数据统计 测试方法
This post is not meant for seasoned statisticians. This is geared towards data scientists and machine learning (ML) learners & practitioners, who like me, do not come from a statistical background.
Ť他的职位是不是意味着经验丰富的统计人员。 这是针对数据科学家和机器学习(ML)学习者和从业者的 ,他们和我一样,并非来自统计背景。
For a person being from a non-statistical background the most confusing aspect of statistics, are the fundamental statistical tests, and when to use which test?. This post is an attempt to mark out the difference between the most common tests and the relevant key assumptions.
对于一个非统计学背景的人来说,统计方面最令人困惑的方面是基本统计检验 ,以及何时使用哪种检验? 这篇文章是试图指出最常见的测试和相关的关键假设之间的差异。
目录 (Table of contents)
Terminologies: (KEY TERMINOLOGIES FOR THIS POST)
术语:( 此职位的主要术语)
- Statistical Test(Hypothesis Testing) 统计检验(假设检验)
- Statistical Assumptions 统计假设
- Parametric tests 参数测试
- Parametric test Flowchart 参数测试流程图
- Dealing with non-normal distributions (Non-Parametric tests) 处理非正态分布(非参数检验)
1)术语: (1) TERMINOLIGIES:)
独立变量和独立变量 (DEPENDENT AND INDEPENDENT VARIABLES)
An independent variable often called “predictor variable”, is a variable that is being manipulated in order to observe the effect on a dependent variable, sometimes called an outcome/output variable.
通常被称为“预测变量”的自变量是为了观察对因变量的影响而被操纵的变量,有时称为结果/输出变量。
- Independent variable(s)-> Predictor variable(s) 自变量->预测变量
- Dependent variable(s) -> Outcome/Output variable(s) 因变量->结果/输出变量
变量类型 (TYPES OF VARIABLES)
It is important to distinguish the difference between the type of variables because this plays a key role in determining the correct type of statistical test to adopt. There are two main categories:
区分变量类型之间的差异非常重要,因为这在确定要采用的正确统计检验类型中起着关键作用。 主要有两个类别:
QUANTITATIVE: express the amounts of things (e.g. the number of cigarettes in a pack). The two different types of quantitative variables are:
数量 : 表达物品的数量(例如,一包香烟的数量)。 两种不同类型的定量变量是:
CONTINOUS (a.k.a Ratio): is used to describe measures and can usually be divided into units smaller than one (e.g. 1.50 kg).
连续 (又称比率 ):用于描述度量,通常可以划分为小于一的单位(例如1.50千克)。
DISCRETE (a.k.a Interval): is used to describe counts and usually can’t be divided into units smaller than one (e.g. 1 cigarette).
DISCRETE (又名Interval ):用于描述计数,通常不能分为小于1的单位(例如1支香烟)。
CATEGORICAL: express groupings of things (e.g. the different type of fruits). The three different types of categorical variables are:
类别 : 表达事物的分组(例如,不同类型的水果)。 三种不同类型的类别变量是:
ORDINAL: represent data with an order (e.g. rankings).
序数:表示具有顺序的数据(例如排名)。
NOMINAL: represent group names (e.g. brands or species names).
名词:代表组名(例如品牌或品种名称)。
BINARY: represent data with a yes/no or 1/0 outcome (e.g. LEFT or RIGHT).
