项目背景
目标
预测一个乘客是否能够在泰坦尼克号事件中幸存。
概述
1912年4月15日,泰坦尼克号在首次航行期间撞上冰山后沉没,船上共有2224名人员(包括乘客和机组人员),共有1502人不幸遇难。造成海难失事的原因之一是乘客和机组人员没有足够的救生艇。尽管在沉船事件中能否幸存有一定的运气因素,但有些人存活几率更大,比如女人,孩子以及上流社会人士。
通过使用机器学习工具来预测哪些人员在时间中幸存。
理解数据
数据总览
Titanic生存模型预测,包含了两组数据:train.csv和test.csv,分别为训练数据集和测试数据集。
首先,导入数据:
import pandas as pd
import numpy as np
import re# 导入数据
train_data = pd.read_csv('train.csv')
#预览数据
train_data.head(2)

可以看到,训练数据集共有12列,其中Survived字段表示该乘客是否获救,其余为乘客信息,包括: PassengerID:乘客ID Pclass:乘客船舱等级 Name:姓名 Sex:性别 Age:年龄 SibSp:兄弟姐妹数量 Parch:父母子女数量 Ticket:船票信息 Fare:票价 Cabin:客舱信息 * Embarked:登船港口
查看数据整体信息:
train_data.info()
<class 'pandas.core.frame.DataFrame'>
RangeIndex: 891 entries, 0 to 890
Data columns (total 12 columns):
PassengerId 891 non-null int64
Survived 891 non-null int64
Pclass 891 non-null int64
Name 891 non-null object
Sex 891 non-null object
Age 714 non-null float64
SibSp 891 non-null int64
Parch 891 non-null int64
Ticket 891 non-null object
Fare 891 non-null float64
Cabin 204 non-null object
Embarked 889 non-null object
dtypes: float64(2), int64(5), object(5)
memory usage: 83.6+ KBtrain_data.describe()
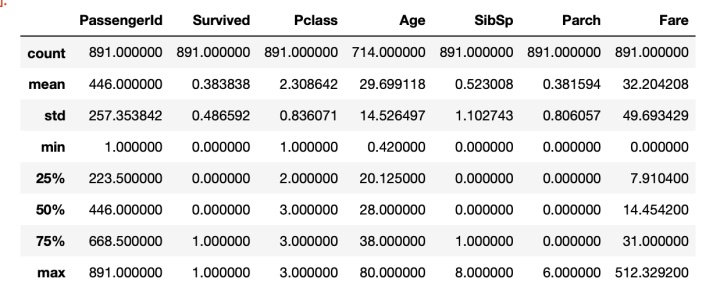
从上面可以看出,训练集共有891名乘客,但是有些属性数据不全,如Age和Cabin。大约有38%的人员最终获救;平均年龄大概是29岁,Age的最小值为0.42,表示的应该是婴儿的年龄,最大值为80岁;Fare票价的平均值为32,中位数为14,平均值是中位数的2.3倍,说明该特征的分布是严重的偏右,且最大值为512,很有可能是一个异常值。
数据初步分析
分析各属性与获救结果的关系,并选择合适的可视化方法进行数据可视化分析
数据分类
- 数值类型: 乘客ID(PassengerID),年龄(Age),票价(Fare),兄弟姐妹数量(SibSp),父母子女数量(Parch)
- 分类数据:
有直接类别的:乘客性别(Sex),客舱等级(Pclass),登船港口(Embarked)
待提取的特征:乘客姓名(Name),客舱号(Cabin),船票号(Ticket)
import matplotlib.pyplot as plt
import seaborn as sns
可视化
- 先从容易入手的3种分类特征进行可视化,SexPclassEmbarked特征分析
fig, axes = plt.subplots(1,3, figsize=(20, 6))
sns.countplot('Sex', hue='Survived', data=train_data, ax=axes[0])
