spotify 数据分析
For many data science students, collecting data is seen as a solved problem. It’s just there in Kaggle or UCI. However, that’s not how data is available daily for working Data Scientists. Also, many of the datasets used for learning have been largely explored, so how innovative would be building a portfolio based on them? What about building your dataset by combining different sources?
对于许多数据科学专业的学生来说,收集数据被视为已解决的问题。 它就在Kaggle或UCI中。 但是,这不是每天为工作的数据科学家提供数据的方式。 此外,已经广泛探索了许多用于学习的数据集,因此如何基于这些数据集构建投资组合? 如何通过组合不同的来源来构建数据集?
Let’s dive in.
让我们潜入。
为什么选择重金属数据? (Why Heavy Metal data?)
Seen by many as a very strict music genre (screaming vocals, fast drums, distorted guitars), it actually goes the other way round. Metal music it’s not as mainstream as most genres but it has, without question, the largest umbrella of subgenres with so many distinct sounds. Therefore, seeing its differences through data could be a good idea, even for the listener not familiarized with it.
被许多人视为非常严格的音乐类型(尖叫的人声,快速的鼓声,失真的吉他),但实际上却相反。 金属音乐不像大多数流派那样流行,但是毫无疑问,它拥有最大的亚流派,并具有如此多的独特声音。 因此,即使对于听众不熟悉的数据,通过数据查看其差异也是一个好主意。
为什么选择维基百科? 怎么样? (Why Wikipedia? How?)
Wikipedia is frequently updated and it presents relevant information on almost every topic. Also, it gathers many useful links in every page. With heavy metal, it would be no different, especially listing the most popular subgenres and their most relevant bands.
维基百科经常更新,并提供几乎每个主题的相关信息。 而且,它在每个页面中收集许多有用的链接。 对于重金属,这没什么两样,特别是列出了最受欢迎的子类型及其最相关的乐队。
The following page presents not only a historic perspective of the style, but also hyperlinks for many other subgenres and fusion genres in the bottom. The idea here is to collect metal genres only by this starting point.
下一页不仅显示了样式的历史观点,还显示了底部许多其他子类型和融合类型的超链接。 这里的想法是仅在此起点上收集金属类型。
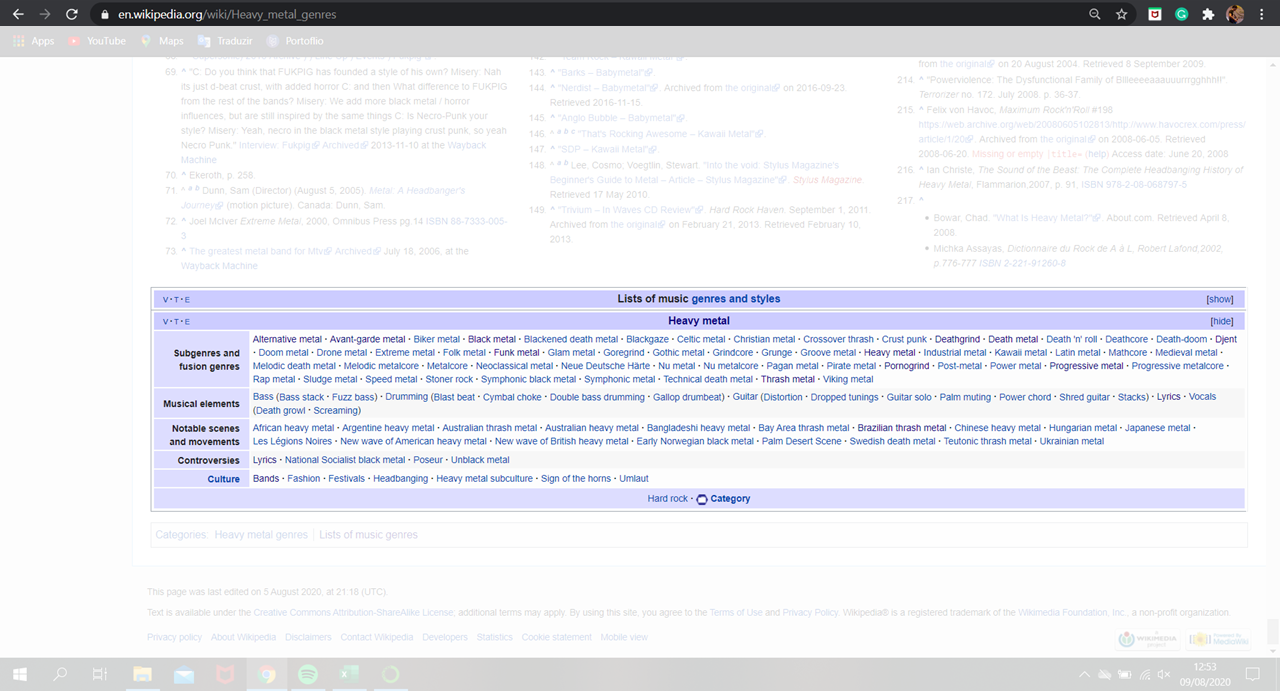
Therefore, the genres’ names in the table were scrapped and compiled in a Python list.