BINARY :表示结果为是/否或1/0的数据(例如,左或右)。
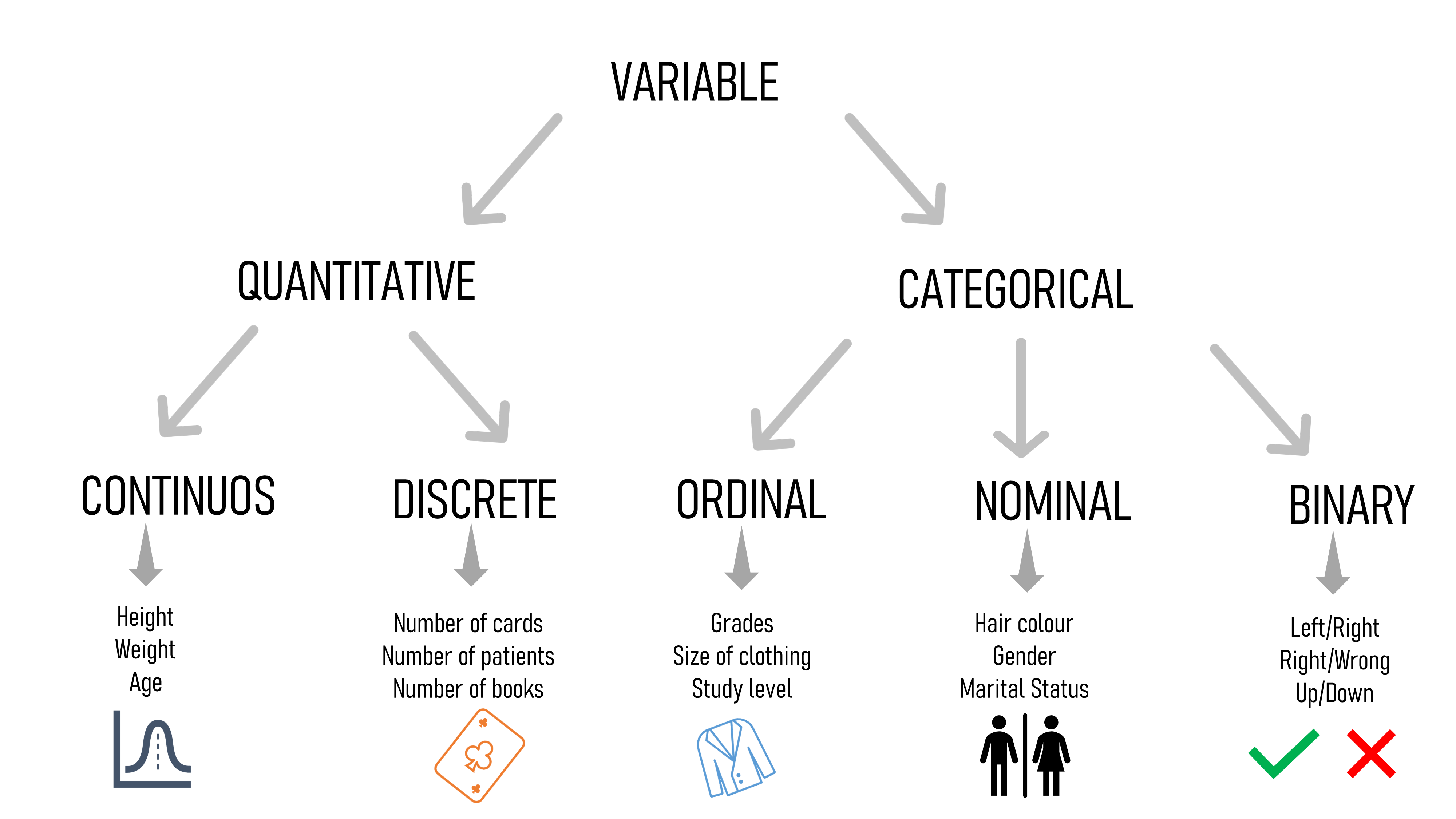
2)统计测试 (2) STATISTICAL TESTS)
Statistics is all about data. Data alone is not interesting. It is the interpretation of the data that we are interested in.
统计信息都是关于数据的。 单独的数据并不有趣。 它是对我们感兴趣的数据的解释。
In Statistics, one very important thing is statistical testing, if statistics “is the interpretation of the data”, statistical testing can be considered as the “formal procedure for investigating our ideas about the world”.
在统计中,非常重要的一件事是统计测试,如果统计“是对数据的解释”,则统计测试可以被视为“调查我们对世界的看法的正式程序”。
In other words, whenever we want to make claims about the distribution of data or whether one set of results are different from another set of results, data scientists must rely on hypothesis testing.