sns.countplot('Pclass', hue='Survived', data=train_data, ax=axes[1])
sns.countplot('Embarked', hue='Survived', data=train_data, ax=axes[2])
<matplotlib.axes._subplots.AxesSubplot at 0x1a253bdac8>
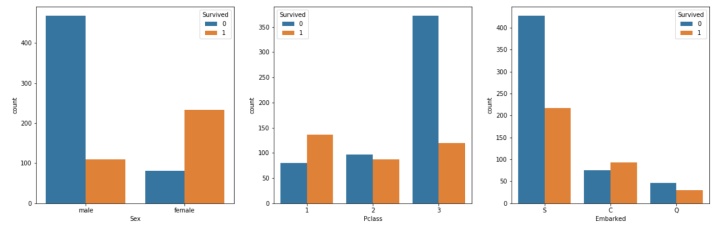
通过观察各特征的分布情况与目标变量之间的关系,初步得出如下结论: Sex:男性总人数大于女性总人数,但女性的存活率要远远高于男性; Pclass:1等舱存活率最高,3等舱存活率明显低于其他舱,这是由于3等舱的多为普通人,而等级越高的舱位越有可能是当时社会地位较高的人; * Embarked:S港口登船的数量最多,但是获救率最低;
不同船舱等级下各性别的获救情况:
train_data[['Sex', 'Pclass', 'Survived']].groupby(['Pclass', 'Sex']).mean()
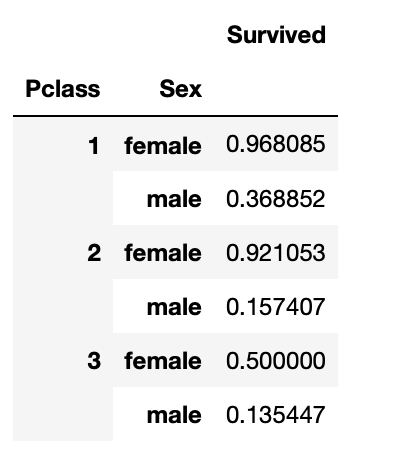
train_data[['Sex', 'Pclass', 'Survived']].groupby(['Pclass', 'Sex']).mean().plot.bar()
<matplotlib.axes._subplots.AxesSubplot at 0x1a25891ef0>
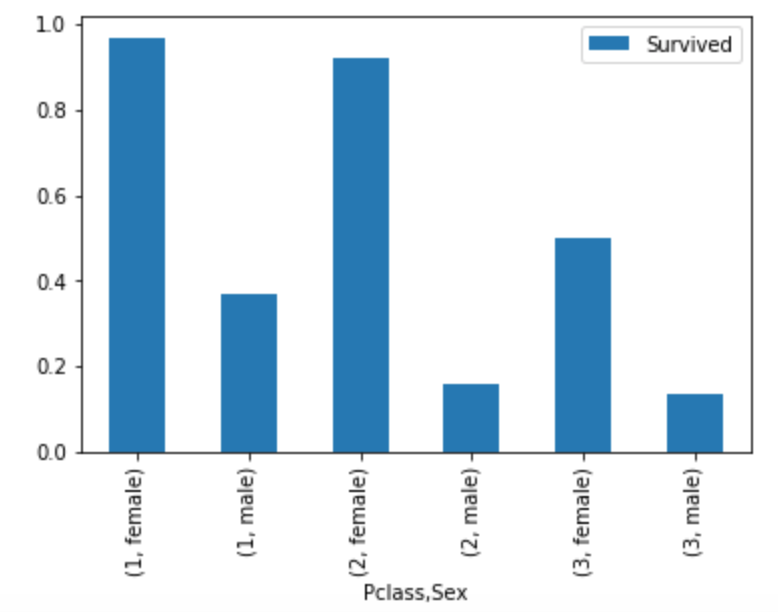
- 亲友的人数与存活与否的关系 SibSp & Parch
fig, axes=plt.subplots(1, 2, figsize=(16, 6))
train_data[['SibSp', 'Survived']].groupby('SibSp').mean().plot.bar(ax=axes[0])
train_data[['Parch', 'Survived']].groupby('Parch').mean().plot.bar(ax=axes[1])
<matplotlib.axes._subplots.AxesSubplot at 0x1a25ae9da0>
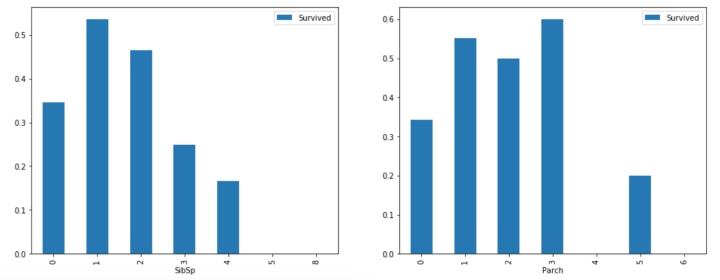
从亲友人数的获救概率上来看,独自一人的乘客获救概率较低
- 年龄特征分析 Age
年龄特征分布:
fig, axes = plt.subplots(1, 2, figsize=(16,6))
train_data['Age'].hist(bins=70, ax=axes[0])
axes[0].set_title('Age')train_data.boxplot(column='Age')
<matplotlib.axes._subplots.AxesSubplot at 0x1a25be7080>
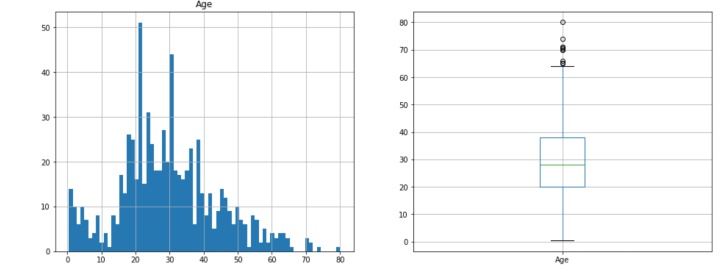
facet = sns.FacetGrid(train_data, aspect=4, row='Sex')
facet.map(sns.kdeplot, 'Age', shade=True)
facet.set(xlim=(0, train_data['Age'].max()))
facet.add_legend()
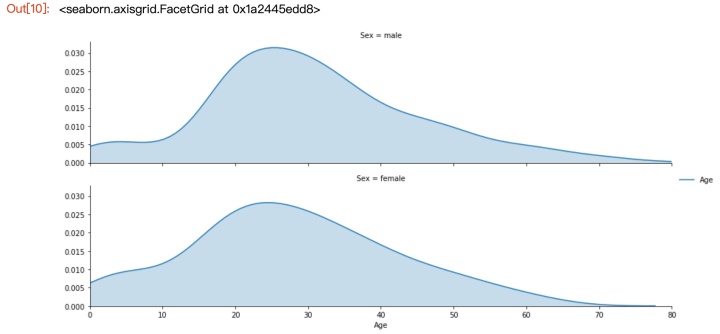
不同年龄下的生存分布情况:
facet = sns.FacetGrid(train_data, hue='Survived', aspect=4)
facet.map(sns.kdeplot, 'Age', shade=True)
facet.set(xlim=(0, train_data['Age'].max()))
facet.add_legend()
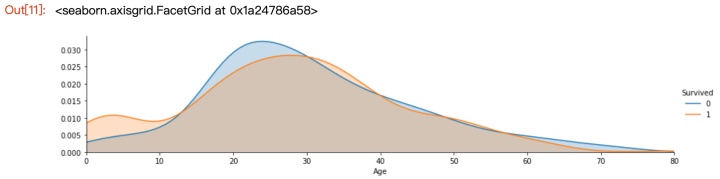