因此,表格中的流派名称被废弃并编译到Python列表中。
from bs4 import BeautifulSoup
import requests
import pandas as pd
import numpy as np
import re# Getting URLssource = 'https://en.wikipedia.org/wiki/Heavy_metal_genres'
response = requests.get(source)
soup = BeautifulSoup(response.text, 'html.parser')
pages = soup.find(class_='navbox-list navbox-odd')
pages = pages.findAll('a')links = []for page in pages:
links.append(('List_of_' + page.get('title').lower().replace(' ','_') + '_bands').replace('_music',''))
After inspecting some of the genres’ pages, we discovered that the most relevant ones have pages listing their most important bands. The pages’ URLs can be presented in one or more of the following patterns:
在检查了某些类型的页面后,我们发现最相关的页面中列出了其最重要的乐队。 页面的URL可以以下一种或多种模式显示:
“https://en.wikipedia.org/wiki/List_of_” + genre + “_bands”
“ https://en.wikipedia.org/wiki/List_of_” +类型+“ _bands”
“https://en.wikipedia.org/wiki/List_of_” + genre + “_bands,_0–K”
“ https://zh.wikipedia.org/wiki/List_of_” +类型+“ _ bands,_0–K”
“https://en.wikipedia.org/wiki/List_of_” + genre + “_bands,_L–Z”
“ https://zh.wikipedia.org/wiki/List_of_” +类型+“ _bands,_L–Z”
After inspecting the links, we were able to detect that the band names were presented in varying forms according to each page. Some were tables, some alphabetical lists and some in both. Each presentation form required a different scrapping approach.
在检查了链接之后,我们能够检测到根据每个页面以不同形式显示的乐队名称。 有些是表格,有些是字母顺序的列表,而两者都有。 每个演示文稿表格都需要不同的报废方法。
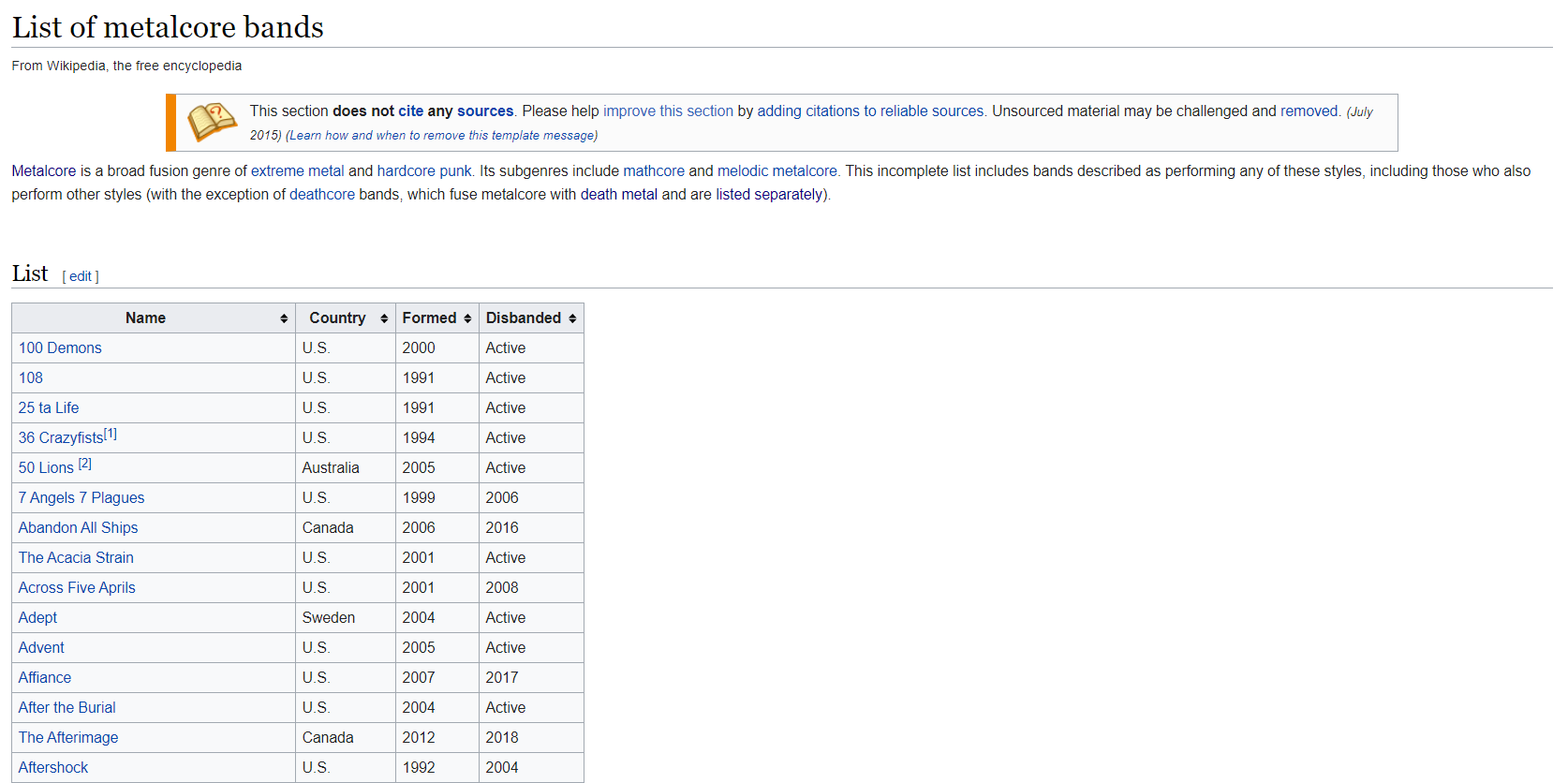
