors for ranking web pages and SEO is often seen as an ‘arms race’ between its practitioners and the search engines. With people looking for the next ‘big thing’ and putting themselves into tribes (white hat, black hat and grey hat).
经常被引用的统计数据是Google的排名算法包含200多个用于对网页进行排名的因素 ,而SEO通常被视为其从业者与搜索引擎之间的“军备竞赛”。 人们正在寻找下一个“大事情”,并将自己纳入部落( 白帽子 , 黑帽子和灰帽子 )。
There is a huge amount of data generated by SEO activity and its plethora of tools. For context, the industry-standard crawling tool Screaming Frog has 26 different reports filled with web page metrics on things you wouldn’t even think are important (but are). That is a lot of data to munge and find interesting insights from.
SEO活动及其大量工具生成了大量数据。 就上下文而言,行业标准的爬网工具Screaming Frog有26种不同的报告,其中包含关于您甚至不认为很重要(但很重要)的内容的网页指标。 需要大量的数据来进行整理并从中找到有趣的见解。
The SEO mindset also lends itself well to the data science ideal of munging data and using statistics and algorithms to derive insights and tell stories. SEO practitioners have been pouring over all of this data for 2 decades trying to figure out the next best thing to do and to demonstrate value to clients.
SEO的思维方式也非常适合数据科学的理想,即处理数据并使用统计数据和算法来获得见解和讲故事。 SEO从业人员已经倾注了所有这些数据长达20年之久,试图找出下一步要做的事情,并向客户展示价值。
Despite access to all of this data, there is still a lot of guesswork in SEO and while some people and agencies test different ideas to see what performs well, a lot of the time it comes down to the opinion of the person with the best track record and overall experience on the team.
尽管可以访问所有这些数据,但SEO仍然存在很多猜测,尽管有些人和机构测试不同的想法以查看效果良好,但很多时候却取决于最佳跟踪者的意见。记录和团队的整体经验。
I’ve found myself in this position a lot in my career and this is something I would like to address now that I have acquired some data science skills of my own. In this article, I will point you to some resources that will allow you to take more data-led approach to your SEO efforts.
在我的职业生涯中,我经常担任这个职位,这是我现在要解决的问题,因为我已经掌握了一些数据科学技能。 在本文中,我将为您指出一些资源,这些资源将使您可以采用更多以数据为主导的方法来进行SEO。
SEO测试 (SEO Testing)
One of the most often asked questions in SEO is ‘We’ve implemented these changes on a client’s webaite, but did they have an effect?’. This often leads to the idea that if the website traffic went up ‘it worked’ and if the traffic went down it was ‘seasonality’. That is hardly a rigorous approach.
SEO中最常被问到的问题之一是“我们已经在客户的Webaite上实施了这些更改,但是它们有效果吗?”。 这通常导致这样的想法:如果网站流量上升,则“正常”,如果流量下降,则为“季节性”。 那不是严格的方法。
A better approach is to put some maths and statistics behind it and analyse it with a data science approach. A lot of the maths and statistics behind data science concepts can be difficult, but luckily there are a lot of tools out there that can help and I would like to introduce one that was made by Google called Causal Impact.
更好的方法是将一些数学和统计信息放在后面,并使用数据科学方法进行分析。 数据科学概念背后的许多数学和统计数据可能很困难,但是幸运的是,那里有很多工具可以提供帮助,我想介绍一下由Google制造的名为因果影响的工具 。
The Causal Impact package was originally an R package, however, there is a Python version if that is your poison and that is what I will be going through in this post. To install it in your Python environment using Pipenv, use the command:
因果影响包最初是R包 ,但是,如果有毒,那就有一个Python版本 ,这就是我将在本文中介绍的内容。 要使用Pipenv在Python环境中安装它,请使用以下命令:
pipenv install pycausalimpact
If you want to learn more about Pipenv, see a post I wrote on it here, otherwise, Pip will work just fine too:
如果您想了解有关Pipenv的更多信息,请参阅我在此处写的一篇文章,否则,Pip也可以正常工作:
pip install pycausalimpact
什么是因果影响? (What is Causal Impact?)