换句话说,每当我们要对数据的分布或一组结果是否与另一组结果有所不同时,数据科学家必须依靠假设检验。
假设检验 (HYPOTHESIS TESTING)
Using Hypothesis Testing, we try to interpret or draw conclusions about the population using sample data, evaluating two mutually exclusive statements about a population to determine which statement is best supported by the sample data.
使用“ 假设检验” ,我们尝试使用样本数据来解释或得出有关总体的结论,评估关于总体的两个互斥陈述,以确定样本数据最能支持哪种陈述。
假设检验有五个主要步骤: (THERE ARE FIVE MAIN STEPS IN HYPOTHESIS TESTING:)
Step 1) State your hypothesis as a Null (Ho) and Alternate (Ha) hypothesis.
步骤1)将您的假设陈述为零(Ho)和替代(Ha)假设。
Step 2) Choose a significance level (also called alpha or α).
步骤2)选择显着性水平(也称为alpha或α)。
Step 3) Collect data in a way designed to test the hypothesis.
步骤3)以旨在检验假设的方式收集数据。
Step 4) Perform an appropriate statistical test: compute the p-value and compare from the test to the significance level.
步骤4)执行适当的统计检验:计算p值,然后将检验与显着性水平进行比较。
Step 5) Decide whether to “ REJECT ” the null hypothesis(Ho) or “ FAIL TO REJECT ” the null hypothesis(Ho).
步骤5)决定是“拒绝”无效假设(Ho)还是“失败”无效假设(Ho)。
Note: Though the specific details might vary, the procedure you will use when testing a hypothesis will always follow some version of these steps.
注意 :尽管具体细节可能有所不同,但是在检验假设时将使用的过程将始终遵循这些步骤的某些版本。
If you want to further understand hypothesis testing, I would highly recommend these two great posts on Hypothesis testing.
如果您想进一步了解假设检验,我强烈推荐有关假设检验的这两篇好文章。
3)统计假设 (3) STATISTICAL ASSUMPTIONS)
Statistical tests make some common assumptions about the data being tested (If these assumptions are violated then the test may not be valid: e.g. the resulting p-value may not be correct)
统计测试对要测试的数据做出一些通用假设(如果违反了这些假设,则该测试可能无效:例如,得出的p值可能不正确)
Independence of observations: the observations/variables you include in your test should not be related(e.g. several tests from a same test subject are not independent, while several tests from multiple different test subjects are independent)
观察结果的独立性 :您包含在测试中的观察值/变量不应该相关(例如,来自同一测试对象的多个测试不是独立的,而来自多个不同测试对象的多个测试是独立的)
Homogeneity of variance: the “variance” within each group is being compared should be similar to the rest of the group variance. If a group has a bigger variance than the other(s) this will limit the test’s effectiveness.
方差的同质性 :比较每个组中的“方差”应与其余组方差相似。 如果组的方差大于其他方,这将限制测试的有效性。
Normality of data: the data follows a normal distribution, normality means that the distribution of the test is normally distributed (or bell-shaped) with mean 0, with 1 standard deviation and a symmetric bell-shaped curve.