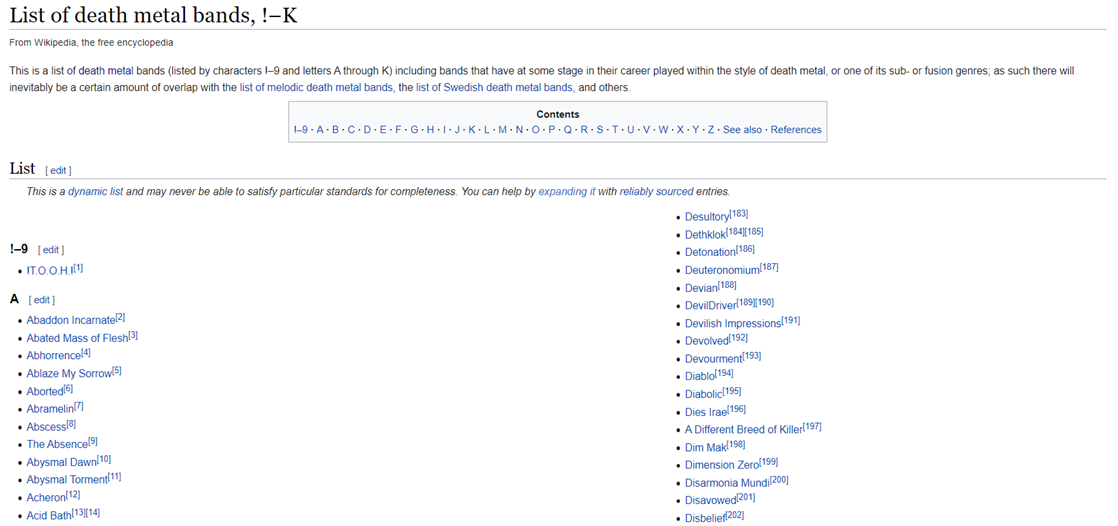
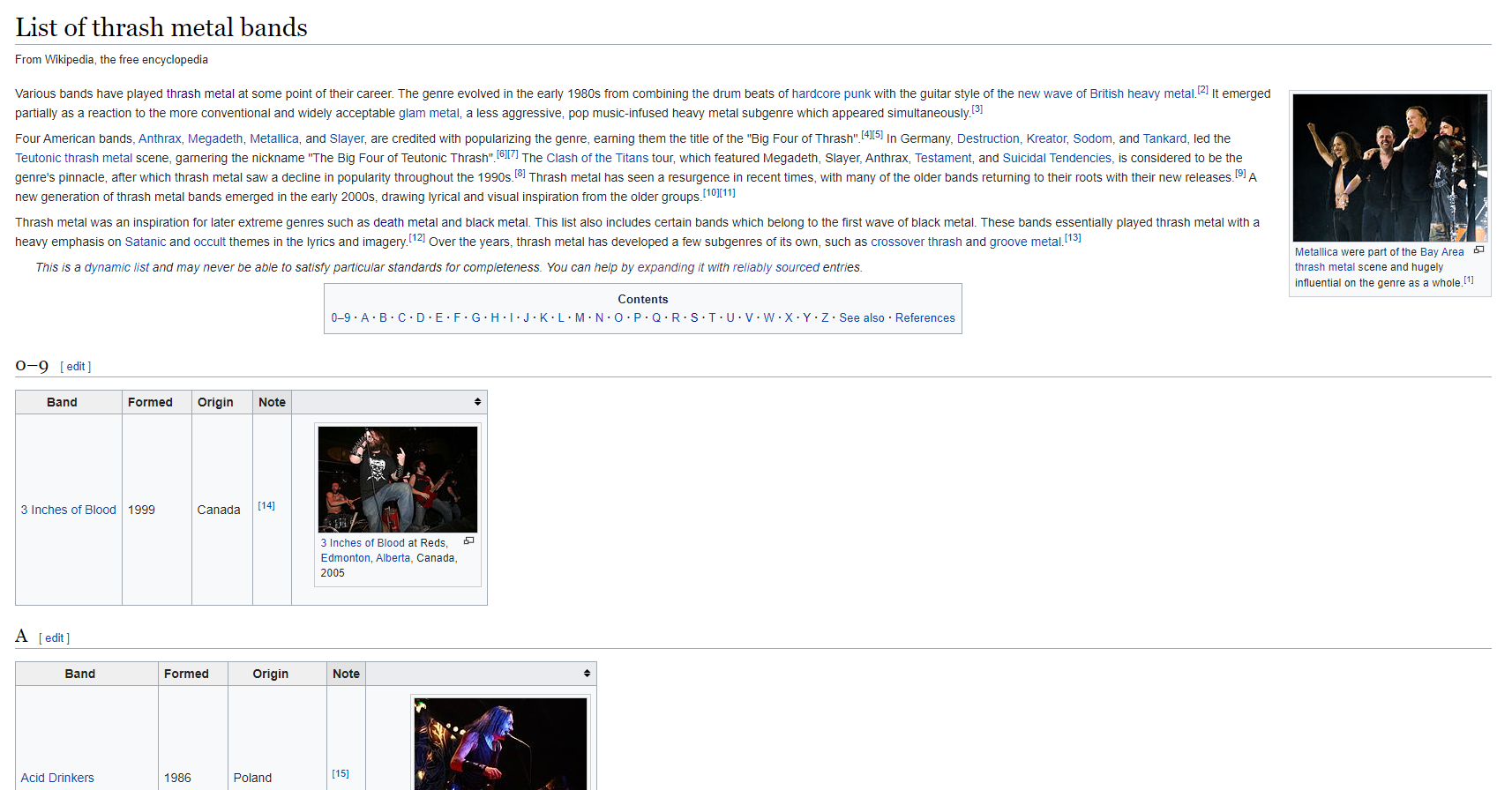
Some band names were polluted with additional characters (mostly for notes or referencing), so a function was developed to deal with these issues.
一些乐队名称被附加字符污染(大多数用于注释或参考),因此开发了一个功能来处理这些问题。
def string_ajustment(band):
"""Ajustment of the retrieved band name string"""
end = band.find('[') # Remove brackets from band name
if end > -1:
band = band[:end]
else:
band = band
end = band.find('(') # Remove parentesis from band name
if end > -1:
band = band[:end]
band = band.title().rstrip() # Uppercase in first letters; last space removal
return band
The scrapping code gathered the data which was later compiled into a Pandas dataframe object.