facet = sns.FacetGrid(train_data, hue='Survived', aspect=4, row='Sex')
facet.map(sns.kdeplot, 'Age', shade=True)
facet.set(xlim=(0, train_data['Age'].max()))
facet.add_legend()
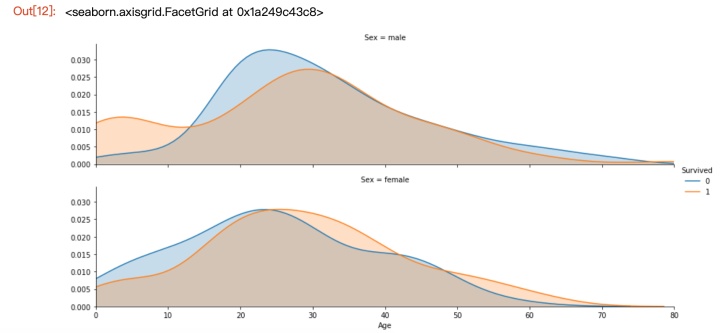
整体观察得知,0到十几岁的孩子生存率最高,20-30岁左右的生存率较低,而对于男性来说,0到十几岁的孩子生存率明显较高,而对于女性来说,则是30-40的年龄段生存率较高。
- 票价特征分析Fare
train_data['Fare'].describe()
count 891.000000
mean 32.204208
std 49.693429
min 0.000000
25% 7.910400
50% 14.454200
75% 31.000000
max 512.329200
Name: Fare, dtype: float64train_data['Fare'].hist(bins=10)
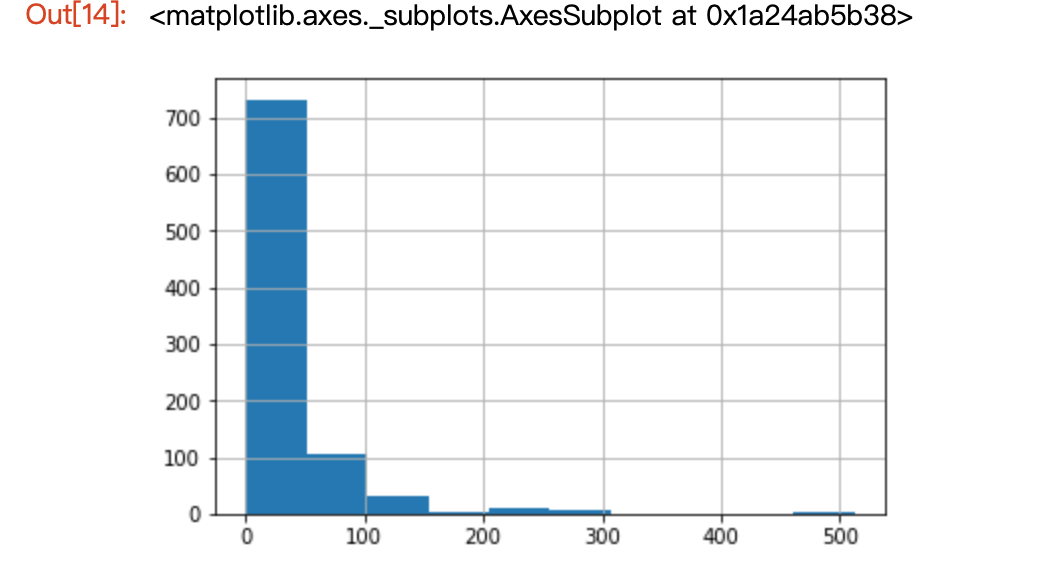
train_data['Fare'][train_data['Survived']==0].mean()
22.117886885245877train_data['Fare'][train_data['Survived']==1].mean()
48.39540760233917
观察得知,低票价的数量多,而高票价的数量少,且生存乘客的平均票价是遇难乘客的2倍多。
乘客姓名,客舱号,船票号
- 乘客姓名特征 Name
#定义函数,从姓名中获取头衔
def getTitle(name):str1 = name.split(',')[1]str2 = str1.split('.')[0]str3 = str2.strip()return str3Title = pd.DataFrame()
Title['Title'] = train_data['Name'].map(getTitle)
Title.head()
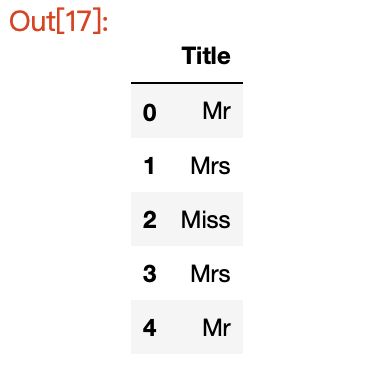
- 船舱特征 Cabin
train_data['Cabin'].describe()
count 204
unique 147
top C23 C25 C27
freq 4
Name: Cabin, dtype: object
由于船舱的缺失值太多,有效值仅为204,在做特征工程的时候可以丢弃,也可以简单的将数据分为有cabin记录和无cabin记录
train_data['Cabin'] = train_data['Cabin'].fillna('U0')
train_data['Has_cabin'] = train_data['Cabin'].apply(lambda x: 0 if x=='U0' else 1)train_data[['Has_cabin', 'Survived']].groupby('Has_cabin').mean().plot.bar()
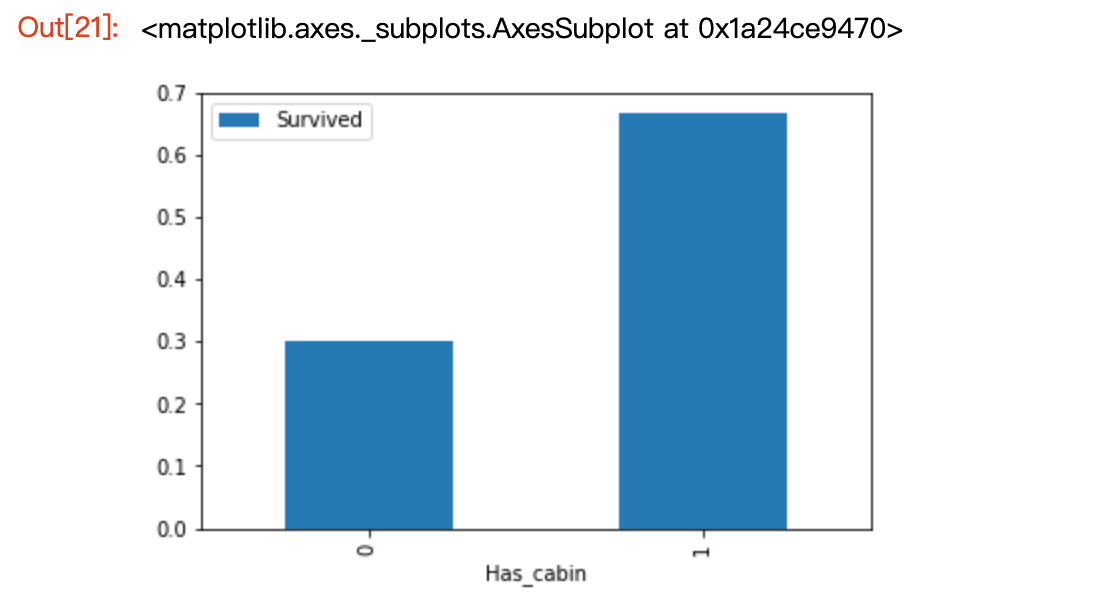
从分析可知,有船舱信息的乘客生存率较高
特征工程
数据准备
特征工程包括几个方面:
1. 变量转换
变量转换的目的是将数据转换为适合模型使用的数据,不同模型接受的数据类型不同。Scikit-learn要求数据都是数值型的numeric,所以要将原始数据类型转换为numeric。
所有的数据都可以归为两类: 定量型(quantitative)变量:如Age 定性性(qualitative)变量:如Embarked
Qualitative数据转换
- 独热编码(Dummy)pd.get_dummies( )