数据的正态性 :数据遵循正态分布,正态性表示测试的分布呈正态分布(或钟形),平均值为0,标准差为1,钟形曲线对称。
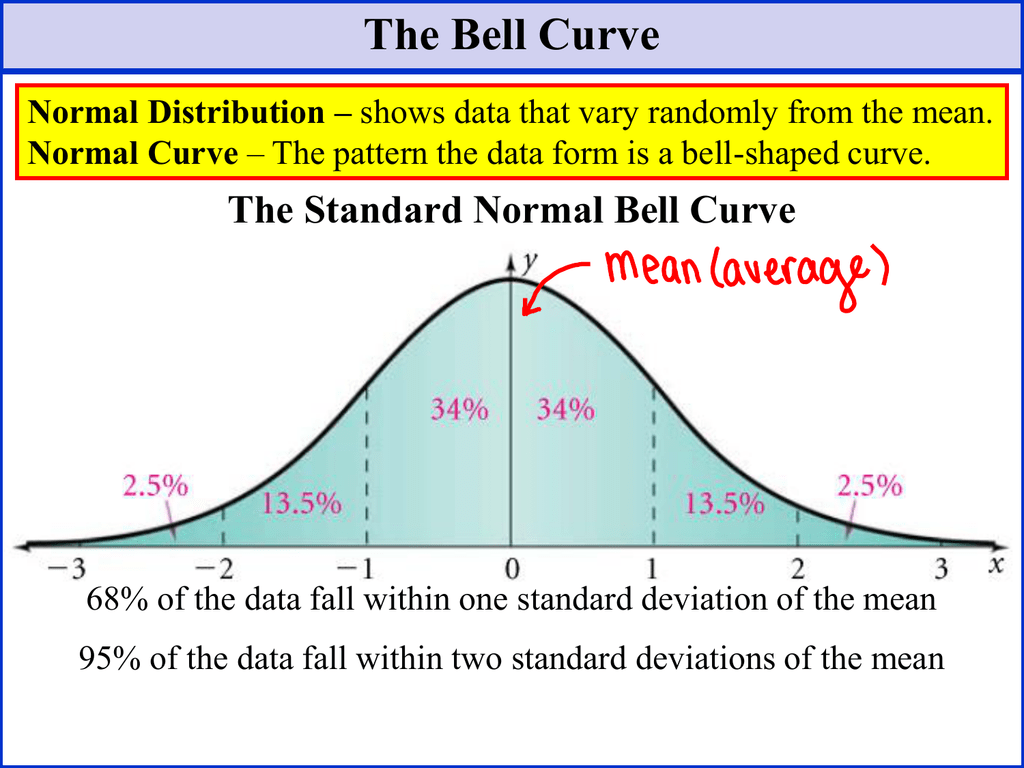
4)参数测试 (4) PARAMETRIC TESTS)
Parametric tests are the ones that can only be run with data that stick with the “three statistical assumptions” mentioned above. The most common types of parametric tests are divided into three categories.
参数测试是只能使用符合上述“三个统计假设”的数据运行的测试。 最常见的参数测试类型分为三类。
回归测试: (Regression tests:)
These tests are used test cause-and-effect relationships, if the change in one or more continuous variable predicts change in another variable.
如果一个或多个连续变量的变化预示着另一个变量的变化,则将这些检验用于检验因果关系 。
Simple linear regression: tests how a change in the predictor variable predicts the level of change in the outcome variable.
简单线性回归:测试预测变量的变化如何预测结果变量的变化水平。
Multiple linear regression: tests how changes in the combination of two or more predictor variables predict the level of change in the outcome variable
多元线性回归:测试两个或多个预测变量组合的变化如何预测结果变量的变化水平
Logistic regression: is used to describe data and to explain the relationship between one dependent (binary) variable and one or more nominal, ordinal, interval or ratio-level independent variable(s).
Logistic回归:用于描述数据并解释一个(二元)变量与一个或多个名义,有序,区间或比率级别的自变量之间的关系。
比较测试: (Comparison tests:)
These tests look for the difference between the means of variables:Comparison of Means.
这些测试寻找变量均值之间的差异:均值比较。
T-tests are used when comparing the means of precisely two groups (e.g. the average heights of men and women).
在精确比较两组的平均值(例如,男性和女性的平均身高)时,使用T检验 。
Independent t-test: Tests the difference between the same variable from different populations (e.g., comparing dogs to cats)
独立t检验 :测试来自不同人群的相同变量之间的差异 (例如,比较狗和猫)
ANOVA and MANOVA tests are used to compare the means of more than two groups or more(e.g. the average weights of children, teenagers, and adults).
ANOVA和MANOVA检验用于比较两组或以上两组的均值(例如,儿童,青少年和成人的平均体重)。
关联测试: (Correlation tests:)
These tests look for an association between variable checking whether two variables are related.