剪贴代码收集了数据,这些数据随后被编译为Pandas数据框对象。
%%timedata = []
genres = []for link in links:
url = 'https://en.wikipedia.org/wiki/' + link
genre = url[url.rfind('/') + 1:]
list_from = ['List_of_', '_bands', ',_!–K', ',_L–Z', '_']
list_to = ['', '', '', '', ' ']
for idx, element in enumerate(list_from):
genre = genre.replace(list_from[idx], list_to[idx])
genre = genre.title()
response = requests.get(url)
soup = BeautifulSoup(response.text, 'html.parser')
# Table detection
tables = []
tables = soup.find_all('table', {'class':'wikitable'}) # 1st attempt
if len(tables) == 0:
tables = soup.find_all('table', {'class':'wikitable sortable'}) # 2nd attempt
# Getting table data
if len(tables) > 0: # pages with tables
genres.append(genre)
for table in tables:
table = table.tbody
rows = table.find_all('tr')
columns = [v.text.replace('\n', '') for v in rows[0].find_all('th')]for i in range(1, len(rows)):
tds = rows[i].find_all('td')
band = tds[0].text.replace('\n', '')
band = string_ajustment(band)
values = [band, genre]
data.append(pd.Series(values)) # Append band
else:
# Getting data in lists
groups = soup.find_all('div', class_ = 'div-col columns column-width') # Groups being lists of bands, 1st attempt
if len(groups) == 0:
groups = soup.find_all('table', {'class':'multicol'}) # Groups being lists of bands, 2nd attempt
for group in groups:
genres.append(genre)
array = group.text.split('\n')[1:len(group.text.split('\n'))-1]
for band in array:
if (band != '0-9'):
band = string_ajustment(band)
if (band.find('Reference') > -1) or (band.find('See also') > -1): # Remove text without band name
break
elif len(band) > 1:
values = [band, genre]
data.append(pd.Series(values)) # Append band
if genre not in genres: # Two possibilities: either data in multiple urls or no data available (non-relevant genre)
additional_links = [link + ',_!–K', link + ',_L–Z']
for additional_link in additional_links:
url = 'https://en.wikipedia.org/wiki/' + additional_link
response = requests.get(url)
soup = BeautifulSoup(response.text, 'html.parser')
groups = soup.find_all('table', {'class':'multicol'}) # Groups being lists of bands
for group in groups:
genres.append(genre)
array = group.text.split('\n')[1:len(group.text.split('\n'))-1]
for band in array:
if (band != '0-9'):
band = string_ajustment(band)
if (band.find('Reference') > -1) or (band.find('See also') > -1): # Remove text without band name
break
elif len(band) > 1:
values = [band, genre]
data.append(pd.Series(values)) # Append band
Creating the Pandas dataframe object:
创建熊猫数据框对象:
df_bands = pd.DataFrame(data)
df_bands.columns = ['Band', 'Genre']
df_bands.drop_duplicates(inplace=True)df_bands
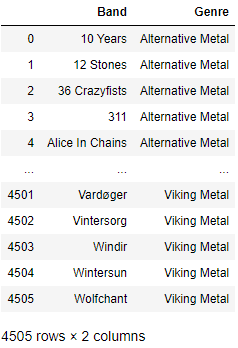
Adding a label to anything is hard and with music genres, it wouldn’t be different. Some bands played different styles through the years and other bands made crossovers between varying musical elements. If the band is listed in multiple Wikipedia pages, our Pandas dataframe presents it multiple times, each time with a different label/genre.
在任何事物上加上标签都是很困难的,而且对于音乐流派来说,也没有什么不同。 多年来,有些乐队演奏风格各异,另一些乐队则在不同的音乐元素之间进行了转换。 如果乐队在多个Wikipedia页面上列出,则我们的Pandas数据框会多次显示该乐队,每次带有不同的标签/流派。
You might be asking how to deal with it.
您可能会问如何处理它 。
Well, it depends on your intentions with the data.
好吧,这取决于您对数据的意图 。
If the intention is to create a genre classifier given the songs’ attributes, the most relevant label could be kept. This information could be found scrapping the number of Google Search results of the band name and the genre, for example. The one with most results should be kept. If the intention is to develop a multi output-multiclass classifier, there is no need to drop the labels.
如果打算根据歌曲的属性创建流派分类器 ,则可以保留最相关的标签。 例如,可以找到这些信息,删除了乐队名称和流派的Google搜索结果数量。 结果最多的一个应该保留。 如果要开发多输出多类分类器 ,则无需删除标签。
为什么选择Spotify? 怎么样? (Why Spotify? How?)
