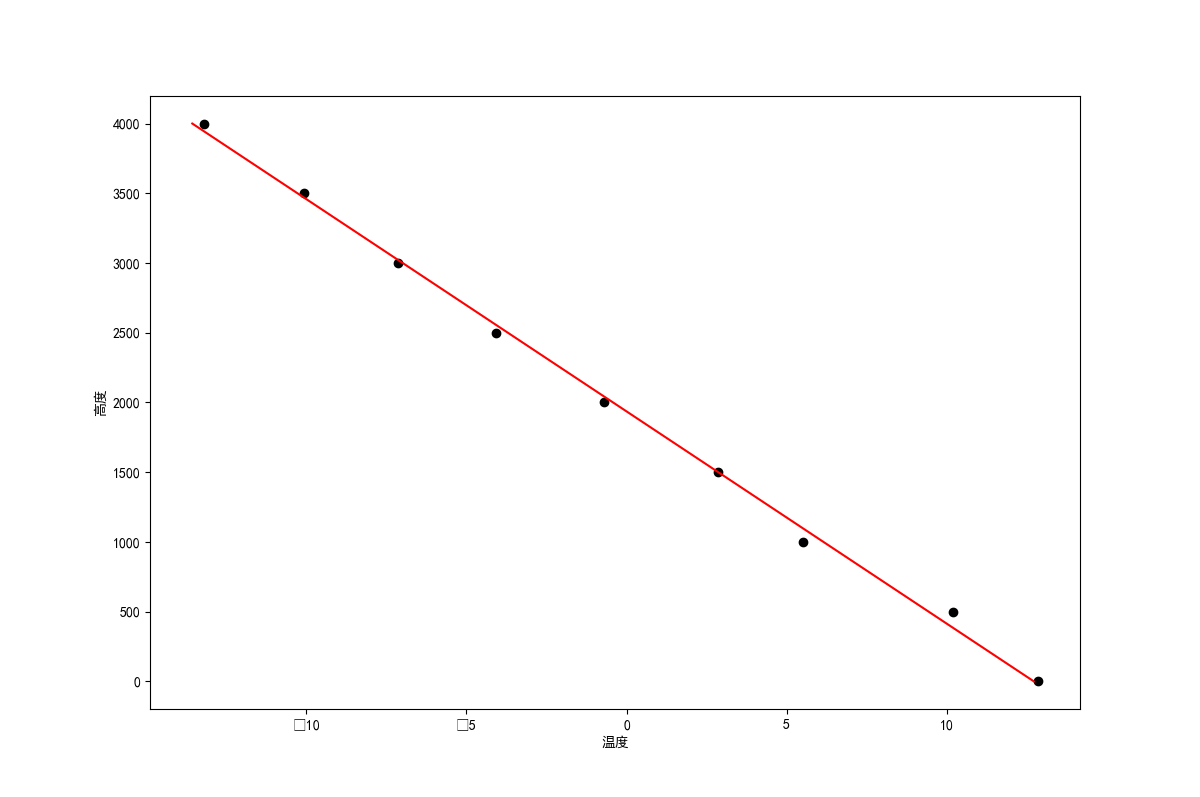
背景资料
随着海拔高度的上升,温度越来越低,经过气象专家的研究,在一定的海拔高度范围内,高度和温度呈线性关系。现有一组实测资料,我们需要对这些数据进行处理拟合,获得此线性关系。
解决思路
采用sklearn库中的LinearRegression线性回归类进行拟合。
代码
# 导入所需的模块
import numpy as np
import matplotlib.pyplot as plt
from sklearn.linear_model import LinearRegression# 已有数据
height = [0.0, 500.0, 1000.0, 1500.0, 2000.0, 2500.0, 3000.0, 3500.0, 4000.0]
temperature = [12.834044009405147, 10.190648986884316, 5.50022874963469, 2.8546651452636795, -0.7064882183657739, -4.065322810462405, -7.1274795772446575, -10.058878545913904, -13.206465051538661]# 数据处理
# sklearn 拟合输入输出一般都是二维数组,这里将一维转换为二维。
height = np.array(height).reshape(-1, 1)