这些测试在变量之间寻找关联,检查两个变量是否相关。
Pearson Correlation: Tests for the strength of the association between two continuous variables.
皮尔逊相关:测试两个连续变量之间关联的强度。
Spearman Correlation: Tests for the strength of the association between two ordinal variables (it does not rely on the assumption of normally distributed data)
Spearman相关性:测试两个序数变量之间的关联强度(它不依赖于正态分布数据的假设)
Chi-Square Test: Tests for the strength of the association between two categorical variables.
卡方检验:测试两个类别变量之间的关联强度。
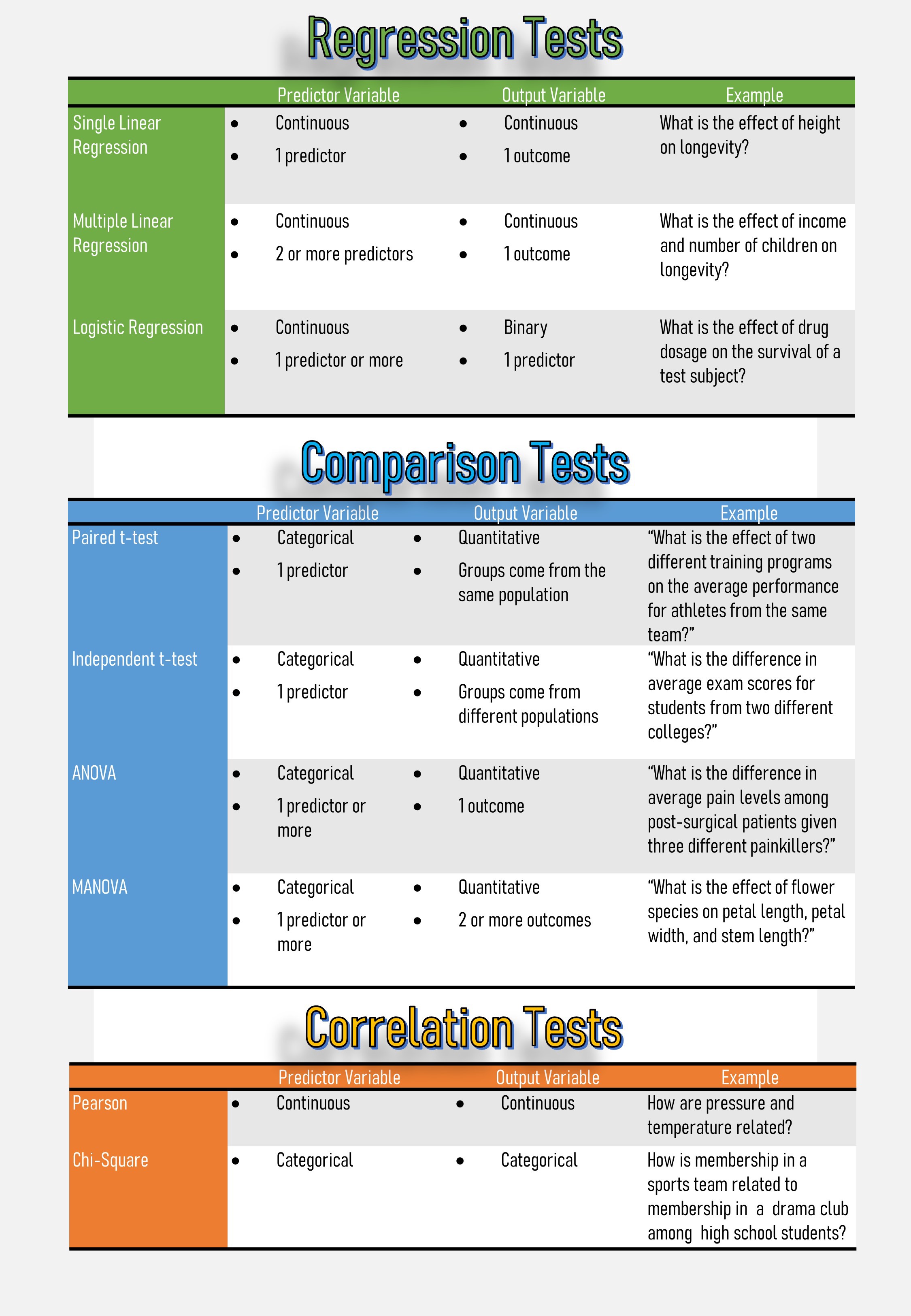
5)流程图:选择参数测试 (5) FLOWCHART: CHOOSING A PARAMETRIC TEST)
This flowchart will help you choose among the above described parametric tests. For nonparametric alternatives, check the following section.