Differently from Wikipedia, Spotify provides an API for data collection. Based on this video by CodingEntrepreneurs with minor changes, we were able to collect an artists’ albums, tracks and its features. The first thing to access the API is to register your application in Spotify’s developer’s page. You’ll be able to find your client_id and your client_secret after registering.
与Wikipedia不同,Spotify提供了用于数据收集的API。 根据CodingEntrepreneurs的 这段视频进行了微小的更改,我们就可以收集艺术家的专辑,曲目及其功能。 访问API的第一件事是在Spotify开发人员页面上注册您的应用程序。 注册后,您将可以找到client_id和client_secret 。
Before using this approach, I’ve tried using Spotipy. However, the amount of data we were trying to collect was requiring too much token refreshes (also in a non-understandable pattern). Thus, we changed our approach to match CodingEntrepreneurs’, which became much more reliable.
在使用这种方法之前,我尝试使用Spotipy 。 但是,我们试图收集的数据量需要太多的令牌刷新(也是一种不可理解的模式)。 因此,我们更改了方法以匹配CodingEntrepreneurs ,这变得更加可靠。
import base64
import requests
import datetime
from urllib.parse import urlencodeclient_id ='YOUR_CLIENT_ID'
client_secret = 'YOUR_CLIENT_SECRET'class SpotifyAPI(object):
access_token = None
access_token_expires = datetime.datetime.now()
access_token_did_expire = True
client_id = None
client_secret = None
token_url = 'https://accounts.spotify.com/api/token'
def __init__(self, client_id, client_secret, *args, **kwargs):
super().__init__(*args, **kwargs)
self.client_id = client_id
self.client_secret = client_secret
def get_client_credentials(self):
"""
Returns a base64 encoded string
"""
client_id = self.client_id
client_secret = self.client_secret
if (client_id == None) or (client_secret == None):
raise Exception('You must set client_id and client secret')
client_creds = f'{client_id}:{client_secret}'
client_creds_b64 = base64.b64encode(client_creds.encode())
return client_creds_b64.decode()
def get_token_headers(self):
client_creds_b64 = self.get_client_credentials()
return {
'Authorization': f'Basic {client_creds_b64}' # <base64 encoded client_id:client_secret>
}
def get_token_data(self):
return {
'grant_type': 'client_credentials'
}
def perform_auth(self):
token_url = self.token_url
token_data = self.get_token_data()
token_headers = self.get_token_headers()
r = requests.post(token_url, data=token_data, headers=token_headers)
if r.status_code not in range(200, 299):
raise Exception('Could not authenticate client.')
data = r.json()
now = datetime.datetime.now()
access_token = data['access_token']
expires_in = data['expires_in'] # seconds
expires = now + datetime.timedelta(seconds=expires_in)
self.access_token = access_token
self.access_token_expires = expires
self.access_token_did_expire = expires < now
return True
def get_access_token(self):
token = self.access_token
expires = self.access_token_expires
now = datetime.datetime.now()
if expires < now:
self.perform_auth()
return self.get_access_token()
elif token == None:
self.perform_auth()
return self.get_access_token()
return token
def get_resource_header(self):
access_token = self.get_access_token()
headers = {
'Authorization': f'Bearer {access_token}'
}
return headers
def get_resource(self, lookup_id, resource_type='albums', version='v1'):
if resource_type == 'tracks':
endpoint = f'https://api.spotify.com/{version}/albums/{lookup_id}/{resource_type}'
elif resource_type == 'features':
endpoint = f'https://api.spotify.com/{version}/audio-features/{lookup_id}'
elif resource_type == 'analysis':
endpoint = f'https://api.spotify.com/{version}/audio-analysis/{lookup_id}'
elif resource_type == 'popularity':
endpoint = f'https://api.spotify.com/{version}/tracks/{lookup_id}'
elif resource_type != 'albums':
endpoint = f'https://api.spotify.com/{version}/{resource_type}/{lookup_id}'
else:
endpoint = f'https://api.spotify.com/{version}/artists/{lookup_id}/albums' # Get an Artist's Albums
headers = self.get_resource_header()
r = requests.get(endpoint, headers=headers)
if r.status_code not in range(200, 299):
return {}
return r.json()
def get_artist(self, _id):
return self.get_resource(_id, resource_type='artists')
def get_albums(self, _id):
return self.get_resource(_id, resource_type='albums')
def get_album_tracks(self, _id):
return self.get_resource(_id, resource_type='tracks')
def get_track_features(self, _id):
return self.get_resource(_id, resource_type='features')def get_track_analysis(self, _id):
return self.get_resource(_id, resource_type='analysis')
def get_track_popularity(self, _id):
return self.get_resource(_id, resource_type='popularity')
def get_next(self, result):
""" returns the next result given a paged result
Parameters:
- result - a previously returned paged result
"""
if result['next']:
return self.get_next_resource(result['next'])
else:
return None
def get_next_resource(self, url):
endpoint = url
headers = self.get_resource_header()
r = requests.get(endpoint, headers=headers)
if r.status_code not in range(200, 299):
return {}
return r.json()
def base_search(self, query_params): # search_type = spotify's type
headers = self.get_resource_header()
endpoint = 'https://api.spotify.com/v1/search'
lookup_url = f'{endpoint}?{query_params}'
r = requests.get(lookup_url, headers=headers)
if r.status_code not in range(200, 299):
return {}
return r.json()
def search(self, query=None, operator=None, operator_query=None, search_type='artist'):
if query == None:
raise Exception('A query is required.')