适用于属性值域较小的特征,如 gender = {‘male’, ‘female’} * Factorizing 因子分解 pd.factorize( )
factorize把相同字符映射为同一个数字,这种映射最后只生产一个特征,不像dummies生成多个特征;
Quantitative数据转换
- Scaling 数据标准化
unscaled data的弊端:1.数据可视化困难;2.数据范围差异过大可能导致大范围数值特征具有更高的权重,在某些对特征大小敏感的模型中会影响结果;
常见的scale方法有:
from sklearn.preprocessing import MinMaxScaler from sklearn.preprocessing import minmax_scale from sklearn.preprocessing import MaxAbsScaler from sklearn.preprocessing import StandardScaler from sklearn.preprocessing import RobustScaler from sklearn.preprocessing import Normalizer
- Binning 将连续数据离散化,存储的值被分布到一些‘箱’中,就像直方图的bin将数据划分成几块一样。
2. 缺失值处理
3. 特征工程
衍生变量:对特征进行衍生,产生新特征
在对数据进行特征工程时,我们不仅需要对训练数据进行处理,还需要同时对测试数据一起处理,使得二者具有相同的数据类型和数据分布。
train_data = pd.read_csv('train.csv')
test_data = pd.read_csv('test.csv')
test_data['Survived'] = 0
df = train_data.append(test_data)
/anaconda3/lib/python3.7/site-packages/pandas/core/frame.py:6211: FutureWarning: Sorting because non-concatenation axis is not aligned. A future version
of pandas will change to not sort by default.To accept the future behavior, pass 'sort=False'.To retain the current behavior and silence the warning, pass 'sort=True'.sort=sort)
df.head(2)

df.info()
<class 'pandas.core.frame.DataFrame'>
Int64Index: 1309 entries, 0 to 417
Data columns (total 12 columns):
Age 1046 non-null float64
Cabin 295 non-null object
Embarked 1307 non-null object
Fare 1308 non-null float64
Name 1309 non-null object
Parch 1309 non-null int64
PassengerId 1309 non-null int64
Pclass 1309 non-null int64
Sex 1309 non-null object
SibSp 1309 non-null int64
Survived 1309 non-null int64
Ticket 1309 non-null object
dtypes: float64(2), int64(5), object(5)
memory usage: 132.9+ KB# 计算缺失率
df.isnull().sum()/len(df)Age 0.200917
Cabin 0.774637
Embarked 0.001528
Fare 0.000764
Name 0.000000