Causal Impact is a library that is used to make predictions on time-series data (such as web traffic) in the event of an ‘intervention’ which can be something like campaign activity, a new product launch or an SEO optimisation that has been put in place.
因果影响是一个库,用于在发生“干预”时对时间序列数据(例如网络流量)进行预测,该干预可以是诸如活动活动,新产品发布或已经进行的SEO优化之类的事情。到位。
You supply two-time series as data to the tool, one time series could be clicks over time for the part of a website that experienced the intervention. The other time series acts as a control and in this example that would be clicks over time for a part of the website that didn’t experience the intervention.
您向工具提供了两个时间序列作为数据,一个时间序列可能是随着时间的流逝而发生的涉及网站干预的部分。 其他时间序列用作控制,在此示例中,将是一段时间内未经历干预的网站的点击次数。
You also supply a data to the tool when the intervention took place and what it does is it trains a model on the data called a Bayesian structural time series model. This model uses the control group as a baseline to try and build a prediction about what the intervention group would have looked like if the intervention hadn’t taken place.
您还可以在发生干预时向工具提供数据,它所做的是在数据上训练一个称为贝叶斯结构时间序列模型的模型 。 该模型以对照组为基准,以尝试建立关于如果未进行干预的情况下干预组的状况的预测。
The original paper on the maths behind it is here, however, I recommend watching this video below by a guy at Google, which is far more accessible:
关于它背后的数学原理的原始文章在这里 ,但是,我建议下面由Google的一个人观看此视频,该视频更容易获得:
在Python中实现因果影响 (Implementing Causal Impact in Python)
After installing the library into your environment as outlined above, using Causal Impact with Python is pretty straightforward, as can be seen in the notebook below by Paul Shapiro:
在如上所述将库安装到您的环境中之后,将因果影响与Python结合使用非常简单,如Paul Shapiro在下面的笔记本中所示:
After pulling in a CSV with the control group data, intervention group data and defining the pre/post periods you can train the model by calling:
在输入包含控制组数据,干预组数据的CSV并定义前后期间后,您可以通过调用以下方法来训练模型:
ci = CausalImpact(data[data.columns[1:3]], pre_period, post_period)
This will train the model and run the predictions. If you run the command:
这将训练模型并运行预测。 如果运行命令:
ci.plot()
You will get a chart that looks like this:
您将获得一个如下所示的图表:
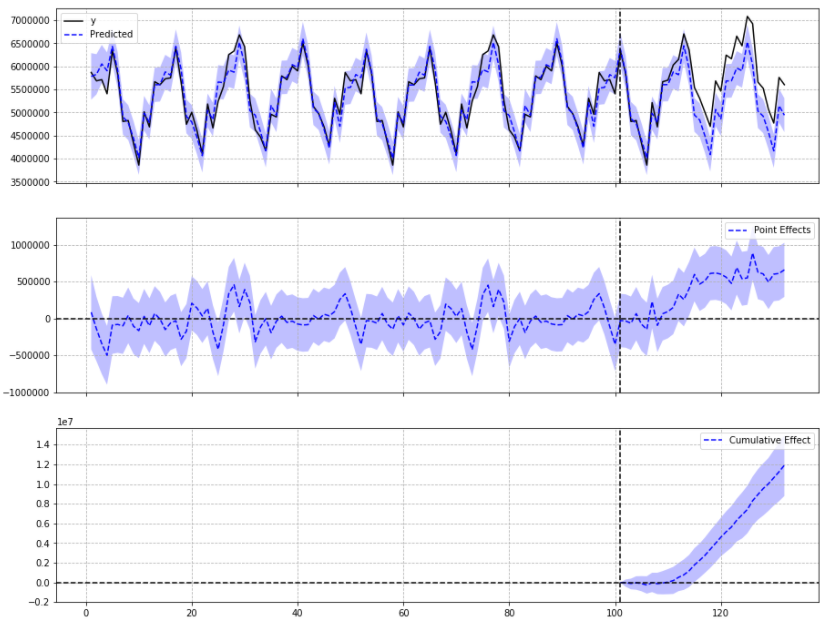
You have three panels here, the first panel showing the intervention group and the prediction of what would have happened without the intervention.