temp = np.array(temperature).reshape(-1, 1)# 拟合
reg = LinearRegression()
reg.fit(height, temp)
a = reg.coef_[0][0] # 系数
b = reg.intercept_[0] # 截距
print('拟合的方程为:Y = %.6fX + %.6f' % (a, b))
# out: 拟合的方程为:Y = -0.006570X + 12.718507# 可视化
prediction = reg.predict(height) # 根据高度,按照拟合的曲线预测温度值
plt.figure('海拔高度~温度关系曲线拟合结果', figsize=(12,8))
plt.rcParams['font.family'] = ['sans-serif'] # 设置matplotlib 显示中文
plt.rcParams['font.sans-serif'] = ['SimHei'] # 设置matplotlib 显示中文
plt.xlabel('温度')
plt.ylabel('高度')
plt.scatter(temp, height, c='black')
plt.plot(prediction, height, c='r')
plt.show()
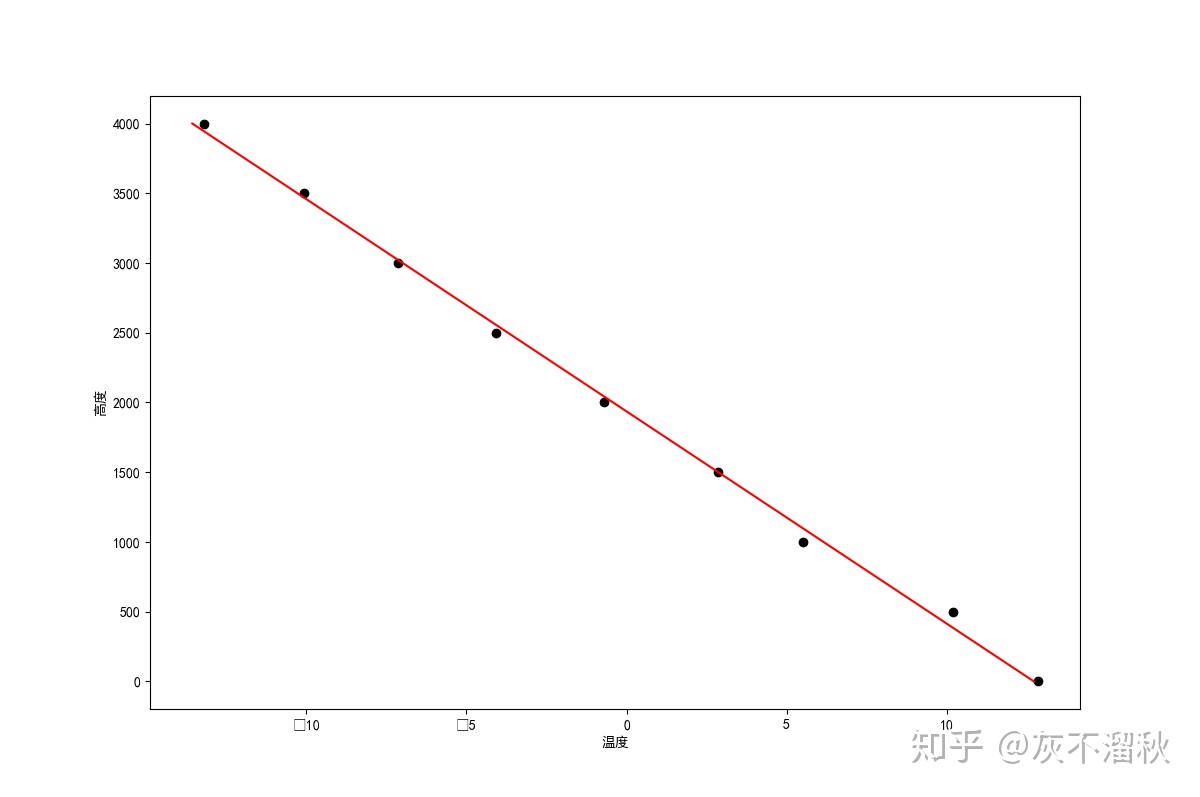