if isinstance(query, dict):
query = ' '.join([f'{k}:{v}' for k, v in query.items()])
if operator != None and operator_query != None:
if (operator.lower() == 'or') or (operator.lower() == 'not'): # Operators can only be OR or NOT
operator = operator.upper()
if isinstance(operator_query, str):
query = f'{query} {operator} {operator_query}'
query_params = urlencode({'q': query, 'type': search_type.lower()})
return self.base_search(query_params)
We implemented our functions to retrieve more specific data, such as band_id given band name, albums given band_id, tracks given album, features given track and popularity given track. To have more control during the process, each one of these processes were performed in a different for-loop and aggregated in a dataframe. Different approaches in this part of the data collection are encouraged, especially aiming performance gain.
我们实现了检索特定数据的功能,例如, band_id指定乐队名称 , 专辑指定band_id , 曲目指定专辑 , 功能指定曲目 , 流行度指定曲目 。 为了在此过程中拥有更多控制权 ,这些过程中的每一个都在不同的for循环中执行,并汇总在一个数据帧中。 鼓励在数据收集的这一部分采用不同的方法, 尤其是针对性能提升 。
Below, we sample the code used to catch band_id given band name.
下面,我们对用于捕获给定乐队名称的band_id的代码进行示例。
spotify = SpotifyAPI(client_id, client_secret)%%timebands_id = []
bands_popularity = []for band in df_unique['Band']:
id_found = False
result = spotify.search(query=band, search_type='artist')
items = result['artists']['items']
if len(items) > 0: # Loop to check whether more than one band is in items and retrieve desired band
i = 0
while i < len(items):
artist = items[i]
if band.lower() == artist['name'].lower():
bands_id.append(artist['id'])
bands_popularity.append(artist['popularity'])
id_found = True
break
i = i + 1
if (id_found == False) or (len(items) == 0): # If band not found
bands_id.append(np.nan)
bands_popularity.append(np.nan)df_unique['Band ID'] = bands_id
df_unique['Band Popularity'] = bands_popularity
df_unique = df_unique.dropna() # Dropping bands with uri not found
df_unique.sort_values('Band')
df_unique
Finally, one could store it in a SQL database, but we did save it into a .csv file. At the end, our final dataframe contained 498576 songs. Not bad.