您在此处有三个面板,第一个面板显示干预组,并预测没有干预的情况。
The second panel shows the pointwise effect, which means the difference between what happened and the prediction made by the model.
第二个面板显示了逐点效应,这意味着发生的事情与模型所做的预测之间的差异。
The final panel shows the cumulative effect of the intervention as predicted by the model.
最后一个面板显示了模型所预测的干预措施的累积效果。
Another useful command to know is:
另一个有用的命令是:
print(ci.summary('report'))
This prints out a full report that is human readable and ideal for summarising and dropping into client slides:
这将打印出一份完整的报告,该报告易于阅读,是汇总和放入客户端幻灯片的理想选择:
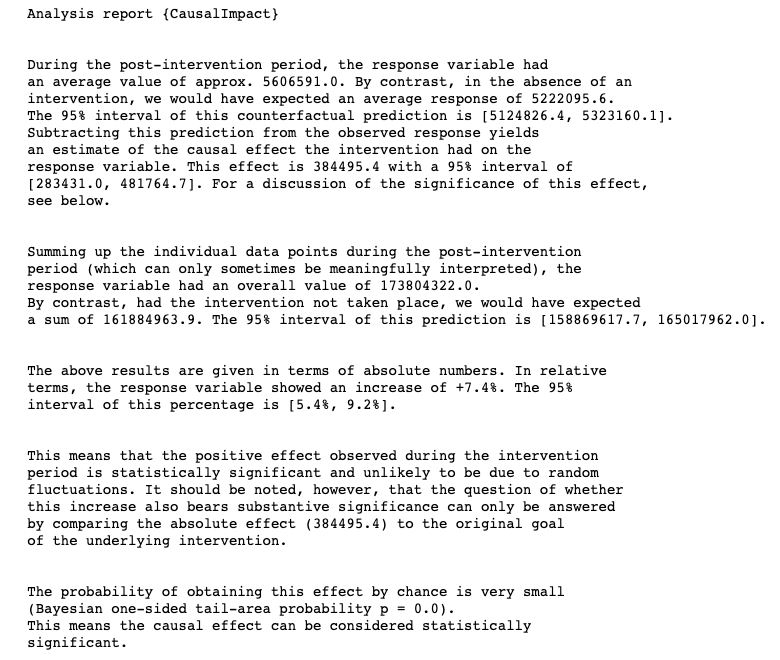
选择一个对照组 (Selecting a control group)
The best way to build your control group is to pick pages which aren’t affected by the intervention at random using a method called stratified random sampling.
建立对照组的最佳方式是使用一种称为分层随机抽样的方法随机选择不受干预影响的页面。
Etsy has done a post on how they’ve used Causal Impact for SEO split testing and they recommend using this method. Random stratified sampling is as the name implies where you pick from the population at random to build the sample. However if what we’re sampling is segmented in some way, we try and maintain the same proportions in the sample as in the population for these segments:
Etsy发表了一篇关于他们如何将因果影响用于SEO拆分测试的文章,他们建议使用此方法。 顾名思义,随机分层抽样是您从总体中随机选择以构建样本的地方。 但是,如果以某种方式对样本进行了细分,则我们将尝试在样本中保持与这些细分中的总体相同的比例:
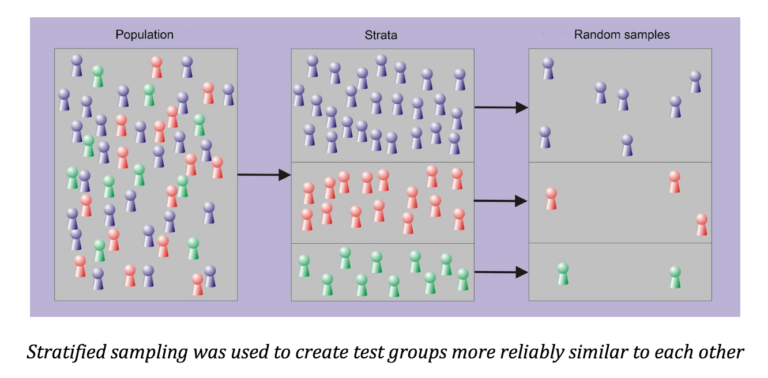
An ideal way to segment web pages for stratified sampling is to use sessions as a metric. If you load your page data into Pandas as a data frame, you can use a lambda function to label each page:
细分网页以进行分层抽样的理想方法是使用会话作为指标。 如果将页面数据作为数据框加载到Pandas中,则可以使用lambda函数标记每个页面:
df["label"] =
df["Sessions"].apply(lambda
x:"Less than 50"
if
x<=50
else
("Less than 100"
if
x<=100
else
("Less than 500"
if
x<=500
else
("Less than 1000"
if
x<=1000
else
("Less than 5000"
if
x<=5000
else
"Greater than 5000")))))
df["label"] =
df["Sessions"].apply(lambda
x:"Less than 50"
if
x<=50
else
("Less than 100"
if
x<=100
else
("Less than 500"
if
x<=500
else
("Less than 1000"
if
x<=1000
else
("Less than 5000"
if
x<=5000
else
"Greater than 5000")))))
From there, you can use test_train_split in sklearn to build your control and test groups:
从那里,您可以在sklearn中使用test_train_split来构建您的控制和测试组:
from
sklearn.model_selection import
train_test_split
from
sklearn.model_selection import
train_test_split
X_train, X_test, y_train, y_test =
train_test_split(selectedPages["URL"],selectedPages["label"], test_size=0.01, stratify=selectedPages["label"])