Parch 0.000000
PassengerId 0.000000
Pclass 0.000000
Sex 0.000000
SibSp 0.000000
Survived 0.000000
Ticket 0.000000
dtype: float64
合并后数据共1309条,其中Age、Cabin、Embarked、Fare项有缺失,根据具体的情况进行缺失值处理
- Fare 票价
Fare仅缺失一个值,可以用平均值填充
df['Fare'] = df['Fare'].fillna(df['Fare'].mean())
- Embarked 登船口
Embarked仅缺失了2条数据,可以众数填充
df['Embarked'].value_counts()
S 914
C 270
Q 123
Name: Embarked, dtype: int64
df['Embarked'] = df['Embarked'].fillna('S')
有三种不同的港口,通过dummies转换为numeric数据
# 为了后面的特征分析,将Embarked特征进行factorizing
df['Embarked'] = pd.factorize(df['Embarked'])[0]
# 使用get_dummies 获取 one_hot 编码
embarked_dummies = pd.get_dummies(df['Embarked'], prefix=df[['Embarked']].columns[0])
df = pd.concat([df, embarked_dummies], axis=1)
- Sex 性别
Sex特征无缺失,需要做变量转换,转换成numeric类型数据
df['Sex'] = pd.factorize(df['Sex'])[0]
sex_dummies = pd.get_dummies(df['Sex'], prefix='Sex')
df = pd.concat([df, sex_dummies], axis=1)
- Pclass 船舱等级
df['Pclass'] = pd.factorize(df['Pclass'])[0]
pclass_dummies = pd.get_dummies(df['Pclass'], prefix='Pclass')
df = pd.concat([df, pclass_dummies], axis=1)
- Cabin 船舱号
Cabin项的缺失值太多,缺失率达到77%,很难进行分析,作为特征输入也会影响模型结果。可以舍弃。但是从有无船舱号这一角度,可以创建一个衍生特征,Has_cabin项。
# 将缺失项填充为U0
df['Cabin'] = df['Cabin'].fillna('U0')
df['Has_cabin'] = df['Cabin'].apply(lambda x: 0 if x=='U0' else 1)
- Name 姓名
观察数据可知,姓名中包含乘客身份信息的称呼,需要从姓名中进行提取
# 从name中提取称呼
df['Title'] = df['Name'].map(lambda x: x.split(',')[1].split('.')[0].strip())
# 建立映射字典
Title_dictionary = {'Capt': 'Officer','Col': 'Officer','Major': 'Officer','Jonkheer':'Royalty','Don': 'Royalty','Sir': 'Royalty','Dr': 'Officer','Rev': 'Officer','the Countess': 'Royalty','Dona': 'Royalty','Mme': 'Mrs','Mlle': 'Miss','Ms': 'Mrs','Mr': 'Mr','Mrs': 'Mrs','Miss': 'Miss','Master': 'Master','Lady': 'Royalty'
}