最后,可以将其存储在SQL数据库中,但是我们确实将其保存到.csv文件中。 最后,我们的最终数据帧包含498576首歌曲。 不错。
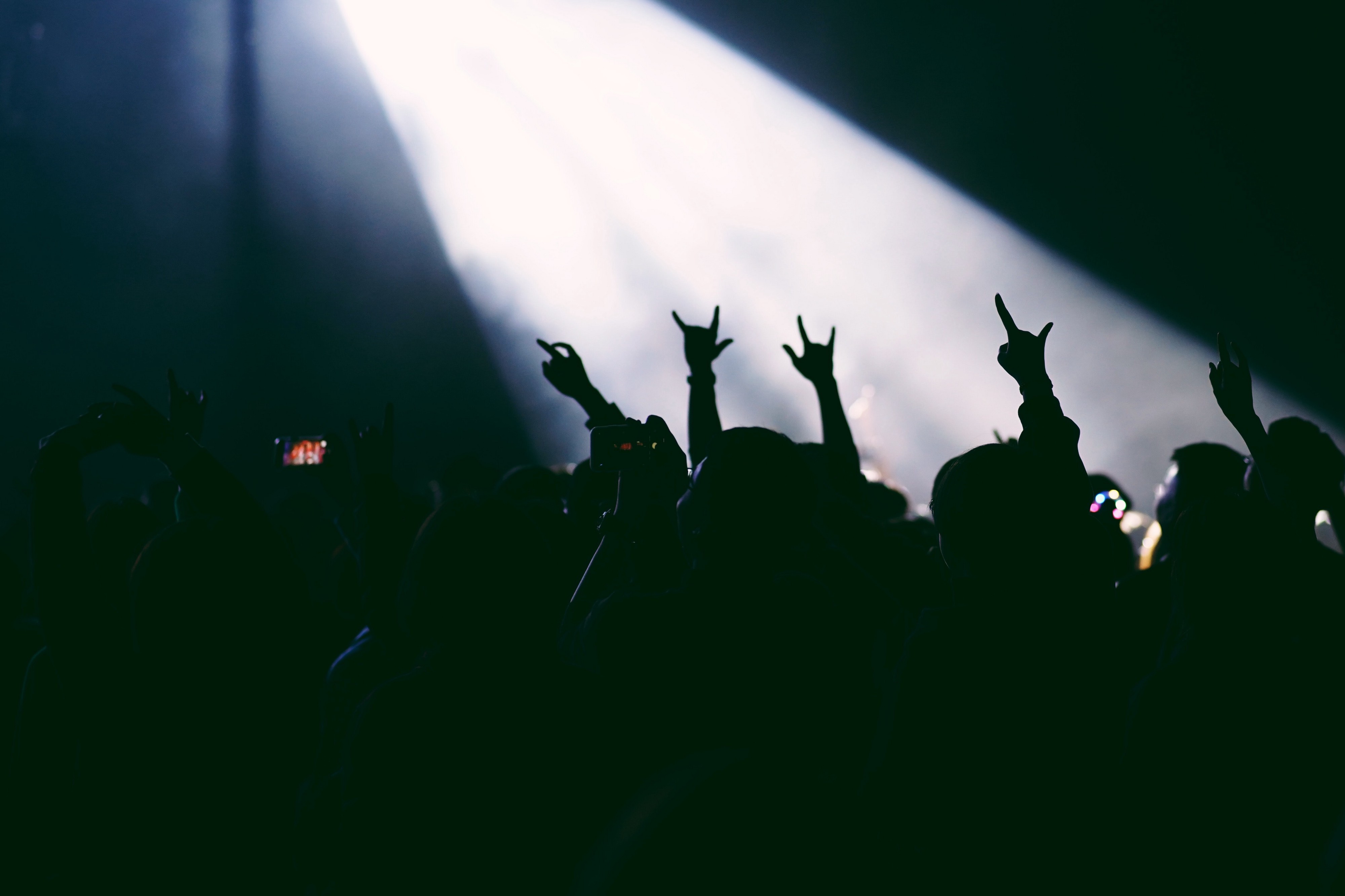
下一步是什么? (What’s next?)
After collecting all this data there are many possibilities. As exposed earlier, one could create genre classifiers giving audio features. Another possibility is to use the features to create playlists/recommender systems. Regression analysis could be applied to predict song/band popularity. Last but not least, developing an exploratory data analysis could mathematically show how each genre differs from each other. What would you like to see?
收集所有这些数据之后,有很多可能性。 如前所述,可以创建提供音频功能的流派分类器。 另一种可能性是使用这些功能来创建播放列表/推荐系统 。 回归分析可用于预测歌曲/乐队的受欢迎程度。 最后但并非最不重要的一点是,进行探索性数据分析可以从数学上显示出每种流派之间的差异。 你想看见什么?
翻译自: https://medium.com/swlh/no-data-no-problem-how-to-collect-heavy-metal-data-from-wikipedia-and-spotify-f879762046ff
spotify 数据分析
本文来自互联网用户投稿,该文观点仅代表作者本人,不代表本站立场。本站仅提供信息存储空间服务,不拥有所有权,不承担相关法律责任。如若转载,请注明出处:http://www.mzph.cn/news/388767.shtml
如若内容造成侵权/违法违规/事实不符,请联系多彩编程网进行投诉反馈email:809451989@qq.com,一经查实,立即删除!