X_train, X_test, y_train, y_test =
train_test_split(selectedPages["URL"],selectedPages["label"], test_size=0.01, stratify=selectedPages["label"])
Note that stratify is set and if you have a list of pages you want to test already then your sample pages should equal the number of pages you want to test. Also, the more pages you have in your sample, the better the model will be. If you use too few pages, the less accurate the model will be.
请注意,已设置分层 ,并且如果您已经有要测试的页面列表,则示例页面应等于要测试的页面数。 另外,样本中的页面越多,模型越好。 如果使用的页面太少,则模型的准确性将降低。
It is is worth noting that JC Chouinard gives a good background on how to do all of this in Python using a method similar to Etsy:
值得注意的是,JC Chouinard为如何使用类似于Etsy的方法在Python中完成所有这些操作提供了良好的背景知识:
结论 (Conclusion)
There are a couple of different use cases that you could use this type of testing. The first would be to test ongoing improvements using split testing and this is similar to the approach that Etsy uses above.
您可以使用几种类型的测试来使用这种类型的测试。 首先是使用拆分测试来测试正在进行的改进,这与Etsy上面使用的方法类似。
The second would be to test an improvement that was made on-site as part of ongoing work. This is similar to an approach outlined in this post, however with this approach you need to ensure your sample size is sufficiently large otherwise your predictions will be very inaccurate. So please do bear that in mind.
第二个是测试正在进行的工作中在现场进行的改进。 这类似于在此列出的方法后 ,但是这种方法,你需要确保你的样本规模足够大,否则你的预测将是非常不准确的。 因此,请记住这一点。
Both ways are valid ways of doing SEO testing, with the former being a type of A/B split test for ongoing optimisation and the latter being an test for something that has already been implemented.
两种方法都是进行SEO测试的有效方法,前一种是用于进行持续优化的A / B拆分测试,而后一种是针对已经实施的测试。
I hope this has given you some insight into how to apply data science principles to your SEO efforts. Do read around these interesting topics and try and come up with other ways to use this library to validate your efforts. If you need background on the Python used in this post I recommend this course.
我希望这使您对如何将数据科学原理应用于SEO有所了解。 请阅读这些有趣的主题,并尝试使用其他方法来使用此库来验证您的工作。 如果您需要本文中使用的Python的背景知识,我建议您学习本课程 。
翻译自: https://towardsdatascience.com/how-to-use-data-science-principles-to-improve-your-search-engine-optimisation-efforts-927712ed0b12
搜索引擎优化学习原理
本文来自互联网用户投稿,该文观点仅代表作者本人,不代表本站立场。本站仅提供信息存储空间服务,不拥有所有权,不承担相关法律责任。如若转载,请注明出处:http://www.mzph.cn/news/389260.shtml
如若内容造成侵权/违法违规/事实不符,请联系多彩编程网进行投诉反馈email:809451989@qq.com,一经查实,立即删除!