df['Title'] = df['Title'].map(Title_dictionary)
title_dummies = pd.get_dummies(df['Title'], prefix='Title')
df = pd.concat([df, title_dummies], axis=1)
- Parch and SibSp
由前面的分析可知,亲友的数量对Survived有所影响,这里将两者合并为FamilySize这一组合项,同时保留这两列。
family = pd.DataFrame()family['FamilySize'] = df['Parch'] + df['SibSp'] + 1
family['Family_Single'] = family['FamilySize'].map(lambda x: 1 if x == 1 else 0)
family['Family_Small'] = family['FamilySize'].map(lambda x: 1 if 2 <= x <=4 else 0)
family['Family_Large'] = family['FamilySize'].map(lambda x: 1 if 5 <= x else 0)family.head()
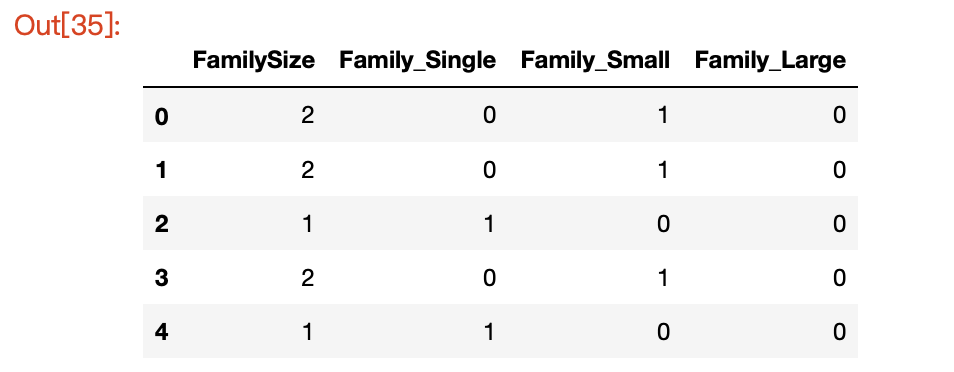
df = pd.concat([df, family], axis=1)
df.head()
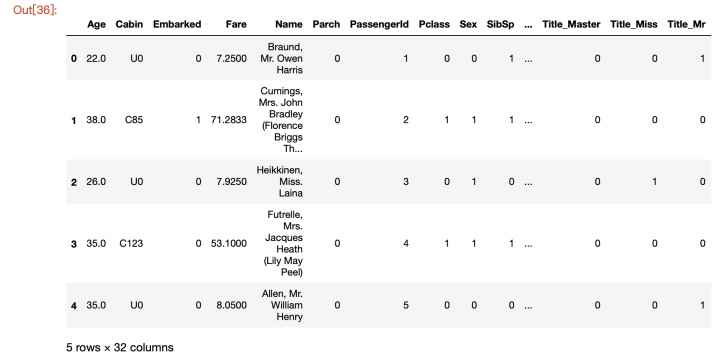
- Age年龄
df['Age'] = df['Age'].fillna(df['Age'].mean())
特征选择
- 对特征间的相关性进行分析
corr_df = df.corr()# 查看各个特征与Survived的相关系数corr_df['Survived'].sort_values(ascending = False)
Survived 1.000000
Sex 0.404020
Sex_1 0.404020
Title_Miss 0.263140
Has_cabin 0.245239
Title_Mrs 0.235600
Pclass_1 0.208166
Family_Small 0.202162
Pclass 0.175184
Fare 0.173630
Embarked_1 0.096513
Pclass_2 0.062279
Title_Master 0.058265
Parch 0.054908
Embarked 0.048409
Title_Royalty 0.036875
FamilySize 0.020555
Embarked_2 -0.012730
Title_Officer -0.013356
SibSp -0.014375
Age -0.060203
Embarked_0 -0.077095
Family_Large -0.081979
Family_Single -0.154285
Pclass_0 -0.231169
PassengerId -0.331493
Sex_0 -0.404020
Title_Mr -0.411211
Name: Survived, dtype: float64
- 标准化
标准化的目的主要是消除不同特征之间的量纲和取值范围不同造成的差异。这些差异,不仅会造成数据偏重不均,还会在可视化方面造成困扰。
使用sklearn.preprocessing.StandardScaler类,该类的好处是可以保存数据集中的参数的「均值、方差」
这里对Age和Fare数据进行标准化处理
from sklearn import preprocessingscale_age_fare = preprocessing.StandardScaler().fit(df[['Age', 'Fare']])
df[['Age', 'Fare']] = scale_age_fare.transform(df[['Age', 'Fare']])
df.head(2)