该流程图将帮助您在上述参数测试中进行选择。 对于非参数替代,请检查以下部分。
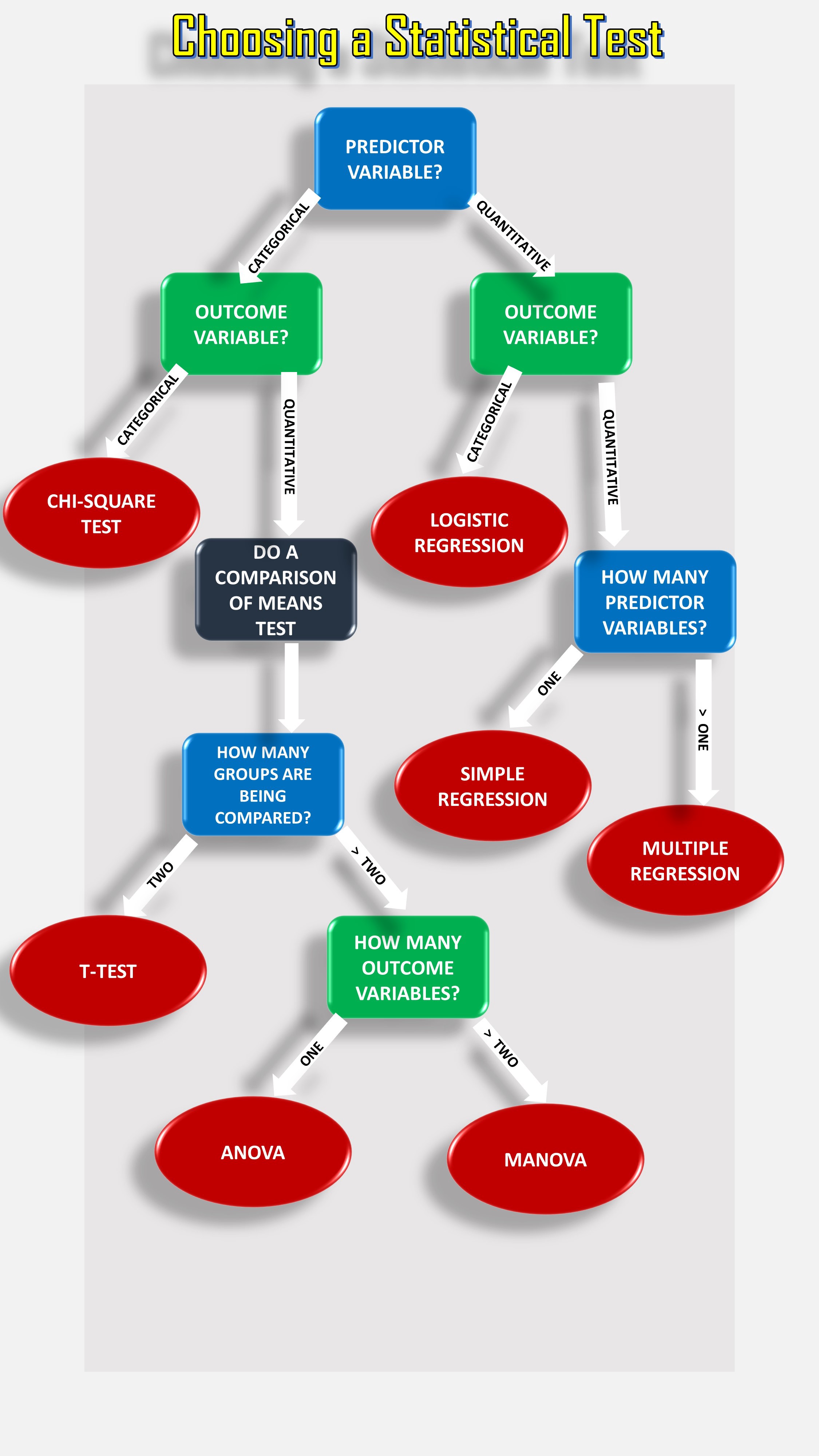
6)处理非正态分布 (6) DEALING WITH NON- NORMAL DISTRIBUTIONS)
Although the normal distribution takes centre part in statistics, many processes follow non-normal distributions. Many datasets naturally fit a non-normal model:
尽管正态分布在统计中占据中心位置,但是许多过程遵循非正态分布。 许多数据集自然适合于非正常模型:
-The number of accidents tends to fit a “Poisson distribution”
-事故数量趋于符合“泊松分布”
-The Lifetimes of products usually fit a “Weibull distribution”.
-产品的使用寿命通常符合“威布尔分布”。
非正态分布的示例 (Example of Non-Normal Distributions)
- Beta Distribution. Beta发行版。
- Exponential Distribution. 指数分布。
- Gamma Distribution. 伽玛分布。
- Inverse Gamma Distribution. 反伽玛分布。
- Log-Normal Distribution. 对数正态分布。
- Logistic Distribution. 物流配送。
- Maxwell-Boltzmann Distribution. Maxwell-Boltzmann分布。
- Poisson Distribution. 泊松分布。
- Skewed Distribution. 分布偏斜。
- Symmetric Distribution. 对称分布。
- Uniform Distribution. 均匀分布。
- Unimodal Distribution. 单峰分布。
- Weibull Distribution. 威布尔分布。
那么,我们如何处理非正态分布? (Well then, How do we deal with non-Normal-Distributions?)
When your data is supposed to fit a normal distribution but doesn’t, we could do a few things to handle them:
当您的数据应该符合正态分布但不符合正态分布时,我们可以做一些事情来处理它们:
- We may still be able to run parametric tests if your sample size is large enough (usually over 20 items) and try to interpret the results accordingly. 如果您的样本量足够大(通常超过20个项目),我们仍然可以运行参数测试,并尝试相应地解释结果。
- We may choose to transform the data with different statistical techniques, forcing it to fit a normal distribution. 我们可能选择使用不同的统计技术来转换数据,迫使其适应正态分布。
If the sample size is small, skewed or if it represents another distribution type, you might run a non-parametric test.
如果样本量小,偏斜或代表其他分布类型,则可以运行非参数检验 。
非参数测试 (Non-Parametric Tests)
Non-parametric tests (figure below) don’t make as many assumptions about the data and are useful when one or more of the three statistical assumptions are violated.
非参数检验(下图)对数据的假设不多,当违反三个统计假设中的一个或多个时很有用。
Note that: The inferences that non-parametric tests make aren’t as strong as the parametric tests.