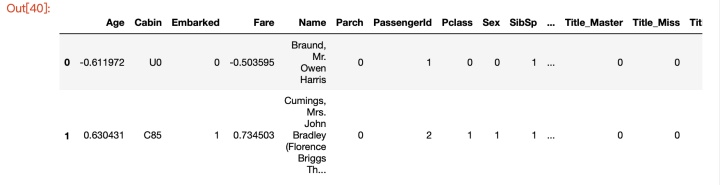
- 弃掉无用特征
在特征工程中,我们从一些原始特征中提取来很多要融合到模型中的特征,但是我们还需要提出一些我们用不到或者非数值特征:
首先,对数据进行一下备份,以便后期的再次分析:
df_backup = df
df.drop(['PassengerId', 'Cabin', 'Embarked', 'Sex', 'Name', 'Title', 'Pclass', 'Parch', 'SibSp', 'Ticket', 'FamilySize'], axis =1, inplace = True)
df.info()
<class 'pandas.core.frame.DataFrame'>
Int64Index: 1309 entries, 0 to 417
Data columns (total 21 columns):
Age 1309 non-null float64
Fare 1309 non-null float64
Survived 1309 non-null int64
Embarked_0 1309 non-null uint8
Embarked_1 1309 non-null uint8
Embarked_2 1309 non-null uint8
Sex_0 1309 non-null uint8
Sex_1 1309 non-null uint8
Pclass_0 1309 non-null uint8
Pclass_1 1309 non-null uint8
Pclass_2 1309 non-null uint8
Has_cabin 1309 non-null int64
Title_Master 1309 non-null uint8
Title_Miss 1309 non-null uint8
Title_Mr 1309 non-null uint8
Title_Mrs 1309 non-null uint8
Title_Officer 1309 non-null uint8
Title_Royalty 1309 non-null uint8
Family_Single 1309 non-null int64
Family_Small 1309 non-null int64
Family_Large 1309 non-null int64
dtypes: float64(2), int64(5), uint8(14)
memory usage: 99.7 KB
df.head()
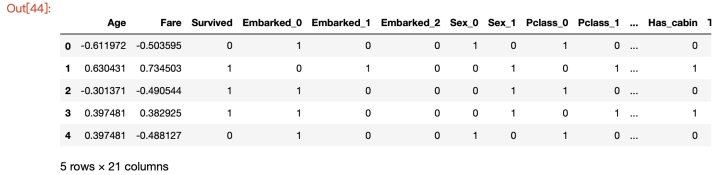
构建模型
划分训练数据集和测试数据集
train_data = df[:891]
test_data = df[891:]train_data_X = train_data.drop(['Survived'], axis=1)
train_data_Y = train_data['Survived']test_data_X = test_data.drop(['Survived'], axis=1)
train_data_X.shape
(891, 20)
from sklearn.model_selection import train_test_split#建立模型用的训练数据集和测试数据集
train_X, test_X, train_y, test_y = train_test_split(train_data_X, train_data_Y, train_size=.8)
/anaconda3/lib/python3.7/site-packages/sklearn/model_selection/_split.py:2179: FutureWarning: From version 0.21, test_size will always complement train_size unless both are specified.FutureWarning)