请注意:非参数测试的推论不如参数测试强。
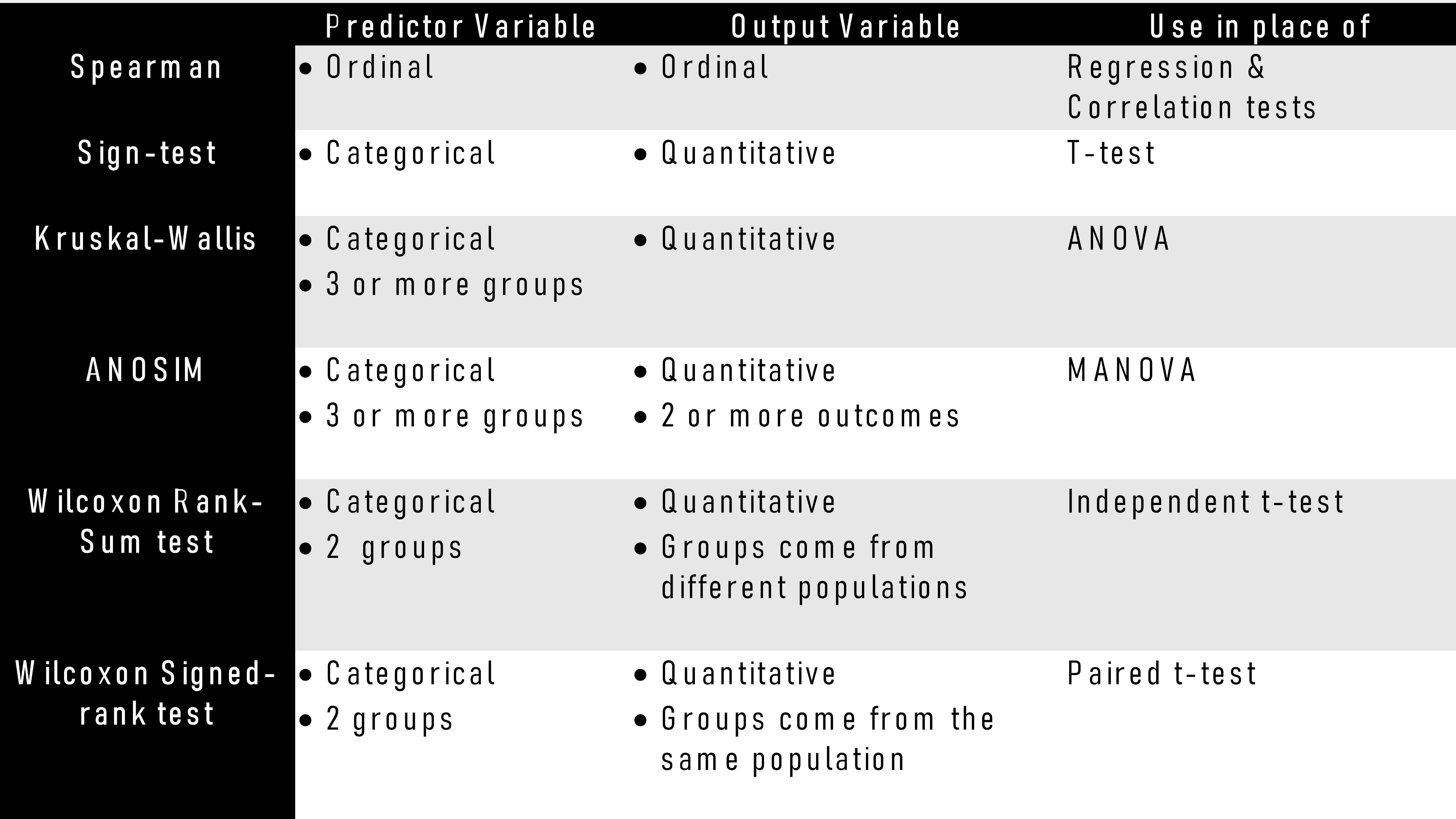
Hope you find this post informative and useful. Please let me know if you have any feedback. Thanks a lot for reading!
希望您发现这篇文章有益和有用。 如果您有任何反馈意见,请告诉我。 非常感谢您的阅读!
翻译自: https://towardsdatascience.com/statistical-testing-understanding-how-to-select-the-best-test-for-your-data-52141c305168
数据统计 测试方法
本文来自互联网用户投稿,该文观点仅代表作者本人,不代表本站立场。本站仅提供信息存储空间服务,不拥有所有权,不承担相关法律责任。如若转载,请注明出处:http://www.mzph.cn/news/388746.shtml
如若内容造成侵权/违法违规/事实不符,请联系多彩编程网进行投诉反馈email:809451989@qq.com,一经查实,立即删除!