train_X.shape
(712, 20)
test_X.shape
(179, 20)
选择机器学习算法
线性回归算法
from sklearn.linear_model import LogisticRegression
model = LogisticRegression()
训练模型
model.fit(train_X, train_y)LogisticRegression(C=1.0, class_weight=None, dual=False, fit_intercept=True,intercept_scaling=1, max_iter=100, multi_class='warn',n_jobs=None, penalty='l2', random_state=None, solver='warn',tol=0.0001, verbose=0, warm_start=False)
评估模型
model.score(test_X, test_y)
0.8212290502793296
方案实施
pred_Y = model.predict(test_data_X)
pred_Y
array([0, 1, 0, 0, 1, 0, 1, 0, 1, 0, 0, 0, 1, 0, 1, 1, 0, 0, 1, 1, 0, 1,1, 0, 1, 0, 1, 0, 0, 0, 0, 0, 1, 1, 1, 0, 1, 1, 0, 0, 0, 0, 0, 1,1, 0, 0, 0, 1, 1, 0, 0, 1, 1, 0, 0, 0, 0, 0, 1, 0, 0, 0, 1, 1, 1,1, 0, 1, 1, 1, 0, 1, 1, 1, 1, 0, 1, 0, 1, 1, 0, 0, 0, 0, 0, 1, 1,1, 1, 1, 0, 1, 0, 0, 0, 1, 0, 1, 0, 1, 0, 0, 0, 1, 0, 0, 0, 0, 0,0, 1, 1, 1, 1, 0, 0, 1, 1, 1, 1, 0, 1, 0, 0, 1, 0, 1, 0, 0, 0, 1,0, 0, 0, 0, 0, 0, 1, 0, 0, 1, 0, 0, 0, 0, 0, 0, 0, 0, 1, 0, 0, 1,0, 0, 1, 1, 0, 1, 1, 1, 1, 0, 0, 1, 0, 0, 1, 1, 0, 0, 0, 0, 0, 1,1, 0, 1, 1, 0, 0, 1, 0, 1, 0, 1, 0, 0, 0, 0, 0, 1, 0, 1, 0, 1, 1,0, 1, 1, 1, 1, 1, 0, 0, 1, 0, 1, 0, 0, 0, 0, 1, 0, 0, 1, 0, 1, 0,1, 0, 1, 0, 1, 1, 0, 1, 0, 0, 0, 1, 0, 0, 0, 0, 0, 0, 1, 1, 1, 1,0, 0, 1, 0, 1, 0, 1, 1, 1, 0, 1, 0, 0, 0, 0, 0, 1, 0, 0, 0, 1, 1,0, 0, 0, 0, 1, 0, 0, 0, 1, 1, 0, 1, 0, 0, 0, 0, 1, 1, 1, 1, 1, 0,0, 0, 0, 0, 0, 1, 0, 0, 0, 0, 1, 0, 0, 0, 0, 0, 0, 0, 1, 1, 0, 1,0, 1, 0, 0, 0, 1, 1, 1, 0, 0, 0, 0, 0, 0, 0, 0, 1, 0, 1, 0, 0, 0,1, 1, 0, 1, 0, 0, 0, 0, 0, 1, 0, 0, 0, 1, 0, 1, 0, 1, 0, 1, 1, 0,0, 0, 1, 0, 1, 0, 0, 1, 0, 1, 1, 0, 1, 0, 0, 1, 1, 0, 0, 1, 0, 0,1, 1, 1, 0, 0, 0, 0, 0, 1, 1, 0, 1, 0, 0, 0, 0, 1, 1, 1, 0, 0, 1,0, 1, 0, 0, 1, 0, 1, 0, 0, 0, 0, 0, 1, 1, 1, 1, 1, 0, 1, 0, 0, 1])